- Department of Medical Ultrasound, Maoming People’s Hospital, Maoming, Guangdong, China
Background: The purpose of this systematic review and meta-analysis is to evaluate the potential significance of radiomics, derived from preoperative magnetic resonance imaging (MRI), in detecting deep stromal invasion (DOI), lymphatic vascular space invasion (LVSI) and lymph node metastasis (LNM) in cervical cancer (CC).
Methods: A rigorous and systematic evaluation was conducted on radiomics studies pertaining to CC, published in the PubMed database prior to March 2024. The area under the curve (AUC), sensitivity, and specificity of each study were separately extracted to evaluate the performance of preoperative MRI radiomics in predicting DOI, LVSI, and LNM of CC.
Results: A total of 4, 7, and 12 studies were included in the meta-analysis of DOI, LVSI, and LNM, respectively. The overall AUC, sensitivity, and specificity of preoperative MRI models in predicting DOI, LVSI, and LNM were 0.90, 0.83 (95% confidence interval [CI], 0.75-0.89) and 0.83 (95% CI, 0.74-0.90); 0.85, 0.80 (95% CI, 0.73-0.86) and 0.75 (95% CI, 0.66-0.82); 0.86, 0.79 (95% CI, 0.74-0.83) and 0.80 (95% CI, 0.77-0.83), respectively.
Conclusion: MRI radiomics has demonstrated considerable potential in predicting DOI, LVSI, and LNM in CC, positioning it as a valuable tool for preoperative precision evaluation in CC patients.
1 Introduction
Uterine cancer remains a prevalent public health challenge that poses a significant threat to women’s well-being globally. The latest cancer statistics in the United States anticipate 66,200 new cases in 2023, ranking third among gynecological malignancies, with an expected 13,030 fatalities, ranking sixth (1). The most common types of uterine cancer are endometrial cancer (EC) and cervical cancer (CC). Deep stromal invasion (DOI), lymphatic vascular space invasion (LVSI) and lymph node metastasis (LNM) are pivotal factors that influence preoperative therapeutic planning and the determination of postoperative adjuvant treatment strategies for uterine cancer. Specifically, DOI is defined as stromal invasion depth exceeding one-third of the myometrial thickness, including parametrial invasion. For example, the expert consensus meetings divided uterine cancer into four risk levels based on histology, grade, stage, and the presence of LVSI, aiming to reflect the likelihood of tumor invasion and recurrence and, thereby guiding potential adjuvant therapy (2, 3). Notably, patients without DOI could choose less aggressive surgery to mitigate perioperative and postoperative complications. Conversely, those suspected of having DOI often require combined chemoradiotherapy following surgery (4). Patients with LVSI positive uterine cancer may still have distant metastasis despite receiving adjuvant treatment (5). A study involving 368 uterine cancer patients revealed that 70% of them underwent unnecessary lymphadenectomy, even among high-risk populations, such as those with tumors larger than 2 cm and evidence of DOI (6). In this context, DOI, LVSI, and LNM determined the patient’s surgical plan and choice of adjuvant therapy through precise preoperative evaluation of the uterine cancer.
Magnetic resonance imaging (MRI) serves as a pivotal tool for preoperative evaluation of uterine cancer. Preoperatively, MRI can facilitate the identification of DOI. A study revealed that a radiologist with 7 years of experience in gynecological cancer imaging achieved a diagnostic sensitivity (SENC) of 70%, while a radiologist with 4 years of experience only attained a SENC of 50% (7). However, microscopic parametric invasion could be detected in approximately 32–36% of IB2/IIA patients, which often fell within a microscopic field that may be undetectable by radiologists (8). LVSI primarily involves microscopic-level analysis, which poses challenges for visual evaluation. Although MRI utilizing quantitative apparent diffusion coefficient has been explored for LVSI assessment, the results have been unsatisfactory (9). In assessing LNM, radiologists primarily rely on visual imaging to analyze the size, morphology, internal structure, and enhancement pattern of lymph nodes (10). For example, swollen lymph nodes, with a nearly circular shape, and irregular edges were common visual signs that were suspected of metastasis. However, similar visual characteristics can also be attributed to inflammatory changes, and postoperative pathological analysis reveals that only 50% of such lymph nodes are truly metastatic (11). Furthermore, some scholars had calculated that the SENC of preoperative MRI for normal sized LNM was only 0.59, with the area under the curve (AUC) of 0.70. Puncture pathology is an important tool of preoperative acquisition for DOI, LVSI, and LNM. However, since puncture is an invasive method, it may not only lead to complications such as tumor dissemination, but also increase the fear and resistance of patients. In addition, due to tumor heterogeneity, biopsy results may yield false-negative outcomes (12, 13). This underscores the urgent need to transcend visual limitations and harness novel technologies that capture more comprehensive tumor image information to achieve precise preoperative evaluation of uterine cancer.
Accurate preoperative identification of DOI or LVSI or LNM in patients with uterine cancer holds significant implications for treatment management, effectively averting the pitfalls of over- and under-treatment. Radiomics (R), an artificial intelligence (AI) technology, transcends the limitations of visual inspection by leveraging computers to quantify microscopic image features, thereby enhancing the utilization of image information and advancing towards precision medicine. Compared to traditional imaging diagnosis, R is capable of reflecting tumor biological characteristics in a more comprehensive and detailed manner, while being unaffected by specimen size, location, or quality, thus better capturing tumor heterogeneitym, including molecular genetic alterations. R has garnered widespread application in preoperative risk assessment of uterine cancer (EC and CC). For example, the R model developed based on T2WI and DWI images had been validated to have SENC and specificity (SPEC) of 0.60 and 0.96 for predicting the LVSI state of early CC (14); the R model based on MRI images of 339 EC patients from 5 centers was developed to predict LVSI with SENC and SPEC of 0.92 and 0.74, respectively (15); the SENC and SPEC of the R model based on enhanced T1WI and T2WI images in predicting the LNM state of early CC were validated to be 0.71 and 0.72, respectively (16). While R has exhibited superior detection performance compared to clinical models (C), the integration of radiomics-clinical models (R-C) offers even greater clinical benefits. For example, the nomogram jointly developed based on R features, MR reported LN status, and the International Federation of Gynecology and Obstetrics (FIGO) stage was superior to both single R model and C model (17). Based on these findings, the predictive potential of R in forecasting DOI, LVSI, and LNM of EC was further validated through meta-analysis. For example, a meta-analysis encompassing 15 studies revealed that the overall SENC and SPEC of R for predicting DOI, LVSI, and LNM were 0.74 and 0.82; 0.66 and 0.75; 0.78 and 0.81, respectively (18). However, only a meta-analysis for CC in terms of LNM has been reported, necessitating further analysis for CC in DOI and LVSI. Additionally, the potential value of R-C models remains unexplored, requiring further meta-analysis to uncover its promise.
The purpose of this systematic review and meta-analysis was to evaluate the considerable potential of R developed from preoperative MRI, in detecting DOI or LVSI or LNM in CC. In addition, the potential based on C, and R-C models in CC was also analyzed, thereby providing insights into the efficacy of these approaches in improving diagnostic accuracy and clinical decision-making.
2 Methods
2.1 Search scheme
A systematic search was undertaken in the PubMed database, encompassing original studies published up to March 31, 2024. The search was guided by a set of keywords including “Radiomics”, “Texture”, “Cervical cancer”, “Parametrial invasion”, “Stromal invasion”, “Lymphovascular space invasion”, “Lymphatic vascular space invasion”, “LVSI”, “Lymph node metastasis”, and “LNM”. Two reviewers with more than 3 years of experience in abdominal imaging diagnosis independently reviewed the original study of preoperative R, including the study abstract and full text. Discrepancies between reviewers were resolved by consensus or, in cases of persistence, by a third reviewer with over five years of abdominal imaging diagnosis expertise. In order to compare the performance of different models, R, C, and R-C models were included separately. It is noteworthy that the C model was exclusively incorporated in studies that had an underlying R cohort.
2.2 Study selection
Inclusion criteria for literature selection: (1) original MRI-based R/texture feature analysis studies. (2) patients with CC confirmed by histopathological examination. (3) preoperative prediction for DOI or LVSI or LNM. (4) availability of data, including true positive (TP), false positive (FP), true negative (TN) and false negative (FN), for calculation purposes.
The exclusion criteria were as follows: (1) R studies based on non CC lesion images. (2) R studies using CT, PET/CT, and ultrasound (US). (3) comments, meta-analyses, case reports, guidelines or errata, repeated studies. (4) postoperative R studies. (5) studies involving preoperative anti-tumor treatment; (6) deep learning studies; (7) Radiomics Quality Score (RQS) of 10 or below.
2.3 Data extraction
The literature data were extracted from the original studies: (1) basic characteristics, such as the author, publication year, country, and study design. (2) cohort characteristics, including the cohort type, sample size, and the population specific to DOI or LVSI or LNM. (3) image characteristics, comprising the image protocol, image segmentation, R extraction software, feature selection strategy, and model algorithms. (4) evaluation indicators, namely the AUC, SENC, SPEC, and the Delong test. The numbers of TP, TN, FP, and FN were calculated according to the SENC and SPEC in each study report, referred to the formula: SENC=TP/(TP+FN), SPEC=TN/(FP+TN). In cases where multiple models were based on the same cohort, the model with superior performance was included.
2.4 Study quality assessment
The RQS was used to evaluate R quality, which was an important tool to measure the rigor of artificial intelligence (AI) study. RQS included 16 evaluation indexes, covering aspects such as image acquisition, image preprocessing, validation, performance evaluation, practicality, open science (19). Additionally, the Quality Assessment of Diagnostic Accuracy Studies (QUADAS-2) also was utilized to evaluate the methodological quality, including: (1) patient selection, (2) index test, (3) reference standard, and (4) flow and timing. The risk of bias in each category was classified as low, high, or unclear. A modified version of QUADAS-2 proposed by Sollini et al. and validated by Bedrikovetski S et al. was utilized (20, 21).
2.5 Statistical analysis
Using the Stata software (version 12.0), a comprehensive analysis was conducted to summarize and calculate the TP, TN, FP, and FN. Forest plots were generated to visually represent the overall SENC and SPEC across studies. The Cochrane diagnostic test and I2 statistic were employed to evaluate heterogeneity among the studies, with I2 values exceeding 50% indicating high heterogeneity (22). Deek’s funnel plots were utilized to evaluate whether the analysis was subject to publication bias. The summary receiver operating characteristic (sROC) curve demonstrated the predictive potential of R studies. MetaDiSc software was utilized for subgroup analysis (23). A statistical significance level of P < 0.05 was set for all analyses.
3 Results
3.1 Results of search scheme
The flowchart depicting search scheme was presented in Figure 1. Among the initial 105 studies retrieved, 57 studies were excluded due to their irrelevance to the current review. A further 12 studies were eliminated as they failed to provide data on TP, TN, FP, and FN, leaving 48 studies for consideration. Out of the remaining 36 studies that met the requirements, the following studies were further excluded: 2 MRI studies based on deep learning algorithms (24, 25); 3 studies based on PET/CT images (26–28), one study based on CT images (29), and 2 studies based on US images (30, 31); 3 studies on predicting the LNM status of CC primary lesions based on lymph node imaging (32–34). Ultimately, 25 studies were selected for analysis. Among these, 1 study was utilized to predict both DOI and LNM (35), while another study was utilized to predict both LVSI and LNM (36). Consequently, 5, 8, and 14 MRI R studies were included in the meta-analysis of DOI (14, 35, 37–39), LVSI (36, 40–46), and LNM (16, 17, 35, 36, 47–56), respectively.
3.2 Study quality assessment
All the included studies exhibited a retrospective design, and only five of them utilized multicenter data. According to the first author’s affiliation, 24 studies were conducted in China, while the remaining one were from Japan. A total of 24 studies provided details on cohort selection criteria and image protocols. Regarding image acquisition, 19 studies were relied a single scanner (4 GE, 6 Philips, and 9 Siemens), 3 studies were utilized two scanners (GE, Philips, and Siemens), and 3 studies remained unclear about the scanner used. For image segmentation, 8 studies employed a single sequence segmentation, 10 studies used two sequence, and 7 studies utilized three or more sequence. 23 studies adopted multi person segmentation, while 13 studies focused on the robustness of intrahepatic cholangiocarcinoma (ICC) validation features. The primary segmentation software included ITK-SNAP and 3D slicer, and 24 studies performed manual segmentation. Prior to feature extraction, 15 studies underwent image normalization processing. Feature extraction was mainly based on the Pyradiomics software package, with 19 study extracting over 1000 features, significantly exceeding the cohort population. All studies employed multi-step feature selection strategies, among which 10 sutides utilized logistic regression (LR), 7 sutides utilized support vector machine (SVM), and 6 sutides utilized least absolute shrinkage and selection operator (LASSO) algorithm to construct models. In terms of model evaluation, 13 studies opted for cross-validation to obtain optimal results, while 7 studies compared model performance using the Delong test. 24 studies conducted internal or external validation of the models. 14 studies performed multi-factor analysis on clinical or R features, and 21 studies constructed R-C models. 15 studies underwent model calibration, and 12 studies reported decision curve results.
The methodological quality of the studies was rigorously evaluated by RQS and QUADAS-2 tools. The results indicated varied methodological quality across the studies, with RQS scores for DOI, LVSI, and LNM ranging from 5–17, 2–17, and 2–17, respectively. Consequently, two studies with RQS scores below 10 were deemed unfit for inclusion and were excluded from further analysis (35, 36). The QUADAS-2 evaluation revealed that in terms of patient selection, 24 studies displayed a low risk of bias, while only 1 study exhibited a higher risk. Similarly, in the index test category, 24 studies demonstrated a low risk of bias, with 1 study identified as having a higher risk. The reference standard test showed a consistently low risk in 25 studies. However, regarding the flow and timing of the studies, the risk of bias remained unclear in all 25 studies.
Finally, 4, 7, and 12 studies were included in the meta-analysis for DOI, LVSI, and LNM, respectively (Table 1). The RQS scores in these studies ranges from 12–17, 13–17, and 11–17, with an average scores of 13.5, 14.9, and 13.8, respectively (Figure 2). Notably, only one study in the patient selection test and one in the index test category were classified as high-risk (Figure 3).
3.3 Preoperative MRI radiomics models for predicting DOI in CC
Table 2 summarized the basic characteristics of 4 studies aimed at predicting DOI in CC. These studies encompassed a total of 8 cohorts, comprising 839 CC patients, including 305 patients with DOI and 534 patients without DOI. The AUC, SENC, and SPEC of all cohorts ranged from 0.83–0.95, 0.60–0.93, and 0.67–0.96, respectively. Furthermore, 3 studies incorporated both R and C models, as well as their R-C models (Table 3). The results indicated that the overall SENC and SPEC of R, C, R-C models were 0.83 (95% confidence interval [CI], 0.75–0.89) and 0.83 (95% CI, 0.74–0.90); 0.79 (95% CI, 0.70–0.86) and 0.72 (95% CI, 0.65–0.78); 0.91 (95% CI, 0.86–0.94) and 0.84 (95% CI, 0.73–0.91), respectively. Notably, significant heterogeneity was observed in both overall SENC (I2 = 54.74%, P=0.03) and SPEC (I2 = 82.34%, P <0.01) of R models. Through sROC curve analysis, the overall AUC of R, C, R-C models were 0.90, 0.82, 0.94, respectively, indicating superior evaluation performance (Figure 4A). Deek’s funnel plots were utilized to detect publication bias in the R models, and the results indicated the absence of such bias (t=0.36, P=0.73) (Figure 4B).
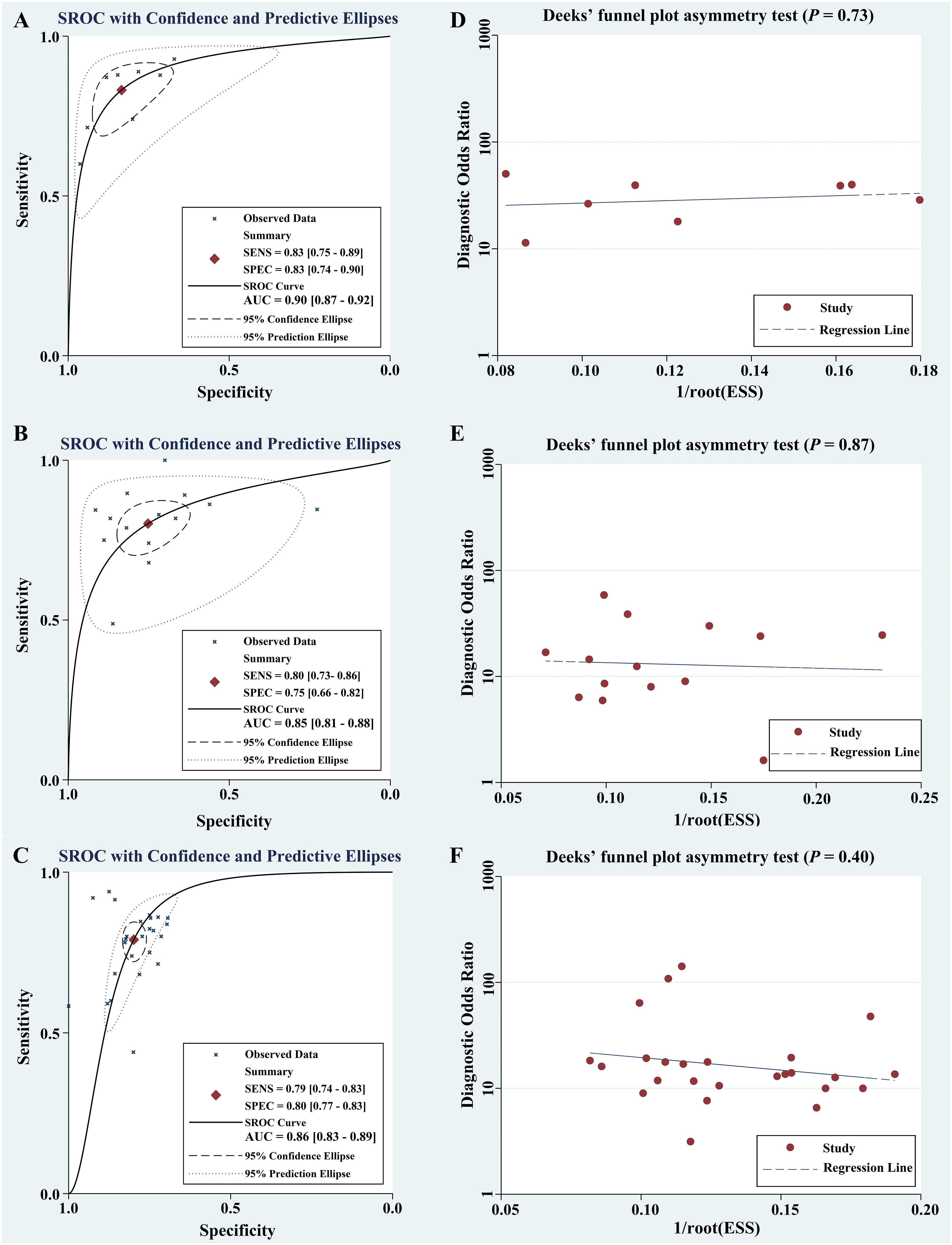
Figure 4 Comprehensive potential evaluation in CC based on preoperative MRI radiomics. Through sROC curve analysis, the meta-analyses based on DOI (A), LVSI (B), and LNM (C) showed overall AUC of 0.90, 0.85, and 0.86, respectively. The presence of publication bias was detected by Deek’s funnel plots, and the results showed no publication bias [DOI, P=0.73, (D);LVSI, P=0.87, (E); LNM, P=0.40, (F)].
A subgroup analysis of the R models based on cohort type revealed no significant difference in the overall AUC between the training and validation cohorts (AUC, 0.90 vs 0.91). However, the overall SENC of the validation cohorts was slightly higher, while the overall SPEC was slightly lower (Table 4).
3.4 Preoperative MRI radiomics models for predicting LVSI in CC
Table 5 summarized the basic characteristics of 7 studies aimed at predicting LVSI in CC. These studies encompassed a total of 14 cohorts, comprising 1243 CC patients, including 577 patients with LVSI and 666 patients without LVSI. The AUC, SENC, and SPEC of all cohorts ranged from 0.63–0.94, 0.49–1.00, and 0.23–0.91, respectively. Furthermore, 3 studies focused solely on C models, while 5 studies integrated R-C models into their analysis (Table 3). The results indicated that the overall SENC and SPEC of R, C, R-C models were 0.80 (95% CI, 0.73-0.86) and 0.75 (95% CI, 0.66–0.82); 0.52 (95% CI, 0.42–0.62) and 0.92 (95% CI, 0.81–0.97); 0.84 (95% CI, 0.79–0.87) and 0.82 (95% CI, 0.78–0.85), respectively. Notably, significant heterogeneity was observed in both overall SENC (I2 = 65.90%, P<0.01) and SPEC (I2 = 81.10%, P<0.01) of R models. Through sROC curve analysis, the overall AUC of R, C, R-C models were 0.85, 0.67, 0.89, respectively, indicating superior evaluation performance (Figure 4C). The existence of publication bias of R models was detected by Deek’s funnel plots were utilized to detect publication bias in the R models, and the results indicated the absence of such bias (t=-0.16, P=0.87) (Figure 4D).
A subgroup analysis of R models was conducted, considering factors such as cohort type, number of centers, scanner type, sequence number, model algorithm, and cross-validation. The findings revealed that no significant difference in the overall AUC between the training and validation cohorts (AUC, 0.86 vs 0.85). Interestingly, studies involving multiple centers demonstrated a lower overall AUC than those from a single center, yet they exhibited higher SENC. When analyzing scanner types, a single Siemens scanner yielded an AUC of 0.84, indicating moderate performance. The sequence number and algorithm types did not seem to significantly impact performance. Notably, cross-validated studies exhibited superior performance, with an AUC of 0.92 compared to 0.81 (Table 4).
3.5 Preoperative MRI radiomics models for predicting LNM in CC
Table 6 summarized the basic characteristics of 12 studies aimed at predicting LNM in CC. These studies encompassed a total of 25 cohorts, comprising 2004 CC patients, including 631patients with LNM and 1373 patients without LNM. The AUC, SENC, and SPEC of all cohorts ranged from 0.70–0.98, 0.44–0.94, and 0.69–1.00, respectively. Furthermore, 7 studies focused solely on C models, while 8 studies integrated R-C models into their analysis (Table 3). The results indicated that the overall SENC and SPEC of R, C, R-C models were 0.79 (95% CI, 0.74-0.83) and 0.80 (95% CI, 0.77-0.83); 0.69 (95% CI, 0.62-0.74) and 0.79 (95% CI, 0.70-0.86); 0.84 (95% CI, 0.80-0.88) and 0.84 (95% CI, 0.79-0.89), respectively. Notably, significant heterogeneity was observed in both overall SENC (I2 = 50.67%, P<0.01) and SPEC (I2 = 36.24%, P=0.04) of R models. Through sROC curve analysis, the overall AUC of R, C, R-C models were 0.86, 0.76, 0.91, respectively, indicating superior evaluation performance (Figure 4E, Table 7). Deek’s funnel plots were utilized to detect publication bias in the R models, and the results indicated the absence of such bias (t=-0.87, P=0.40) (Figure 4F).
A thorough subgroup analysis was performed on R models, encompassing factors such as cohort type, center number, scanner type, sequence number, model algorithm, and cross-validation. The findings indicate a marginal difference in performance between the training and validation cohorts, with AUC values of 0.87 and 0.84, respectively. The number of centers did not significantly influence performance, with AUC values 0.87 and 0.86. Similarly, Philips and Siemens scanners displayed comparable performance, with AUCs of 0.87 and 0.85, respectively. Additionally, the sequence number, algorithm types, and cross-validation appeared to have minimal impact on the models’ overall performance (Table 4).
3.6 Meta-analysis investigation of preoperative radiomics in uterine cancer
The meta-analysis investigating preoperative MRI R models for uterine cancer was summarized in Table 8. To our knowledge, no previous meta-analysis had been found to predict DOI and LVSI in CC. 2 meta-analyses were found for LNM prediction in CC (57, 58), reporting overall SENC and SPEC of 0.80 and 0.76; 0.84 and 0.73, respectively. Additionally, 2 meta-analyses were simultaneously found for DOI, LVSI and LNM prediction in EC (18, 59). 1 meta-analyses were solely found for LVSI prediction in EC (60).
4 Discussion
4.1 Principal findings
In comparison to traditional MRI visual imaging, R base on MRI images harnesses a broader range of image information to assess preoperative biological characteristics of uterine cancer, encompassing DOI, LVSI, and LNM. To our knowledge, this systematic review and meta-analysis was the first comprehensive analysis of preoperative R, C, and R-C models in predicting DOI, LVSI, and LNM, aiming to elucidate the potential of R in preoperative evaluation of CC biological characteristics. The meta-analysis results demonstrated satisfactory diagnostic accuracy. For the meta-analysis of DOI, the results based on R, C, and R-C showed overall SENS, SPEC, and AUC of 0.83, 0.83, and 0.90; 0.79, 0.72, and 0.82; 0.91, 0.84, and 0.94, respectively. In the meta-analysis of LVSI, the corresponding values for R, C, and R-C models were 0.80, 0.75, and 0.85; 0.52, 0.92, and 0.67; 0.84, 0.82, and 0.89, respectively. Finally, for LNM, the corresponding values for R, C, and R-C models were 0.79, 0.80, and 0.86; 0.69, 0.79, and 0.76; 0.84, 0.84, and 0.91, respectively. Compared to the R models, the R-C models exhibited a marginal improvement in performance, while significantly outperforming C models. Although R had shown great potential in predicting CC biological characteristics, it also exhibited high heterogeneity in meta-analyses, with RQS scores indicating a higher methodological quality risk, similar to other R meta-analyses. This highlights urgent need for the development of the more reliable methodological quality standardization process in R.
R, analogous to gene sequencing, quantifies the high-throughput tumor information embedded in medical images, thereby enhancing the utilization of image data in clinical decision-making. This approach has the potential to address clinical challenges that traditional qualitative imaging diagnosis cannot, approaching the explanatory power of genomics in diseases. The application of MRI-based R has been extensively explored in elucidating the biological characteristics of uterine cancer, with over a hundred publications, particularly in EC. In a meta-analysis encompassing 33 preoperative studies, R demonstrated promising SENC and SPEC in predicting DOI, LVSI, and LNM were 0.75 and 0.81; 0.76 and 0.76; 0.87 and 0.80, respectively (56); a separate meta-analysis based on five studies revealed similar trends, with SENC and SPEC values of 0.74 and 0.82; 0.66 and 0.75; 0.83 and 0.74, respectively (18). However, in the context of CC, meta-analytic reports in 2022 appeared to be limited to predictions of LNM. For example, scholars such as Li L conducted a meta-analysis based on 12 studies, revealing a predictive performance of 0.83 for preoperative LNM (57); Ren J and other scholars conducted a meta-analysis based on 8 studies, reported a predictive performance of 0.86 for preoperative LNM using image-based R (58). Notably, there was a significant overlap between the studies included in these two meta-analyses, suggesting a quantitative expansion rather than qualitative advancement. Therefore, the predictive potential of MRI-R for DOI and LVSI in CC remains an area requiring further exploration and elucidation.
Despite its promise, R encounters notable challenges in the standardization and assessment of methodological quality. Some scholars had proposed that R need to establish rigorous evaluation and reporting standards, namely RQS. However, in practice, the RQS scores in various studies tend to be relatively low. For example, an analysis encompassing 33 studies on EC revealed an average RQS of 7 points, ranging from 5 to 12 (59). In a CC meta-analysis of 8 studies, the mean RQS was 13.5, spanning from 6 to 16 points (58). The average RQS for DOI, LVSI, and LNM in this review were 13.5, 14.9, and 13.8, respectively, aligning with findings from other retrospective studies. According to the results of the QUADAS-2 tool, only one study exhibited a high risk in the index test, while the remainder presented low risks in patient selection and reference standard. However, both the flow and timing tests showed unclear risk levels, a trend consistent with other meta-analyses (61). The findings of this review and other evaluations suggest that the RQS does not fully capture the quality of study design in its current application, necessitating a more comprehensive and standardized evaluation process.
In this review, for the first time, the potential of R in predicting the biological characteristics of CC was comprehensively analyzed based on R, C, and R-C models. To ensure the reliability of the conclusions, methodological quality assessment was utilized to exclude low-quality studies. Although the number of studies included was small, the results showed satisfaction. In the comprehensive analysis of DOI, LVSI, and LNM, the R models performed better than the C model, slightly lower than the R-C models. Both R and R-C models exhibit moderate to superior performance. However, the comprehensive analysis of R revealed a high degree of heterogeneity. Drawing parallels from other meta-analyses, this heterogeneity is closely associated with factors such as cohort types, number of centers, scanner types, image protocols, number of sequences, algorithm variations, and the implementation of cross-validation. To further explore the sources of heterogeneity, this meta-analysis also conducted subgroup analysis on the sources of heterogeneity, and the results showed that in the DOI analysis, there seemed to be no significant difference in overall performance between cohorts. The SENC of the validation cohort was higher, but the SPEC was lower than that of the training cohort. In the analysis of LVSI, the validation cohorts seemed to outperform the training cohort, with higher SENC. The number of centers and algorithm types did not significantly influence performance, while cross-validation appeared to enhance predictive capabilities. In the LNM analysis, the performance between cohorts was comparable, with the training cohort demonstrating higher SENC and the validation cohorts exhibiting higher SPEC. The number of centers, number of sequences, algorithm types, and cross-validation did not appear to have a significant impact on predictive performance. This subgroup analysis, based on the overall AUC, did not significantly affect the analysis of DOI and LNM, while the analysis of LVSI seemed to be influenced by cohort type and cross validation.
4.2 Practical implications
The preoperative biological behavior assessment of CC patients, such as DOI, LVSI, and LNM, was beneficial for clinical decision-making. In practice, traditional MRI imaging mainly relied on visual information to evaluate the biological behavior of CC patients. However, the limitations of visual information, differences in equipment, and the long-term cultivation of visual experience by physicians made it difficult to meet the needs of precision medicine in terms of evaluation results. In addition, clinical information also could not effectively evaluate the biological behavior of CC patients. In summary, more effective and timely methods are needed for preoperative biological evaluation of CC patients. The R model based on AI broke through the limitations of the naked eye and greatly improved the utilization of MRI image information. Its prediction of DOI, LVSI, and LNM in CC patients was satisfactory, surpassing the level of clinical doctors. As is well known, developing countries, such as China, often lag behind developed countries in the cultivation of medical equipment and medical talents. The demand and supply of healthcare are severely imbalanced. In the future, AI models entering clinical practice can serve as an auxiliary tool for preoperative risk stratification in CC patients, improving the diagnostic efficiency of radiologists, avoiding the harm caused by pathological examinations, reducing medical burden, providing greater medical supply, and easing doctor-patient conflicts. However, the differences in imaging equipment and the robustness of AI model construction pose serious challenges to the sustainability of AI entering clinical practice. At present, developing countries need to invest more computer equipment and scientific research to further optimize and update AI technology, enhance AI computing intelligence, and overcome various problems faced by equipment and model development processes.
4.3 Limitations
This review encountered several limitations that were noteworthy (1): the relatively small number of studies included for DOI and LVSI evaluations could not entirely exclude the possibility of overzealous exclusion during the literature screening process. Consequently, the generalizability of the meta-analysis results remained a pertinent discussion topic. (2) the meta-analysis results revealed that the overall SENS and SPEC were highly heterogeneous. This heterogeneity primarily stemmed from the lack of standardization in scientific rigor and clinical relevance. (3) The use of the Risk of Bias in RQS and QUADAS-2 tool had evaluation limitations, resulting in controversial interpretations. (4) All studies were retrospective studies, lacking the validation of AI’s effectiveness through high-quality, multicenter, prospective studies.
5 Conclusions
This system review and meta-analysis indicates that preoperative R based on MRI images can effectively evaluate the DOI, LVSI, and LNM status in uterine cancer. Moreover, R combined with C factors can improve evaluation performance. However, it is acknowledged that the current research on risk assessment is heterogeneous and thus requires further development and refinement to achieve the goal of facilitating preoperative clinical diagnosis and treatment decisions with precision.
Data availability statement
The original contributions presented in the study are included in the article/Supplementary Material. Further inquiries can be directed to the corresponding author.
Author contributions
LW: Conceptualization, Investigation, Methodology, Project administration, Software, Visualization, Funding acquisition, Writing – original draft, Writing – review & editing. SoL: Investigation, Methodology, Software, Visualization, Writing – original draft, Writing – review & editing. ShL: Data curation, Formal analysis, Methodology, Validation, Writing – original draft, Writing – review & editing. YL: Investigation, Methodology, Software, Validation, Visualization, Writing – original draft, Writing – review & editing. DW: Data curation, Project administration, Resources, Supervision, Writing – original draft, Writing – review & editing.
Funding
The author(s) declare financial support was received for the research, authorship, and/or publication of this article. This study was funded by the Guangdong Medical Science and Technology Research Fund project, with funding number A2024269.
Conflict of interest
The authors declare that the research was conducted in the absence of any commercial or financial relationships that could be construed as a potential conflict of interest.
Publisher’s note
All claims expressed in this article are solely those of the authors and do not necessarily represent those of their affiliated organizations, or those of the publisher, the editors and the reviewers. Any product that may be evaluated in this article, or claim that may be made by its manufacturer, is not guaranteed or endorsed by the publisher.
Supplementary material
The Supplementary Material for this article can be found online at: https://www.frontiersin.org/articles/10.3389/fonc.2024.1416378/full#supplementary-material
References
1. Siegel RL, Miller KD, Wagle NS, Jemal A. Cancer statistics, 2023. CA Cancer J Clin. (2023) 73:17–48. doi: 10.3322/caac.21763
2. Celli V, Guerreri M, Pernazza A, Cuccu I, Palaia I, Tomao F, et al. MRI- and histologic-molecular-based radio-genomics nomogram for preoperative assessment of risk classes in endometrial cancer. Cancers (Basel). (2022) 14:5881. doi: 10.3390/cancers14235881
3. Mereu L, Pecorino B, Ferrara M, Tomaselli V, Scibilia G, Scollo P. Neoadjuvant chemotherapy plus radical surgery in locally advanced cervical cancer: retrospective single-center study. Cancers (Basel). (2023) 15:5207. doi: 10.3390/cancers15215207
4. Woo S, Kim SY, Cho JY, Kim SH. Apparent diffusion coefficient for prediction of parametrial invasion in cervical cancer: a critical evaluation based on stratification to a Likert scale using T2-weighted imaging. Radiol Med. (2018) 123:209–16. doi: 10.1007/s11547-017-0823-x
5. Tortorella L, Restaino S, Zannoni GF, Vizzielli G, Chiantera V, Cappuccio S, et al. Substantial lymph-vascular space invasion (LVSI) as predictor of distant relapse and poor prognosis in low-risk early-stage endometrial cancer. J Gynecol Oncol. (2021) 32:e11. doi: 10.3802/jgo.2021.32.e11
6. Karalok A, Turan T, Basaran D, Turkmen O, Comert Kimyon G, Tulunay G, et al. Lymph node metastasis in patients with endometrioid endometrial cancer: overtreatment is the main issue. Int J Gynecol Cancer. (2017) 27:748–53. doi: 10.1097/IGC.0000000000000937
7. Moloney F, Ryan D, Twomey M, Hewitt M, Barry J. Comparison of MRI and high-resolution transvaginal sonography for the local staging of cervical cancer. J Clin Ultrasound. (2016) 44:78–84. doi: 10.1002/jcu.22288
8. Park JY, Kim DY, Kim JH, Kim YM, Kim YT, Nam JH. Laparoscopic versus open radical hysterectomy in patients with stage IB2 and IIA2 cervical cancer. J Surg Oncol. (2013) 108:63–9. doi: 10.1002/jso.23347
9. Li S, Liu J, Zhang F, Yang M, Zhang Z, Liu J, et al. Novel T2 mapping for evaluating cervical cancer features by providing quantitative T2 maps and synthetic morphologic images: A preliminary study. J Magn Reson Imaging. (2020) 52:1859–69. doi: 10.1002/jmri.27297
10. Li H, Chen XL, Liu H, Lu T, Li ZL. MRI-based multiregional radiomics for predicting lymph nodes status and prognosis in patients with resectable rectal cancer. Front Oncol. (2023) 12:1087882. doi: 10.3389/fonc.2022.1087882
11. Adachi T, Eguchi S, Beppu T, Ueno S, Shiraishi M, Okuda K, et al. Prognostic impact of preoperative lymph node enlargement in intrahepatic cholangiocarcinoma: A multi-institutional study by the kyushu study group of liver surgery. Ann Surg Oncol. (2015) 22:2269–78. doi: 10.1245/s10434–014-4239–8
12. Li L, Wu C, Huang Y, Chen J, Ye D, Su Z, et al. Radiomics for the preoperative evaluation of microvascular invasion in hepatocellular carcinoma: A meta-analysis. Front Oncol. (2022) 12:831996. doi: 10.3389/fonc.2022.831996
13. Felfli M, Liu Y, Zerka F, Voyton C, Thinnes A, Jacques S, et al. Systematic review, meta-analysis and radiomics quality score assessment of CT radiomics-based models predicting tumor EGFR mutation status in patients with non-small-cell lung cancer. Int J Mol Sci. (2023) 24:11433. doi: 10.3390/ijms241411433
14. Wang T, Gao T, Guo H, Wang Y, Zhou X, Tian J, et al. Preoperative prediction of parametrial invasion in early-stage cervical cancer with MRI-based radiomics nomogram. Eur Radiol. (2020) 30:3585–93. doi: 10.1007/s00330–019-06655–1
15. Liu XF, Yan BC, Li Y, Ma FH, Qiang JW. Radiomics feature as a preoperative predictive of lymphovascular invasion in early-stage endometrial cancer: A multicenter study. Front Oncol. (2022) 12:966529. doi: 10.3389/fonc.2022.966529
16. Kan Y, Dong D, Zhang Y, Jiang W, Zhao N, Han L, et al. Radiomic signature as a predictive factor for lymph node metastasis in early-stage cervical cancer. J Magn Reson Imaging. (2019) 49:304–10. doi: 10.1002/jmri.26209
17. Xiao M, Ma F, Li Y, Li Y, Li M, Zhang G, et al. Multiparametric MRI-based radiomics nomogram for predicting lymph node metastasis in early-stage cervical cancer. J Magn Reson Imaging. (2020) 52:885–96. doi: 10.1002/jmri.27101
18. Di Donato V, Kontopantelis E, Cuccu I, Sgamba L, Golia D'Augè T, Pernazza A, et al. Magnetic resonance imaging-radiomics in endometrial cancer: a systematic review and meta-analysis. Int J Gynecol Cancer. (2023) 33:1070–6. doi: 10.1136/ijgc-2023–004313
19. Lambin P, Leijenaar RTH, Deist TM, Peerlings J, de Jong EEC, van Timmeren J, et al. Radiomics: the bridge between medical imaging and personalized medicine. Nat Rev Clin Oncol. (2017) 14:749–62. doi: 10.1038/nrclinonc.2017.141
20. Sollini M, Antunovic L, Chiti A, Kirienko M. Towards clinical application of image mining: a systematic review on artificial intelligence and radiomics. Eur J Nucl Med Mol Imaging. (2019) 46:2656–72. doi: 10.1007/s00259-019-04372-x
21. Bedrikovetski S, Dudi-Venkata NN, Kroon HM, Seow W, Vather R, Carneiro G, et al. Artificial intelligence for pre-operative lymph node staging in colorectal cancer: a systematic review and meta-analysis. BMC Cancer. (2021) 21:1058. doi: 10.1186/s12885-021-08773-w
22. Jia LL, Zhao JX, Pan NN, Shi LY, Zhao LP, Tian JH, et al. Artificial intelligence model on chest imaging to diagnose COVID-19 and other pneumonias: A systematic review and meta-analysis. Eur J Radiol Open. (2022) 9:100438. doi: 10.1016/j.ejro.2022.100438
23. Wang M, Mei T, Gong Y. The quality and clinical translation of radiomics studies based on MRI for predicting Ki-67 levels in patients with breast cancer. Br J Radiol. (2023) 96:20230172. doi: 10.1259/bjr.20230172
24. Wu Q, Wang S, Zhang S, Wang M, Ding Y, Fang J, et al. Development of a deep learning model to identify lymph node metastasis on magnetic resonance imaging in patients with cervical cancer. JAMA Netw Open. (2020) 3:e2011625. doi: 10.1001/jamanetworkopen.2020.11625
25. Li P, Feng B, Liu Y, Chen Y, Zhou H, Chen Y, et al. Deep learning nomogram for predicting lymph node metastasis using computed tomography image in cervical cancer. Acta Radiol. (2023) 64:360–9. doi: 10.1177/02841851211058934
26. Li X, Xu C, Yu Y, Guo Y, Sun H. Prediction of lymphovascular space invasion using a combination of tenascin-C, cox-2, and PET/CT radiomics in patients with early-stage cervical squamous cell carcinoma. BMC Cancer. (2021) 21:866. doi: 10.1186/s12885–021-08596–9
27. Zhang Z, Li X, Sun H. Development of machine learning models integrating PET/CT radiomic and immunohistochemical pathomic features for treatment strategy choice of cervical cancer with negative pelvic lymph node by mediating COX-2 expression. Front Physiol. (2022) 13:994304. doi: 10.3389/fphys.2022.994304
28. Lucia F, Bourbonne V, Pleyers C, Dupré PF, Miranda O, Visvikis D, et al. Multicentric development and evaluation of 18F-FDG PET/CT and MRI radiomics models to predict para-aortic lymph node involvement in locally advanced cervical cancer. Eur J Nucl Med Mol Imaging. (2023) 50:2514–28. doi: 10.1007/s00259-023-06180-w
29. Chen X, Liu W, Thai TC, Castellano T, Gunderson CC, Moore K, et al. Developing a new radiomics-based CT image marker to detect lymph node metastasis among cervical cancer patients. Comput Methods Programs Biomed. (2020) 197:105759. doi: 10.1016/j.cmpb.2020.105759
30. Jin X, Ai Y, Zhang J, Zhu H, Jin J, Teng Y, et al. Noninvasive prediction of lymph node status for patients with early-stage cervical cancer based on radiomics features from ultrasound images. Eur Radiol. (2020) 30:4117–24. doi: 10.1007/s00330–020-06692–1
31. Yi J, Lei X, Zhang L, Zheng Q, Jin J, Xie C, et al. The influence of different ultrasonic machines on radiomics models in prediction lymph node metastasis for patients with cervical cancer. Technol Cancer Res Treat. (2022) 21:15330338221118412. doi: 10.1177/15330338221118412
32. Song J, Hu Q, Ma Z, Zhao M, Chen T, Shi H. Feasibility of T2WI-MRI-based radiomics nomogram for predicting normal-sized pelvic lymph node metastasis in cervical cancer patients. Eur Radiol. (2021) 31:6938–48. doi: 10.1007/s00330-021-07735-x
33. Liu Y, Fan H, Dong D, Liu P, He B, Meng L, et al. Computed tomography-based radiomic model at node level for the prediction of normal-sized lymph node metastasis in cervical cancer. Transl Oncol. (2021) 14:101113. doi: 10.1016/j.tranon.2021.101113
34. Yang S, Zhang W, Liu C, Li C, Hua K. Predictive value and potential association of PET/CT radiomics on lymph node metastasis of cervical cancer. Ann Med Surg (Lond). (2023) 86:805–10. doi: 10.1097/MS9.0000000000001412
35. Yamada I, Oshima N, Miyasaka N, Wakana K, Wakabayashi A, Sakamoto J, et al. Texture analysis of apparent diffusion coefficient maps in cervical carcinoma: correlation with histopathologic findings and prognosis. Radiol Imaging Cancer. (2020) 2:e190085. doi: 10.1148/rycan.2020190085
36. Wu Q, Shi D, Dou S, Shi L, Liu M, Dong L, et al. Radiomics analysis of multiparametric MRI evaluates the pathological features of cervical squamous cell carcinoma. J Magn Reson Imaging. (2019) 49:1141–8. doi: 10.1002/jmri.26301
37. Ren J, Li Y, Yang JJ, Zhao J, Xiang Y, Xia C, et al. MRI-based radiomics analysis improves preoperative diagnostic performance for the depth of stromal invasion in patients with early stage cervical cancer. Insights Imaging. (2022) 13:17. doi: 10.1186/s13244–022-01156–0
38. Yan H, Huang G, Yang Z, Chen Y, Xiang Z. Machine learning-based multiparametric magnetic resonance imaging radiomics model for preoperative predicting the deep stromal invasion in patients with early cervical cancer. J Imaging Inform Med. (2024) 37:230–46. doi: 10.1007/s10278-023-00906-w
39. Xiao ML, Fu L, Wei Y, Liu AE, Cheng JJ, Ma FH, et al. Intratumoral and peritumoral MRI radiomics nomogram for predicting parametrial invasion in patients with early-stage cervical adenocarcinoma and adenosquamous carcinoma. Eur Radiol. (2024) 34:852–62. doi: 10.1007/s00330–023-10042–2
40. Du W, Wang Y, Li D, Xia X, Tan Q, Xiong X, et al. Preoperative prediction of lymphovascular space invasion in cervical cancer with radiomics -based nomogram. Front Oncol. (2021) 11:637794. doi: 10.3389/fonc.2021.637794
41. Li Z, Li H, Wang S, Dong D, Yin F, Chen A, et al. MR-based radiomics nomogram of cervical cancer in prediction of the lymph-vascular space invasion preoperatively. J Magn Reson Imaging. (2019) 49:1420–6. doi: 10.1002/jmri.26531
42. Huang G, Cui Y, Wang P, Ren J, Wang L, Ma Y, et al. Multi-parametric magnetic resonance imaging-based radiomics analysis of cervical cancer for preoperative prediction of lymphovascular space invasion. Front Oncol. (2022) 11:663370. doi: 10.3389/fonc.2021.663370
43. Xiao M, Li Y, Ma F, Zhang G, Qiang J. Multiparametric MRI radiomics nomogram for predicting lymph-vascular space invasion in early-stage cervical cancer. Br J Radiol. (2022) 95:20211076. doi: 10.1259/bjr.20211076
44. Wang S, Liu X, Wu Y, Jiang C, Luo Y, Tang X, et al. Habitat-based radiomics enhances the ability to predict lymphovascular space invasion in cervical cancer: a multi-center study. Front Oncol. (2023) 13:1252074. doi: 10.3389/fonc.2023.1252074
45. Cui L, Yu T, Kan Y, Dong Y, Luo Y, Jiang X. Multi-parametric MRI-based peritumoral radiomics on prediction of lymph-vascular space invasion in early-stage cervical cancer. Diagn Interv Radiol. (2022) 28:312–21. doi: 10.5152/dir.2022.20657
46. Wu Y, Wang S, Chen Y, Liao Y, Yin X, Li T, et al. A multicenter study on preoperative assessment of lymphovascular space invasion in early-stage cervical cancer based on multimodal MR radiomics. J Magn Reson Imaging. (2023) 58:1638–48. doi: 10.1002/jmri.28676
47. Hou L, Zhou W, Ren J, Du X, Xin L, Zhao X, et al. Radiomics analysis of multiparametric MRI for the preoperative prediction of lymph node metastasis in cervical cancer. Front Oncol. (2020) 10:1393. doi: 10.3389/fonc.2020.01393
48. Wu Q, Wang S, Chen X, Wang Y, Dong L, Liu Z, et al. Radiomics analysis of magnetic resonance imaging improves diagnostic performance of lymph node metastasis in patients with cervical cancer. Radiother Oncol. (2019) 138:141–8. doi: 10.1016/j.radonc.2019.04.035
49. Shi J, Dong Y, Jiang W, Qin F, Wang X, Cui L, et al. MRI-based peritumoral radiomics analysis for preoperative prediction of lymph node metastasis in early-stage cervical cancer: A multi-center study. Magn Reson Imaging. (2022) 88:1–8. doi: 10.1016/j.mri.2021.12.008
50. Yu YY, Zhang R, Dong RT, Hu QY, Yu T, Liu F, et al. Feasibility of an ADC-based radiomics model for predicting pelvic lymph node metastases in patients with stage IB-IIA cervical squamous cell carcinoma. Br J Radiol. (2019) 92:20180986. doi: 10.1259/bjr.20180986
51. Deng X, Liu M, Sun J, Li M, Liu D, Li L, et al. Feasibility of MRI-based radiomics features for predicting lymph node metastases and VEGF expression in cervical cancer. Eur J Radiol. (2021) 134:109429. doi: 10.1016/j.ejrad.2020.109429
52. Yan L, Yao H, Long R, Wu L, Xia H, Li J, et al. A preoperative radiomics model for the identification of lymph node metastasis in patients with early-stage cervical squamous cell carcinoma. Br J Radiol. (2020) 93:20200358. doi: 10.1259/bjr.20200358
53. Zhang Z, Wan X, Lei X, Wu Y, Zhang J, Ai Y, et al. Intra- and peri-tumoral MRI radiomics features for preoperative lymph node metastasis prediction in early-stage cervical cancer. Insights Imaging. (2023) 14:65. doi: 10.1186/s13244-023-01405-w
54. Wang T, Li YY, Ma NN, Wang PA, Zhang. A MRI radiomics-based model for prediction of pelvic lymph node metastasis in cervical cancer. World J Surg Oncol. (2024) 22:55. doi: 10.1186/s12957-024-03333-5. B.
55. Xia X, Li D, Du W, Wang Y, Nie S, Tan Q, et al. Radiomics based on nomogram predict pelvic lymphnode metastasis in early-stage cervical cancer. Diagnostics (Basel). (2022) 12:2446. doi: 10.3390/diagnostics12102446
56. Xiao ML, Wei Y, Zhang J, Jian JM, Song Y, Lin ZJ, et al. MRI texture analysis for preoperative prediction of lymph node metastasis in patients with nonsquamous cell cervical carcinoma. Acad Radiol. (2022) 29:1661–71. doi: 10.1016/j.acra.2022.01.005
57. Li L, Zhang J, Zhe X, Tang M, Zhang X, Lei X, et al. A meta-analysis of MRI-based radiomic features for predicting lymph node metastasis in patients with cervical cancer. Eur J Radiol. (2022) 151:110243. doi: 10.1016/j.ejrad.2022.110243
58. Ren J, Li Y, Liu XY, Zhao J, He YL, Jin ZY, et al. Diagnostic performance of ADC values and MRI-based radiomics analysis for detecting lymph node metastasis in patients with cervical cancer: A systematic review and meta-analysis. Eur J Radiol. (2022) 156:110504. doi: 10.1016/j.ejrad.2022.110504
59. He J, Liu Y, Li J, Liu S. Accuracy of radiomics in the diagnosis and preoperative high-risk assessment of endometrial cancer: a systematic review and meta-analysis. Front Oncol. (2024) 14:1334546. doi: 10.3389/fonc.2024.1334546
60. Yager J, Linn LS, Leake B, Gastaldo G, Palkowski C. Initial clinical judgments by internists, family physicians, and psychiatrists in response to patient vignettes: II. Ordering of laboratory tests, consultations, and treatments. Gen Hosp Psychiatry. (1986) 8:152–8. doi: 10.1016/0163–8343(86)90073–3
Keywords: cervical cancer, radiomics, deep stromal invasion, lymphatic vascular invasion, lymph node metastasis
Citation: Wu L, Li S, Li S, Lin Y and Wei D (2024) Preoperative magnetic resonance imaging-radiomics in cervical cancer: a systematic review and meta-analysis. Front. Oncol. 14:1416378. doi: 10.3389/fonc.2024.1416378
Received: 12 April 2024; Accepted: 27 May 2024;
Published: 04 July 2024.
Edited by:
Paolo Scollo, Kore University of Enna, ItalyReviewed by:
Salvatore Lavalle, San Raffaele Hospital (IRCCS), ItalyBasilio Pecorino, Kore University of Enna, Italy
Giuseppe Scibilia, gynecology and obstetrics department, Italy
Mattia Tarascio, Cannizzaro Hospital, Italy
Copyright © 2024 Wu, Li, Li, Lin and Wei. This is an open-access article distributed under the terms of the Creative Commons Attribution License (CC BY). The use, distribution or reproduction in other forums is permitted, provided the original author(s) and the copyright owner(s) are credited and that the original publication in this journal is cited, in accordance with accepted academic practice. No use, distribution or reproduction is permitted which does not comply with these terms.
*Correspondence: Dayou Wei, d2VpZGF5b3VtbUAxNjMuY29t