- 1Department of Human Medicine, School of Medicine and Health Sciences, Klinikum Oldenburg, Carl von Ossietzky Universität Oldenburg and University Hospital for General and Visceral Surgery, Oldenburg, Germany
- 2Department of Human Medicine, School of Medicine and Health Sciences, Carl von Ossietzky Universität Oldenburg, Oldenburg, Germany
- 3Center for Blistering Diseases, University Medical Center Groningen, University of Groningen, Groningen, Netherlands
- 4Department of Pathology and Medical Biology, University Medical Center Groningen, University of Groningen, Groningen, Netherlands
- 5Department of Pathology, Antwerp University Hospital, University of Antwerp, Antwerp, Belgium
Introduction: Colorectal cancer (CRC) ranks as the third most prevalent malignancy globally, with a concerning rise in incidence among young adults. Despite progress in understanding genetic predispositions and lifestyle risk factors, the intricate molecular mechanisms of CRC demand exploration. MicroRNAs (miRNAs) emerge as key regulators of gene expression and their deregulation in tumor cells play pivotal roles in cancer progression.
Methods: NanoString's nCounter technology was utilized to measure the expression of 827 cancer-related miRNAs in tumor tissue and adjacent non-involved normal colon tissue from five patients with locoregional CRC progression. These expression profiles were then compared to those from the primary colon adenocarcinoma (COAD) cohort in The Cancer Genome Atlas (TCGA).
Results and discussion: Intriguingly, 156 miRNAs showed a contrasting dysregulation pattern in reccurent tumor compared to their expression in the TCGA COAD cohort. This observation implies dynamic alterations in miRNA expression patterns throughout disease progression. Our exploratory study contributes to understanding the regulatory landscape of recurrent CRC, emphasizing the role of miRNAs in disease relapse. Notable findings include the prominence of let-7 miRNA family, dysregulation of key target genes, and dynamic changes in miRNA expression patterns during progression. Univariate Cox proportional hazard models highlighted miRNAs associated with adverse outcomes and potential protective factors. The study underscores the need for more extensive investigations into miRNA dynamics during tumor progression and the value of stage specific biomarkers for prognosis.
1 Introduction
Colorectal cancer (CRC) is the third most common malignancy worldwide and the second leading cause of cancer death (1). Beyond genetic predisposition (2), the multifaceted etiology of CRC involves an interplay of risk factors such as dietary habits, age, obesity, and physical inactivity, alongside protective factors like regular physical activity and diets rich in fruits, vegetables, and fiber (3–6). Eighty of CRC are localized at the time of diagnosis and surgical resection remains the only curative option (4). However, the recurrence rate of CRC is between 30 to 50% and local recurrence without distal metastases is the most frequent recurrence (7). The recurrence and survival are most influenced by the stage of the tumor. Unraveling the intricate molecular mechanisms underlying CRC necessitates exploring beyond conventional risk factors, delving into the realm of molecular markers. The incidence of CRC is increasing, particularly in the population under 50 (8, 9). Regular screening remains pivotal (10–12), and novel markers are needed for CRC diagnosis, as well as to predict the response to treatment (4, 7).
MicroRNAs (miRNAs) are short non-coding RNAs, 17-25 nucleotides long, that were discovered in 1993 (13). In the past few decades, they have emerged as crucial regulators of gene expression. MiRNAs regulate the gene expression predominantly through the inhibition or degradation of messenger RNAs (14). MiRNAs are also being investigated as possible biomarkers of various diseases (15–17), as well as cancer (18–20). In cancer, including CRC, miRNAs have significant influence on disease prognosis, metastasis and response to treatment. MiRNAs orchestrate complex networks that govern key cellular processes (21–24).
MicroRNAs (miRNAs) are critically involved in the advancement and metastasis of colorectal cancer (CRC). Notably, miRNAs exhibit a dichotomous nature, with some functioning as oncogenic (e.g., miR21, miR221/222, miR135, miR223) and others as tumor-suppressive (e.g., miR-143/145, miR-203, miR-200 family) agents regulating Wnt/β-catenin and TGF-β signaling in CRC (25).
While elevated levels of miR-221 in tumor tissues have been reported in some studies (26, 27), there was no observed difference in miRNA-221 expression between locoregional recurrent CRC tumor tissue and healthy tissue. Increased miR-221 levels have been associated with poor overall survival and a negative prognosis in cancer (28), and it has also been implicated in affecting the efficacy of chemotherapy (29–32). However, the comprehensive understanding of the role of miRNAs in cancer development and progression, particularly within the specific context of CRC, remains an active area of investigation that necessitates further exploration. Particularly, the literature lacks substantial information regarding the miRNA profile of recurrent CRC.
Our exploratory study provides initial insights into the relationship between miRNA expression and tumor progression by investigating the miRNA profile of locoregional recurrent CRC, comparing it to the healthy surrounding tissue within the same patient. While previous studies have explored miRNA expression in primary and metastatic CRC tumors (33, 34), a focused examination of the distinct miRNA landscape associated with locoregional recurrence is lacking. This investigation might fill a critical gap in current understanding of the molecular intricacies underlying CRC relapse and may pave the way for identifying potential therapeutic targets.
2 Materials and methods
2.1 Selection of patients and patient material
Samples from cancerous and healthy tissue were obtained from 5 patients with a locoregional recurrent CRC that were analyzed in a previous study (35). The cancerous part was taken from the malignancy, while the healthy tissue was taken from the tumor-free tissue margin of the same specimen. Patient characteristics are summarized in Table 1.
2.2 RNA isolation
Total RNA from 5 tissue sections (20 µm thick) was isolated using the miRNeasy FFPE Kit (Qiagen, Germany) as previously described (35). Briefly, sections were deparaffinized with Heptane. After incubation with proteinase K and heat inactivated samples were centrifuged for 15 min at 20.000 x g. DNA was digested with DNase I. Lysate was then mixed with 100% ethanol and applied to an RNeasy MiniElute spin column. After washing the column RNA was eluted in 30 µl RNase-free water. Total RNA was quantified using the Qubit RNA high sensitivity assay (ThermoFisher) according to supplier’s instructions.
2.3 NanoString miRNA expression profiling
The NanoString nCounter technology (NanoString Technologies, Seattle, WA) was used to quantify miRNAs expression according to supplier’s instructions (MAN-C0009-07). Briefly, 100 ng total RNA was used in the adaptor ligation step and subsequently 10 ng was used for hybridization with miRNA capture probes (humanv3: Catalog number CSO-MIR3-12). Post-hybridization, samples were processed on an nCounter SPRINT platform according to supplier’s instructions (MAN-10017).
2.4 Data analysis
Data from NanoString assay were analyzed by Rosalind (Rosalind.bio). Normalization, fold changes and p-values were calculated using default criteria provided by NanoString. After background subtraction using POS_A probe correction factors, normalization was conducted in two stages: positive control normalization and codeset normalization. The codeset normalization factor was determined based on the 100 probes with the highest counts. For both stages, the geometric mean of each probeset is utilized to create a normalization factor. ROSALIND calculates fold changes and p-values for comparisons using the t-test method. P-value adjustment was carried out using the Benjamini-Hochberg method to estimate false discovery rates (FDR). The top targeted gene predictions were analyzed in ROSALIND using the multiMiR R library (36).
Gene set enrichment analysis (GSEA) adapted for microRNAs was performed using miRNA Enrichment Analysis and Annotation Tool (miEAA) 2.1 webserver (37).
MiRNA expression data from The Cancer Genome Atlas (TCGA) were analyzed in R. Data from the TCGA Colon Adenocarcinoma (COAD) project, including clinical data and miRNA expression data of 461 patients with CRC, was downloaded using the TCGAbiolinks R package (38). Differential expression was calculated using the DESeq2 R package after removal of miRNAs with less than 10 reads across all the samples and variance stabilizing transformation (39). P-value adjustment was performed using the Benjamini-Hochberg method of estimating false discovery rates (FDR).
Univariate Cox proportional hazard models and Kaplan-Meier curves were generated using the survival and survminer R packages (40).
3 Results
Utilizing NanoString analysis on a comprehensive panel of 827 cancer-related miRNA targets, we successfully detected 798 miRNAs. A comparative analysis of paired normal biopsies from the tumor-free tissue margin and recurrent tumor biopsies from the malignancies of five subjects revealed significant dysregulation in 588 targets (Figure 1). MiRNA expression in normal and tumor tissue of the top up- and down- regulated miRNAs (Figure 1B) is shown in Supplementary Figure 1.
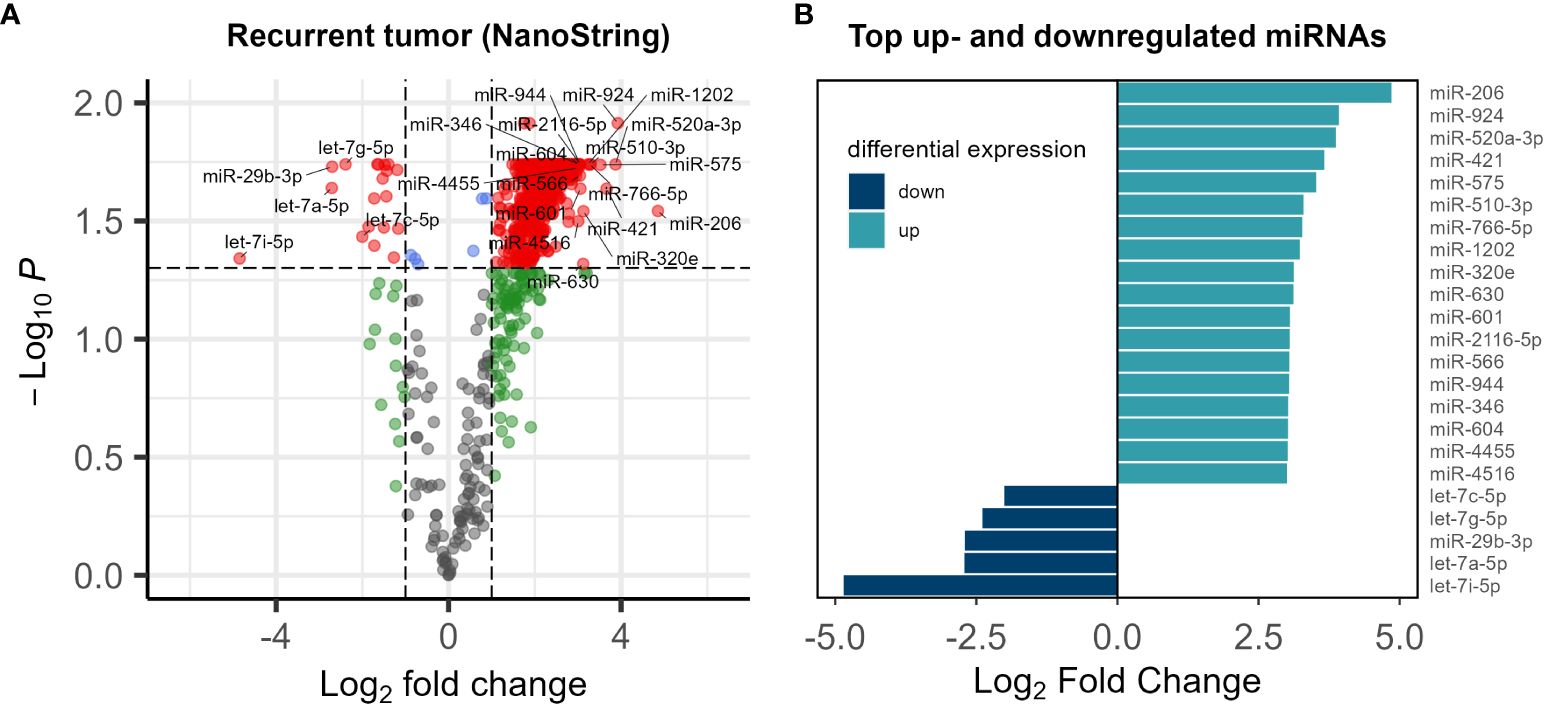
Figure 1 (A) Volcano plot of the miRNA expression analysis. Log2 fold change vs. log10 p-value of 798 miRNAs in 5 patients. Significantly dysregulated miRNAs (p-value<0.05) are shown in red (B) list of top up- and down- regulated miRNAs Log2 fold change <-2 or >2 and p-value<0.05.
Our investigation into CRC relapse identified key target genes, shedding light on potential dysregulation in miRNA processing (Figure 2).
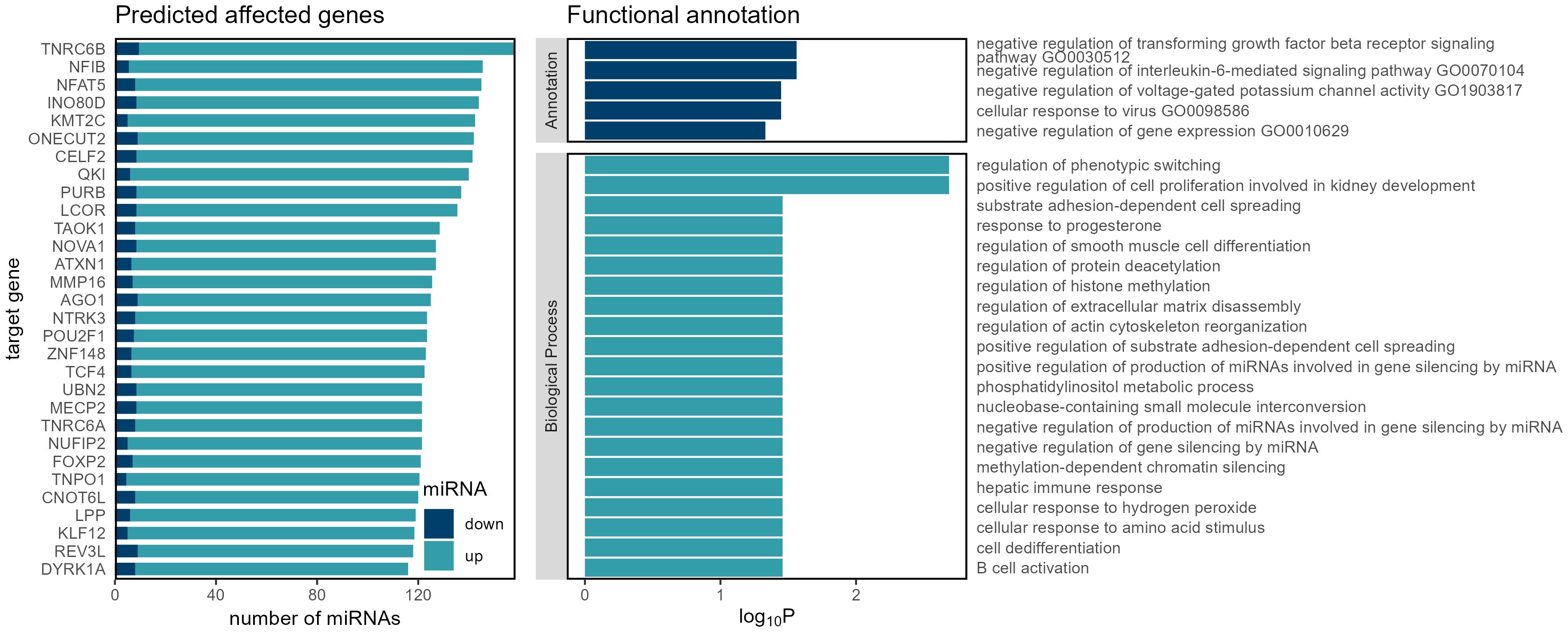
Figure 2 Genes, functions and biological processes that are affected by miRNA dysregulation in recurrent colorectal carcinoma.
The identification of these targets prompted an exploration of enriched biological processes, revealing pathways related to the negative regulation of interleukin-6-mediated signaling, negative regulation of transforming growth factor beta receptor signaling, and cellular response to virus — processes commonly intertwined with immune response and signaling cascades implicated in cancer progression (41, 42).
In our subsequent investigation, we aimed to discern differences in miRNA expression between primary and recurrent tumors. Lacking paired tissue samples from the same patients, we conducted a differential expression analysis using publicly available data from the colon adenocarcinoma (COAD) cohort of The Cancer Genome Atlas (TCGA) database. The dataset included miRNA expression information from 461 patients, encompassing 8 normal tissue samples and 455 primary tumor samples. Due to the scarcity of recurrent and metastatic tumor representation in the cohort (only 1 sample each), these particular samples were excluded from further analysis. The comparative analysis between healthy and primary tumor tissues unveiled dysregulation in 588 out of 1881 detected miRNAs. Remarkably, when comparing our dataset of recurrent tumors with the TCGA dataset of primary tumors, we found an overlap of 777 miRNAs out of the 798 detected in our recurrent tumor dataset. Notably, among the miRNAs that showed dysregulation in both primary and recurrent tumors, 156 exhibited an opposite misregulation pattern., i.e. some were upregulated in primary tumors while downregulated in recurrent tumors, or vice versa, as illustrated in Figure 3. This intriguing observation suggests dynamic changes in miRNA expression patterns during the transition from primary to recurrent tumors, highlighting potential regulatory shifts associated with disease progression.
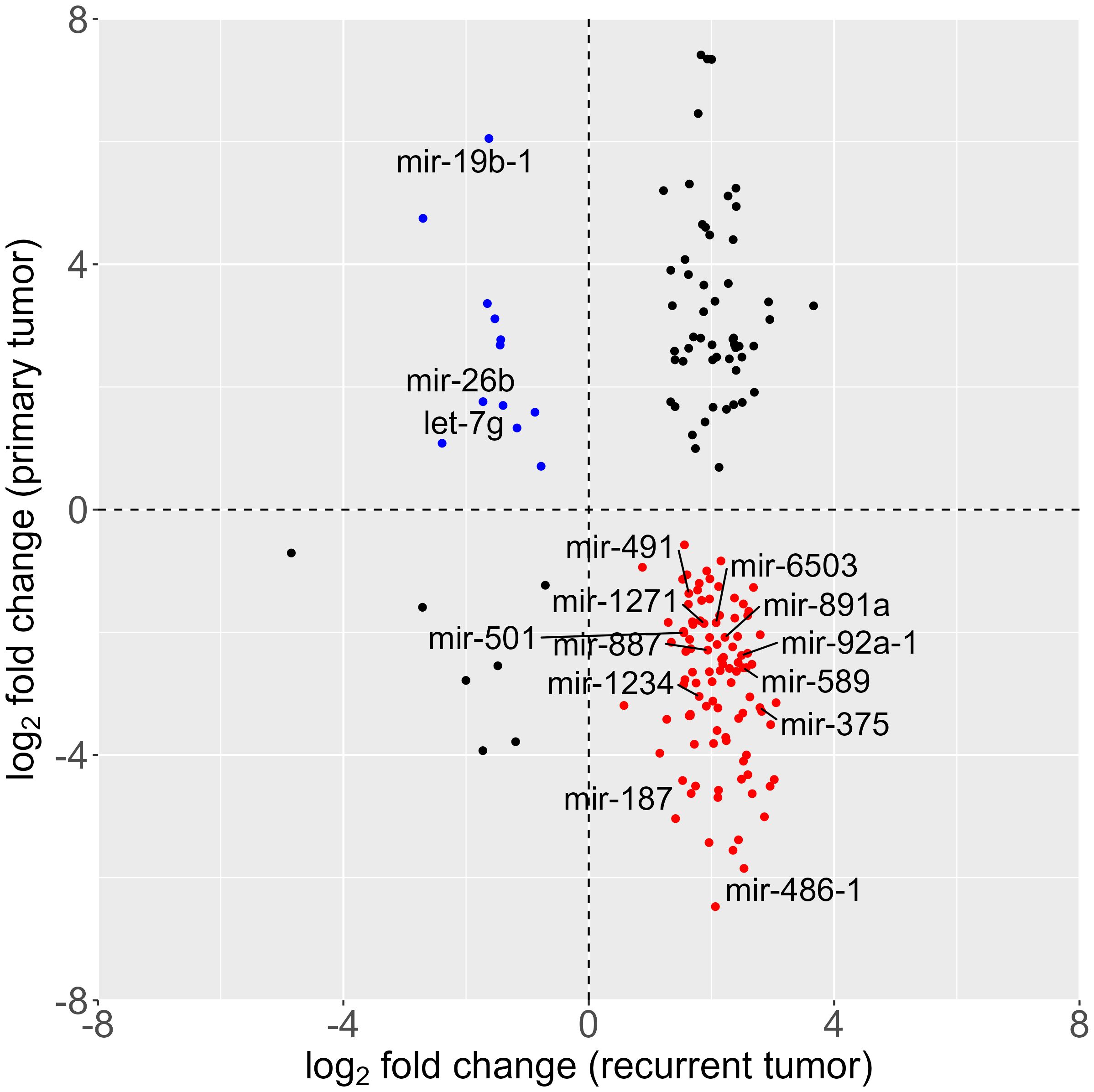
Figure 3 Scatter plot of differential miRNA expression; log2-fold change of tumor vs. healthy tissue in primary (analysis of the colorectal carcinoma cohort, data downloaded from The Cancer Genome Atlas database) and recurrent (NanoString analysis) tumor. (red: overexpressed in recurrent and underexpressed in primary tumor; blue: overexpressed in primary tumor and underexpressed in recurrent tumor).
We then conducted univariate Cox proportional hazard models to assess the correlation between miRNAs with opposite regulation in primary and recurrent tumors and overall survival. The TCGA COAD cohort, lacking data on disease-free progression or time to recurrence, limited our analysis to overall survival as the sole predictor. Out of the 156 miRNAs with opposite dysregulation patterns in primary and recurrent tumors, 14 had statistically significant associations with overall survival, as evidenced by their respective hazard ratios and p-values (Table 2).
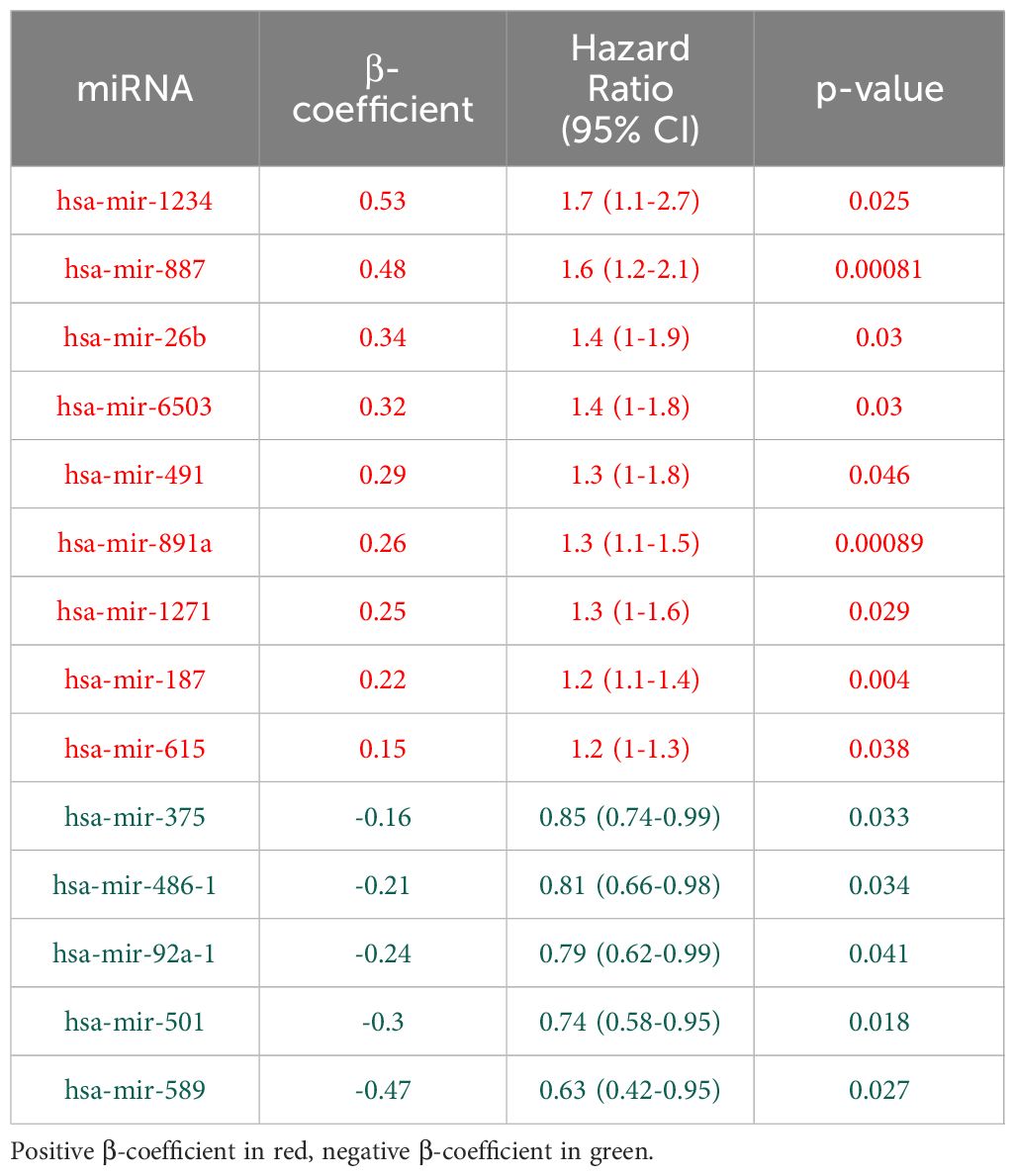
Table 2 Univariate Cox proportional hazard models of miRNAs that show different expression pattern in primary (dataset downloaded from The Cancer Genome Atlas database) and recurrent tumor (NanoString analysis).
The positive β-coefficients and hazard ratios for certain miRNAs (e.g. hsa-mir-1234, hsa-mir-887, hsa-mir-26b) suggest a potential association with increased risk of adverse outcomes. Conversely, miRNAs with negative β-coefficients and hazard ratios below 1 (e.g. hsa-mir-375, hsa-mir-486-1, hsa-mir-92a-1) may indicate a potential protective effect. Supplementary Figure 2 presents the miRNA expression levels in normal and recurrent tumor tissues, while Figure 4 displays the corresponding Kaplan-Meier survival curves.
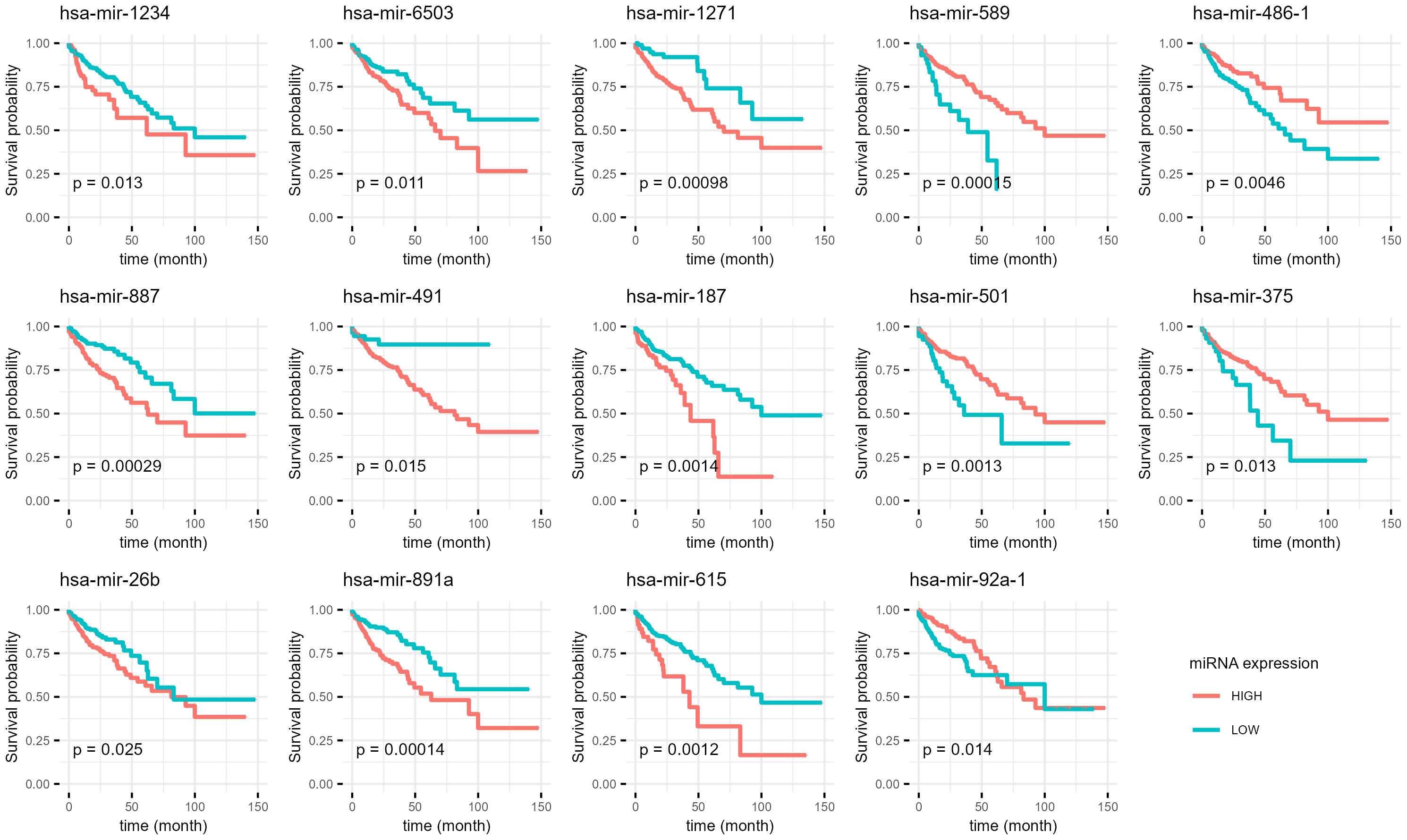
Figure 4 Kaplan-Meier curves of miRNAs which are differentially expressed in primary and recurrent tumor and potential predictors of overall survival. The data from the NanoString analysis of our samples was compared with publicly available dataset from The Cancer Genome Atlas database. P-Value (p) for each is shown for every graph.
4 Discussion
In the broader context of microRNA expression patterns in recurrent CRC, our exploratory study contributes to the growing but still limited understanding of the intricate regulatory landscape associated with disease relapse. MiRNAs play crucial roles in various cellular processes, and their dysregulation has been implicated in cancer progression (43, 44), including CRC (25, 45, 46). In a previous study (35) we focused on the expression of four specific miRNAs — miRNA-21, miRNA-215, miRNA-221, and miRNA-222 — that are implicated in fibrosis and angiogenesis in recurrent CRC (35). A highly variable expression was observed, with only miRNA-21 showing significant upregulation. Our exploratory study of CRC recurrence through miRNA expression analysis has provided insights into the intricate molecular landscape associated with disease recurrence. The dysregulation of 588 miRNAs out of 798 targets (Figure 1), with a notable prominence of the let-7 family, aligns with emerging evidence implicating let-7 in CRC development and progression (47–49). Let-7 miRNAs is downregulated in several cancers and is thought to act as a tumor suppressor (47, 50, 51). Notably, miRNAs of the let-7 family emerged as prominently overrepresented among the differentially expressed miRNAs recurrent tumors. This finding provides a nuanced understanding of the miRNA landscape associated with CRC relapse, emphasizing the potential involvement of the let-7 family in the underlying molecular mechanisms of disease recurrence.
Beyond this, the identification of key target genes (Figure 2), including Argonaute RNA-induced silencing complex (RISC) Catalytic Component 1 (AGO1), Trinucleotide Repeat Containing Adaptor 6B (TNRC6B), NOVA Alternative Splicing Regulator 1 (NOVA1), and Matrix Metallopeptidase 16 (MMP16), offers valuable clues into potential dysregulation in miRNA processing during cancer relapse (52–55).
AGO1 is a central player in RISC, and both the TNRC6B and NOVA1 are associated with RNA processing. This suggests a link between miRNA dysregulation and the intricate regulatory mechanisms driving cancer relapse (53, 56, 57). These findings echo broader discussions in the field regarding the pivotal role of AGO proteins and their interplay with miRNAs in cancer progression (58). The connection with RNA processing factors, like TNRC6B and NOVA1, further underscores the multifaceted nature of miRNA-mediated regulatory networks in the context of cancer recurrence (57, 59, 60).
MMP16 is a member of family of proteolytic enzymes and has a high expression in certain tumors (52, 61–63). It is known for its role in extracellular matrix remodeling. Studies link it to processes involved in cancer proliferation and metastasis in several types of cancers (64–66), including CRC (52, 52).
The observed link between these target genes and enriched biological processes associated with miRNA processing highlights the intricate regulatory networks potentially contributing to CRC relapse. Further investigation into AGO1, TNRC6B, NOVA1, MMP16, and their connection to miRNA processing could provide deeper insights into the molecular mechanisms underlying cancer recurrence.
Expanding our analysis to the TCGA COAD cohort, we observed an overlap of 777 miRNAs between recurrent tumors and primary tumors, with 156 miRNAs exhibiting opposite dysregulation patterns. This observation aligns with recent studies highlighting the dynamic changes in miRNA expression during cancer progression (43, 67, 68), including in CRC (25). The identification of these miRNAs prompts further investigations into their functional roles and the underlying molecular mechanisms associated with the transition from primary to recurrent tumors (69). MiRNA-19b has been identified as a promising prognostic marker for patient recurrence in CRC in previous studies (70, 71). Suppression of exosomal miR-19b could impact oxaliplatin sensitivity in CRC cell line (72) and miR-19b independently predicted both patient outcome and response to preoperative chemotherapy (70). Interestingly, our current study presents a nuanced perspective, as miRNA-19b was found to be upregulated in the TCGA cohort of primary tumors and downregulated in our cohort of tumor relapse. This discrepancy further underlines the need for further and more extensive studies.
The application of univariate Cox proportional hazard models offered insights into the prognostic significance of miRNAs with distinct expression patterns in primary and recurrent tumors. MiRNAs such as hsa-mir-1234, hsa-mir-887, and hsa-mir-26b, exhibiting positive hazard ratios, align with discussions on miRNA biomarkers associated with adverse outcomes in CRC (26, 73). Conversely, hsa-mir-375, hsa-mir-486-1, and hsa-mir-92a-1, with negative hazard ratios, resonate with studies highlighting miRNAs as potential protective factors in cancer progression (74, 75).
It is essential to acknowledge the limitations of our study, particularly the small sample size (n=5) in an exploratory setting, highlighting the need for more extensive investigations to draw robust conclusions. The use of FFPE samples is a technical limitation of this study. However, it has been shown that NanoString technology is robust and effective even with low-quality RNA samples isolated from FFPE tissue. This technology offers advantages such as sensitivity, reproducibility, and technical robustness in challenging sample conditions (76).
In summary, our study offers preliminary insights into the landscape of recurrent CRC research through integrated miRNA expression analysis, target gene identification, and functional enrichment. While acknowledging the limitations of our small sample size, the dysregulated miRNAs, target genes, and enriched biological processes identified in our study provide valuable groundwork for further investigation. These findings contribute to our evolving understanding of cancer recurrence and offer valuable insights for ongoing discussions in the field. Further investigations into the specific roles of miRNAs, target genes, and the dynamic changes in miRNA expression patterns will be necessary for advancing therapeutic strategies and refining prognostic assessments in CRC.
Data availability statement
The raw data supporting the conclusions of this article will be made available by the authors, without undue reservation.
Ethics statement
The studies involving humans were approved by Medizinische Ethikkommision Oldenburg. The studies were conducted in accordance with the local legislation and institutional requirements. The ethics committee/institutional review board waived the requirement of written informed consent for participation from the participants or the participants’ legal guardians/next of kin because this is a retrospective study and some patients had deceased prior to given consent. Data was fully anonymized. Surviving patients have consented to the use of their clinical data and tissue samples.
Author contributions
NM: Conceptualization, Data curation, Formal analysis, Funding acquisition, Investigation, Project administration, Software, Supervision, Visualization, Writing – original draft, Writing – review & editing. NK: Writing – original draft, Writing – review & editing. GN: Writing – original draft, Writing – review & editing. LV: Investigation, Methodology, Writing – review & editing. MM: Investigation, Writing – review & editing. MB: Funding acquisition, Resources, Supervision, Writing – review & editing. AT: Conceptualization, Funding acquisition, Resources, Supervision, Writing – review & editing.
Funding
The author(s) declare that no financial support was received for the research, authorship, and/or publication of this article.
Acknowledgments
We acknowledge the use of generative AI technology in producing written content for this manuscript. Specifically, parts of the manuscripts were refined using ChatGPT, version 3.5, a language model developed by OpenAI.
Conflict of interest
The authors declare that the research was conducted in the absence of any commercial or financial relationships that could be construed as a potential conflict of interest.
Publisher’s note
All claims expressed in this article are solely those of the authors and do not necessarily represent those of their affiliated organizations, or those of the publisher, the editors and the reviewers. Any product that may be evaluated in this article, or claim that may be made by its manufacturer, is not guaranteed or endorsed by the publisher.
Supplementary material
The Supplementary Material for this article can be found online at: https://www.frontiersin.org/articles/10.3389/fonc.2024.1407217/full#supplementary-material
References
1. Bray F, Laversanne M, Sung H, Ferlay J, Siegel RL, Soerjomataram I, et al. Global cancer statistics 2022: GLOBOCAN estimates of incidence and mortality worldwide for 36 cancers in 185 countries. CA: A Cancer J Clin. (2024) 74:229–63. doi: 10.3322/caac.21834
2. Hampel H, Kalady MF, Pearlman R, Stanich PP. Hereditary colorectal cancer. Hematology/Oncology Clinics. (2022) 36:429–47. doi: 10.1016/j.hoc.2022.02.002
3. Song M, Garrett WS, Chan AT. Nutrients, foods, and colorectal cancer prevention. Gastroenterology. (2015) 148:1244–1260.e1216. doi: 10.1053/j.gastro.2014.12.035
4. Thanikachalam K, Khan G. Colorectal cancer and nutrition. Nutrients. (2019) 11:164. doi: 10.3390/nu11010164
5. Zhou E, Rifkin S. Colorectal cancer and diet: risk versus prevention, is diet an intervention? Gastroenterol Clinics. (2021) 50:101–11. doi: 10.1016/j.gtc.2020.10.012
6. Sninsky JA, Shore BM, Lupu GV, Crockett SD. Risk factors for colorectal polyps and cancer. Gastrointestinal Endoscopy Clinics. (2022) 32:195–213. doi: 10.1016/j.giec.2021.12.008
7. Guraya SY. Pattern, stage, and time of recurrent colorectal cancer after curative surgery. Clin Colorectal Cancer. (2019) 18:e223–8. doi: 10.1016/j.clcc.2019.01.003
8. Patel SG, Ahnen DJ. Colorectal cancer in the young. Curr Gastroenterol Rep. (2018) 20:1–12. doi: 10.1007/s11894-018-0618-9
9. Baidoun F, Elshiwy K, Elkeraie Y, Merjaneh Z, Khoudari G, Sarmini MT, et al. Colorectal cancer epidemiology: recent trends and impact on outcomes. Curr Drug Targets. (2021) 22:998–1009. doi: 10.2174/18735592MTEx9NTk2y
10. Fitzpatrick-Lewis D, Ali MU, Warren R, Kenny M, Sherifali D, Raina P. Screening for colorectal cancer: a systematic review and meta-analysis. Clin colorectal Cancer. (2016) 15:298–313. doi: 10.1016/j.clcc.2016.03.003
11. Bretthauer M, Løberg M, Wieszczy P, Kalager M, Emilsson L, Garborg K, et al. Effect of colonoscopy screening on risks of colorectal cancer and related death. New Engl J Med. (2022) 387:1547–56. doi: 10.1056/NEJMoa2208375
12. Gupta S. Screening for colorectal cancer. Hematology/Oncology Clinics. (2022) 36:393–414. doi: 10.1016/j.hoc.2022.02.001
13. Lee RC, Feinbaum RL, Ambros V. The C. elegans heterochronic gene lin-4 encodes small RNAs with antisense complementarity to lin-14. cell. (1993) 75:843–54. doi: 10.1016/0092-8674(93)90529-Y
14. Correia de Sousa M, Gjorgjieva M, Dolicka D, Sobolewski C, Foti M. Deciphering miRNAs’ action through miRNA editing. Int J Mol Sci. (2019) 20:6249. doi: 10.3390/ijms20246249
15. Wu HZY, Ong KL, Seeher K, Armstrong NJ, Thalamuthu A, Brodaty H, et al. Circulating microRNAs as biomarkers of Alzheimer’s disease: a systematic review. J Alzheimer’s Dis. (2016) 49:755–66. doi: 10.3233/JAD-150619
16. Zheng M-L, Zhou N-K, Luo C-H. MiRNA-155 and miRNA-132 as potential diagnostic biomarkers for pulmonary tuberculosis: A preliminary study. Microbial pathogenesis. (2016) 100:78–83. doi: 10.1016/j.micpath.2016.09.005
17. Zhou S-S, Jin J-P, Wang J-Q, Zhang Z-G, Freedman JH, Zheng Y, et al. miRNAS in cardiovascular diseases: potential biomarkers, therapeutic targets and challenges. Acta Pharmacologica Sin. (2018) 39:1073–84. doi: 10.1038/aps.2018.30
18. Bertoli G, Cava C, Castiglioni I. MicroRNAs: new biomarkers for diagnosis, prognosis, therapy prediction and therapeutic tools for breast cancer. Theranostics. (2015) 5:1122. doi: 10.7150/thno.11543
19. Daoud AZ, Mulholland EJ, Cole G, Mccarthy HO. MicroRNAs in Pancreatic Cancer: Biomarkers, prognostic, and therapeutic modulators. BMC Cancer. (2019) 19:1–13. doi: 10.1186/s12885-019-6284-y
20. He B, Zhao Z, Cai Q, Zhang Y, Zhang P, Shi S, et al. miRNA-based biomarkers, therapies, and resistance in Cancer. Int J Biol Sci. (2020) 16:2628. doi: 10.7150/ijbs.47203
21. To KK, Tong CW, Wu M, Cho WC. MicroRNAs in the prognosis and therapy of colorectal cancer: From bench to bedside. World J Gastroenterol. (2018) 24:2949. doi: 10.3748/wjg.v24.i27.2949
22. Budakoti M, Panwar AS, Molpa D, Singh RK, Büsselberg D, Mishra AP, et al. Micro-RNA: The darkhorse of cancer. Cell Signalling. (2021) 83:109995. doi: 10.1016/j.cellsig.2021.109995
23. Zhu X, Kudo M, Huang X, Sui H, Tian H, Croce CM, et al. Frontiers of microRNA signature in non-small cell lung cancer. Front Cell Dev Biol. (2021) 9:643942. doi: 10.3389/fcell.2021.643942
24. Mangiapane G, Pascut D, Dalla E, Antoniali G, Degrassi M, Croce LS, et al. Clinical significance of apurinic/apyrimidinic endodeoxyribonuclease 1 and microRNA axis in hepatocellular carcinoma. J Clin Transl Hepatol. (2023) 11:1291–307. doi: 10.14218/JCTH.2022.00179
25. Huang X, Zhu X, Yu Y, Zhu W, Jin L, Zhang X, et al. Dissecting miRNA signature in colorectal cancer progression and metastasis. Cancer Lett. (2021) 501:66–82. doi: 10.1016/j.canlet.2020.12.025
26. Nishida N, Nagahara M, Sato T, Mimori K, Sudo T, Tanaka F, et al. Microarray analysis of colorectal cancer stromal tissue reveals upregulation of two oncogenic miRNA clusters. Clin Cancer Res. (2012) 18:3054–70. doi: 10.1158/1078-0432.CCR-11-1078
27. Yau T, Wu C, Dong Y, Tang C, Ng S, Chan F, et al. microRNA-221 and microRNA-18a identification in stool as potential biomarkers for the non-invasive diagnosis of colorectal carcinoma. Br J Cancer. (2014) 111:1765–71. doi: 10.1038/bjc.2014.484
28. Cai K, Shen F, Cui J-H, Yu Y, Pan H-Q. Expression of miR-221 in colon cancer correlates with prognosis. Int J Clin Exp Med. (2015) 8:2794.
29. Zhao Y, Zhao L, Ischenko I, Bao Q, Schwarz B, Nieß H, et al. Antisense inhibition of microRNA-21 and microRNA-221 in tumor-initiating stem-like cells modulates tumorigenesis, metastasis, and chemotherapy resistance in pancreatic cancer. Targeted Oncol. (2015) 10:535–48. doi: 10.1007/s11523-015-0360-2
30. Chen D, Yan W, Liu Z, Zhang Z, Zhu L, Liu W, et al. Downregulation of miR-221 enhances the sensitivity of human oral squamous cell carcinoma cells to Adriamycin through upregulation of TIMP3 expression. Biomedicine Pharmacotherapy. (2016) 77:72–8. doi: 10.1016/j.biopha.2015.12.002
31. Liu P, Sun M, Jiang W, Zhao J, Liang C, Zhang H. Identification of targets of miRNA-221 and miRNA-222 in fulvestrant-resistant breast cancer. Oncol Lett. (2016) 12:3882–8. doi: 10.3892/ol.2016.5180
32. Zhao L, Zou D, Wei X, Wang L, Zhang Y, Liu S, et al. MiRNA-221-3p desensitizes pancreatic cancer cells to 5-fluorouracil by targeting RB1. Tumor Biol. (2016) 37:16053–63. doi: 10.1007/s13277-016-5445-8
33. Qi C, Chen Y, Zhou Y, Huang X, Li G, Zeng J, et al. Delineating the underlying molecular mechanisms and key genes involved in metastasis of colorectal cancer via bioinformatics analysis. Oncol Rep. (2018) 39:2297–305. doi: 10.3892/or
34. Zhang T, Guo J, Gu J, Wang Z, Wang G, Li H, et al. Identifying the key genes and microRNAs in colorectal cancer liver metastasis by bioinformatics analysis and in vitro experiments. Oncol Rep. (2019) 41:279–91. doi: 10.3892/or.2018.6840
35. Kotnik N, El-Sourani N, Raap U, Raab H-R, Bockhorn M, Meyer H, et al. Expression of microRNA in locoregional recurrent rectal cancer. Anticancer Res. (2020) 40:2947–53. doi: 10.21873/anticanres.14273
36. Ru Y, Kechris KJ, Tabakoff B, Hoffman P, Radcliffe RA, Bowler R, et al. The multiMiR R package and database: integration of microRNA-target interactions along with their disease and drug associations. Nucleic Acids Res. (2014) 42:e133. doi: 10.1093/nar/gku631
37. Aparicio-Puerta E, Hirsch P, Schmartz GP, Kern F, Fehlmann T, Keller A. miEAA 2023: updates, new functional microRNA sets and improved enrichment visualizations. Nucleic Acids Res. (2023) 51:W319–25. doi: 10.1093/nar/gkad392
38. Colaprico A, Silva TC, Olsen C, Garofano L, Cava C, Garolini D, et al. TCGAbiolinks: an R/Bioconductor package for integrative analysis of TCGA data. Nucleic Acids Res. (2016) 44:e71. doi: 10.1093/nar/gkv1507
39. Love MI, Huber W, Anders S. Moderated estimation of fold change and dispersion for RNA-seq data with DESeq2. Genome Biol. (2014) 15:550. doi: 10.1186/s13059-014-0550-8
40. Therneau TM. A Package for Survival Analysis in R (2023). Available online at: https://CRAN.R-project.org/package=survival (Accessed 28.02.2024 2024).
41. Ungefroren H. TGF-beta signaling in cancer: control by negative regulators and crosstalk with proinflammatory and fibrogenic pathways. Cancers. (2019) 11(3):384. doi: 10.3390/cancers11030384
42. Hirano T. IL-6 in inflammation, autoimmunity and cancer. Int Immunol. (2021) 33(3):127–48. doi: 10.1093/intimm/dxaa078
43. Hussen BM, Hidayat HJ, Salihi A, Sabir DK, Taheri M, Ghafouri-Fard S. MicroRNA: A signature for cancer progression. Biomedicine Pharmacotherapy. (2021) 138:111528. doi: 10.1016/j.biopha.2021.111528
44. Li B, Cao Y, Sun M, Feng H. Expression, regulation, and function of exosome-derived miRNAs in cancer progression and therapy. FASEB J. (2021) 35:e21916. doi: 10.1096/fj.202100294RR
45. Balacescu O, Sur D, Cainap C, Visan S, Cruceriu D, Manzat-Saplacan R, et al. The impact of miRNA in colorectal cancer progression and its liver metastases. Int J Mol Sci. (2018) 19:3711. doi: 10.3390/ijms19123711
46. Wang D, Wang X, Song Y, Si M, Sun Y, Liu X, et al. Exosomal miR-146a-5p and miR-155-5p promote CXCL12/CXCR7-induced metastasis of colorectal cancer by crosstalk with cancer-associated fibroblasts. Cell Death Dis. (2022) 13:380. doi: 10.1038/s41419-022-04825-6
47. Mizuno R, Kawada K, Sakai Y. The molecular basis and therapeutic potential of Let-7 microRNAs against colorectal cancer. Can J Gastroenterol Hepatol. (2018) 19:5769591. doi: 10.1155/2018/5769591
48. Chang CM, Wong HS, Huang CY, Hsu WL, Maio ZF, Chiu SJ, et al. Functional effects of let-7g expression in colon cancer metastasis. Cancers. (2019) 11(4):489. doi: 10.3390/cancers11040489
49. Niculae AM, Dobre M, Herlea V, Manuc TE, Trandafir B, Milanesi E, et al. Let-7 microRNAs are possibly associated with perineural invasion in colorectal cancer by targeting IGF axis. Life. (2022) 12:1638. doi: 10.3390/life12101638
50. Wang Y, Hu X, Greshock J, Shen L, Yang X, Shao Z, et al. Genomic DNA copy-number alterations of the let-7 family in human cancers. Plos One. (2012) 7(9):e44399. doi: 10.1371/journal.pone.0044399
51. Ferneza S, Fetsych M, Shuliak R, Makukh H, Volodko N, Yarema R, et al. Clinical significance of microRNA-200 and let-7 families expression assessment in patients with ovarian cancer. ecancermedicalscience. (2021) 15:1249. doi: 10.3332/ecancer.2021.1249
52. Wu S, Ma C, Shan S, Zhou L, Li W. High expression of matrix metalloproteinases 16 is associated with the aggressive Malignant behavior and poor survival outcome in colorectal carcinoma. Sci Rep. (2017) 7:46531. doi: 10.1038/srep46531
53. Xin Y, Li Z, Zheng H, Ho J, Chan MTV, Wu WKK. Neuro-oncological ventral antigen 1 (NOVA1): Implications in neurological diseases and cancers. Cell Prolif. (2017) 50(4):e12348. doi: 10.1111/cpr.12348
54. Wu G, Fan F, Hu P, Wang C. AGO1 enhances the proliferation and invasion of cholangiocarcinoma via the EMT-associated TGF-b signaling pathway. Am J Transl Res. (2020) 12:2890–902.
55. Sun X, Luo Z, Gong L, Tan X, Chen J, Liang X, et al. Identification of significant genes and therapeutic agents for breast cancer by integrated genomics. Bioengineered. (2021) 12:2140–54. doi: 10.1080/21655979.2021.1931642
56. de Rooij LA, Mastebroek DJ, Ten Voorde N, van der Wall E, Van Diest PJ, Moelans CB. The microRNA lifecycle in health and cancer. Cancers. (2022) 14(23):5748. doi: 10.3390/cancers14235748
57. Altuna-Coy A, Ruiz-Plazas X, Arreaza-Gil V, Segarra-Tomas J, Chacon MR. In silico analysis of prognostic and diagnostic significance of target genes from prostate cancer cell lines derived exomicroRNAs. Cancer Cell Int. (2023) 23(4):275. doi: 10.1186/s12935-023-03123-1
58. Nowak I, Sarshad AA. Argonaute proteins take center stage in cancers. Cancers. (2021) 13(4):788. doi: 10.3390/cancers13040788
59. Murakami Y, Tamori A, Itami S, Tanahashi T, Toyoda H, Tanaka M, et al. The expression level of miR-18b in hepatocellular carcinoma is associated with the grade of Malignancy and prognosis. BMC Cancer. (2013) 13:99. doi: 10.1186/1471-2407-13-99
60. Choi S, Cho N, Kim EM, Kim KK. The role of alternative pre-mRNA splicing in cancer progression. Cancer Cell Int. (2023) 23:249. doi: 10.1186/s12935-023-03094-3
61. Nakada M, Nakamura H, Ikeda E, Fujimoto N, Yamashita J, Sato H, et al. Expression and tissue localization of membrane-type 1, 2, and 3 matrix metalloproteinases in human astrocytic tumors. Am J Pathol. (1999) 154:417–28. doi: 10.1016/S0002-9440(10)65288-1
62. Bartsch JE, Staren ED, Appert HE. Matrix metalloproteinase expression in breast cancer. J Surg Res. (2003) 110:383–92. doi: 10.1016/S0022-4804(03)00007-6
63. Lowy AM, Clements WM, Bishop J, Kong L, Bonney T, Sisco K, et al. β-Catenin/Wnt signaling regulates expression of the membrane type 3 matrix metalloproteinase in gastric cancer. Cancer Res. (2006) 66:4734–41. doi: 10.1158/0008-5472.CAN-05-4268
64. Cao L, Chen C, Zhu H, Gu X, Deng D, Tian X, et al. MMP16 is a marker of poor prognosis in gastric cancer promoting proliferation and invasion. Oncotarget. (2016) 7:51865. doi: 10.18632/oncotarget.v7i32
65. Jiang C, Wang J, Dong C, Wei W, Li J, Li X. Membranous type matrix metalloproteinase 16 induces human prostate cancer metastasis. Oncol Lett. (2017) 14:3096–102. doi: 10.3892/ol.2017.6536
66. Shen Z, Wang X, Yu X, Zhang Y, Qin L. MMP16 promotes tumor metastasis and indicates poor prognosis in hepatocellular carcinoma. Oncotarget. (2017) 8:72197. doi: 10.18632/oncotarget.v8i42
67. Harrandah AM, Mora RA, Chan EK. Emerging microRNAs in cancer diagnosis, progression, and immune surveillance. Cancer Lett. (2018) 438:126–32. doi: 10.1016/j.canlet.2018.09.019
68. Pudova EA, Kobelyatskaya AA, Katunina IV, Snezhkina AV, Fedorova MS, Guvatova ZG, et al. Dynamic profiling of exosomal microRNAs in blood plasma of patients with castration-resistant prostate cancer. Front Biosci. (2022) 14:15. doi: 10.31083/j.fbs1402015
69. Oura K, Morishita A, Masaki T. Molecular and functional roles of microRNAs in the progression of hepatocellular carcinoma-A review. Int J Mol Sci. (2020) 21(21):8362. doi: 10.3390/ijms21218362
70. Santos A, Cristobal I, Rubio J, Carames C, Luque M, Sanz-Alvarez M, et al. MicroRNA-19b plays a key role in 5-fluorouracil resistance and predicts tumor progression in locally advanced rectal cancer patients. Int J Mol Sci. (2022) 23:12447. doi: 10.3390/ijms232012447
71. Santos A, Cristobal I, Carames C, Luque M, Sanz-Alvarez M, Madoz-Gurpide J, et al. Deregulation of the miR-19b/PPP2R5E signaling axis shows high functional impact in colorectal cancer cells. Int J Mol Sci. (2023) 24(9):7779. doi: 10.3390/ijms24097779
72. Gu Y, Yu J, Zhang J, Wang C. Suppressing the secretion of exosomal miR-19b by gw4869 could regulate oxaliplatin sensitivity in colorectal cancer. Neoplasma. (2019) 66:39–45. doi: 10.4149/neo_2018_180306N155
73. Wei HT, Guo EN, Liao XW, Chen LS, Wang JL, Ni M, et al. Genome−scale analysis to identify potential prognostic microRNA biomarkers for predicting overall survival in patients with colon adenocarcinoma. Oncol Rep. (2018) 40:1947–58. doi: 10.3892/or
74. Lang B, Zhao S. miR-486 functions as a tumor suppressor in esophageal cancer by targeting CDK4/BCAS2. Oncol Rep. (2018) 39:71–80. doi: 10.3892/or.2017.6064
75. Wei J, Lu Y, Wang R, Xu X, Liu Q, He S, et al. MicroRNA-375: potential cancer suppressor and therapeutic drug. Biosci Rep. (2021) 41(9):BSR20211494. doi: 10.1042/BSR20211494
Keywords: miRNA, recurrent colorectal cancer, locoregional colorectal cancer, Let-7, stagespecific expression, NanoString expression profiling
Citation: Meyer NH, Kotnik N, Noubissi Nzeteu GA, van Kempen LC, Mastik M, Bockhorn M and Troja A (2024) Unraveling the MicroRNA tapestry: exploring the molecular dynamics of locoregional recurrent rectal cancer. Front. Oncol. 14:1407217. doi: 10.3389/fonc.2024.1407217
Received: 26 March 2024; Accepted: 21 June 2024;
Published: 12 July 2024.
Edited by:
David Gibbons, St. Vincent’s University Hospital, IrelandReviewed by:
Erik Rene Lizárraga-Verdugo, Autonomous University of Sinaloa, MexicoMarwa Mahmoud Hussein, Cairo University, Egypt
Copyright © 2024 Meyer, Kotnik, Noubissi Nzeteu, van Kempen, Mastik, Bockhorn and Troja. This is an open-access article distributed under the terms of the Creative Commons Attribution License (CC BY). The use, distribution or reproduction in other forums is permitted, provided the original author(s) and the copyright owner(s) are credited and that the original publication in this journal is cited, in accordance with accepted academic practice. No use, distribution or reproduction is permitted which does not comply with these terms.
*Correspondence: N. Helge Meyer, aGVsZ2UubWV5ZXJAdW5pLW9sZGVuYnVyZy5kZQ==
†These authors share first authorship