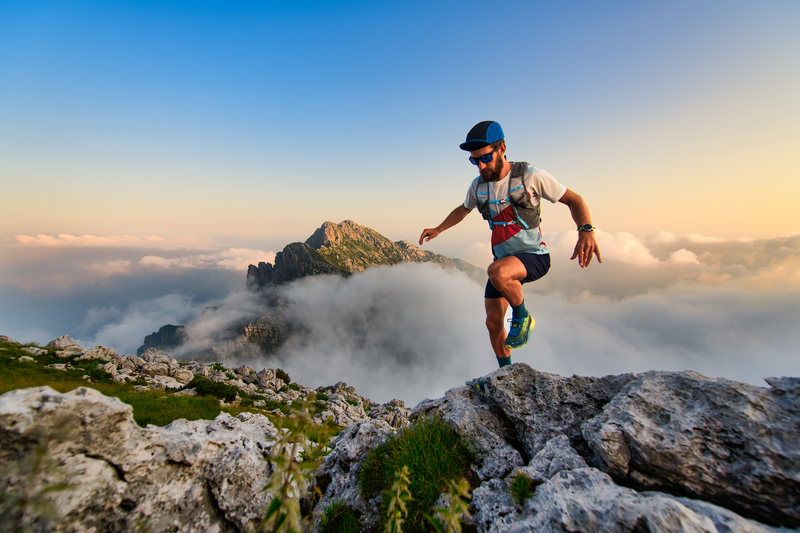
94% of researchers rate our articles as excellent or good
Learn more about the work of our research integrity team to safeguard the quality of each article we publish.
Find out more
REVIEW article
Front. Oncol. , 29 May 2024
Sec. Cancer Immunity and Immunotherapy
Volume 14 - 2024 | https://doi.org/10.3389/fonc.2024.1407580
This article is part of the Research Topic Cell Death in Cancer Immunology View all 15 articles
Despite that colorectal and liver cancer are among the most prevalent tumours in the world, the identification of non-invasive biomarkers to aid on their diagnose and subsequent prognosis is a current unmet need that would diminish both their incidence and mortality rates. In this context, conventional flow cytometry has been widely used in the screening of biomarkers with clinical utility in other malignant processes like leukaemia or lymphoma. Therefore, in this review, we will focus on how advanced cytometry panels covering over 40 parameters can be applied on the study of the immune system from patients with colorectal and hepatocellular carcinoma and how that can be used on the search of novel biomarkers to aid or diagnose, prognosis, and even predict clinical response to different treatments. In addition, these multiparametric and unbiased approaches can also provide novel insights into the specific immunopathogenic mechanisms governing these malignant diseases, hence potentially unravelling novel targets to perform immunotherapy or identify novel mechanisms, rendering the development of novel treatments. As a consequence, computational cytometry approaches are an emerging methodology for the early detection and predicting therapies for gastrointestinal cancers.
Currently, and according to the International Agency for Research on Cancer (1), the most prevalent cancers in the world are lung, breast, colorectal, prostate, stomach, and liver cancers, respectively. Although lung cancer is currently the one with the highest mortality, tumours within the gastrointestinal tract (colorectal, liver, and stomach) are the most prevalent ones when considering overall incidence and mortality. Among them, colorectal cancer (CRC) is actually the second global cause (1) of cancer-related mortality. Most of the CRC cases are adenocarcinoma, and 60%–65% of them are sporadic, 25% have a CRC family case without any hereditary syndrome, and 5% of them are caused by a cancer hereditary syndrome such as non-polyposis colorectal cancer (or Lynch syndrome) and familial adenomatous polyposis (FAP). Lynch syndrome is caused by mutations in genes encoding mismatch DNA repairing proteins, while FAP is due to mutations in the tumour suppressor gene APC. Moreover, colorectal cancer can be subdivided based on microsatellite stability (MSS) and microsatellite instability (MSI) due to this deficiency of mismatch repairing proteins. MSI tumours have better prognosis, as they can be easily treated with immunotherapy than MSS tumours (2–4). While there are more several factors such as aging or location (right side CRC has worse survival than the left side) (5) underlying its development, the most relevant risk contributors include modifiable elements such as obesity, sedentary lifestyle, low fruit and vegetable intake, red meat consumption, and smoking and alcoholic habits. Its frequency is higher in economically developed countries, although it is also increasing in westernised developing societies (6–8). Indeed, in 2022, colorectal cancer was the most diagnosed tumour and the second leading cause of cancer death in Europe (9). Its 5-year survival rate ranges from 10% to 90% depending on the stage at diagnose with a better prognosis if it is diagnosed at earlier stages. Screening methods include faecal occult blood test or double contrast barium enema, which are subsequently followed (if positive) by a colonoscopy (10).
Gastric cancer is the fifth most common tumour (1) in the world, and, as CRC, most of them are adenocarcinoma (11). It is usually classified as cardia gastric (upper stomach) and non-cardia gastric cancer (mid and distal stomach) having both different risk factors and epidemiological patterns (12). Of the gastric cancer cases, 90% are due to environmental factors while the remaining 10% have a familiar component. Hereditary diffuse gastric cancer, which has a worse survival than sporadic gastric tumours, is commonly caused by germinal mutations in CDH1 gene, which codes E-cadherin protein. Hereditary Peutz–Jeghers syndrome predisposes to early-onset gastric cancer. Lynch syndrome also increases the lifetime risk of developing it (13–17). Whereas non-cardia gastric cancer is related to smoking and deficient diet, cardia class correlates with obesity and gastroesophageal reflux disease (18). Despite overall predisposing factors including dietary factors (like high consumption of processed meats, smoked foods, and high salt diets) (19), Helicobacter pylori infection is responsible for almost 90% of distal gastric cancers. Indeed, the International Agency for Research on Cancer labels this bacterium as a human carcinogen due to its correlation with gastric cancer, as it provokes gastritis, which can derive on stomach atrophy, metaplasia, and dysplasia. Its 5-year survival rate for early stages is 75.4% (20), so screening analysis, such as esophagogastroduodenoscopy, are usually implemented.
Last, but not the least, liver cancer remains the sixth most common tumour (1) worldwide. As opposed to both colorectal and gastric cancer, this one is a remarkable heterogeneous disease that has been redefined over time. However, hepatocellular carcinoma is its most frequent histological manifestation appearing in approximately 90% of the cases (21). Risk factors include alcohol abuse, diabetes, exposure to aflatoxin, and genetic predisposition. Nevertheless, almost 80% (22) of hepatocellular carcinoma are associated with hepatitis B/C virus infection. In addition, high-risk groups include patients with non-alcoholic fatty liver and cirrhosis. The Barcelona Clinic for Liver Cancer (BCLC) classifies hepatocellular carcinoma status and determine treatments options based on it (from 0 to D). Another common indicator is the MELD model (Model for End-stage Liver Disease), which examines the bilirubin, creatinine, and International Normalised Ratio (INR) from each patient to predict the 3-month mortality with a statistical confidence of 80%, in order to prioritise those patients who need an earlier transplant (23). Although early stages of hepatocellular carcinoma are treatable, only 20% of diagnosed patients survive more than 1 year. Liquid biopsies such as cell-free DNA or α-fetoprotein are being studied as potential early detection biomarkers, but further research is required (22, 24, 25).
Given therefore the aggressive nature of digestive tumours, there is no doubt that early diagnosis is still the best weapon to fight them. In this regard, the identification of novel biomarkers can provide novel tools not only to aid on their diagnosis and predict their subsequent prognosis (26–30) but also unveil novel targets to perform immune therapy. However, although this has been partially achieved in the gastric one given the role that H. pylori infection elicits on its trigger, such goal remains elusive for both CRC and liver cancer.
Regarding hepatocellular carcinoma (HCC), alpha-fetoprotein (AFP) is widely used combined with abdominal ultrasound in order to determine HCC illness. However, it is also true that targeted therapies are essential for the treatment of hepatocellular carcinoma (HCC), yet tackling this type of cancer is challenging owing to its molecular heterogeneity. Some predictive biomarkers based on gene polymorphisms (like VEGFD), serum protein levels, or clinical predictors such as hypertension can be used to identify responders to sorafenib or levantinib, both of which serve as first-line systemic therapies (31). However, it is obvious that more studies are needed to obtain new biomarkers with utility in the clinical practice.
Related to CRC, clinicians focus on DNA signatures to design treatment options. As previously stated, high MSI is the phenotype of 15% colorectal tumours. These tumours typically do not metastasise and show poor response to chemotherapy. Despite this, they have a better clinical course than MSS tumours because of the higher production of neoantigens, making them more susceptible to treatment with immunotherapy (32). Other therapeutic strategies focus on the MAPK pathway status. RAS, which controls cells proliferation, is often mutated in CRC (33), and monoclonal antibodies (mAbs) anti-EFGR as cetuximab are typically used to stop the abnormal signalling. Other mAbs like encorafenib targets KRAF, which is usually mutated in CRC, and it is used when anti-EFGR are not effective, as it is downstream in the MAPK pathway (32). Focusing on liquid biopsies, several serum proteins such as carcinoembryonic antigen (CEA) are found in CRC. However, they do not correlate significantly with prognosis or survival rates. New approaches also investigate circulating tumour cells and circulating tumour DNA in the blood. In contrast with CEA and other serum proteins, these markers correlate with treatment response and survival rates, making them desirable biomarkers to study in depth before starting a therapeutic option (32, 34, 35).
Building from the current needs, this manuscript will therefore focus on the utility that flow cytometry elicits on the search of novel biomarkers with aid on the clinical practise of digestive tumours.
Conventional flow cytometry (CFC) is a fluorescence-based technique, which allows the immunotyping of immune cells at single-cell resolution, which has been used in the clinical setting for over 50 years (36). In addition, it is currently used for B- and T-cell leukaemia and lymphoma immunophenotyping, helping to identify their optimal treatment. However, given that CFC is based on the use of fluorochrome-labelled specific antibodies, the maximum number of fluorochromes—and therefore markers—which can be analysed by classical CFC, is restricted to the number of available channels in the equipment. Therefore, conventional cytometers in the clinical setting do not usually provide more than 8 or 10 channels, hence limiting the number of parameters that can be analysed. Although that has been recently overridden by the development of novel equipment, which display up to 20 channels and, therefore, can identify up to 20 markers at the same time (37), the development of CFC panels using more than 15 markers simultaneously requires advanced skills to compensate the fluorescence among the different markers. In addition, fluorochromes with a similar emission wavelength or close emission peaks cannot be combined in the same panel, hence limiting the capacity to perform complex panels.
In order to overcome these limitations, mass cytometry or CyTOF (cytometry time-of-flight) allows the analysis of over 40 markers at the single-cell level. That can be achieved because, as opposed to CFC, antibodies are tagged with heavy metals (like stable lanthanide isotopes) instead of fluorochromes, so cells are characterised based on atomic weight (38). Therefore, mass cytometry has proven to be highly valuable in the deep characterisation of immune cells (39) given its ability to identify over 40 parameters within a single cell (40, 41), rendering it a “pseudo-omic” technique (38), which has allowed the identification of novel biomarkers (42–46).
The capacity, however, of developing complex panels with over 40 parameters in a single-cell comes with a cost. Although flow cytometry data analyses are traditionally performed following a hierarchical gating strategy of serial selections in two-dimensional plots, this approach is time consuming, subjective, and can be also influenced by the operator’s experience and biases. In addition, the amount of obtained data with complex panels is exponentially increased. Therefore, when addressing complex panels, it becomes obvious that novel unbiased approaches are required in order to address the whole variability (47, 48). Thus, computational approaches based on unsupervised algorithms have emerged to address such complexity (49).
To that end, preliminary data cleaning (either with an unsupervised cleaning algorithm or with manual removal) is essential to remove outlier events due to abnormal flow behaviours resulting from clogs and other common technical problems. Once that has been performed, a subsequent unbiased exploratory approach can be performed on the dataset in an efficient and reproducible way. Dimensionality reduction algorithms, which are commonly used to perform an unsupervised and exploratory cytometry analysis, organise the data based on the protein expression levels, projecting all the variables into two or three dimensions. T-distributed Stochastic Neighbour Embedding (t-SNE) focuses on the non-linear differences between cells in protein marker expression to create a two-dimension map retaining the local data structure. However, the global distances are not reliable, and the number of cells that it can support is limited (50). Hence, it is common to perform a randomised method of event downsizing to a given dataset called “Downsampling” or “Subsampling”. Although this entails the possibility of losing under-represented populations along the way, it is possible to perform a subsequent unsupervised analysis by directly addressing the previously identified populations of interest, allowing the use of a larger number of events from these populations, which would confer greater robustness to the results. In addition, it is possible to implement the hierarchical analysis as a way of validating the results obtained, thus avoiding the loss of information that would involve doing the process in reverse (50, 51). Hierarchical Stochastic Neighbour Embedding (H-SNE) overcomes t-SNE because it allows to achieve dimension reduction algorithm without subsampling, becoming a solid and powerful single-cell analysis method (52). Given that t-SNE quadratically scales its computation time in function of the number of cells being analysed, another algorithm option is the Uniform Manifold Approximation and Projection (UMAP), which has a faster computation time than t-SNE, preserves better the global data structure, and attaches additional data to an existing plot, reasons why it has a powerful clinical monitoring impact (for instance, comparing a treatment efficacy) (50). However, such approaches have certain issues, such as the absence of a standardised and agreed-upon pipeline protocol (due to his novelty) or the inability to eliminate subjectivity in the analysis steps and the technical limitations. Although computational cytometry approaches become more widespread, they are a highly computationally demanding method (50, 51).
In spite of that, results obtained by computational cytometry approaches are data-driven and operator-independent, hence increasing their reproducibility, comparability, and accuracy, which, altogether, allow the identification of novel cell subsets related to a specific condition that otherwise may have gone unnoticed (50, 51) so they can be further analysed (and isolated) following directed hierarchical gating approaches.
Mass cytometry and computational cytometry have been used in several studies involving mouse models to better understand patient’s cancer and improve their therapeutic options. For instance, although PD-1/PD-L1 is a promising immunotherapy based on the inhibition of these immune checkpoints, not all patients derive benefit from this treatment; hence, it may need to be enhanced by other immune checkpoint blockade (ICB). Beyrend et al. (53) generated a CRC mouse model treated with PBS or PD-L1 therapy, and their tumour-infiltrating lymphocytes (TILs) were analysed by computational and mass cytometry. They found that the PD-L1 group showed a distinct T-cell subset expressing LAG-3 (inhibitory molecule) and ICOS (activating molecule). These findings suggest that enhancing antitumour immunity could be achieved by targeting LAG-3 or ICOS. Moreover, Krieg et al. (54) found that the complement component 3a receptor (C3aR) was downregulated in patients with CRC independently of their MSS or MSI status. Despite the role of the C3a–C3aR interaction in maintaining homeostasis and creating a tumour-free microenvironment, their CRC model lacking C3aR demonstrated that their protumourogenic faecal microbiota induced a significant immune infiltrate, which could be targeted with ICB. In addition, macrophages in CRC, known as tumour-associated macrophages (TAMs), can develop a proinflammatory (M1) or anti-inflammatory (M2) phenotype, leading this last one to T-cell exhaustion and poor prognosis (55). MS4A4A is a transmembrane protein related to these events, and it serves as a biomarker for the M2 phenotype (56); however, the role in CRC of TAMs and MS4A4A remains unclear. Using murine models, Li et al. (57) showed that MS4A4A induces polarisation towards the M2 phenotype, leading to T-cell exhaustion and a poor prognosis. Blocking MS4A4A with anti-MS4A4A detained CRC progression and enhanced the efficacy of anti-PD-1 therapy.
On this wise, Tang et al. (58) found that colon cancer mice injected with IFN-γ and anti-PD-1 had reduced tumour growth and less amount of M2 macrophages; however, they also observed an upregulation of LAG-3 in response to anti-PD-1 therapy.
Oncolytic virus therapy is a kind of nanomaterial used to treat cancer. Zhang et al. (59) designed a protein nanocage with the structure of hepatitis B virus and CpG motifs, which are immunostimulatory. Using a CRC murine model, they found by mass cytometry that after being exposed to the nanocages, the tumour microenvironment was modified having a higher expression of CD8 (cytotoxic) T cells and decreasing the T-cell exhaustion. The treatment led to enhanced antitumour immunity.
Specifically focused in the human setting, the application of novel and complex multiparametric panels in the CRC setting by CyTOF has proved that there is a huge immune diversity between different patients and within each individual, not only between the blood and the intestinal immune infiltrate but also between the affected and non-affected tissue (60). In addition, certain circulating immune cells such as monocytes and NK cells subsets are altered during perioperative period (61), confirming that CRC requires a personalised approach.
Mass cytometry has, for instance, proved that the pregnane X receptor mediates the mechanisms of oxaliplatin therapy resistance in tumour cells by eliminating the drug from the cells (62). In addition, and following the first cycle of oxaliplatin chemotherapy, patients reduce their total NK cell numbers due to a specific decrease in the CD56dimCD16− subset. In a similar manner, the CD16+ NK fraction was also reduced due the presence of several proinflammatory cytokines (IL-2, IL-15, and IL-18), which ultimately leads to lose their cytotoxic activity (63). In addition, Ting Zhang et al. (64) have shown that although EpCAM+CD4+ T-cell subsets can infiltrate the tumour in a CCR5- and CCR6-dependent manner, they nevertheless displayed an exhausted and immunosuppressive phenotype, as they were both not only PD-1+ but also PD-L1+. Not surprisingly, and given its immune-suppressive effect, such cell subset has become the target for immune checkpoint blockade therapies. In addition, Yang Luo et al. (65) found that high levels of circulating T-cell co-expressing CD103+CD39+ predict clinical response to such immune checkpoint inhibitors.
In line with the above, Tsunenori et al. (66) proved that IL-6 produced by stromal and tumour cells correlated with lower Treg (CD4+FOXP3+) cells in the tumour microenvironment. In addition, IL-6 serum levels positively correlated with enhanced levels of myeloid-derived suppressor cells (MDSCs) and effector regulatory T-cells (eTreg), two types of immunosuppressive cell subsets, while high levels of IL-6 in the tumour microenvironment correlates with immune evasion and a debilitated antitumour activity. In a similar manner, eTreg (defined as BLIMP-1+FOXP3+) were increased in the tumour infiltrate. These cells can be further divided into FOXP3lo and FOXP3hi. In addition, higher levels of the first subset in the tumour infiltrate correlate with good outcomes while the later correlate with bad prognosis. Therefore, and given that eTreg cells have been associated with both good and bad prognosis in CRC, it seems obvious that further investigation is required in order to specifically identify the specific subset, which can be used as a therapeutic target (67).
Cytokeratin 20 (CK20) is a common biomarker used to determine CRC tumour stage and histological grade. In addition, its expression on tumour cells is related to poor prognosis, whereas its absence indicates a less-invasive cancer (68). Despite that, terminally differentiated epithelial cells have a lower proportion of CK20 expression in CRC tissues referred to controls, while its expression also differs, within CRC patients, based on the tumour microsatellite stability. This reveals the necessity of fully understanding the colorectal cancer status of the patients in each of its different stages (69).
On the other hand, it is obvious that the gut microbiota can also modulate the outcome of immune responses in CRC (70). Indeed, mucosa-associated invariant T cells (MAIT) cells recognise bacterial riboflavin antigens following presentation by the non-classic histocompatibility complex MR1. Building from that, Shamin Li et al. (71) found that tumour-infiltrating MAIT cells are CD39+ and display an increased expression of exhaustion markers PD-1+ and CTL4+ coupled with lower cytokine production. Together, these suggest that chronic activation of MAIT cells induces the expression of CD39 together with senescence markers leading to T-cell exhaustion. In addition, Fusobacterium nucleatum induces CD39 expression on MAIT in a TCR-dependent manner, hence providing a mechanism by which such bacteria are bad prognosis factors in CRC.
One of the most advantageous locations for identifying biomarkers are liquid biopsies like peripheral blood where we have PBMCs. Kong et al. (72) studied the differences between immune subsets during CRC development. Through mass cytometry and computational analysis, they revealed that central memory CD4+ T cells, switched B cells, CD16− NK cells, monocytes, and basophils were increased in adenocarcinoma compared with adenoma and healthy controls, while double-negative T cells were decreased in adenoma and adenocarcinoma. Moreover, effector CD4+ T cells and naive B cells were higher in CRC patients with lymph node metastasis, while basophils and unswitched B cells were lower.
Mass cytometry can also monitor the effects of a clinical trial in the patient’s immune system. Monjazeb et al. (73) performed mass cytometry to evaluate the immune impact on the patients of a phase 2 trial of combined anti-CTLA-4 and anti-PD-1 therapy. They found that patient’s PBMCs had a lower amount of CD4+ and CD8+ T cells compared with patients treated with two different radiation regimens (with generally no differences between them). This included activation markers such as CXCR3 or ICOS.
Yang et al. (74) decided to use mass cytometry among other exploratory methods to study primary liver cancers and liver metastases from other cancers including CRC. They found that hepatic metastatic CRC had higher levels of CD4+ Treg, LAG-3+CD4+ T-cells, CD27+PD-1+CD8+ T-cells, and lower levels of CD57+PD-1+CD8+ T cells than non-hepatic metastatic CRC. Circulating levels of this immune subsets have therefore the potential to be considered as novel biomarkers to predict patients with a higher probability of subsequently developing metastases.
Mass cytometry has also been implemented in the context of liver cancer and its main variant, hepatocellular carcinoma (HCC). The full comprehension of HCC illness becomes complicated due to its heterogeneity. In addition, although there is a wide range of different treatments based on tumour stage, they however do not always translate into clinical remission (75); it appears mandatory to explore new targets and therapeutic options (21). Moreover, mass cytometry approaches can be also applied as diagnostic tools. Hence, a complex panel applied on blood samples can identify several immune disturbances, which can be used to allow an early detection of different solid tumours including HCC (56).
Animal models of HCC are often used to study this condition through mass cytometry. In the search of new proposing target markers for therapies, research in mice revealed that GDF15 protein induces enhancement of Treg through CD48 (76), and this leads to immunosuppression in HCC; blockade of this protein could potentially be translated to human therapies. When studying effectiveness of treatments, it was found that SUMOylation levels are higher in HCC liver than in normal liver tissues. Subsequently, it was proved that inhibition of this process enhances antitumour activity and could be a therapeutic option (77). In another study using mice, it was found that “M2-like” tumour-associated macrophages are more prevalent in a specific type of mouse model resistant to anti-anti-PD-1 therapy (78). However, the conclusion highlights the wide diversity in the immune tumour microenvironments among preclinical models, in addition to the intrinsic heterogeneity of HCC in humans. It seems imperative to expand studies in mouse models to obtain translational results for humans. Therefore, in this review, we will focus on human studies.
Focused on the human setting, potential new therapeutic strategies could benefit from mass cytometry studies regarding HCC cells mechanisms. For example, it has been revealed that tumour cells and their intrinsic activation of β-catenin pathway results in the recruitment of MDSC and the formation of immune “desert” phenotype, which could be a potential treatment strategy option (79). However, immune system description regarding HCC involves not only novel immunotherapy targets but also prognosis and evolution biomarkers and cell subsets. Undeniably, the role of NK cells remain fundamental for immunity and especially in cancer (80). Indeed, CD56+ and CD56dim NK cells may be useful as immunotherapies targets for HCC patients, as they have impairment phenotypes in hepatocarcinoma affected from different aetiologies, especially in non-alcoholic fatty liver disease (NAFLD) patients (21, 81).
In HCC, it is also possible to explore the patient’s immunity depending on the stage or type of development that they have achieved. Although patients with HCC who successfully had a transplant are scarce, they display a unique immune fingerprint, since mass cytometry approaches have shown that such differential immune profile can be used to stratify and predict patients with risk of recurrence, which can be very helpful when designing patient treatment (82).
Also building from mass cytometry results, it has been proven that the CD161+/CD161− ratio within peripheral CD8+PD-1+ T cells predicts subsequent disease outcome with better prognosis with higher ratios (83). Specifically focused on the tissue (affected, non-affected, and border area), although PD-1 expression does not predict the outcome of the patients, it is actually the balance between tissue resident memory and PD-1+ exhausted T cells that is relevant for such outcome, since a high tissue resident memory/exhausted T-cell ratio in tumour microenvironment determines positive patient prognosis (84, 85). Better prognosis conditions are expected from HCC patients who have a non-terminally exhausted phenotype in tumour-resident memory T cells, which are specific to HBV response (86), and also for those patients with enriched CD4+CD8+ T cells, especially if they are expressing PD-1 and located in leading-edge regions (87).
When focusing on ongoing therapies, radiofrequency ablation is the primary first-line treatment option for HCC patients who are not eligible for surgery. Aiming to study its effects on tumour immune response, a mass cytometry study revealed that decreased levels of CD8+ effector and memory T cells, among others, correlated with a worst immune response against tumour cells (88). As for current therapy options, immune checkpoint inhibitors (including PD-1/PD-L1) have reached long-term response rates of 14%–20% in HCC patients. However, there is no information about patients who would benefit from this treatment due to its aetiology. In addition, CyTOF analyses revealed that neither viral aetiology nor the current viral status in HCC patients were variables to consider when deciding to prescribe PD-1 inhibitor therapy (89). On the other hand, the use of threonine tyrosine kinase inhibitor changes the proportion of circulating immune subsets before following treatment, with bigger changes on patients with a better outcome (90). Another scenario in which immune activation occurs, leading to a favourable response in HCC patients, is Yttrium90-Radioembolisation treatment (91). There are other types of uncommon treatments under study, such as the use of compound 2,5-dimethylcelecoxib (92), which has proven an increased infiltrate of NK cells within HCC tumours, resulting in favourable prognosis and suggesting its potential as a therapy target.
In summary, HCC pathology, heterogeneity, and treatment response have been broadly studied by CyTOF. Some immune cell subsets like CD56 NK cells or the CD161+/CD161− ratio within CD8+PD-1+ T cells may indeed be useful to stratify patients based on their aetiology and their subsequent outcome, while other subsets can predict clinical response to immune checkpoint inhibitors.
Mass cytometry has undoubtedly shown a great potential in the study of human gastrointestinal tumours. Nevertheless, it is also true that although this approach overcomes most of the CFC approaches, it also has some specific handicaps (41, 93).
The main one is the low acquisition rate, as it only allows to acquire approximately 500 cells per second instead of more than 10,000 in CFC. In addition, it is an expensive technique, since the costs of not only the equipment but also the special reagents and labelling compounds are significantly higher than those in flow cytometry. In a similar manner, cell vaporisation is an irreversible process and restricts the potential for subsequent ad hoc sorting. Likewise, due to lower cell recovery, there is a decrease in sensitivity for detecting low abundance proteins. Last, but not the least, as with a classical sorter, the equipment requires operation by a highly trained person, hence limiting its widespread accessibility.
An alternative to overcome these limitations is spectral cytometry, which combines the principal features of CyTOF while abrogating its handicaps. Therefore, as opposed to the CFC, spectral cytometry provides a measure of the entire fluorescence emission spectrum. That way, classical issues associated with CFC compensation and autofluorescence issues are reduced through a concrete unmixing algorithm (94). In addition, as it uses fluorochromes, reagents are usually cheaper, since it is not the same ones used in CFC. Moreover, its acquisition speed is high (up to 30,000 events per second) (95). Overall, these are even more attractive features, especially considering that comparable results can be expected from both procedures (96).
However, both spectral and mass cytometry data must offer high-quality, reliable, and robust information to be considered valid. Hence, a careful panel design and validation, titration, and optimisation of antibodies and reference controls are required. Having said that, and given the properties of mass cytometry, it is also true that the implementation of novel panels is usually faster in that approach, since no unmixing controls are required to optimise a panel. Either way, sample staining on both approaches should always include normalisation control and correct equipment handling (97). On the contrary, both mass and spectral cytometry are capable of performing complex panels of up to 40 parameters (47). As a consequence, all the known immune cell subsets can be identified in a given sample by using these techniques. Hence, the ability to obtain such a wide range of information from each analysis provides a major reason to implement these approaches, spectral or mass cytometry, in various types of studies, especially in those aimed at discovering new biomarkers needed for some illnesses. In this regard, Table 1 summarises the different aspects to take into consideration before considering mass or spectral cytometry approaches. Therefore, it seems obvious that spectral cytometry overtakes the approaches performed by mass cytometry, as it is faster, cheaper, and requires a smaller sample size. Indeed, there are several issues that need to be addressed in the near future for both CRC and HCC, and they may be overcome when using different types of approaches, for example spectral cytometry studies, which, to the best of our knowledge, have not been performed in this context.
In addition, these techniques provide a high-resolution single-cell analysis, so the more parameters can be detected on cancer cells, the more specific will be the exploration of the tumour heterogeneity. The classification of the function and phenotype of the immune cells leads to an accurate examination of the altered cellular process, early diagnosis and detection, and prediction of therapy response. The integration of cytometry, proteomics, and genomics technologies will bring a promising precision medicine in order to improve patients’ outcomes and life expectancy (98).
The only current approach for CRC diagnosis and monitoring is the use of regular colonoscopies, which, however, are not only invasive and uncomfortable for the patients but also expensive and time consuming for the health systems. On the other hand, regarding HCC, most of its current knowledge has been obtained from murine models, which, although essential to deepen our understanding on such disease, they may not always translate into the human setting (99, 100). Therefore, given the large amount of information that computational cytometry approaches provide, these approaches will allow the identification of novel (and better) biomarkers to aid on CRC or HCC diagnosis, monitoring, or even predicting disease outcome. In addition, given that both mass and spectral cytometry usually focus on the study of the immune system, they may not only identify biomarkers but also provide novel insights into the specific pathogenic mechanisms potentially unravelling novel targets to perform immunotherapy or identify novel mechanisms, hence rendering to the development of novel treatments.
CG: Methodology, Writing – original draft. AG: Methodology, Writing – original draft. JH: Methodology, Writing – original draft. SC: Supervision, Writing – review & editing. DB: Supervision, Writing – review & editing.
The author(s) declare financial support was received for the research, authorship, and/or publication of this article. CC and AH are currently funded by the Spanish Association Against Cancer (Asociación Española Contra el Cáncer—AECC). The lab is currently funded by the Spanish Ministry of Science (PID2019–104218RB-I00), Programa Estratégico Instituto de Biología y Genética Molecular (IBGM Junta de Castilla y León. Ref. CVC8485), and the European Commission—NextGenerationEU (Regulation EU 2020/2094), through CSIC’s Global Health Platform (PTI Salud Global).
The authors declare that the research was conducted in the absence of any commercial or financial relationships that could be construed as a potential conflict of interest.
All claims expressed in this article are solely those of the authors and do not necessarily represent those of their affiliated organizations, or those of the publisher, the editors and the reviewers. Any product that may be evaluated in this article, or claim that may be made by its manufacturer, is not guaranteed or endorsed by the publisher.
1. IARC. Home. Available online at: https://www.iarc.who.int/ (Accessed September 13, 2022).
2. Keum N, Giovannucci E. Global burden of colorectal cancer: emerging trends, risk factors and prevention strategies. Nat Rev Gastroenterol Hepatol. (2019) 16:713–32. doi: 10.1038/s41575–019-0189–8
3. Jasperson KW, Tuohy TM, Neklason DW, Burt RW. Hereditary and familial colon cancer. Gastroenterology. (2010) 138:2044–58. doi: 10.1053/j.gastro.2010.01.054
4. Li K, Luo H, Huang L, Luo H, Zhu X. Microsatellite instability: a review of what the oncologist should know. Cancer Cell Int. (2020) 20:16. doi: 10.1186/s12935–019-1091–8
5. Bufill JA. Colorectal cancer: evidence for distinct genetic categories based on proximal or distal tumor location. Ann Intern Med. (1990) 113:779–88. doi: 10.7326/0003–4819-113–10-779
6. Center MM, Jemal A, Smith RA, Ward E. Worldwide variations in colorectal cancer. CA Cancer J Clin. (2009) 59:366–78. doi: 10.3322/caac.20038
7. Jemal A, Bray F, Center MM, Ferlay J, Ward E, Forman D. Global cancer statistics. CA Cancer J Clin. (2011) 61:69–90. doi: 10.3322/caac.20107
8. Arnold M, Sierra MS, Laversanne M, Soerjomataram I, Jemal A, Bray F. Global patterns and trends in colorectal cancer incidence and mortality. Gut. (2017) 66:683–91. doi: 10.1136/gutjnl-2015–310912
9. Observatorio AECC. Available online at: https://observatorio.contraelcancer.es/.
10. Schreuders EH, Ruco A, Rabeneck L, Schoen RE, Sung JJY, Young GP, et al. Colorectal cancer screening: a global overview of existing programmes. Gut. (2015) 64:1637–49. doi: 10.1136/gutjnl-2014–309086
11. Vishwanath A, Krishna S, Manudhane AP, Hart PA, Krishna SG. Early-onset gastrointestinal Malignancies: an investigation into a rising concern. Cancers (Basel). (2024) 16:1553. doi: 10.3390/cancers16081553
12. Japanese Gastric Cancer Association. Japanese classification of gastric carcinoma: 3rd English edition. Gastric Cancer. (2011) 14:101–12. doi: 10.1007/s10120-011-0041-5
13. Dong E, Duan L, Wu BU. Racial and ethnic minorities at increased risk for gastric cancer in a regional US population study. Clin Gastroenterol Hepatol. (2017) 15:511–7. doi: 10.1016/j.cgh.2016.11.033
14. Setia N, Wang CX, Lager A, Maron S, Shroff S, Arndt N, et al. Morphologic and molecular analysis of early-onset gastric cancer. Cancer. (2021) 127:103–14. doi: 10.1002/cncr.33213
15. Figueiredo J, Melo S, Carneiro P, Moreira AM, Fernandes MS, Ribeiro AS, et al. Clinical spectrum and pleiotropic nature of CDH1 germline mutations. J Med Genet. (2019) 56:199–208. doi: 10.1136/jmedgenet-2018–105807
16. Carneiro F, Oliveira C, Suriano G, Seruca R. Molecular pathology of familial gastric cancer, with an emphasis on hereditary diffuse gastric cancer. J Clin Pathol. (2008) 61:25–30. doi: 10.1136/jcp.2006.043679
17. Takahashi M, Sakayori M, Takahashi S, Kato T, Kaji M, Kawahara M, et al. A novel germline mutation of the LKB1 gene in a patient with Peutz-Jeghers syndrome with early-onset gastric cancer. J Gastroenterol. (2004) 39:1210–4. doi: 10.1007/s00535-004-1474-y
18. Thrift AP, Wenker TN, El-Serag HB. Global burden of gastric cancer: epidemiological trends, risk factors, screening and prevention. Nat Rev Clin Oncol. (2023) 20:338–49. doi: 10.1038/s41571–023-00747–0
19. Ilic M, Ilic I. Epidemiology of stomach cancer. World J Gastroenterol. (2022) 28:1187–203. doi: 10.3748/wjg.v28.i12.1187
20. National Cancer Institute. Cancer Stat Facts: Stomach Cancer. Available online at: https://seer.cancer.gov/statfacts/html/stomach.html (Accessed May 2, 2024).
21. Yoshida Y, Yoshio S, Yamazoe T, Mori T, Tsustui Y, Kawai H, et al. Phenotypic characterization by single-cell mass cytometry of human intrahepatic and peripheral NK cells in patients with hepatocellular carcinoma. Cells. (2021) 10:1495. doi: 10.3390/cells10061495
22. Li L, Wang H. Heterogeneity of liver cancer and personalized therapy. Cancer Lett. (2016) 379:191–7. doi: 10.1016/j.canlet.2015.07.018
23. Luo X, Leanza J, Massie AB, Garonzik-Wang JM, Haugen CE, Gentry SE, et al. MELD as a metric for survival benefit of liver transplantation. Am J Transplant. (2018) 18:1231–7. doi: 10.1111/ajt.14660
24. Kulik L, El-Serag HB. Epidemiology and management of hepatocellular carcinoma. Gastroenterology. (2019) 156:477–491.e1. doi: 10.1053/j.gastro.2018.08.065
25. Johnson P, Zhou Q, Dao DY, Lo YMD. Circulating biomarkers in the diagnosis and management of hepatocellular carcinoma. Nat Rev Gastroenterol Hepatol. (2022) 19:670–81. doi: 10.1038/s41575-022-00620-y
26. Ahlquist DA. Universal cancer screening: revolutionary, rational, and realizable. NPJ Precis Oncol. (2018) 2:23. doi: 10.1038/s41698-018-0066-x
27. Cohen JD, Li L, Wang Y, Thoburn C, Afsari B, Danilova L, et al. Detection and localization of surgically resectable cancers with a multi-analyte blood test. Sci (1979). (2018) 359:926–30. doi: 10.1126/science.aar3247
28. Martínez-Bosch N, Cristóbal H, Iglesias M, Gironella M, Barranco L, Visa L, et al. Soluble AXL is a novel blood marker for early detection of pancreatic ductal adenocarcinoma and differential diagnosis from chronic pancreatitis. EBioMedicine. (2022) 75:103797. doi: 10.1016/j.ebiom.2021.103797
29. Hüttenhain R, Soste M, Selevsek N, Röst H, Sethi A, Carapito C, et al. Reproducible quantification of cancer-associated proteins in body fluids using targeted proteomics. Sci Transl Med. (2012) 4:142ra94-142ra94. doi: 10.1126/scitranslmed.3003989
30. Beretov J, Wasinger VC, Millar EKA, Schwartz P, Graham PH, Li Y. Proteomic analysis of urine to identify breast cancer biomarker candidates using a label-free LC-MS/MS approach. PloS One. (2015) 10:e0141876. doi: 10.1371/journal.pone.0141876
31. Wang Y, Deng B. Hepatocellular carcinoma: molecular mechanism, targeted therapy, and biomarkers. Cancer Metastasis Rev. (2023) 42:629–52. doi: 10.1007/s10555–023-10084–4
32. Crutcher M, Waldman S. Biomarkers in the development of individualized treatment regimens for colorectal cancer. Front Med (Lausanne). (2022) 9:1062423. doi: 10.3389/fmed.2022.1062423
33. Wan M-L, Wang Y, Zeng Z, Deng B, Zhu B-S, Cao T, et al. Colorectal cancer (CRC) as a multifactorial disease and its causal correlations with multiple signaling pathways. Biosci Rep. (2020) 40(3):BSR20200265. doi: 10.1042/BSR20200265
34. Kidess E, Heirich K, Wiggin M, Vysotskaia V, Visser BC, Marziali A, et al. Mutation profiling of tumor DNA from plasma and tumor tissue of colorectal cancer patients with a novel, high-sensitivity multiplexed mutation detection platform. Oncotarget. (2015) 6:2549–61. doi: 10.18632/oncotarget.3041
35. Krebs MG, Metcalf RL, Carter L, Brady G, Blackhall FH, Dive C. Molecular analysis of circulating tumour cells-biology and biomarkers. Nat Rev Clin Oncol. (2014) 11:129–44. doi: 10.1038/nrclinonc.2013.253
36. Bendall SC, Nolan GP, Roederer M, Chattopadhyay PK. A deep profiler’s guide to cytometry. Trends Immunol. (2012) 33:323–32. doi: 10.1016/j.it.2012.02.010
37. Monaco G, Chen H, Poidinger M, Chen J, de Magalhães JP, Larbi A. flowAI: automatic and interactive anomaly discerning tools for flow cytometry data. Bioinformatics. (2016) 32:2473–80. doi: 10.1093/bioinformatics/btw191
38. Bjornson ZB, Nolan GP, Fantl WJ. Single-cell mass cytometry for analysis of immune system functional states. Curr Opin Immunol. (2013) 25:484–94. doi: 10.1016/j.coi.2013.07.004
39. Bandura DR, Baranov VI, Ornatsky OI, Antonov A, Kinach R, Lou X, et al. Mass cytometry: technique for real time single cell multitarget immunoassay based on inductively coupled plasma time-of-flight mass spectrometry. Anal Chem. (2009) 81:6813–22. doi: 10.1021/ac901049w
40. Spitzer MH, Nolan GP. Mass cytometry: single cells, many features. Cell. (2016) 165:780–91. doi: 10.1016/j.cell.2016.04.019
41. Millian DE, Saldarriaga OA, Wanninger T, Burks JK, Rafati YN, Gosnell J, et al. Cutting-edge platforms for analysis of immune cells in the hepatic microenvironment—Focus on tumor-associated macrophages in hepatocellular carcinoma. Cancers (Basel). (2022) 14:1861. doi: 10.3390/cancers14081861
42. DiGiuseppe JA, Wood BL. Applications of flow cytometric immunophenotyping in the diagnosis and posttreatment monitoring of B and T lymphoblastic leukemia/lymphoma. Cytometry B Clin Cytom. (2019) 96:256–65. doi: 10.1002/cyto.b.21833
43. Krieg C, Nowicka M, Guglietta S, Schindler S, Hartmann FJ, Weber LM, et al. High-dimensional single-cell analysis predicts response to anti-PD-1 immunotherapy. Nat Med. (2018) 24:144–53. doi: 10.1038/nm.4466
44. Gadalla R, Noamani B, MacLeod BL, Dickson RJ, Guo M, Xu W, et al. Validation of cyTOF against flow cytometry for immunological studies and monitoring of human cancer clinical trials. Front Oncol. (2019) 9:415. doi: 10.3389/fonc.2019.00415
45. Rochigneux P, Lisberg A, Garcia A, Granjeaud S, Madroszyk A, Fattori S, et al. Mass cytometry reveals classical monocytes, NK cells, and ICOS+ CD4+ T cells associated with pembrolizumab efficacy in patients with lung cancer. Clin Cancer Res. (2022) 28:5136–48. doi: 10.1158/1078–0432.CCR-22–1386
46. Anandan S, Thomsen LCV, Gullaksen S-E, Abdelaal T, Kleinmanns K, Skavland J, et al. Phenotypic characterization by mass cytometry of the microenvironment in ovarian cancer and impact of tumor dissociation methods. Cancers (Basel). (2021) 13:755. doi: 10.3390/cancers13040755
47. Park LM, Lannigan J, Jaimes MC. OMIP-069: forty-color full spectrum flow cytometry panel for deep immunophenotyping of major cell subsets in human peripheral blood. Cytometry A. (2020) 97:1044–51. doi: 10.1002/cyto.a.24213
48. Conrad VK, Dubay CJ, Malek M, Brinkman RR, Koguchi Y, Redmond WL. Implementation and validation of an automated flow cytometry analysis pipeline for human immune profiling. Cytometry Part A. (2019) 95:183–91. doi: 10.1002/cyto.a.23664
49. Saeys Y, Van Gassen S, Lambrecht BN. Computational flow cytometry: Helping to make sense of high-dimensional immunology data. Nat Rev Immunol. (2016) 16:449–62. doi: 10.1038/nri.2016.56
50. Keyes TJ, Domizi P, Lo Y, Nolan GP, Davis KL. A cancer biologist’s primer on machine learning applications in high-dimensional cytometry. Cytometry Part A. (2020) 97:782–99. doi: 10.1002/cyto.a.24158
51. Diggins KE, Ferrell PB, Irish JM. Methods for discovery and characterization of cell subsets in high dimensional mass cytometry data. Methods. (2015) 82:55–63. doi: 10.1016/j.ymeth.2015.05.008
52. van Unen V, Höllt T, Pezzotti N, Li N, Reinders MJT, Eisemann E, et al. Visual analysis of mass cytometry data by hierarchical stochastic neighbour embedding reveals rare cell types. Nat Commun. (2017) 8:1740. doi: 10.1038/s41467–017-01689–9
53. Beyrend G, van der Gracht E, Yilmaz A, van Duikeren S, Camps M, Höllt T, et al. PD-L1 blockade engages tumor-infiltrating lymphocytes to co-express targetable activating and inhibitory receptors. J Immunother Cancer. (2019) 7:217. doi: 10.1186/s40425–019-0700–3
54. Krieg C, Weber LM, Fosso B, Marzano M, Hardiman G, Olcina MM, et al. Complement downregulation promotes an inflammatory signature that renders colorectal cancer susceptible to immunotherapy. J Immunother Cancer. (2022) 10:e004717. doi: 10.1136/jitc-2022–004717
55. Gentles AJ, Newman AM, Liu CL, Bratman SV, Feng W, Kim D, et al. The prognostic landscape of genes and infiltrating immune cells across human cancers. Nat Med. (2015) 21:938–45. doi: 10.1038/nm.3909
56. Mattiola I, Tomay F, De Pizzol M, Silva-Gomes R, Savino B, Gulic T, et al. The macrophage tetraspan MS4A4A enhances dectin-1-dependent NK cell-mediated resistance to metastasis. Nat Immunol. (2019) 20:1012–22. doi: 10.1038/s41590-019-0417-y
57. Li Y, Shen Z, Chai Z, Zhan Y, Zhang Y, Liu Z, et al. Targeting MS4A4A on tumour-associated macrophages restores CD8+ T-cell-mediated antitumour immunity. Gut. (2023) 72:2307–20. doi: 10.1136/gutjnl-2022–329147
58. Tang Y, Wei J, Ge X, Yu C, Lu W, Qian Y, et al. Intratumoral injection of interferon gamma promotes the efficacy of anti-PD1 treatment in colorectal cancer. Cancer Lett. (2024) 588:216798. doi: 10.1016/j.canlet.2024.216798
59. Zhang Q, Dong J, Wang J, Wang J, Wang C, Li Y, et al. Integration of protein nanocage with cpG motifs: A virus-mimicked core-shell nanostructure to ignite antitumor immunity. Small. (2023) 19:e2301281. doi: 10.1002/smll.202301281
60. Di J, Liu M, Fan Y, Gao P, Wang Z, Jiang B, et al. Phenotype molding of T cells in colorectal cancer by single-cell analysis. Int J Cancer. (2020) 146:2281–95. doi: 10.1002/ijc.32856
61. Zhou C, Wang Z, Jiang B, Di J, Su X. Monitoring pre- and post-operative immune alterations in patients with locoregional colorectal cancer who underwent laparoscopy by single-cell mass cytometry. Front Immunol. (2022) 13:807539. doi: 10.3389/fimmu.2022.807539
62. Dong Y, Wang Z, Xie G, Li C, Zuo W, Meng G, et al. Pregnane X receptor is associated with unfavorable survival and induces chemotherapeutic resistance by transcriptional activating multidrug resistance-related protein 3 in colorectal cancer. Mol Cancer. (2017) 16:71. doi: 10.1186/s12943–017-0641–8
63. Shinko D, McGuire HM, Diakos CI, Pavlakis N, Clarke SJ, Byrne SN, et al. Mass cytometry reveals a sustained reduction in CD16+ Natural killer cells following chemotherapy in colorectal cancer patients. Front Immunol. (2019) 10:2584. doi: 10.3389/fimmu.2019.02584
64. Zhang T, Lv J, Tan Z, Wang B, Warden AR, Li Y, et al. Immunocyte profiling using single-cell mass cytometry reveals epCAM+ CD4+ T cells abnormal in colon cancer. Front Immunol. (2019) 10:1571. doi: 10.3389/fimmu.2019.01571
65. Luo Y, Zong Y, Hua H, Gong M, Peng Q, Li C, et al. Immune-infiltrating signature-based classification reveals CD103+CD39+ T cells associate with colorectal cancer prognosis and response to immunotherapy. Front Immunol. (2022) 13:1011590. doi: 10.3389/fimmu.2022.1011590
66. Yamamoto T, Tsunedomi R, Nakajima M, Suzuki N, Yoshida S, Tomochika S, et al. IL-6 levels correlate with prognosis and immunosuppressive stromal cells in patients with colorectal cancer. Ann Surg Oncol. (2023) 30:5267–77. doi: 10.1245/s10434-023-13527-y
67. Norton SE, Ward-Hartstonge KA, McCall JL, Leman JKH, Taylor ES, Munro F, et al. High-dimensional mass cytometric analysis reveals an increase in effector regulatory T cells as a distinguishing feature of colorectal tumors. J Immunol. (2019) 202:1871–84. doi: 10.4049/jimmunol.1801368
68. Lassmann S, Bauer M, Soong R, Schreglmann J, Tabiti K, Nährig J, et al. Quantification of CK20 gene and protein expression in colorectal cancer by RT-PCR and immunohistochemistry reveals inter- and intratumour heterogeneity. J Pathol. (2002) 198:198–206. doi: 10.1002/path.1196
69. Simmons AJ, Scurrah CR, McKinley ET, Herring CA, Irish JM, Washington MK, et al. Impaired coordination between signaling pathways is revealed in human colorectal cancer using single-cell mass cytometry of archival tissue blocks. Sci Signal. (2016) 9:rs11-rs11. doi: 10.1126/scisignal.aah4413
70. Helmink BA, Khan MAW, Hermann A, Gopalakrishnan V, Wargo JA. The microbiome, cancer, and cancer therapy. Nat Med. (2019) 25:377–88. doi: 10.1038/s41591–019-0377–7
71. Li S, Simoni Y, Becht E, Loh CY, Li N, Lachance D, et al. Human tumor-infiltrating MAIT cells display hallmarks of bacterial antigen recognition in colorectal cancer. Cell Rep Med. (2020) 1:100039. doi: 10.1016/j.xcrm.2020.100039
72. Kong X-X, Xu J-S, Hu Y-T, Jiao Y-R, Chen S, Yu C-X, et al. Circulation immune cell landscape in canonical pathogenesis of colorectal adenocarcinoma by CyTOF analysis. iScience. (2024) 27:109229. doi: 10.1016/j.isci.2024.109229
73. Monjazeb AM, Giobbie-Hurder A, Lako A, Thrash EM, Brennick RC, Kao KZ, et al. A randomized trial of combined PD-L1 and CTLA-4 inhibition with targeted low-dose or hypofractionated radiation for patients with metastatic colorectal cancer. Clin Cancer Res. (2021) 27:2470–80. doi: 10.1158/1078–0432.CCR-20–4632
74. Yang S, Qian L, Li Z, Li Y, Bai J, Zheng B, et al. Integrated multi-omics landscape of liver metastases. Gastroenterology. (2023) 164:407–423.e17. doi: 10.1053/j.gastro.2022.11.029
75. Chen Z, Xie H, Hu M, Huang T, Hu Y, Sang N, et al. Recent progress in treatment of hepatocellular carcinoma. Am J Cancer Res. (2020) 10:2993–3036.
76. Wang Z, He L, Li W, Xu C, Zhang J, Wang D, et al. GDF15 induces immunosuppression via CD48 on regulatory T cells in hepatocellular carcinoma. J Immunother Cancer. (2021) 9:e002787. doi: 10.1136/jitc-2021–002787
77. Wang Z, Pan B, Su L, Yu H, Wu X, Yao Y, et al. SUMOylation inhibitors activate anti-tumor immunity by reshaping the immune microenvironment in a preclinical model of hepatocellular carcinoma. Cell Oncol (Dordr). (2024) 47:513–32. doi: 10.1007/s13402-023-00880-z
78. Zabransky DJ, Danilova L, Leatherman JM, Lopez-Vidal TY, Sanchez J, Charmsaz S, et al. Profiling of syngeneic mouse HCC tumor models as a framework to understand anti-PD-1 sensitive tumor microenvironments. Hepatology. (2023) 77:1566–79. doi: 10.1002/hep.32707
79. Wang K, Wu J, Yang Z, Zheng B, Shen S, Wang R-R, et al. Hyperactivation of β-catenin signal in hepatocellular carcinoma recruits myeloid-derived suppressor cells through PF4-CXCR3 axis. Cancer Lett. (2024) 586:216690. doi: 10.1016/j.canlet.2024.216690
80. Sajid M, Liu L, Sun C. The dynamic role of NK cells in liver cancers: role in HCC and HBV associated HCC and its therapeutic implications. Front Immunol. (2022) 13:887186. doi: 10.3389/fimmu.2022.887186
81. Sakamoto Y, Yoshio S, Doi H, Mori T, Matsuda M, Kawai H, et al. Increased frequency of dysfunctional siglec-7–CD57+PD-1+ Natural killer cells in patients with non-alcoholic fatty liver disease. Front Immunol. (2021) 12:603133. doi: 10.3389/fimmu.2021.603133
82. Wei X, Xie W, Yin W, Yang M, Khan AR, Su R, et al. Prediction of tumor recurrence by distinct immunoprofiles in liver transplant patients based on mass cytometry. Am J Cancer Res. (2022) 12:4160–76.
83. Li Z, Zheng B, Qiu X, Wu R, Wu T, Yang S, et al. The identification and functional analysis of CD8+PD-1+CD161+ T cells in hepatocellular carcinoma. NPJ Precis Oncol. (2020) 4:28. doi: 10.1038/s41698–020-00133–4
84. Barsch M, Salié H, Schlaak AE, Zhang Z, Hess M, Mayer LS, et al. T-cell exhaustion and residency dynamics inform clinical outcomes in hepatocellular carcinoma. J Hepatol. (2022) 77:397–409. doi: 10.1016/j.jhep.2022.02.032
85. Chew V, Lai L, Pan L, Lim CJ, Li J, Ong R, et al. Delineation of an immunosuppressive gradient in hepatocellular carcinoma using high-dimensional proteomic and transcriptomic analyses. Proc Natl Acad Sci USA. (2017) 114:E5900–9. doi: 10.1073/pnas.1706559114
86. Cheng Y, Gunasegaran B, Singh HD, Dutertre C-A, Loh CY, Lim JQ, et al. Non-terminally exhausted tumor-resident memory HBV-specific T cell responses correlate with relapse-free survival in hepatocellular carcinoma. Immunity. (2021) 54:1825–1840.e7. doi: 10.1016/j.immuni.2021.06.013
87. Zheng B, Wang D, Qiu X, Luo G, Wu T, Yang S, et al. Trajectory and functional analysis of PD-1high CD4+CD8+ T cells in hepatocellular carcinoma by single-cell cytometry and transcriptome sequencing. Adv Sci (Weinh). (2020) 7:2000224. doi: 10.1002/advs.202000224
88. Zhao Y, Yang T, Ouyang Y, Rao W, Liu K, Zheng J, et al. Radiofrequency ablation plays double role in immunosuppression and activation of PBMCs in recurrent hepatocellular carcinoma. Front Immunol. (2024) 15:1339213. doi: 10.3389/fimmu.2024.1339213
89. Ho WJ, Danilova L, Lim SJ, Verma R, Xavier S, Leatherman JM, et al. Viral status, immune microenvironment and immunological response to checkpoint inhibitors in hepatocellular carcinoma. J Immunother Cancer. (2020) 8:e000394. doi: 10.1136/jitc-2019–000394
90. Chan CY-K, Chiu DK-C, Yuen VW-H, Law C-T, Wong BP-Y, Thu KL, et al. CFI-402257, a TTK inhibitor, effectively suppresses hepatocellular carcinoma. Proc Natl Acad Sci. (2022) 119:1–11. doi: 10.1073/pnas.2119514119
91. Chew V, Lee YH, Pan L, Nasir NJM, Lim CJ, Chua C, et al. Immune activation underlies a sustained clinical response to Yttrium-90 radioembolisation in hepatocellular carcinoma. Gut. (2019) 68:335–46. doi: 10.1136/gutjnl-2017–315485
92. Pan B, Chen Z, Zhang X, Wang Z, Yao Y, Wu X, et al. 2,5-dimethylcelecoxib alleviated NK and T-cell exhaustion in hepatocellular carcinoma via the gastrointestinal microbiota-AMPK-mTOR axis. J Immunother Cancer. (2023) 11:e006817. doi: 10.1136/jitc-2023–006817
93. Newell EW, Cheng Y. Mass cytometry: blessed with the curse of dimensionality. Nat Immunol. (2016) 17:890–5. doi: 10.1038/ni.3485
94. Niewold P, Ashhurst TM, Smith AL, King NJC. Evaluating spectral cytometry for immune profiling in viral disease. Cytometry Part A. (2020) 97:1165–79. doi: 10.1002/cyto.a.24211
95. Mondelo-Macía P, León-Mateos L, Bernardo D, Díaz-Peña R. Top-of-the-art cytometry as a novel tool to aid in lung cancer immunotherapy. Transl Lung Cancer Res. (2023) 12:957–61. doi: 10.21037/tlcr-23–80
96. Ferrer-Font L, Mayer JU, Old S, Hermans IF, Irish J, Price KM. High-dimensional data analysis algorithms yield comparable results for mass cytometry and spectral flow cytometry data. Cytometry A. (2020) 97:824–31. doi: 10.1002/cyto.a.24016
97. Bonilla DL, Reinin G, Chua E. Full spectrum flow cytometry as a powerful technology for cancer immunotherapy research. Front Mol Biosci. (2021) 7:612801. doi: 10.3389/fmolb.2020.612801
98. Irish JM, Doxie DB. High-dimensional single-cell cancer biology. Curr Top Microbiol Immunol. (2014) 377:1–21. doi: 10.1007/82_2014_367
99. Bjornson-Hooper ZB, Fragiadakis GK, Spitzer MH, Chen H, Madhireddy D, Hu K, et al. A comprehensive atlas of immunological differences between humans, mice, and non-human primates. Front Immunol. (2022) 13:867015. doi: 10.3389/fimmu.2022.867015
Keywords: spectral cytometry, mass cytometry, colorectal cancer, hepatocellular carcinoma, computational cytometry, biomarkers, gastric cancer
Citation: G. de Castro C, G. del Hierro A, H-Vázquez J, Cuesta-Sancho S and Bernardo D (2024) State-of-the-art cytometry in the search of novel biomarkers in digestive cancers. Front. Oncol. 14:1407580. doi: 10.3389/fonc.2024.1407580
Received: 26 March 2024; Accepted: 10 May 2024;
Published: 29 May 2024.
Edited by:
Shensi Shen, Sichuan University, ChinaCopyright © 2024 G. de Castro, G. del Hierro, H-Vázquez, Cuesta-Sancho and Bernardo. This is an open-access article distributed under the terms of the Creative Commons Attribution License (CC BY). The use, distribution or reproduction in other forums is permitted, provided the original author(s) and the copyright owner(s) are credited and that the original publication in this journal is cited, in accordance with accepted academic practice. No use, distribution or reproduction is permitted which does not comply with these terms.
*Correspondence: Sara Cuesta-Sancho, c2FyYS5jdWVzdGEudXZhQGdtYWlsLmNvbQ==; David Bernardo, ZC5iZXJuYXJkby5vcmRpekBnbWFpbC5jb20=
†These authors have contributed equally to this work and share first authorship
‡These authors have contributed equally to this work and share senior authorship
Disclaimer: All claims expressed in this article are solely those of the authors and do not necessarily represent those of their affiliated organizations, or those of the publisher, the editors and the reviewers. Any product that may be evaluated in this article or claim that may be made by its manufacturer is not guaranteed or endorsed by the publisher.
Research integrity at Frontiers
Learn more about the work of our research integrity team to safeguard the quality of each article we publish.