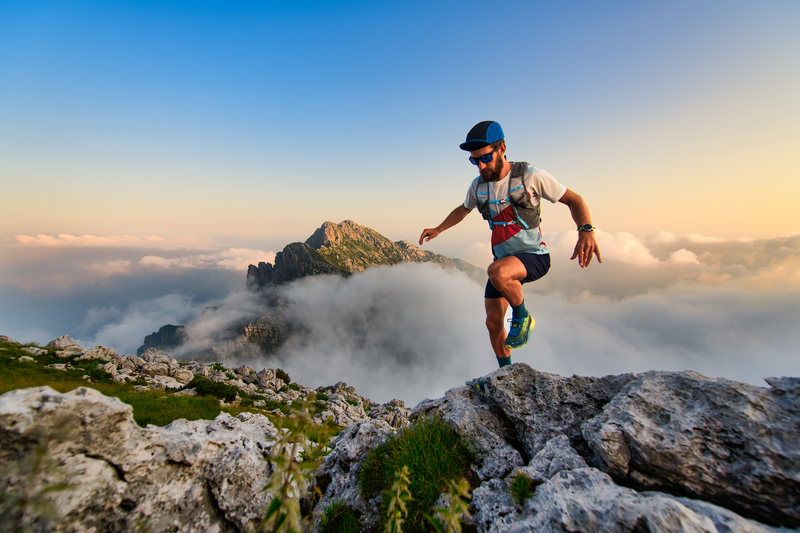
94% of researchers rate our articles as excellent or good
Learn more about the work of our research integrity team to safeguard the quality of each article we publish.
Find out more
REVIEW article
Front. Oncol. , 03 September 2024
Sec. Cancer Imaging and Image-directed Interventions
Volume 14 - 2024 | https://doi.org/10.3389/fonc.2024.1415859
Hepatocellular Carcinoma (HCC), the most common primary liver cancer, is a significant contributor to worldwide cancer-related deaths. Various medical imaging techniques, including computed tomography, magnetic resonance imaging, and ultrasound, play a crucial role in accurately evaluating HCC and formulating effective treatment plans. Artificial Intelligence (AI) technologies have demonstrated potential in supporting physicians by providing more accurate and consistent medical diagnoses. Recent advancements have led to the development of AI-based multi-modal prediction systems. These systems integrate medical imaging with other modalities, such as electronic health record reports and clinical parameters, to enhance the accuracy of predicting biological characteristics and prognosis, including those associated with HCC. These multi-modal prediction systems pave the way for predicting the response to transarterial chemoembolization and microvascular invasion treatments and can assist clinicians in identifying the optimal patients with HCC who could benefit from interventional therapy. This paper provides an overview of the latest AI-based medical imaging models developed for diagnosing and predicting HCC. It also explores the challenges and potential future directions related to the clinical application of AI techniques.
Hepatocellular Carcinoma (HCC), the most common primary liver malignancy, is linked to high mortality rates and stands as a leading cause of cancer-related deaths worldwide (1). Accurate diagnosis and staging of HCC are crucial for improving patient survival rates and treatment outcomes. However, early diagnosis of HCC presents a significant challenge, especially for individuals with chronic liver disease. A notable characteristic of liver cancer is its strong association with liver fibrosis, with over 80% of hepatocellular carcinomas (HCCs) developing in fibrotic or cirrhotic livers (2). This indicates that liver fibrosis plays a vital role in the liver’s premalignant environment.
Medical imaging techniques, including Computed Tomography (CT), Magnetic Resonance Imaging (MRI), and Ultrasound (US), play an essential role in the diagnosis and staging of HCC, supplementing clinical findings, biological markers, and blood tests. CT scans provide detailed cross-sectional images of the liver, aiding in the identification and characterization of tumors (3). MRI offers superior soft tissue contrast, making it invaluable for assessing the extent of liver cancer (4). US, a non-invasive and cost-effective imaging modality, can detect liver tumors by generating liver images using sound waves (5). However, each of these imaging methods has its limitations. For instance, CT scans expose patients to ionizing radiation, potentially heightening the risk of radiation-induced cancer. Moreover, CT scans can be expensive and less accessible in certain healthcare settings. While MRI can produce high-quality images, it can be time-consuming and may not be suitable for patients with claustrophobia or those with metal implants. US has limitations in image quality, particularly in patients with obesity or excessive intestinal gas. Recently, advanced MRI techniques, such as MR Elastography (MRE) and gadoxetic acid-enhanced MRI, have been introduced for liver imaging. These techniques provide high-resolution images without the harmful effects of radiation (6). MRE measures the stiffness of liver tissue, which can assist in differentiating between benign and malignant liver tumors. Gadoxetic acid-enhanced MRI offers dynamic imaging of the liver and can enhance the detection and characterization of HCC.
Diagnosing HCC poses significant challenges. These challenges arise from the prevalence of typical radiological features that are common to other liver tumors or benign conditions. Such similarities in imaging characteristics can lead to misdiagnosis or delayed diagnosis. As a result, patients with liver lesions exhibiting these typical features may require histological confirmation or rigorous monitoring to ensure accurate diagnosis and appropriate treatment.
In recent years, the potential of Artificial Intelligence (AI) techniques in diagnosing HCC has been the subject of extensive research. These techniques have been explored for various purposes such as detecting and evaluating HCC, facilitating treatment, and predicting treatment response (7–13). Numerous studies have investigated the use of AI models in conjunction with different modalities, including electronic health record (EHR) reports, clinical parameters, biological markers, and blood test results, for diagnosing liver cancer (14, 15). AI techniques have emerged as powerful tools capable of extracting valuable insights from voluminous EHRs and developing multimodal AI methods. These methods provide a more comprehensive and accurate depiction of the liver’s internal structure and function.
While many researchers have shown interest in exploring the potential of AI techniques in liver cancer research, there remains a gap in comprehensively evaluating the implementation of single-modal and multi-modal AI techniques for diagnosing HCC. This study aims to bridge this gap by providing a comprehensive review of the most recently developed AI-based techniques that utilize both single and multi-modal data for diagnosing HCC. AI-based techniques hold the potential to enhance early diagnosis, improve diagnostic accuracy, and improve treatment outcomes for patients with HCC. This pivotal area of research could lead to significant advancements in liver cancer diagnosis and prediction.
This research explores the application of AI methodologies in diagnosing and prognosticating primary liver cancer, specifically HCC. The objective is to encapsulate the latest and most relevant discoveries in this rapidly evolving field.
A thorough literature review was conducted using databases such as PubMed, Scopus, Semantic Scholar, IEEEXplore, and Web of Science, up until March 31, 2024. During this process, several key terms such as “artificial intelligence”, “deep learning”, “machine learning”, “liver cancer”, “hepatocellular carcinoma”, “multi-modal”, “medical imaging”, “US”, “CT”, and “MRI” were searched in the title and/or abstract or all field. References from relevant articles were examined to identify additional qualifying publications.
An expert review of the eligible literature was carried out, and the most informative and pertinent citations were chosen for inclusion. The studies selected were those that integrated AI techniques with medical imaging datasets, including US, CT, and MRI, in conjunction with Electronic Health Records (EHR) and clinical parameters. Studies that did not utilize medical imaging techniques or AI models specifically targeting primary liver cancer were excluded.
The search was confined to peer-reviewed articles, conference proceedings, dissertations, and book chapters published in English from January 2010 to March 2024. These publications were retrieved, screened, and reviewed by the authors. One researcher then undertook the data extraction, focusing on the methods and results of each study.
As depicted in Figure 1, our study selection process began with 1334 records. After removing 885 duplicates, we screened 450 records. The title and abstract screening led to the exclusion of 240 studies, leaving 210 for full-text review. Following a comprehensive evaluation, 177 articles (7, 16–186) were deemed suitable for this study. We categorized the modalities into four groups: US (n = 34), CT (n = 95), MRI (n = 34), and multi-modal (n=19). The characteristics of the included studies are detailed in Tables 1–10.
Table 8. Summary of studies evaluating AI-based multi-modal models for liver and liver tumors segmentation.
AI techniques, including Machine Learning (ML) and Deep Learning (DL), have been extensively investigated in application and interest within the field of liver cancer research (187–190). ML utilizes data to develop algorithms that can identify specific behavioral patterns and build predictive models. The objective of ML is to create a model that leverages statistical dependencies and correlations within a dataset, eliminating the need for explicit programming. This process is divided into two stages: training and validation. During the training stage, the model is exposed to a portion of the available data (training dataset). In the validation stage, the model’s performance is evaluated on a separate subset of the dataset (test dataset) to assess its ability to generalize its training performance to unseen data. Well-known ML algorithms, such as Support Vector Machines (SVM) and Artificial Neural Networks (ANNs), have been applied in HCC management (191, 192).
DL technology, a subset of ML, has shown remarkable efficacy in the analysis of liver images. This is largely due to its ability to process large volumes of data through multiple layers of artificial neurons. These neurons are engineered to emulate the intricate structure of the human brain and its biological neural networks. A unique characteristic of DL algorithms is that these layers of features are not manually constructed with human expertise. Rather, they are autonomously learned from data using a general-purpose learning procedure. This facilitates an end-to-end mapping from the input to the output, essentially converting the image into classification methods. In ML methods, success is contingent upon accurate segmentation and the selection of expert-designed features. DL approaches can surmount these limitations as they can identify the regions of the image most associated with the outcome through self-training. Moreover, they can discern the features of the region that informed the decision through multiple layers.
Convolutional Neural Networks (CNNs) are presently the most prevalent DL algorithms employed for the diagnosis and management of HCC (193–195). The uniqueness of CNNs compared to Fully Connected Networks lies in their ability to capture spatial hierarchies through convolutional and pooling layers, their parameter efficiency due to shared weights, and their effectiveness in processing structured data like images and videos. The fundamental principles of CNNs include local connections, shared weights, pooling, and the use of numerous layers. These components collectively enhance the accuracy and efficiency of the entire system. A standard CNN model is composed of an input layer, an output layer, and several hidden layers. These hidden layers encompass convolutional layers, pooling layers, and fully-connected layers. By repeatedly applying convolution and pooling, fully-connected layers are subsequently utilized for classification or predictions. There exists a variety of layer combinations, and numerous Deep Neural Network (DNN) architectures have been successfully implemented for HCC diagnosis and prediction. These include Fully Convolutional Networks (FCNs) (196), 3D U-Net (197), Recurrent Neural Networks (RNNs) (198), Graph Convolutional Networks (GCNs) (199, 200), Generative Adversarial Networks (GANs) (16, 201), AlexNet (202), and VGGNet-19 (203). These models are specifically engineered to eliminate fully connected layers and restore spatial dimensions, thereby augmenting DL capabilities even when there is a scarcity of labeled data. However, it is imperative to address domain adaptation and dataset bias to ensure the success of transfer learning (TL). This is because these factors can significantly influence the performance and generalizability of the models.
In contrast to CNNs, Fully Convolutional Networks (FCNs) are engineered to preserve spatial information, thereby enhancing their effectiveness for pixel-level predictions. This attribute renders FCNs particularly apt for liver tumor segmentation, as they employ convolutional layers in lieu of fully connected ones (196).
U-Net, conversely, utilizes an encoder-decoder model equipped with skip connections. This architecture enables it to amalgamate local and global context information, thereby augmenting object localization precision. Despite the limitations posed by scarce training data, 3D U-Net has exhibited remarkable results in the classification of liver lesions (197).
RNNs, encompassing Long Short-Term Memory (LSTM) and Gated Recurrence Unit (GRU), are specifically tailored to scrutinize sequential data by capturing temporal dependencies. These models have been successfully deployed for predicting HCC recurrence post liver transplantation (198). By addressing the vanishing gradients issue and capitalizing on temporal dependencies, they have substantially enhanced prediction accuracy.
Graph Convolutional Networks (GCNs) offer a variety of techniques for graph convolution, which are instrumental in clinically predicting Microvascular Invasion (MVI) in Hepatocellular Carcinoma (HCC) (199). These techniques include spectral-based and spatial-based GCN approaches, each carrying unique computational implications. DenseGCN, a contemporary architecture, has been introduced for the identification of liver cancer. It integrates advanced techniques such as similarity network fusion and denoising autoencoders, significantly boosting detection accuracy (200).
Generative Adversarial Networks (GANs) have demonstrated their value in generating synthetic images and augmenting data across a range of medical applications. In the realm of liver tumor detection, Tripartite GAN offers a cost-effective and non-invasive alternative by generating contrast-enhanced MRI images, eliminating the need for contrast agent injection (201). Another promising application is the Mask-Attention GAN, which generates realistic tumor images in CT scans for training and evaluation purposes (16).
Transfer Learning (TL) strategies have been employed in the field of medical imaging to mitigate overfitting issues arising from limited data. Within the TL framework, knowledge can be shared and transferred between different tasks. The workflow comprises two steps: pretraining on a large dataset and fine-tuning on the target dataset. Essentially, by fine-tuning the DL architecture, the knowledge gleaned from one dataset can be transferred to a dataset procured from another center.
US is recommended in clinical guidelines for the detection of HCC in patients with cirrhosis. However, its efficacy can be influenced by several factors, including operator experience, equipment quality, and patient morphology. Previous studies have indicated that the sensitivity of HCC detection using conventional US ranges from 59% to 78% (204). To enhance sensitivity and specificity, various US modalities have been explored. For instance, Contrast-Enhanced Ultrasound (CEUS) has been demonstrated to improve the sensitivity of HCC detection. These models serve as invaluable tools for predicting HCC recurrence, guiding treatment decisions, and improving patient outcomes. This study investigates the most recently developed AI-based approaches for evaluating detection, prognostication, treatment response, and survival in HCC. Table 1 provides a summary of the results from studies evaluating AI-based US approaches for HCC diagnosis.
This section outlines the recently developed AI-based US models for diagnosing HCC. These applications encompass diagnosing focal liver lesions (FLLs), distinguishing between benign and malignant liver lesions, differentiating HCC from focal nodular hyperplasia (FNH), cirrhotic parenchyma (PAR), and intrahepatic cholangiocarcinoma (ICC) (see Table 1). Among these studies, Bharti et al. (21) proposed a Support Vector Machine (SVM) model that integrates three classifiers using B-mode US data to assess and differentiate various stages of liver disease, achieving a classification accuracy of 96.6%.
In 2020, Brehar et al. (24) demonstrated that a CNN model, trained on two distinct US machine datasets (GE9 and GE7), surpassed conventional ML models (SVM, Random Forest (RF), Multi-Layer Perceptron, and AdaBoost) in differentiating between HCC and PAR. The proposed model achieved Area Under the Curve (AUC) values of 0.91 and 0.95 and accuracies of 84.84% and 91% in the GE9 and GE7 datasets, respectively. In 2023, Jeon et al. (35) proposed a CNN model using quantitative US data from 173 patients for diagnosing hepatic steatosis, achieving an AUC of 0.97, a sensitivity of 90%, and a specificity of 91%.
CEUS generally outperforms B-mode US in diagnosing FLLs and HCC, and AI has augmented its capabilities in identifying potential malignancies. Several research groups have studied the differentiation of benign and malignant FLLs (refer to Table 1). In 2020, Huang et al. (43) investigated the use of an SVM model for evaluating diagnostic accuracy when differentiating between atypical HCCs (aHCC) and FNH using CEUS data. The proposed SVM model achieved an AUC of 0.944, a sensitivity of 94.76%, and a specificity of 93.62%.
In 2021, Căleanu et al. (44) proposed a DL model to classify five types of FLLs using CEUS data, obtaining a general accuracy of 88%. Hu et al. (45) investigated a CNN model trained on four-phase CEUS video data from 363 patients. The proposed CNN model achieved an accuracy of 91% and an AUC of 0.934 on the testing dataset, slightly outperforming resident radiologists and matching experts.
In a study conducted by Virmani et al. (7), a Neural Network Ensemble (NNE) model was proposed to distinguish a normal liver from four distinct liver lesions, achieving an impressive accuracy of 95%. The diagnoses for the included liver lesions were confirmed through experienced radiologists, clinical follow-ups, and other associated findings.
In 2017, Hassan et al. (20) introduced an ANN model that achieved a classification accuracy of 97.2% for benign and malignant FLLs. In 2019, Schmauch et al. (22) developed a supervised DL model, specifically a CNN, utilizing a French radiology public challenge dataset for diagnosing FLLs. The model was capable of detecting FLLs and categorizing them as benign (such as cyst, FNH, and angioma) or malignant (like HCC, metastasis), achieving a mean AUC of 0.935 and 0.916 in the training dataset. Despite promising results, further validation is required due to the limited number of images used for training.
In 2020, Yang et al. (23) conducted a multicenter study to develop a Deep Convolutional Neural Network (DCNN) using an US database, along with background and clinical parameters (such as HBV, HCV, lesion margin, morphology) for characterizing FLLs. The model achieved an AUC of 0.924 for distinguishing benign from malignant lesions in the external validation dataset. The model demonstrated superior accuracy compared to clinical radiologists and CECT, albeit slightly lower than Contrast-Enhanced Magnetic Resonance Imaging (CE-MRI) (87.9%). This approach could potentially enhance radiologists’ performance and reduce the reliance on CECT/CEMR and biopsy.
In 2021, Mao et al. (25) developed various ML-based models for distinguishing primary liver cancer and secondary liver cancer by extracting radiomic features from US images. The Logistic Regression (LR) model outperformed other ML models in this study. Ren et al. (30) applied a Support Vector Machine (SVM) model in B-mode US for predicting the pathological grading of HCC, achieving an AUC of 0.874 in the test set. The same research group also developed another SVM model for differentiating HCC from Intrahepatic Cholangiocarcinoma (ICC), yielding good performances (31). In these studies, liver lesions were pathologically confirmed and used as the standard reference.
In 2017, Guo et al. (40) demonstrated that a multiple-kernel learning-based model could enhance the sensitivity, specificity, and overall accuracy of CEUS for detecting HCC. Later, Ta et al. (41) proposed an ANN model using CEUS data for differentiating benign liver lesions from malignant ones. The model showed promising results, classifying liver lesions as benign or malignant with accuracy comparable to expert radiologists and superior to physicians. Huang et al. (43) constructed an SVM model for differentiating atypical HCC (aHCC) and FNH using CEUS data, achieving an average accuracy of 94.4% compared to pathology reports and clinical follow-up.
In 2021, Wang et al. (46) proposed an SVM model using CEUS data, which could discriminate HCC pathological grading with an AUC of 0.72. More recently, Zhou et al. (48) investigated CNN-Long Short-Term Memory (LSTM), 3D CNN, and ML-TIC models for classifying benign and malignant liver lesions using CEUS data from 440 patients, achieving AUC values of 0.91, 0.88, and 0.78, respectively.
Surgery, Transcatheter Arterial Chemoembolization (TACE), and Microwave Ablation are widely recognized as treatment methods for liver cancer. Each method requires meticulous candidate evaluation to ensure optimal therapeutic effectiveness (38–40). Wu et al. (203) employed ResNet18 in B-mode US to predict HCC recurrence after Microwave Ablation. The model achieved C-index values of 0.695, 0.715, 0.721, and 0.721 for early relapse, late relapse, and relapse-free survival in HCC patients, respectively.
Liu et al. (42) developed two DL-based models using CEUS data to predict the two-year progression-free survival of HCC patients undergoing either Radiofrequency Ablation or Surgical Resection. The models achieved C-index values of 0.726 and 0.741 for Radiofrequency Ablation and Surgical Resection, respectively. When the Surgical model was applied to predict outcomes for patients initially treated with Ablation, it suggested that approximately 17.3% of Ablation patients could potentially experience a longer two-year progression-free survival if they underwent Surgery. Conversely, the Ablation predictive model indicated that 27.3% of Surgical patients might achieve a longer two-year progression-free survival if they had received Ablation treatment. These CEUS-based models provide accurate survival assessments for HCC patients and facilitate optimal treatment selection. Furthermore, the same research group employed a DL model to quantitatively analyze CEUS videos (43). They developed three models to predict personalized responses of HCC patients after their first TACE session. The CEUS-based model outperformed the other two ML models, achieving a higher AUC value (0.93 vs 0.80 vs 0.81).
In another study, Ma et al. (44) applied a Radiomics model in dynamic CEUS to predict early and late recurrence in patients with an HCC lesion less than 5cm in diameter after Thermal Ablation. The prediction model yielded an AUC of 0.84 for early recurrence and a C-index of 0.77 for late recurrence in the test group. The proposed model, which combines CEUS, US Radiomics, and clinical factors, performed well in predicting early HCC recurrence after Ablation and could stratify the high risk of late recurrence.
Lastly, Liu et al. (16) introduced DL models in CEUS to predict the two-year progression-free survival rate of HCC patients, demonstrating exceptional accuracy in guiding treatment decisions. Other researchers have incorporated additional pattern recognition classifiers into DCNN algorithms using CEUS to improve the diagnosis of FLLs. However, previous studies only involved small sample sizes, thus standardized imaging data or external validations are required to validate the model’s generalizability across populations.
Numerous research groups have explored the application of AI in liver cancer research, specifically leveraging CT scan technology. This section delves into AI-based CT methodologies for diagnosing and predicting HCC. Tables 2 and 3 encapsulate selected studies, which can be categorized into three distinct groups: segmentation of liver and liver tumors, characterization of FLLs, and evaluation of prognostication, treatment response, and survival in HCC patients.
The segmentation of liver and liver tumors plays a crucial role in assessing tumor burden, detecting early recurrence, extracting image features, and formulating treatment plans. The manual segmentation of liver and liver lesions is a significant challenge and is time-consuming due to the extensive range of radiographic features in HCC. AI-driven CT models have emerged as powerful tools for the automatic segmentation of liver and liver tumors. Table 2 provides a summary of recently developed AI-driven CT models for segmentation of liver and liver tumors.
In 2015, Li et al. (49) introduced a DCNN for the segmentation of liver tumors in CT scans, achieving a precision rate of 82.67%. In 2017, Vivanti et al. (50) examined a CNN-based segmentation model for the automatic detection of recurrence during follow-up, achieving a true positive rate of 86% for lesions larger than 5 mm (28). Subsequently, Sun et al. (51) and Das et al. (52) conducted comprehensive studies on the automatic segmentation of tumors in the liver using CNN-based architectures such as Fully Convolutional Networks (FCNs) and U-Net. In 2017, Sun et al. (51) proposed an FCNs model for the segmentation of liver tumors, achieving high accuracy.
Since 2017, the Liver Tumor Segmentation Challenge (LiTS) has been encouraging researchers to create AI models for the automatic segmentation of liver tumors. This challenge utilizes a multinational dataset of CT images, known as LiTS17, which includes 130 CT images for training and 70 CT images for testing. Over the past few years, this challenge has seen participation from more than 280 research teams worldwide, with models based on Fully Convolutional Networks (FCN) or U-Net achieving top scores for the segmentation of liver and liver tumors.
At present, the highest-scoring model, MAD-UNet (83), has achieved Dice score of 0.9727 for the segmentation of liver using the LiTS17 dataset. While these results are promising, there is a notable variability in both the imaging characteristics of liver tumors and their delineation. This highlights the need for universal and standardized methods for liver tumor segmentation.
Table 3 summarizes the results of studies that have evaluated AI-based CT models for diagnosing HCC. Mokrane et al. (106) developed a ML model using 13,920 CT images from 189 patients. This model was able to distinguish HCC from non-HCC lesions in cirrhotic patients, achieving AUC values of 0.81 and 0.66 in the training and external validation datasets, respectively.
In 2019, Khan et al. (107) developed a SVM model that classified FLLs as benign or malignant, achieving an accuracy of 98.3%. Das et al. (52) proposed a CAD system based on a watershed transform and Gaussian Mixture Model (GMM) for accurate and automated liver lesion detection using CT scan data. The liver was first separated using the watershed transform method, and the liver lesion was segmented using the GMM algorithm. Texture features were extracted and fed into a DNN model to automatically classify three types of liver tumors, including hemangioma, HCC, and metastatic carcinoma. The proposed model achieved a classification accuracy of 99.38% and a Jaccard index of 98.18%.
In 2020, Li et al. (108) developed a CAD system using ANN, SVM, and CNN models for diagnosing three types of HCC lesions, including nodular, diffuse, and massive. The experimental results demonstrated that the CNN model outperformed both the ANN and SVM models in classifying nodular and massive lesions, but not diffuse lesions.
In 2021, Mao et al. (25) developed a gradient boosting-based model using clinical parameters and CECT data for pathological grading of HCC. The combined model exhibited the best performance with an AUC of 0.8014 in the test set. Shi et al. (109) compared the performance of a DL-based three-phase CECT model with a four-phase CT protocol for distinguishing HCC from other FLLs. The DL-based three-phase CECT protocol without pre-contrast achieved a similar diagnostic accuracy (85.6%) to the four-phase CT protocol (83.3%). These findings suggest that omitting the pre-contrast phase might not compromise accuracy while reducing a patient’s radiation dose.
Several CNN-based models have been developed using CT data for diagnosing HCC. In 2018, Yasaka et al. (110) proposed a CNN model using three-phase CT for distinguishing malignant liver lesions from indeterminate and benign liver lesions. The proposed model achieved a median AUC of 0.92 in the test set. In 2019, Todoroki et al. (111) developed a CNN-based model using multiphasic CT images for detecting and classifying five types of FLLs. Ben-Cohen et al. (91) introduced a FCN architecture with sparsity-based false positive reduction for liver tumor detection, outperforming traditional models. By employing the FCN-4s model and sparsity-based fine-tuning, they successfully detected 94.7% of small lesions, surpassing the performance of the U-Net model.
In 2021, Zhou et al. (112) proposed a multi-modality and multi-scale CNN model for automatically detecting and classifying FLLs in multi-phasic CT. The model obtained an average test precision of 82.8%, recall of 93.4%, and F1-score of 87.8%. The model achieved average accuracies of 82.5% and 73.4% for the binary and six-class classification, respectively. In this study, the classification performance of the model was placed between a junior and senior physician’s evaluation. This preliminary study showed that this CNN-based model can accurately locate and classify FLLs, and could assist inexperienced physicians in reaching a diagnosis in clinical practice. Similarly, Ponnoprat et al. (113) constructed a two-step model based on CNN and SVM for distinguishing HCC and intrahepatic cholangiocarcinoma (ICC), and the model achieved a classification accuracy of 88%.
In 2021, Krishnan et al. (114) introduced a novel multi-level ensemble architecture for detecting and classifying HCC from other FLLs. This innovative approach highlights the potential of ensemble techniques in improving the specificity and sensitivity of liver cancer diagnosis using CT imaging.
In 2023, Manjunath et al. (115) developed a novel DL model using CT data to detect and classify liver tumors. The experimental results demonstrated that the proposed model improved accuracy, Dice similarity coefficient, and specificity compared to existing algorithms, emphasizing the continuous evolution of DL models for precise liver cancer diagnosis.
Numerous research groups have focused their efforts on the applications of AI models using CT and CECT images for the prognostication of HCC. Table 4 provides a summary of the results from studies that evaluated AI-based CT models for HCC prognostication. Among these studies, Peng et al. (131) proposed a novel AI model based on conventional Machine Learning (cML) and DL methods. This model utilized CT data from 310 patients to predict TACE in patients with HCC. The experimental results demonstrated that the proposed model achieved AUC values of 0.995 and 0.994 in the training and testing datasets, respectively.
In 2021, Jiang et al. (81) developed a 3D CNN using CT data from 405 patients. This model was designed to predict Microvascular Invasion (MVI) in patients with HCC and obtained commendable AUC values of 0.98 and 0.906 in the training and testing datasets, respectively.
In 2022, Yang et al. (132) conducted an investigation of various AI models using CECT data from 283 patients. The aim was to predict MVI in patients with HCC. The experimental results revealed that the DL-based clinical-radiological model achieved the best performance with an accuracy of 96.47%, a sensitivity of 90.91%, a specificity of 97.30%, a precision of 89.4%, an F1 score of 87%, and an AUC of 0.909.
To date, the application of AI models in MRI for diagnosing HCC has not been extensively adopted. The development of MRI features poses technical challenges and incurs substantial costs, resulting in a scarcity of published studies with relatively small sample sizes. This section explores the progression of AI-based MRI models for the diagnosis of HCC.
In recent years, a multitude of research groups have focused on the applications of AI models utilizing MRI data for the automated segmentation of the liver and liver tumors. Table 5 encapsulates the AI-based MRI models recently developed for the segmentation of liver and liver tumors. Among the various studies, the most remarkable performance was delivered by Hossain et al. (139), who pioneered a cascaded network to address anatomical ambiguity. This model, which employs T1-weighted MRI data for liver segmentation, exhibited an impressive performance with a Dice coefficient of 0.9515, Intersection over Union (IoU) of 0.921, and an accuracy of 99.7%.
More recently, Gross et al. (140) developed a DCNN model using T1-weighted MRI data from 470 patients for liver segmentation. The results suggested that the proposed DCNN model achieved mean Dice values of 0.968, 0.966, and 0.928 in the training, validation, and public testing datasets, respectively.
Table 6 encapsulates the advancements in AI-based MRI models for diagnosing HCC. These models have shown promise in improving the detection and classification of FLLs, including HCC. In 2019, Hamm et al. (158) proposed a CNN model capable of classifying six types of FLLs, namely adenoma, cyst, Focal Nodular Hyperplasia (FNH), HCC, Intrahepatic Cholangiocarcinoma (ICC), and metastases. The model demonstrated an impressive overall accuracy of 92%, with sensitivity values spanning from 60% to 100%, and specificity values between 89% and 99%. This study highlighted the potential of DL in accurately identifying various types of FLLs.
Wang et al. (159) developed an interpretable DL model using MRI images. The model achieved a positive predictive rate of 76.5% and a sensitivity of 82.9% for classifying FLLs. The interpretability of this model enhances its clinical utility by offering insights into the decision-making process.
Trivizakis et al. (160) employed a 3D CNN model with Diffusion-Weighted Magnetic Resonance (DW-MR) data to classify primary and metastatic liver tumors. The model achieved an accuracy of 83%, underscoring the potential of DL in enhancing liver tumor recognition, particularly in datasets with limited size and disease specificity.
In 2020, Zhen et al. (161) pioneered several CNN models, including a distinctive model that utilizes unenhanced MR images for liver tumor diagnosis, thereby eliminating the need for contrast agent injection. This innovative approach demonstrated a performance on par with experienced radiologists, suggesting a potential reduction in patient discomfort and risks associated with contrast agents.
Kim et al. (162) introduced a CNN model that achieved an impressive AUC of 0.97, a sensitivity of 94%, and a specificity of 99% for HCC detection using a training dataset of 455 patients. In a validation dataset of 45 patients, the model maintained an AUC of 0.90, sensitivity of 87%, and specificity of 93% for HCC detection. This study underscored the capability of deep learning models in accurately identifying HCC, a critical step in early diagnosis and treatment planning.
Wu et al. (16) developed a DL model based on multiphase, contrast-enhanced MRI to differentiate between different grades of liver tumors for HCC diagnosis. The model utilized a CNN to classify the Liver Imaging Reporting and Data System tumor grades of liver lesions based on MRI data acquired at three-time points. The DL CNN model achieved high accuracy, sensitivity, precision, and AUC, providing valuable clinical guidance for differentiating between intermediate LR-3 liver lesions and more likely malignant LR-4/LR-5 lesions in HCC diagnosis.
In 2021, Wan et al. (163) proposed a CNN architecture based on multi-scale and multi-level fusion (MMF-CNN) for detecting liver lesions in MRI images. The model’s effectiveness was confirmed through comparative analysis with other DL models, emphasizing its potential to improve diagnostic accuracy and efficiency. The proposed MMF-CNN architecture is a promising approach to accurately and efficiently detect liver lesions in MRI images, which can significantly improve patient outcomes.
Oestmann et al. (164) presented a CNN model that employs multiphasic MR images to differentiate between HCC and non-HCC lesions. The model demonstrated high sensitivities and specificities for both lesion types, achieving 92.7% and 82.0% sensitivities for HCC and non-HCC lesions, respectively, and specificities of 82.0% for both HCC and non-HCC lesions. The research underscored the importance of accurately distinguishing between HCC and non-HCC lesions to guide appropriate treatment strategies for liver cancer patients.
Bousabarah et al. (148) proposed a CNN for detecting and segmenting HCC using multiphase contrast-enhanced MRI data. The model exhibited a promising performance with 73% and 75% sensitivities for validation and testing datasets, respectively. The performance evaluation compared the automatically detected lesions with manual segmentation. The mean Dice score values between the identified lesions using the CNN model and manual segmentations were 0.64 and 0.68 for the validation and testing datasets, respectively.
The advancements in CNN-based MRI models for diagnosing HCC have significantly enhanced the accuracy, efficiency, and precision of lesion classification and detection. From distinguishing different types of FLLs to detecting targeted HCC, these CNN-based models have showcased remarkable performance metrics and potential clinical utility. Further research and validation studies are essential to fully assess the capabilities of these models in clinical settings, paving the way for personalized and effective treatment strategies in liver cancer management.
A select number of research groups have ventured into the application of AI models and MRI-based data for HCC prognostication. Table 7 encapsulates a summary of studies evaluating AI-based MRI models for this purpose.
In 2021, Gao et al. (168) scrutinized various AI models using T2-weighted MRI data from 225 patients to predict Microvascular Invasion (MVI) in patients with HCC. The H-DARnet model outshone others, achieving an accuracy of 82.6%, a sensitivity of 79.5%, a specificity of 73.8%, and an AUC of 0.775.
Wei et al. (187) investigated the fusion DL model and the Contrast-Dependent Learning Model (CDLM) using gadoxetic acid-enhanced MRI (EOB-MRI) data from 225 patients for predicting MVI in patients with HCC. Both models exhibited robust performance, with the Fusion DL model achieving an accuracy of 89.4%, a sensitivity of 78.1%, a specificity of 95.3%, and an AUC of 0.93. The CDLM model achieved an accuracy of 92.4%, a sensitivity of 93.9%, a specificity of 91.6%, and an AUC of 0.962 in the training dataset.
In 2023, Chen et al. (169) explored four models (KNN, SVM, Lasso, and DNN) using T2-weighted MRI data from 144 patients for predicting Transarterial Chemoembolization (TACE) outcomes in patients with HCC. Among these, the Lasso model achieved the best performance.
These studies underscore the potential of AI models in conjunction with MRI data for predicting HCC, demonstrating promising results in terms of accuracy, sensitivity, specificity, and AUC. Further research in this area could catalyze significant advancements in the early detection and treatment of HCC.
AI-based multi-modal techniques are swiftly ascending to prominence in the realm of medical imaging, attributed to its extraordinary ability to amplify diagnostic accuracy and forecast outcomes. AI-based multi-modal model integrates multiple modalities, such as medical imaging data, Electronic Health Records (EHR) and clinical parameters, thereby substantially enhancing the efficacy of AI algorithms. AI-based multi-modal models have proven successful in predicting treatment responses, evaluating survival rates, and staging a multitude of diseases. Such techniques have been deployed in a plethora of studies pertaining to liver imaging applications, yielding encouraging results. The continued exploration and refinement of these techniques hold great promise for the future of medical imaging and patient care.
Table 8 encapsulates a summary of studies that evaluate AI-based multi-modal models for the segmentation of liver and liver tumors. Among the various studies, the most remarkable performance was demonstrated by Hille et al. (173). They explored the SWTR-Unet model using a combination of 61,440 MRI images and 189,600 CT images for the segmentation of both the liver and hepatic lesions. The proposed multi-modal model achieved Dice coefficients of 0.98 and 0.81 for the segmentation of the liver and hepatic lesions, respectively.
AI-based multi-modal models offer a comprehensive and robust approach to HCC diagnosis, enabling disease prediction, classification, treatment response prediction, survival rate determination, and disease staging. The outcomes of studies evaluating AI-based multi-modal models for HCC diagnosis are summarized in Table 9.
In 2020, Menegotto et al. (176) utilized a DCNN for HCC diagnosis, incorporating CT data and various EHR parameters. These parameters encompassed demographic factors, clinical history, laboratory test results, and other pertinent medical information. The model achieved accurate HCC diagnosis by considering 20 unique EHR parameters, highlighting the potential of integrating diverse clinical data for enhanced disease identification. Subsequently, they (177) developed an Xception CNN model using CT data and EHR parameters for HCC diagnosis. This method accurately detected HCC, demonstrating the potential of combining various modalities for improved HCC identification.
Zhen et al. (161) developed a multi-modal model that combines Google’s Inception-ResNetV2 CNN with an autoencoder neural network. This model was used to diagnose HCC using MRI data and clinical parameters, including age, gender, tumor markers, liver function, and other relevant factors. The study confirmed the potential of combining medical imaging and clinical data to improve HCC diagnosis, emphasizing the importance of such techniques in enhancing healthcare outcomes.
In 2021, Gao et al. (118) employed a multi-modal model based on the VGG16 architecture to detect HCC in CT images. The study aimed to determine the model’s accuracy in detecting HCC by incorporating eight EHR parameters, including age, gender, platelet count, bilirubin levels, tumor markers, and hepatitis B virus status. The research findings demonstrated the capacity of multi-modal DL to accurately identify HCC. This study underscores the potential of ML algorithms in assisting the early detection and diagnosis of HCC, which may lead to improved patient outcomes. Li et al. (179) investigated a ML-based multi-modal model using three-phase CEUS data from 266 patients and a radiologist’s score for evaluating the diagnostic accuracy when differentiating between atypical Hepatocellular Carcinoma (aHCC) and Focal Nodular Hyperplasia (FNH). The proposed model achieved the highest AUC of 0.93 in aHCC and FNH differentiation.
In 2022, Liu et al. (180) proposed a DL model to detect malignancy by combining clinical parameters and CEUS data from 303 patients. The model achieved the best performance with AUC values of 0.969 and 0.957 and accuracies of 96% and 94% in the IntraVenous (IV) and ExtraVenous (EV) groups, respectively. Further research is necessary to identify the optimal combination of modalities and variables for specific medical tasks. The development of standardized protocols and datasets is critical to facilitate the comparison and reproducibility of multi-modal AI models in medical image analysis.
A multitude of studies have explored the use of AI-based multi-modal models for prognostication of HCC. The insights from these studies are compiled in Table 10. Among these, a significant contribution was made by Sun et al. (183), who implemented a hybrid model combining GhostNet and CNN models. This integrated model leveraged CT data and clinical parameters to predict the response of TACE treatment in HCC patients. The proposed method exhibited remarkable performance, achieving an accuracy of 98% and an AUC of 0.98. This model demonstrated its potential in predicting TACE treatment responses, thereby assisting healthcare providers in devising personalized treatment plans and making informed decisions. This approach shows promise in improving patient outcomes and raising the bar in clinical practice.
In the past decade, AI models’ application in medical imaging for HCC diagnosis and prediction has emerged as a significant research area. While individual medical imaging methods such as US, CT, and MRI have been explored (205–208), there is a lack of comprehensive reviews focusing on AI-based models using both single and multi-modal modalities. This study aims to fill that gap, reviewing AI models developed for HCC diagnosis and prediction using both single and multi-modal methods from January 2010 to March 2024.
Despite AI-based diagnostic models not significantly improving overall diagnostic accuracy for pathologists, they have shown increased precision within specific subgroups. However, several challenges must be addressed before integrating these models into clinical workflows. The efficacy of AI models depends on both the models’ accuracy and the quality of the datasets used. Factors such as biases, mislabeling, lack of standardization, and missing data can undermine these datasets. Overfitting and spectrum biases are prevalent issues in AI-based medical imaging models. Therefore, the need for standardized methods for AI-based data analysis and comprehensive strategies to tackle missing data is evident.
AI tools intended for medical applications could be categorized as medical devices and must adhere to pertinent regulations. Both the FDA and the European Commission have initiated plans to tackle this issue. Intellectual property concerns, particularly those associated with post-marketing modifications, could pose safety risks. The performance of AI models is intimately linked to the training dataset. The importance of large datasets is paramount, and the promotion of data sharing is necessary, which brings forth ethical and privacy considerations. The clinical performance of AI and the requirement for post-approval validation are significant issues. The development of explainable AI models is vital for securing clinicians’ trust and reliance on AI-based CAD systems. Customized prospective clinical trials are indispensable to fully comprehend the role of AI in HCC management.
Looking ahead, the integration of AI in HCC management presents an exciting frontier in medical science. As we continue to refine AI models and address the challenges, we move closer to a future where AI plays a pivotal role in personalized patient care. The potential of AI to analyze vast amounts of data and make precise predictions can lead to early detection and more effective treatment strategies for HCC. This not only improves patient outcomes but also paves the way for a new era in healthcare, where technology and human expertise work hand in hand for the betterment of patient care.
Several strategies are essential for the future of AI in HCC diagnosis and prediction. First, the development of standardized methods for AI-based data analysis and comprehensive strategies to handle missing data are crucial. Second, universal approaches to handle missing data and improve data quality are vital for enhancing the robustness and reliability of DL-based diagnostic tools. Promoting data sharing initiatives can facilitate the availability of large, diverse datasets necessary for training and validating DL models.
In addition to the aforementioned strategies, the exploration of advanced technologies such as transfer learning can further enhance the role of AI in HCC diagnosis and prediction. This technology can adapt pre-trained DL models to new tasks with limited labeled data. This addresses the challenge of acquiring extensive datasets in medical imaging, a common hurdle in the healthcare sector. Federated Learning (FL) is emerging as a transformative trend in healthcare. It enables a collaborative approach to ML development across multiple institutions, eliminating the need for direct data sharing. This innovative method involves the exchange of model parameters only, thereby ensuring the privacy of individual datasets. In the context of liver cancer, where patient data is both sensitive and heavily regulated, FL offers a unique advantage. It allows for the integration of fragmented healthcare data sources while preserving privacy. This enhances the scope and accuracy of ML models, making them more effective and reliable. As such, FL is poised to become an invaluable tool for future research and clinical implementation in liver cancer treatment. It offers the potential to significantly advance patient care, marking a new era in the field of liver cancer treatment.
The development of explainable AI models is another critical step towards earning the trust and reliance of clinicians on AI-based CAD systems. The synergy of researchers, clinicians, and policymakers is a cornerstone in propelling innovation and setting the gold standard for the application of AI techniques in liver cancer care. A comprehensive approach is required to augment AI techniques for HCC diagnosis and management. This involves addressing key aspects such as interpretability, accuracy, data integration, ethical considerations, and validation processes. By tackling these areas, we can tap into the full potential of AI technology, leading to a revolution in HCC diagnosis and prediction. Customized prospective clinical trials are paramount to gain a complete understanding of the role of AI in HCC management. Regulatory bodies like the FDA and the European Commission have kick-started plans to address the regulatory compliance of AI-based diagnostic tools. These plans demand further development and implementation. The challenges and future directions underscore the intricacy of incorporating AI in HCC diagnosis and prediction. However, with persistent research and development, AI holds the promise to bring about a paradigm shift in this field.
This paper offers an exhaustive exploration of AI-driven models for the diagnosis and prediction of HCC, leveraging both medical imaging data and additional clinical information. The potential of AI-based methodologies in diagnosing HCC is vast, yet several hurdles need to be overcome before they can be seamlessly incorporated into clinical workflows to enhance patient diagnosis and treatment outcomes. Despite the presence of challenges such as data quality, model overfitting, regulatory compliance, and the necessity for explainable AI models, the potential advantages are considerable. AI models have the capacity to augment precision within specific patient subgroups. Furthermore, the development of standardized methods for data analysis can significantly bolster the robustness and reliability of these tools. Navigating these intricacies, it becomes evident that a multi-pronged strategy is essential to fully harness the transformative power of AI technology in revolutionizing HCC diagnosis and treatment. With ongoing research and development, AI stands poised to usher in a paradigm shift in the field of HCC diagnosis and prediction, ultimately leading to enhanced patient outcomes and heralding a new epoch in healthcare.
LW: Conceptualization, Data curation, Formal analysis, Investigation, Methodology, Resources, Validation, Writing – original draft, Writing – review & editing. MF: Investigation, Writing – review & editing. AA: Investigation, Writing – review & editing.
The author(s) declare that no financial support was received for the research, authorship, and/or publication of this article.
The authors would like to acknowledge Copilot for reference formatting and proof reading of this work.
The authors declare that the research was conducted in the absence of any commercial or financial relationships that could be construed as a potential conflict of interest.
All claims expressed in this article are solely those of the authors and do not necessarily represent those of their affiliated organizations, or those of the publisher, the editors and the reviewers. Any product that may be evaluated in this article, or claim that may be made by its manufacturer, is not guaranteed or endorsed by the publisher.
1. Shirono T, Niizeki T, Iwamoto H, Shimose S, Suzuki H, Kawaguchi T, et al. Therapeutic outcomes and prognostic factors of unresectable intrahepatic cholangiocarcinoma: A data mining analysis. J Clin Med. (2021) 10:987. doi: 10.3390/jcm10050987
2. Affo S, Yu LX, Schwabe RF. The role of cancer-associated fibroblasts and fibrosis in liver cancer. Annu Rev Pathol. (2017) 12:153–86. doi: 10.1146/annurev-pathol-052016-100322
3. Rehani MM, Szczykutowicz TP, Zaidi H. CT is still not a low-dose imaging modality. Med Phys. (2020) 47:293–6. doi: 10.1002/mp.14000
4. Feng Z, Zhao H, Guan S, Wang W, Rong P. Diagnostic performance of MRI using extracellular contrast agents versus gadoxetic acid for hepatocellular carcinoma: a systematic review and meta-analysis. Liver International: Off J Int Assoc Study Liver. (2021) 41:1117–28. doi: 10.1111/liv.14850
5. Tharwat M. Ultrasonography of the liver in healthy and diseased camels (camelus dromedaries). J Vet Med Sci. (2020) 82:1–9. doi: 10.1292/jvms.19-0690
6. Cho HJ, Kim B, Kim HJ, Huh J, Cheong JY. Liver stiffness measured by MR elastography is a predictor of early HCC recurrence after treatment. Eur Radiol. (2020) 30:4182–92. doi: 10.1007/s00330-020-06792-y
7. Virmani J, Kumar V, Kalra N, Khandelwal N. Neural network ensemble based CAD system for focal liver lesions from B-mode ultrasound. J Digit Imaging. (2014) 27:520–37. doi: 10.1007/s10278-014-9685-0
8. Xu Y, Cai M, Lin L, Zhang Y, Tong R. PA-ResSeg: a phase attention residual network for liver tumor segmentation from multi-phase CT images. Med Phys. (2021) 48:3752–66. doi: 10.1002/mp.14922
9. Mehltretter J, Fratila R, Benrimoh D, Kapelner A, Turecki G. Differential treatment benefit prediction for treatment selection in depression: a deep learning analysis of stard and comed data. Comput Psychiatry. (2020) 4:1–15. doi: 10.1162/cpsy_a_00029
10. Lanhong Y, Zheyuan Z, Elif K, Cemal Y, Temel T, Ulas B. A review of deep learning and radiomics approaches for pancreatic cancer diagnosis from medical imaging. Curr Opin Gastroenterol. (2023) 39:436–337. doi: 10.1097/MOG.0000000000000966
11. Ye Y, Zhang N, Wu D, Huang B, Cai X, Ruan X, et al. Deep learning combined with radiologist’s intervention achieves accurate segmentation of hepatocellular carcinoma in dual-phase magnetic resonance images. BioMed Res Int. (2024) 2024:9267554. doi: 10.1155/2024/9267554
12. Xin H, Zhang Y, Lai Q, Liao N, Zhang J, Liu Y, et al. Automatic origin prediction of liver metastases via hierarchical artificial-intelligence system trained on multiphasic CT data: a retrospective, multicentre study. EClinicalMedicine. (2024) 69:102464. doi: 10.1016/j.eclinm.2024.102464
13. Urhuţ MC, Săndulescu LD, Streba CT, Mămuleanu M, Ciocâlteu A, Cazacu SM, et al. Diagnostic performance of an artificial intelligence model based on contrast-enhanced ultrasound in patients with liver lesions: A comparative study with clinicians. Diagnost (Basel). (2023) 13:3387. doi: 10.3390/diagnostics13213387
14. Hu X, Li X, Zhao W, Cai J, Wang P. Multimodal imaging findings of primary liver clear cell carcinoma: a case presentation. Front Med (Lausanne). (2024) 11:1408967. doi: 10.3389/fmed.2024.1408967
15. Wang Q, Zhou Y, Yang H, Zhang J, Zeng X, Tan Y. MRI-based clinical-radiomics nomogram model for predicting microvascular invasion in hepatocellular carcinoma. Med Phys. (2024) 51:4673–86. doi: 10.1002/mp.17087
16. Wu Y, White GM, Cornelius T, Gowdar I, Ansari MH, Supanich MP, et al. Deep learning LI-RADS grading system based on contrast enhanced multiphase MRI for differentiation between LR-3 and LR-4/LR-5 liver tumors. Ann Transl Med. (2020) 8:701. doi: 10.21037/atm.2019.12.151
17. Xian GM. An identification method of Malignant and benign liver tumors from ultrasonography based on GLCM texture features and fuzzy SVM. Expert System App. (2010) 37:6737–41. doi: 10.1016/j.eswa.2010.02.067
18. Mittal D, Kumar V, Saxena SC, Khandelwal N, Kalra N. Neural network based focal liver lesion diagnosis using ultrasound images. Comput Med Imaging Graph. (2011) 35:315–23. doi: 10.1016/j.compmedimag.2011.01.007
19. Hwang YN, Lee JH, Kim GY, Jiang YY, Kim SM. Classification of focal liver lesions on ultrasound images by extracting hybrid textural features and using an artificial neural network. BioMed Mater Eng. (2015) 26 Suppl 1:S1599–611. doi: 10.3233/BME-151459
20. Hassan TM, Elmogy M, Sallam ES. Diagnosis of focal liver diseases based on deep learning technique for ultrasound images. Arab J Sci Eng. (2017) 42:3127–40. doi: 10.1007/s13369-016-2387-9
21. Bharti P, Mittal D, Ananthasivan R. Preliminary study of chronic liver classification on ultrasound images using an ensemble model. Ultrason Imaging. (2018) 40:357–79. doi: 10.1177/0161734618787447
22. Schmauch B, Herent P, Jehanno P, Dehaene O, Saillard C, Aubé C, et al. Diagnosis of focal liver lesions from ultrasound using deep learning. Diagn Interv Imaging. (2019) 100:227–33. doi: 10.1016/j.diii.2019.02.009
23. Yang Q, Wei J, Hao X, Kong D, Yu X, Jiang T, et al. Improving B-mode ultrasound diagnostic performance for focal liver lesions using deep learning: A multicentre study. EBioMedicine. (2020) 56:102777. doi: 10.1016/j.ebiom.2020.102777
24. Brehar R, Mitrea DA, Vancea F, Marita T, Nedevschi S, Lupsor-Platon M, et al. Comparison of deep-learning and conventional machine-learning methods for the automatic recognition of the hepatocellular carcinoma areas from ultrasound images. Sensors (Basel). (2020) 20:3085. doi: 10.3390/s20113085
25. Mao B, Ma J, Duan S, Xia Y, Tao Y, Zhang L. Preoperative classification of primary and metastatic liver cancer via machine learning-based ultrasound radiomics. Eur Radiol. (2021) 31:4576–86. doi: 10.1007/s00330-020-07562-6
26. Ryu H, Shin SY, Lee JY, Lee KM, Kang HJ, Yi J. Joint segmentation and classification of hepatic lesions in ultrasound images using deep learning. Eur Radiol. (2021) 31:8733–42. doi: 10.1007/s00330-021-07850-9
27. Tiyarattanachai T, Apiparakoon T, Marukatat S, Sukcharoen S, Geratikornsupuk N, Anukulkarnkusol N, et al. Development and validation of artificial intelligence to detect and diagnose liver lesions from ultrasound images. PloS One. (2021) 16:e0252882. doi: 10.1371/journal.pone.0252882
28. Xi IL, Wu J, Guan J, Zhang PJ, Horii SC, Soulen MC, et al. Deep learning for differentiation of benign and Malignant solid liver lesions on ultrasonography. Abdom Radiol (NY). (2021) 46:534–43. doi: 10.1007/s00261-020-02564-w
29. Marya NB, Powers PD, Fujii-Lau L, Abu Dayyeh BK, Gleeson FC, Chen S, et al. Application of artificial intelligence using a novel EUS-based convolutional neural network model to identify and distinguish benign and Malignant hepatic masses. Gastrointest Endosc. (2021) 93:1121–30. doi: 10.1016/j.gie.2020.08.024
30. Ren S, Li Q, Liu S, Qi Q, Duan S, Mao B, et al. Clinical value of machine learning-based ultrasomics in preoperative differentiation between hepatocellular carcinoma and intrahepatic cholangiocarcinoma: A multicenter study. Front Oncol. (2021) 11:749137. doi: 10.3389/fonc.2021.749137
31. Ren S, Qi Q, Liu S, Duan S, Mao B, Chang Z, et al. Preoperative prediction of pathological grading of hepatocellular carcinoma using machine learning-based ultrasomics: A multicenter study. Eur J Radiol. (2021) 143:109891. doi: 10.1016/j.ejrad.2021.109891
32. Nishida N, Yamakawa M, Shiina T, Mekada Y, Nishida M, Sakamoto N, et al. Artificial intelligence (AI) models for the ultrasonographic diagnosis of liver tumors and comparison of diagnostic accuracies between AI and human experts. J Gastroenterol. (2022) 57:309–21. doi: 10.1007/s00535-022-01849-9
33. Zhang WB, Hou SZ, Chen YL, Mao F, Dong Y, Chen JG, et al. Deep learning for approaching hepatocellular carcinoma ultrasound screening dilemma: identification of α-fetoprotein-negative hepatocellular carcinoma from focal liver lesion found in high-risk patients. Front Oncol. (2022) 12:862297. doi: 10.3389/fonc.2022.862297
34. Wu JP, Ding WZ, Wang YL, Liu S, Zhang XQ, Yang Q, et al. Radiomics analysis of ultrasound to predict recurrence of hepatocellular carcinoma after microwave ablation. Int J Hyperthermia. (2022) 39:595–604. doi: 10.1080/02656736.2022.2062463
35. Jeon SK, Lee JM, Joo I, Yoon JH, Lee G. Two-dimensional convolutional neural network using quantitative US for noninvasive assessment of hepatic steatosis in NAFLD. Radiology. (2023) 307:e221510. doi: 10.1148/radiol.221510
36. Streba CT, Ionescu M, Gheonea DI, Sandulescu L, Ciurea T, Saftoiu A, et al. Contrast-enhanced ultrasonography parameters in neural network diagnosis of liver tumors. World J Gastroenterol. (2012) 18:4427–34. doi: 10.3748/wjg.v18.i32.4427
37. Wu K, Chen X, Ding M. Deep learning based classification of focal liver lesions with contrast-enhanced ultrasound. Optik. (2014) 125:4057–63. doi: 10.1016/j.ijleo.2014.01.114
38. Gatos I, Tsantis S, Spiliopoulos S, Skouroliakou A, Theotokas I, Zoumpoulis P, et al. A new automated quantification algorithm for the detection and evaluation of focal liver lesions with contrast-enhanced ultrasound. Med Phys. (2015) 42:3948–59. doi: 10.1118/1.4921753
39. Kondo S, Takagi K, Nishida M, Iwai T, Kudo Y, Ogawa K, et al. Computer-aided diagnosis of focal liver lesions using contrast-enhanced ultrasonography with perflubutane microbubbles. IEEE Trans Med Imaging. (2017) 36:1427–37. doi: 10.1109/TMI.2017.2659734
40. Guo LH, Wang D, Qian YY, Zheng X, Zhao CK, Li XL, et al. A two-stage multi-view learning framework based computer-aided diagnosis of liver tumors with contrast enhanced ultrasound images. Clin Hemorheol Microcirc. (2018) 69:343–54. doi: 10.3233/CH-170275
41. Ta CN, Kono Y, Eghtedari M, Oh YT, Robbin ML, Barr RG, et al. Focal liver lesions: computer-aided diagnosis by using contrast-enhanced US cine recordings. Radiology. (2018) 286:1062–71. doi: 10.1148/radiol.2017170365
42. Pan F, Huang Q, Li X. Classification of liver tumors with CEUS based on 3D-CNN. Int Confer Adv Rob Mechat. (2019), 845–9. doi: 10.1109/ICARM.2019.8834190
43. Huang Q, Pan F, Li W, Yuan F, Hu H, Huang J, et al. Differential diagnosis of atypical hepatocellular carcinoma in contrast-enhanced ultrasound using spatio-temporal diagnostic semantics. IEEE J BioMed Health Inform. (2020) 24:2860–9. doi: 10.1109/JBHI.2020.2977937
44. Căleanu CD, Sîrbu CL, Simion G. Deep neural architectures for contrast enhanced ultrasound (CEUS) focal liver lesions automated diagnosis. Sensors (Basel). (2021) 21:4126. doi: 10.3390/s21124126
45. Hu HT, Wang W, Chen LD, Ruan SM, Chen SL, Li X, et al. Artificial intelligence assists identifying Malignant versus benign liver lesions using contrast-enhanced ultrasound. J Gastroenterol Hepatol. (2021) 36:2875–83. doi: 10.1111/jgh.15522
46. Wang M, Fu F, Zheng B, Bai Y, Wu Q, Wu J, et al. Development of an AI system for accurately diagnose hepatocellular carcinoma from computed tomography imaging data. Br J Cancer. (2021) 125:1111–21. doi: 10.1038/s41416-021-01511-w
47. Turco S, Tiyarattanachai T, Ebrahimkheil K, Eisenbrey J, Kamaya A, Mischi M, et al. Interpretable machine learning for characterization of focal liver lesions by contrast-enhanced ultrasound. IEEE Trans Ultrason Ferroelectr Freq Control. (2022) 69:1670–81. doi: 10.1109/TUFFC.2022.3161719
48. Zhou Z, Xia T, Zhang T, Du M, Zhong J, Huang Y, et al. Prediction of preoperative microvascular invasion by dynamic radiomic analysis based on contrast-enhanced computed tomography. Abdom Radiol (NY). (2024) 49:611–24. doi: 10.1007/s00261-023-04102-w
49. Li W, Jia F, Hu Q. Automatic segmentation of liver tumor in CT images with deep convolutional neural networks. J Comput Commun. (2015) 3:146–51. doi: 10.4236/jcc.2015.311023
50. Vivanti R, Szeskin A, Lev-Cohain N, Sosna J, Joskowicz L. Automatic detection of new tumors and tumor burden evaluation in longitudinal liver CT scan studies. Int J Comput Assist Radiol Surg. (2017) 12:1945–57. doi: 10.1007/s11548-017-1660-z
51. Sun C, Guo S, Zhang H, Li J, Chen M, Ma S, et al. Automatic segmentation of liver tumors from multiphase contrast-enhanced CT images based on FCNS. Artif Intell Med. (2017) 83:58–66. doi: 10.1016/j.artmed.2017.03.008
52. Das A, Acharya UR, Panda SS, Sabut S. Deep learning based liver cancer detection using watershed transform and gaussian mixture model techniques. Cogn Syst Res. (2019) 54:165–75. doi: 10.1016/j.cogsys.2018.12.009
53. Ibragimov B, Toesca D, Chang D, Koong A, Xing L. Combining deep learning with anatomical analysis for segmentation of the portal vein for liver SBRT planning. Phys Med Biol. (2017) 62:8943–58. doi: 10.1088/1361-6560/aa9262
54. Chlebus G, Meine H, Moltz JH, Schenk A. Neural network-based automatic liver tumor segmentation with random forestbased candidate fltering. arXiv:170600842 [cs]. (2017). doi: 10.48550/arXiv.1706.00842
55. Tang W, Zou D, Yang S, Shi J. DSL: automatic liver segmentation with faster R-CNN and deepLab. In: Kůrková V, Manolopoulos Y, Hammer B, Iliadis L, Maglogiannis I, editors. Artificial Neural Networks and Machine Learning – ICANN 2018. ICANN 2018. Lecture Notes in Computer Science(), vol. vol 11140 . Springer, Cham (2018). doi: 10.1007/978-3-030-01421-6_14
56. Gibson E, Giganti F, Hu Y, Bonmati E, Bandula S, Gurusamy K, et al. Automatic multi-organ segmentation on abdominal CT with dense V-networks. IEEE Trans Med Imaging. (2018) 37:1822–34. doi: 10.1109/TMI.2018.2806309
57. Li X, Chen H, Qi X, Dou Q, Fu CW, Heng PA. H-DenseUNet: hybrid densely connected UNet for liver and tumor segmentation from CT volumes. IEEE Trans Med Imaging. (2018) 37:2663–74. doi: 10.1109/TMI.2018.2845918
58. Enokiya Y, Iwamoto Y, Chen YW. Automatic liver segmentation using U-Net with wasserstein GANs. JOIG. (2018) 6:152–9. doi: 10.18178/joig.6.2.152-159
59. Chen Y, Wang K, Liao X, Qian Y, Wang Q, Yuan Z, et al. Channel-Unet: A spatial channel-wise convolutional neural network for liver and tumors segmentation. Front Genet. (2019) 10:1110. doi: 10.3389/fgene.2019.01110
60. Song LI, Geoffrey K.F. TSO, Kaijian HE. Bottleneck feature supervised U-Net for pixel-wise liver and tumor segmentation. Expert Syst Appl. (2020) 145:113131. doi: 10.1016/j.eswa.2019.113131
61. Jin Q, Meng Z, Sun C, Cui H, Su R. RA-UNet: A hybrid deep attention-aware network to extract liver and tumor in CT scans. Front Bioeng Biotechnol. (2020) 8:605132. doi: 10.3389/fbioe.2020.605132
62. Tran S-T, Cheng C-H, Liu D-G. A Multiple Layer U-Net, Un-Net, for Liver and Liver Tumor Segmentation in CT Vol. 9. Piscataway, NJ, USA: IEEE Access (2021) p. 3752–64.
63. Zhang C, Ai D, Feng C, Fan J, Song H, Yang J. (2020). Dial/Hybrid cascade 3DResUNet for liver and tumor segmentation, in: Proceedings of the 2020 4th International Conference on Digital Signal Processing, ACM, New York, NY, USA. pp. 92–6.
64. Sakashita N, Shirai K, Ueda Y, Ono A, Teshima T. Convolutional neural network-based automatic liver delineation on contrast-enhanced and non-contrast-enhanced CT images for radiotherapy planning. Rep Pract Oncol Radiother. (2020) 25:981–6. doi: 10.1016/j.rpor.2020.09.005
65. Abdalbagi F, Viriri S, Mohammed MT. Bata-Unet: deep learning model for liver segmentation. Signal Image Processing: Int J (SIPIJ). (2020) 11, 227196252. doi: 10.5121/sipij.2020.11505
66. Affane A, Kucharski A, Chapuis P, Freydier S, Lebre M-A, Vacavant A, et al. Segmentation of liver anatomy by combining 3D U-net approaches. Appl Sci. (2021) 11:4895. doi: 10.3390/app11114895
67. Chi JN, Han XY, Wu CD, Wang H, Ji P. X-Net: Multi-branch UNet-like network for liver and tumor segmentation from 3D abdominal CT scans. Neurocomputing. (2021) 459:81–96. doi: 10.1016/j.neucom.2021.06.021
68. Zhang Y, Peng C, Peng L, Xu Y, Lin L, Tong R, et al. DeepRecS: from RECIST diameters to precise liver tumor segmentation. IEEE J BioMed Health Inform. (2022) 26:614–25. doi: 10.1109/JBHI.2021.3091900
69. Zhao Z, Ma Z, Liu Y, Zeng Z, Chow PK. Multi-slice dense-sparse learning for efficient liver and tumor segmentation. Annu Int Conf IEEE Eng Med Biol Soc. (2021) 2021:3582–5. doi: 10.1109/EMBC46164.2021.9629698
70. Wardhana G, Naghibi H, Sirmacek B, Abayazid M. Toward reliable automatic liver and tumor segmentation using convolutional neural network based on 2.5D models. Int J Comput Assist Radiol Surg. (2021) 16:41–51. doi: 10.1007/s11548-020-02292-y
71. Han L, Chen YH, Li JM, Zhong BW, Lei YZ, Sun MH. Liver segmentation with 2.5D perpendicular UNets. Comput Electrical Eng. (2021) 91:107118. doi: 10.1016/j.compeleceng.2021.107118
72. Liu Z, Han K, Wang Z, Zhang J, Song Y, Yao X, et al. Automatic liver segmentation from abdominal CT volumes using improved convolution neural networks. Multimedia Syst. (2021) 27:111–24. doi: 10.1007/s00530-020-00709-x
73. Fan T, Wang G, Wang X, Li Y, Wang H. MSN-Net: A multi-scale context nested U-Net for liver segmentation. SIViP. (2021) 15:1089–97. doi: 10.1007/s11760-020-01835-9
74. Islam M, Khan KN, Khan MS. (2021). Evaluation of preprocessing techniques for U-net based automated liver segmentation, in: 2021 International Conference on Artificial Intelligence (ICAI), Islamabad, Pakistan. IEEE, Piscataway, NJ, USA, pp. 187–92. doi: 10.1109/ICAI52203.2021.9445204
75. Araújo JDL, da Cruz LB, Diniz JOB, Ferreira JL, Silva AC, de Paiva AC, et al. Liver segmentation from computed tomography images using cascade deep learning. Comput Biol Med. (2022) 140:105095. doi: 10.1016/j.compbiomed.2021.105095
76. Senthilvelan J, Jamshidi N. A pipeline for automated deep learning liver segmentation (PADLLS) from contrast enhanced CT exams. Sci Rep. (2022) 12:15794. doi: 10.1038/s41598-022-20108-8
77. Jeong JG, Choi S, Kim YJ, Lee WS, Kim KG. Deep 3D attention CLSTM U-Net based automated liver segmentation and volumetry for the liver transplantation in abdominal CT volumes. Sci Rep. (2022) 12:6370. doi: 10.1038/s41598-022-09978-0
78. Pettit RW, Marlatt BB, Corr SJ, Havelka J, Rana A. nnU-net deep learning method for segmenting parenchyma and determining liver volume from computed tomography images. Ann Surg Open. (2022) 3:e155. doi: 10.1097/as9.0000000000000155
79. Khoshkhabar M, Meshgini S, Afrouzian R, Danishvar S. Automatic liver tumor segmentation from CT images using graph convolutional network. Sensors (Basel). (2023) 23:7561. doi: 10.3390/s23177561
80. Ananda S, Jain RK, Li Y, Iwamoto Y, Han XH, Kanasaki S, et al. A boundary-enhanced liver segmentation network for multi-phase CT images with unsupervised domain adaptation. Bioeng (Basel). (2023) 10:899. doi: 10.3390/bioengineering10080899
81. Jiang L, Ou J, Liu R, Zou Y, Xie T, Xiao H, et al. RMAU-Net: Residual Multi-Scale Attention U-Net For liver and tumor segmentation in CT images. Comput Biol Med. (2023) 158:106838. doi: 10.1016/j.compbiomed.2023.106838
82. Özcan F, Uçan ON, Karaçam S, Tunçman D. Fully automatic liver and tumor segmentation from CT image using an AIM-unet. Bioeng (Basel). (2023) 10:215. doi: 10.3390/bioengineering10020215
83. Wang J, Zhang X, Guo L, Shi C, Tamura S. Multi-scale attention and deep supervision-based 3D UNet for automatic liver segmentation from CT. Math Biosci Eng. (2023) 20:1297–316. doi: 10.3934/mbe.2023059
84. Li J, Liu K, Hu Y, Zhang H, Heidari AA, Chen H, et al. Eres-UNet++: Liver CT image segmentation based on high-efficiency channel attention and Res-UNet+. Comput Biol Med. (2023) 158:106501. doi: 10.1016/j.compbiomed.2022.106501
85. Yang Z, Li S. Dual-path network for liver and tumor segmentation in CT images using swin transformer encoding approach. Curr Med Imaging. (2023) 19:1114–23. doi: 10.2174/1573405619666221014114953
86. Song Z, Wu H, Chen W, Slowik A. Improving automatic segmentation of liver tumor images using a deep learning model. Heliyon. (2024) 10:e28538. doi: 10.1016/j.heliyon.2024.e28538
87. Yang S, Liang Y, Wu S, Sun P, Chen Z. SADSNet: A robust 3D synchronous segmentation network for liver and liver tumors based on spatial attention mechanism and deep supervision. J Xray Sci Technol. (2024) 32:707–23. doi: 10.3233/XST-230312
88. Huang S, Luo J, Ou Y, Shen W, Pang Y, Nie X, et al. SD-Net: a semi-supervised double-cooperative network for liver segmentation from computed tomography (CT) images. J Cancer Res Clin Oncol. (2024) 150:79. doi: 10.1007/s00432-023-05564-7
89. Guo S, Wang H, Agaian S, Han L, Song X. LRENet: a location-related enhancement network for liver lesions in CT images. Phys Med Biol. (2024) 69, 035019. doi: 10.1088/1361-6560/ad1d6b
90. Dou Q, Chen H, Jin Y, Yu L, Qin J, Heng PA. 3D deeply supervised network for automatic liver segmentation from CT volumes. In: Ourselin S, Joskowicz L, Sabuncu M, Unal G, Wells W, editors. Medical Image Computing and Computer-Assisted Intervention – MICCAI 2016. MICCAI 2016. Lecture Notes in Computer Science(), vol. vol 9901 . Springer, Cham (2016). doi: 10.1007/978-3-319-46723-8_18
91. Ben-Cohen A, Klang E, Kerpel A, Konen E, Amitai MM, Greenspan H. Fully convolutional network and sparsity-based dictionary learning for liver lesion detection in CT examinations. Neurocomputing. (2018) 275:1585–94. doi: 10.1016/j.neucom.2017.10.001
92. Lee Sg, Bae JS, Kim H, Kim JH, Yoon S. Liver lesion detection from weakly-labeled multi-phase CT volumes with a grouped single shot MultiBox detector. In: Frangi A, Schnabel J, Davatzikos C, Alberola-López C, Fichtinger G, editors. Medical Image Computing and Computer Assisted Intervention – MICCAI 2018. MICCAI 2018. Lecture Notes in Computer Science(), vol. vol 11071 . Springer, Cham (2018). doi: 10.1007/978-3-030-00934-2_77
93. Gruber N, Antholzer S, Jaschke W, Kremser C, Haltmeier M. (2019). A joint deep learning approach for automated liver and tumor segmentation, in: 2019 13th International conference on Sampling Theory and Applications (SampTA), Bordeaux, France. IEEE, Piscataway, NJ, USA, pp. 1–5. doi: 10.1109/SampTA45681.2019.9030909
94. Yu W, Fang B, Liu Y, Gao M, Zheng S, Wang Y. (2019). Liver vessels segmentation based on 3D residual U-NET, in: 2019 IEEE International Conference on Image Processing (ICIP), Taipei, Taiwan. IEEE, Piscataway, NJ, USA, pp. 250–4.
95. Budak Ü, Guo Y, Tanyildizi E, Şengür A. Cascaded deep convolutional encoder-decoder neural networks for efficient liver tumor segmentation. Med Hypotheses. (2020) 134:109431. doi: 10.1016/j.mehy.2019.109431
96. Almotairi S, Kareem G, Aouf M, Almutairi B, Salem MA. Liver tumor segmentation in CT scans using modified SegNet. Sensors (Basel). (2020) 20:1516. doi: 10.3390/s20051516
97. Hettihewa K, Kobchaisawat T, Tanpowpong N, Chalidabhongse TH. MANet: a multi-attention network for automatic liver tumor segmentation in computed tomography (CT) imaging. Sci Rep. (2023) 13:20098. doi: 10.1038/s41598-023-46580-4
98. Shui Y, Wang Z, Liu B, Wang W, Fu S, Li Y. A three-path network with multi-scale selective feature fusion, edge-inspiring and edge-guiding for liver tumor segmentation. Comput Biol Med. (2024) 168:107841. doi: 10.1016/j.compbiomed.2023.107841
99. Suganeshwari G, Appadurai JP, Kavin BP, C K, Lai WC. En-DeNet based segmentation and gradational modular network classification for liver cancer diagnosis. Biomedicines. (2023) 11:1309. doi: 10.3390/biomedicines11051309
100. Balasubramanian PK, Lai WC, Seng GH, C K, Selvaraj J. APESTNet with mask R-CNN for liver tumor segmentation and classification. Cancers (Basel). (2023) 15:330. doi: 10.3390/cancers15020330
101. Liu L, Wu K, Wang K, Han Z, Qiu J, Zhan Q, et al. SEU2-Net: multi-scale U2-Net with SE attention mechanism for liver occupying lesion CT image segmentation. PeerJ Comput Sci. (2024) 10:e1751. doi: 10.7717/peerj-cs.1751
102. Xu J, Jiang W, Wu J, Zhang W, Zhu Z, Xin J, et al. Hepatic and portal vein segmentation with dual-stream deep neural network. Med Phys. (2024) 51:5441–56. doi: 10.1002/mp.17090
103. Zhang H, Luo K, Deng R, Li S, Duan S. Deep learning-based CT imaging for the diagnosis of liver tumor. Comput Intell Neurosci. (2022) 2022:3045370. doi: 10.1155/2022/3045370
104. Xie T, Li Y, Lin Z, Liu X, Zhang X, Zhang Y, et al. Deep learning for fully automated segmentation and volumetry of Couinaud liver segments and future liver remnants shown with CT before major hepatectomy: a validation study of a predictive model. Quant Imaging Med Surg. (2023) 13:3088–103. doi: 10.21037/qims-22-1008
105. Wesdorp NJ, Zeeuw JM, Postma SCJ, Roor J, van Waesberghe JHTM, van den Bergh JE, et al. Deep learning models for automatic tumor segmentation and total tumor volume assessment in patients with colorectal liver metastases. Eur Radiol Exp. (2023) 7:75. doi: 10.1186/s41747-023-00383-4
106. Mokrane FZ, Lu L, Vavasseur A, Otal P, Peron JM, Luk L, et al. Radiomics machine-learning signature for diagnosis of hepatocellular carcinoma in cirrhotic patients with indeterminate liver nodules. Eur Radiol. (2020) 30:558–70. doi: 10.1007/s00330-019-06347-w
107. Khan AA, Narejo GB. Analysis of abdominal computed tomography images for automatic liver cancer diagnosis using image processing algorithm. Curr Med Imaging Rev. (2019) 15:972–82. doi: 10.2174/1573405615666190716122040
108. Li J, Wu Y, Shen N, Zhang J, Chen E, Sun J, et al. A fully automatic computer-aided diagnosis system for hepatocellular carcinoma using convolutional neural networks. Biocybern BioMed Eng. (2020) 40:238–48. doi: 10.1016/j.bbe.2019.05.008
109. Shi W, Kuang S, Cao S, Hu B, Xie S, Chen S, et al. Deep learning assisted differentiation of hepatocellular carcinoma from focal liver lesions: choice of four-phase and three-phase CT imaging protocol. Abdom Radiol (NY). (2020) 45:2688–97. doi: 10.1007/s00261-020-02485-8
110. Yasaka K, Akai H, Abe O, Kiryu S. Deep learning with convolutional neural network for differentiation of liver masses at dynamic contrast-enhanced CT: A preliminary study. Radiology. (2018) 286:887–96. doi: 10.1148/radiol.2017170706
111. Todoroki Y, Iwamoto Y, Lin L, Hu H, Chen YW. Automatic detection of focal liver lesions in multi-phase CT images using A multi-channel & Multi-scale CNN. Annu Int Conf IEEE Eng Med Biol Soc. (2019) 2019:872–5. doi: 10.1109/EMBC.2019.8857292
112. Zhou J, Wang W, Lei B, Ge W, Huang Y, Zhang L, et al. Automatic detection and classification of focal liver lesions based on deep convolutional neural networks: A preliminary study. Front Oncol. (2021) 10:581210. doi: 10.3389/fonc.2020.581210
113. Ponnoprat D, Inkeaw P, Chaijaruwanich J, Traisathit P, Sripan P, Inmutto N, et al. Classification of hepatocellular carcinoma and intrahepatic cholangiocarcinoma based on multi-phase CT scans. Med Biol Eng Comput. (2020) 58:2497–515. doi: 10.1007/s11517-020-02229-2
114. Krishan A, Mittal D. Ensembled liver cancer detection and classification using CT images. Proc Inst Mech Eng H. (2021) 235:232–44. doi: 10.1177/0954411920971888
115. Manjunath RV, Ghanshala A, Kwadiki K. Deep learning algorithm performance evaluation in detection and classification of liver disease using CT images. Multimed Tools Appl. (2023) 15:1–18. doi: 10.1007/s11042-023-15627-z
116. Phan DV, Chan CL, Li AA, Chien TY, Nguyen VC. Liver cancer prediction in a viral hepatitis cohort: A deep learning approach. Int J Cancer. (2020) 147:2871–8. doi: 10.1002/ijc.33245
117. Wang W, Wu SS, Zhang JC, Xian MF, Huang H, Li W, et al. Preoperative pathological grading of hepatocellular carcinoma using ultrasomics of contrast-enhanced ultrasound. Acad Radiol. (2021) 28:1094–101. doi: 10.1016/j.acra.2020.05.033
118. Gao R, Zhao S, Aishanjiang K, Cai H, Wei T, Zhang Y, et al. Deep learning for differential diagnosis of Malignant hepatic tumors based on multi-phase contrastenhanced CT and clinical data. J Hematol Oncol. (2021) 14:154. doi: 10.1186/s13045-021-01167-2
119. Shah S, Mishra R, Szczurowska A, Guziński M. Non-invasive multi-channel deep learning convolutional neural networks for localization and classification of common hepatic lesions. Pol J Radiol. (2021) 86:e440–8. doi: 10.5114/pjr.2021.108257
120. Lee H, Lee H, Hong H, Bae H, Lim JS, Kim J. Classification of focal liver lesions in CT images using convolutional neural networks with lesion information augmented patches and synthetic data augmentation. Med Phys. (2021) 48:5029–46. doi: 10.1002/mp.15118
121. Kim DW, Lee G, Kim SY, Ahn G, Lee JG, Lee SS, et al. Deep learning-based algorithm to detect primary hepatic Malignancy in multiphase CT of patients at high risk for HCC. Eur Radiol. (2021) 31:7047–57. doi: 10.1007/s00330-021-07803-2
122. Nakai H, Fujimoto K, Yamashita R, Sato T, Someya Y, Taura K, et al. Convolutional neural network for classifying primary liver cancer based on triple-phase CT and tumor marker information: a pilot study. Jpn J Radiol. (2021) 39:690–702. doi: 10.1007/s11604-021-01106-8
123. Zhao X, Liang P, Yong L, Jia Y, Gao J. Radiomics study for differentiating focal hepatic lesions based on unenhanced CT images. Front Oncol. (2022) 12:650797. doi: 10.3389/fonc.2022.650797
124. Naaqvi Z, Akbar S, Hassan SA, Ul Ain Q. (2022). Detection of Liver Cancer through Computed Tomography Images using Deep Convolutional Neural Networks, in: 2022 2nd International Conference on Digital Futures and Transformative Technologies (ICoDT2), Rawalpindi, Pakistan. pp. 1–6. doi: 10.1109/ICoDT255437.2022.9787429
125. Li S, Yuan L, Lu T, Yang X, Ren W, Wang L, et al. Deep learning imaging reconstruction of reduced-dose 40 keV virtual monoenergetic imaging for early detection of colorectal cancer liver metastases. Eur J Radiol. (2023) 168:111128. doi: 10.1016/j.ejrad.2023.111128
126. Mulé S, Ronot M, Ghosn M, Sartoris R, Corrias G, Reizine E, et al. Automated CT LI-RADS v2018 scoring of liver observations using machine learning: A multivendor, multicentre retrospective study. JHEP Rep. (2023) 5:100857. doi: 10.1016/j.jhepr.2023.100857
127. Kang HJ, Lee JM, Ahn C, Bae JS, Han S, Kim SW, et al. Low dose of contrast agent and low radiation liver computed tomography with deep-learning-based contrast boosting model in participants at high-risk for hepatocellular carcinoma: prospective, randomized, double-blind study. Eur Radiol. (2023) 33:3660–70. doi: 10.1007/s00330-023-09520-4
128. Lee IC, Tsai YP, Lin YC, Chen TC, Yen CH, Chiu NC, et al. A hierarchical fusion strategy of deep learning networks for detection and segmentation of hepatocellular carcinoma from computed tomography images. Cancer Imaging. (2024) 24:43. doi: 10.1186/s40644-024-00686-8
129. Bilello M, Gokturk SB, Desser T, Napel S, Jeffrey RB Jr, Beaulieu CF. Automatic detection and classification of hypodense hepatic lesions on contrast-enhanced venous-phase CT. Med Phys. (2004) 31:2584–93. doi: 10.1118/1.1782674
130. Cannella R, Borhani AA, Minervini MI, Tsung A, Furlan A. Evaluation of texture analysis for the differential diagnosis of focal nodular hyperplasia from hepatocellular adenoma on contrast-enhanced CT images. Abdom Radiol (NY). (2019) 44:1323–30. doi: 10.1007/s00261-018-1788-5
131. Peng J, Huang J, Huang G, Zhang J. Predicting the initial treatment response to transarterial chemoembolization in intermediate-stage hepatocellular carcinoma by the integration of radiomics and deep learning. Front Oncol. (2021) 11:730282. doi: 10.3389/fonc.2021.730282
132. Yang Y, Zhou Y, Zhou C, Ma X. Deep learning radiomics based on contrast enhanced computed tomography predicts microvascular invasion and survival outcome in early stage hepatocellular carcinoma. Eur J Surg Oncol. (2022) 48:1068–77. doi: 10.1016/j.ejso.2021.11.120
133. Fu S, Lai H, Huang M, Li Q, Liu Y, Zhang J, et al. Multi-task deep learning network to predict future macrovascular invasion in hepatocellular carcinoma. EClinicalMedicine. (2021) 42:101201. doi: 10.1016/j.eclinm.2021.101201
134. Pino C, Vecchio G, Fronda M, Calandri M, Aldinucci M, Spampinato C. TwinLiverNet: Predicting TACE Treatment Outcome from CT scans for Hepatocellular Carcinoma using Deep Capsule Networks. Annu Int Conf IEEE Eng Med Biol Soc. (2021) 2021:3039–43. doi: 10.1109/EMBC46164.2021.9630913
135. Ji G-W, Zhu F-P, Xu Q, Wang K, Wu M-Y, Tang W-W, et al. Machine-learning analysis of contrast-enhanced CT radiomics predicts recurrence of hepatocellular carcinoma after resection: A multi-institutional study. EBioMedicine. (2019) 50:156–65. doi: 10.1016/j.ebiom.2019.10.057
136. Mao B, Zhang L, Ning P, Ding F, Wu F, Lu G, et al. Preoperative prediction for pathological grade of hepatocellular carcinoma via machine learning-based radiomics. Eur Radiol. (2020) 30:6924–32. doi: 10.1007/s00330-020-07056-5
137. Wei J, Jiang H, Zeng M, Wang M, Niu M, Gu D, et al. Prediction of microvascular invasion in hepatocellular carcinoma via deep learning: A multi-center and prospective validation study. Cancers (Basel). (2021) 13:2368. doi: 10.3390/cancers13102368
138. Wang F, Chen Q, Chen Y, Zhu Y, Zhang Y, Cao D, et al. A novel multimodal deep learning model for preoperative prediction of microvascular invasion and outcome in hepatocellular carcinoma. Eur J Surg Oncol. (2023) 49:156–64. doi: 10.1016/j.ejso.2022.08.036
139. Hossain MSA, Gul S, Chowdhury MEH, Khan MS, Sumon MSI, Bhuiyan EH, et al. Deep learning framework for liver segmentation from T1-weighted MRI images. Sensors (Basel). (2023) 23:8890. doi: 10.3390/s23218890
140. Gross M, Huber S, Arora S, Ze’evi T, Haider S, Kucukkaya A, et al. Automated MRI liver segmentation for anatomical segmentation, liver volumetry, and the extraction of radiomics. Eur Radiol. (2024) 34:5056–65. doi: 10.1007/s00330-023-10495-5
141. Masoumi H, Behrad A, Pourmina MA, Roosta A. Automatic liver segmentation in MRI images using an iterative watershed algorithm and artificial neural network. Biomed Signal Process Control. (2012) 7:429–37. doi: 10.1016/j.bspc.2012.01.002
142. Le TN, Bao PT, Huynh HT. Liver tumor segmentation from MR images using 3D fast marching algorithm and single hidden layer feedforward neural network. BioMed Res Int. (2016) 2016:3219068. doi: 10.1155/2016/3219068
143. Chlebus G, Meine H, Abolmaali N, Schenk A. Automatic liver and tumor segmentation in late-phase MRI using fully convolutional neural networks. Proc CURAC. (2018), 195–200. Available at: https://www.researchgate.net/publication/327732676_Automatic_Liver_and_Tumor_Segmentation_in_Late-Phase_MRI_Using_Fully_Convolutional_Neural_Networks
144. Wang K, Mamidipalli A, Retson T, Bahrami N, Hasenstab K, Blansit K, et al. Automated CT and MRI liver segmentation and biometry using a generalized convolutional neural network. Radiol Artif Intell. (2019) 1:180022. doi: 10.1148/ryai.2019180022
145. Xiao X, Qiang Y, Zhao J, Yang X, Yang X. Segmentation of liver lesions without contrast agents with radiomics-guided densely UNet-nested GAN. IEEE Access. (2021) 9:2864–78. doi: 10.1109/ACCESS.2020.3047429
146. Ivashchenko OV, Rijkhorst E-J, ter Beek LC, Hoetjes NJ, Pouw B, Nijkamp J, et al. A workflow for automated segmentation of the liver surface, hepatic vasculature and biliary tree anatomy from multiphase MR images. Magnetic Resonance Imaging. (2020) 68:53–65. doi: 10.1016/j.mri.2019.12.008
147. Liu M, Vanguri R, Mutasa S, Ha R, Liu YC, Button T, et al. Channel width optimized neural networks for liver and vessel segmentation in liver iron quantification. Comput Biol Med. (2020) 122:103798. doi: 10.1016/j.compbiomed.2020.103798
148. Bousabarah K, Letzen B, Tefera J, Savic L, Schobert I, Schlachter T, et al. Automated detection and delineation of hepatocellular carcinoma on multiphasic contrast enhanced MRI using deep learning. Abdom Radiol. (2021) 46:216–25. doi: 10.1007/s00261-020-02604-5
149. Nowak S, Mesropyan N, Faron A, Block W, Reuter M, Attenberger UI, et al. Detection of liver cirrhosis in standard T2-weighted MRI using deep transfer learning. Eur Radiol. (2021) 31:8807–15. doi: 10.1007/s00330-021-07858-1
150. Zhao J, Li D, Xiao X, Accorsi F, Marshall H, Cossetto T, et al. United adversarial learning for liver tumor segmentation and detection of multi-modality non-contrast MRI. Med Image Anal. (2021) 73:102154. doi: 10.1016/j.media.2021.102154
151. Zheng R, Wang Q, Lv S, Li C, Wang C, Chen W, et al. Automatic liver tumor segmentation on dynamic contrast enhanced MRI using 4D information: deep learning model based on 3D convolution and convolutional LSTM. IEEE Trans Med Imaging. (2022) 41:2965–76. doi: 10.1109/TMI.2022.3175461
152. Han X, Wu X, Wang S, Xu L, Xu H, Zheng D, et al. Automated segmentation of liver segment on portal venous phase MR images using a 3D convolutional neural network. Insights Imaging. (2022) 13:26. doi: 10.1186/s13244-022-01163-1
153. Zbinden L, Catucci D, Suter Y, Berzigotti A, Ebner L, Christe A, et al. Convolutional neural network for automated segmentation of the liver and its vessels on non-contrast T1 vibe Dixon acquisitions. Sci Rep. (2022) 12:22059. doi: 10.1038/s41598-022-26328-2
154. Wang J, Peng Y, Jing S, Han L, Li T, Luo J. A deep-learning approach for segmentation of liver tumors in magnetic resonance imaging using UNet++. BMC Cancer. (2023) 23:1060. doi: 10.1186/s12885-023-11432-x
155. Zbinden L, Catucci D, Suter Y, Hulbert L, Berzigotti A, Brönnimann M, et al. Automated liver segmental volume ratio quantification on non-contrast T1–Vibe Dixon liver MRI using deep learning. Eur J Radiol. (2023) 167:111047. doi: 10.1016/j.ejrad.2023.111047
156. Oh N, Kim JH, Rhu J, Jeong WK, Choi GS, Kim JM, et al. 3D auto-segmentation of biliary structure of living liver donors using magnetic resonance cholangiopancreatography for enhanced preoperative planning. Int J Surg. (2024) 110:1975–82. doi: 10.1097/JS9.0000000000001067
157. Fallahpoor M, Nguyen D, Montahaei E, Hosseini A, Nikbakhtian S, Naseri M, et al. Segmentation of liver and liver lesions using deep learning. Phys Eng Sci Med. (2024) 47:611–9. doi: 10.1007/s13246-024-01390-4
158. Hamm CA, Wang CJ, Savic LJ, Ferrante M, Schobert I, Schlachter T, et al. Deep learning for liver tumor diagnosis part i: development of a convolutional neural network classifier for multi-phasic MRI. Eur Radiol. (2019) 29:3338–47. doi: 10.1007/s00330-019-06205-9
159. Wang CJ, Hamm CA, Letzen BS, Duncan JS. A probabilistic approach for interpretable deep learning in liver cancer diagnosis. Proc SPIE 10950 Med Imaging 2019: Computer-Aided Diagnosis 109500U. (2019). doi: 10.1117/12.2512473
160. Trivizakis E, Manikis GG, Nikiforaki K, Drevelegas K, Constantinides M, Drevelegas A, et al. Extending 2-D convolutional neural networks to 3-D for advancing deep learning cancer classification with application to MRI liver tumor differentiation. IEEE J BioMed Health Inf. (2019) 23:923–30. doi: 10.1109/JBHI.6221020
161. Zhen SH, Cheng M, Tao YB, Wang YF, Juengpanich S, Jiang ZY, et al. Deep learning for accurate diagnosis of liver tumor based on magnetic resonance imaging and clinical data. Front Oncol. (2020) 10:680. doi: 10.3389/fonc.2020.00680
162. Kim J, Min JH, Kim SK, Shin SY, Lee MW. Detection of hepatocellular carcinoma in contrast-enhanced magnetic resonance imaging using deep learning classifier: a multi-center retrospective Study. Sci Rep. (2020) 10:9458. doi: 10.1038/s41598-020-65875-4
163. Wan Y, Zheng Z, Liu R, Zhu Z, Zhou H, Zhang X, et al. A multi-scale and multi-level fusion approach for deep learning-based liver lesion diagnosis in magnetic resonance images with visual explanation. Life. (2021) 11:582. doi: 10.3390/life11060582
164. Oestmann PM, Wang CJ, Savic LJ, Hamm CA, Stark S, Schobert I, et al. Deep learning-assisted differentiation of pathologically proven atypical and typical hepatocellular carcinoma (HCC) versus non-HCC on contrast-enhanced MRI of the liver. Eur Radiol. (2021) 31:4981–90. doi: 10.1007/s00330-020-07559-1
165. Jansen MJA, Kuijf HJ, Veldhuis WB, Wessels FJ, Viergever MA, Pluim JPW. Automatic classification of focal liver lesions based on MRI and risk factors. PloS One. (2019) 14:e0217053. doi: 10.1371/journal.pone.0217053
166. Hamm CA, Beetz NL, Savic LJ, Penzkofer T. [Artificial intelligence and radiomics in MRI-based prostate diagnostics. Radiologe. (2020) 60:48–55. doi: 10.1007/s00117-019-00613-0
167. Lin Y-S, Huang P-H, Chen Y-Y. Deep learning-based hepatocellular carcinoma histopathology image classification: accuracy versus training dataset size. IEEE Access. (2021) 9:33144–57. doi: 10.1109/ACCESS.2021.3060765
168. Gao F, Qiao K, Yan B, Wu M, Wang L, Jian Chen J, et al. Hybrid network with difference degree and attention mechanism combined with radiomics (H-DARnet) for MVI prediction in HCC. Magn Reson Imaging. (2021) 83:27–40. doi: 10.1016/j.mri.2021.06.018
169. Chen M, Kong C, Qiao E, Chen Y, Chen W, Jiang X, et al. Multi-algorithms analysis for pretreatment prediction of response to transarterial chemoembolization in hepatocellular carcinoma on multiphase MRI. Insights Imaging. (2023) 14:38. doi: 10.1186/s13244-023-01380-2
170. He T, Fong JN, Moore LW, Ezeana CF, Victor D, Divatia M, et al. An imageomics and multi-network based deep learning model for risk assessment of liver transplantation for hepatocellular cancer. Comput Med Imaging Graph. (2021) 89:101894. doi: 10.1016/j.compmedimag.2021.101894
171. Tian Y, Komolafe TE, Zheng J, Zhou G, Chen T, Zhou B, et al. Assessing PD-L1 expression level via preoperative MRI in HCC based on integrating deep learning and radiomics features. Diagnost (Basel). (2021) 11:1875. doi: 10.3390/diagnostics11101875
172. Wang T, Li Z, Yu H, Duan C, Feng W, Chang L, et al. Prediction of microvascular invasion in hepatocellular carcinoma based on preoperative Gd-EOB-DTPA-enhanced MRI: Comparison of predictive performance among 2D, 2D-expansion and 3D deep learning models. Front Oncol. (2023) 13:987781. doi: 10.3389/fonc.2023.987781
173. Hille G, Agrawal S, Tummala P, Wybranski C, Pech M, Surov A, et al. Joint liver and hepatic lesion segmentation in MRI using a hybrid CNN with transformer layers. Comput Methods Programs Biomed. (2023) 240:107647. doi: 10.1016/j.cmpb.2023.107647
174. Yang J, Dvornek NC, Zhang F, Zhuang J, Chapiro J, Lin M, et al. Domain-agnostic learning with anatomy-consistent embedding for cross-modality liver segmentation. IEEE Int Conf Comput Vis Workshops. (2019) 2019:10. doi: 10.1109/iccvw.2019.00043
175. Hong J, Yu SC-H, Chen W. Unsupervised domain adaptation for cross-modality liver segmentation via joint adversarial learning and self-learning. Appl Soft Computing. (2022) 121:108729. doi: 10.1016/j.asoc.2022.108729
176. Menegotto AB, Lopes Becker CD, Cazella SC. Computer-aided hepatocarcinoma diagnosis using multimodal deep learning. In: Novais P, Lloret J, Chamoso P, Carneiro D, Navarro E, Omatu S, editors. Ambient Intelligence – Software and Applications–10th International Symposium on Ambient Intelligence. ISAmI 2019. Advances in Intelligent Systems and Computing. Springer, Cham (2020). p. Vol. 1006.
177. Menegotto AB, Becker CDL, Cazella SC. Computer-aided diagnosis of hepatocellular carcinoma fusing imaging and structured health data. Health Inf Sci Syst. (2021) 9:20. doi: 10.1007/s13755-021-00151-x
178. Gao R, Zhao S, Aishanjiang K, Cai H, Wei T, Zhang Y, et al. Deep learning for differential diagnosis of Malignant hepatic tumors based on multi-phase contrast-enhanced CT and clinical data. J Hematol Oncol. (2021) 14:154. doi: 10.1186/s13045-021-01167-2
179. Li W, Lv XZ, Zheng X, Ruan SM, Hu HT, Chen LD, et al. Machine learning-based ultrasomics improves the diagnostic performance in differentiating focal nodular hyperplasia and atypical hepatocellular carcinoma. Front Oncol. (2021) 11:544979. doi: 10.3389/fonc.2021.544979
180. Liu L, Tang C, Li L, Chen P, Tan Y, Hu X, et al. Deep learning radiomics for focal liver lesions diagnosis on long-range contrast-enhanced ultrasound and clinical factors. Quant Imaging Med Surg. (2022) 12:3213–26. doi: 10.21037/qims-21-1004
181. Khan RA, Fu M, Burbridge B, Luo Y, Wu F-X. A multi-modal deep neural network for multi-class liver cancer diagnosis. Neural Networks. (2023) 165:553–61. doi: 10.1016/j.neunet.2023.06.013
182. Liu QP, Xu X, Zhu FP, Zhang YD, Liu XS. Prediction of prognostic risk factors in hepatocellular carcinoma with transarterial chemoembolization using multi-modal multi-task deep learning. EClinicalMedicine. (2020) 23:100379. doi: 10.1016/j.eclinm.2020.100379
183. Sun Z, Shi Z, Xin Y, Zhao S, Jiang H, Wang D, et al. Artificial intelligent multi-modal point-of-care system for predicting response of transarterial chemoembolization in hepatocellular carcinoma. Front Bioeng Biotechnol. (2021) 9:761548. doi: 10.3389/fbioe.2021.761548
184. Song D, Wang Y, Wang W, Wang Y, Cai J, Zhu K, et al. Using deep learning to predict microvascular invasion in hepatocellular carcinoma based on dynamic contrast-enhanced MRI combined with clinical parameters. J Cancer Res Clin Oncol. (2021) 147:3757–67. doi: 10.1007/s00432-021-03617-3
185. Jia X, Sun Z, Mi Q, Yang Z, Yang D. A multimodality-contribution-aware TripNet for histologic grading of hepatocellular carcinoma. IEEE/ACM Trans Comput Biol Bioinform. (2022) 19:2003–16. doi: 10.1109/TCBB.2021.3079216
186. Hu X, Zhou J, Li Y, Wang Y, Guo J, Sack I, et al. Added value of viscoelasticity for MRI-based prediction of Ki-67 expression of hepatocellular carcinoma using a deep learning combined radiomics (DLCR) model. Cancer. (2022) 14:2575. doi: 10.3390/cancers14112575
187. Wei H, Zheng T, Zhang X, Zheng C, Jiang D, Wu Y, et al. Deep learning-based 3D quantitative total tumor burden predicts early recurrence of BCLC A and B HCC after resection. Eur Radiol. (2024). doi: 10.1007/s00330-024-10941-y
188. Li Z, Ma B, Shui S, Tu Z, Peng W, Chen Y, et al. An integrated platform for decoding hydrophilic peptide fingerprints of hepatocellular carcinoma using artificial intelligence and two-dimensional nanosheets. J Mater Chem B. (2024) 12:7532–42. doi: 10.1039/d4tb00700j
189. Wu Y, Zhuo C, Lu Y, Luo Z, Lu L, Wang J, et al. A machine learning clinic scoring system for hepatocellular carcinoma based on the Surveillance, Epidemiology, and End Results database. J Gastrointest Oncol. (2024) 15:1082–100. doi: 10.21037/jgo-24-230
190. Park IG, Yoon SJ, Won SM, Oh KK, Hyun JY, Suk KT, et al. Gut microbiota-based machine-learning signature for the diagnosis of alcohol-associated and metabolic dysfunction-associated steatotic liver disease. Sci Rep. (2024) 14:16122. doi: 10.1038/s41598-024-60768-2
191. Geng Z, Wang S, Ma L, Zhang C, Guan Z, Zhang Y, et al. Prediction of microvascular invasion in hepatocellular carcinoma patients with MRI radiomics based on susceptibility weighted imaging and T2-weighted imaging. Radiol Med. (2024) 129:1130–42. doi: 10.1007/s11547-024-01845-4
192. Liu X, Hou Y, Wang X, Yu L, Wang X, Jiang L, et al. Machine learning-based development and validation of a scoring system for progression-free survival in liver cancer. Hepatol Int. (2020) 14:567–76. doi: 10.1007/s12072-020-10046-w
193. Phan AC, Ngoan Trieu T, Cang Phan T. Hounsfield unit variations-based liver lesions detection and classification using deep learning. Curr Med Imaging. (2023) 20:e280423216354. doi: 10.2174/1573405620666230428121748
194. Ou J, Jiang L, Bai T, Zhan P, Liu R, Xiao H. ResTransUnet: An effective network combined with Transformer and U-Net for liver segmentation in CT scans. Comput Biol Med. (2024) 177:108625. doi: 10.1016/j.compbiomed.2024.108625
195. Nakao Y, Nishihara T, Sasaki R, Fukushima M, Miuma S, Miyaaki H, et al. Investigation of deep learning model for predicting immune checkpoint inhibitor treatment efficacy on contrast-enhanced computed tomography images of hepatocellular carcinoma. Sci Rep. (2024) 14:6576. doi: 10.1038/s41598-024-57078-y
196. Li S, Jiang H, Pang W. Joint multiple fully connected convolutional neural network with extreme learning machine for hepatocellular carcinoma nuclei grading. Comput Biol Med. (2017) 84:156–67. doi: 10.1016/j.compbiomed.2017.03.017
197. Park J, Bae JS, Kim JM, Witanto JN, Park SJ, Lee JM. Development of a deep-learning model for classification of LI-RADS major features by using subtraction images of MRI: a preliminary study. Abdom Radiol (NY). (2023) 48:2547–56. doi: 10.1007/s00261-023-03962-6
198. Qu WF, Tian MX, Lu HW, Zhou YF, Liu WR, Tang Z, et al. Development of a deep pathomics score for predicting hepatocellular carcinoma recurrence after liver transplantation. Hepatol Int. (2023) 17:927–41. doi: 10.1007/s12072-023-10511-2
199. Liu Y, Zhang Z, Zhang H, Wang X, Wang K, Yang R, et al. Clinical prediction of microvascular invasion in hepatocellular carcinoma using an MRI-based graph convolutional network model integrated with nomogram. Br J Radiol. (2024) 97:938–46. doi: 10.1093/bjr/tqae056
200. Han Y, Akhtar J, Liu G, Li C, Wang G. Early warning and diagnosis of liver cancer based on dynamic network biomarker and deep learning. Comput Struct Biotechnol J. (2023) 21:3478–89. doi: 10.1016/j.csbj.2023.07.002
201. Zhao J, Li D, Kassam Z, Howey J, Chong J, Chen B, et al. Tripartite-GAN: Synthesizing liver contrast-enhanced MRI to improve tumor detection. Med Image Anal. (2020) 63:101667. doi: 10.1016/j.media.2020.101667
202. Yan M, Zhang X, Zhang B, Geng Z, Xie C, Yang W, et al. Deep learning nomogram based on Gd-EOB-DTPA MRI for predicting early recurrence in hepatocellular carcinoma after hepatectomy. Eur Radiol. (2023) 33:4949–61. doi: 10.1007/s00330-023-09419-0
203. Liu Y, Lu M, Zhong JP. Magan: mask attention generative adversarial network for liver tumor CT image synthesis. J Healthc Eng. (2021) 2021:6675259. doi: 10.21203/rs.3.rs-41685/v1
204. Hanna RF, Miloushev VZ, Tang A, Finklestone LA, Brejt SZ, Sandhu RS, et al. Comparative 13-year meta-analysis of the sensitivity and positive predictive value of ultrasound, CT, and MRI for detecting hepatocellular carcinoma. Abdom Radiol (NY). (2016) 41:71–90. doi: 10.1007/s00261-015-0592-8
205. Lakshmipriya B, Pottakkat B, Ramkumar G. Deep learning techniques in liver tumour diagnosis using CT and MR imaging-A systematic review. Artif Intell Med. (2023) 29:102557. doi: 10.1016/j.artmed.2023.102557
206. Wei Q, Tan N, Xiong S, Luo W, Xia H, Luo B. Deep learning methods in medical image-based hepatocellular carcinoma diagnosis: a systematic review and meta-analysis. Cancers. (2023) 15:5701. doi: 10.3390/cancers15235701
207. Velichko YS, Gennaro N, Karri M, Antalek M, Bagci U. A comprehensive review of deep learning approaches for magnetic resonance imaging liver tumor analysis. Adv Clin Radiol. (2023) 5:1–5. doi: 10.1016/j.yacr.2023.06.001
Keywords: artificial intelligence, deep learning, machine learning, liver cancer, hepatocellular carcinoma, medical imaging, diagnosis, prediction
Citation: Wang L, Fatemi M and Alizad A (2024) Artificial intelligence techniques in liver cancer. Front. Oncol. 14:1415859. doi: 10.3389/fonc.2024.1415859
Received: 11 April 2024; Accepted: 15 August 2024;
Published: 03 September 2024.
Edited by:
Moti Freiman, Technion Israel Institute of Technology, IsraelReviewed by:
Jitendra Kuldeep, Cancer Research Center of Marseille, FranceCopyright © 2024 Wang, Fatemi and Alizad. This is an open-access article distributed under the terms of the Creative Commons Attribution License (CC BY). The use, distribution or reproduction in other forums is permitted, provided the original author(s) and the copyright owner(s) are credited and that the original publication in this journal is cited, in accordance with accepted academic practice. No use, distribution or reproduction is permitted which does not comply with these terms.
*Correspondence: Lulu Wang, bHdhbmczODFAaG90bWFpbC5jb20=; d2FuZy5sdWx1QG1heW8uZWR1
Disclaimer: All claims expressed in this article are solely those of the authors and do not necessarily represent those of their affiliated organizations, or those of the publisher, the editors and the reviewers. Any product that may be evaluated in this article or claim that may be made by its manufacturer is not guaranteed or endorsed by the publisher.
Research integrity at Frontiers
Learn more about the work of our research integrity team to safeguard the quality of each article we publish.