- 1Department of Radiation Oncology, National Cancer Center/National Clinical Research Center for Cancer/Cancer Hospital, Chinese Academy of Medial Sciences and Peking Union Medical College, Beijing, China
- 2School of Physics and Technology, Wuhan University, Wuhan, China
- 3Department of Radiotherapy Technology, The Cancer and Tuberculosis Hospital, Jiamusi, China
Purpose: Difficulties remain in dose optimization and evaluation of cervical cancer radiotherapy that combines external beam radiotherapy (EBRT) and brachytherapy (BT). This study estimates and improves the accumulated dose distribution of EBRT and BT with deep learning–based dose prediction.
Materials and methods: A total of 30 patients treated with combined cervical cancer radiotherapy were enrolled in this study. The dose distributions of EBRT and BT plans were accumulated using commercial deformable image registration. A ResNet-101–based deep learning model was trained to predict pixel-wise dose distributions. To test the role of the predicted accumulated dose in clinic, each EBRT plan was designed using conventional method and then redesigned referencing the predicted accumulated dose distribution. Bladder and rectum dosimetric parameters and normal tissue complication probability (NTCP) values were calculated and compared between the conventional and redesigned accumulated doses.
Results: The redesigned accumulated doses showed a decrease in mean values of V50, V60, and D2cc for the bladder (−3.02%, −1.71%, and −1.19 Gy, respectively) and rectum (−4.82%, −1.97%, and −4.13 Gy, respectively). The mean NTCP values for the bladder and rectum were also decreased by 0.02‰ and 0.98%, respectively. All values had statistically significant differences (p < 0.01), except for the bladder D2cc (p = 0.112).
Conclusion: This study realized accumulated dose prediction for combined cervical cancer radiotherapy without knowing the BT dose. The predicted dose served as a reference for EBRT treatment planning, leading to a superior accumulated dose distribution and lower NTCP values.
1 Introduction
The combination of external beam radiotherapy (EBRT) and brachytherapy (BT) is a standard treatment for cervical cancer. The EBRT part of the treatment aims to treat the whole pelvis, including the tumor and the lymph nodes at risk. The BT part of the treatment aims to boost the residual tumor in multiple fractions. Ideally, all treatment parts should be combined for dose optimization and evaluation. However, EBRT and each fraction of BT are optimized independently in current clinical practice. This approach may result in a less than optimal accumulated dose distribution that limits the dosimetric advantage of combined radiotherapy. If treatment planners knew the possible accumulated dose distribution before EBRT treatment planning, then they could modify the dose to the organs at risk (OARs) from EBRT and leave more OAR dose space for BT. Additionally, the independent dose optimization results in multiple dose distributions associated with different planning images, which creates difficulties for total dose evaluation. Currently, radiation oncologists (ROs) only assess total dose to OARs by summing the dose-volume parameters of EBRT and BT. This approach is a “worst case assumption” and usually does not reflect the actual doses received by OARs, especially in the case of significant dose gradients from external beam boosts. If a concrete accumulated dose distribution could be predicted before treatment initiation, then ROs could estimate the curative effect and radiation toxicity, thereby helping make better treatment decisions. Therefore, accumulated dose prediction has potential benefits for both dose optimization and total dose estimation.
Deep learning is an advanced technique with the capability to build robust prediction models in complex feature extraction. Recently, deep learning has rapidly developed and been successfully implemented in medicine and radiotherapy applications, such as toxicity prediction, automatic segmentation, synthetic image generation, and quality assurance (1–6). A convolutional neural network (CNN) is a deep learning algorithm that automatically extracts multi-level features from input data, resulting in more concise and effective prediction. Research groups have developed prediction models based on CNNs and demonstrated their success in three-dimensional dose distribution prediction (7–12). Furthermore, knowledge-based planning (KBP) utilizing prior patient treatment plans to make dosimetric predictions for new patients has shown promise in assisting treatment planning. Studies have proved that KBP can improve plan quality consistency (13–18). For cervical cancer, these methods have been applied separately to EBRT or BT (19–32).
For accurate image registration and dose accumulation, a deformable image registration (DIR) method has been developed. This method accounts for anatomic variations and provides a spatial transformation relationship between volume elements of corresponding structures in different images. This transformation can be applied to dose distributions, thereby enabling dose accumulation with high-precision. Recent studies have proved the feasibility of DIR for evaluating accumulated doses in combined radiotherapy. However, DIR remains a challenge for combined cervical cancer radiotherapy as large and complex deformations are likely to occur in the pelvic cavity (33–38).
In this study, we attempted to predict accumulated dose distribution to assist treatment planning and obtain a superior dose distribution. We accumulated the dose distributions from EBRT and BT using commercial DIR and used them to train a CNN-based dose prediction model. Then, we quantitatively evaluated the prediction performance. The practicability of the predicted accumulated dose in clinic was verified by redesigning EBRT plans.
2 Materials and methods
2.1 Patient data
A total of 30 cervical cancer patients who underwent 25 fractions of EBRT using volumetric-modulated arc therapy (VMAT) followed by more than 3 fractions of high-dose-rate BT were selected in this study. CT scans were performed with a Brilliance CT Big Bore (Philips, Amsterdam, Netherlands) or a Somatom Definition AS 40 (Siemens Healthcare, Forchheim, Germany) with 512 × 512 matrix. The CT slice thickness was 5 mm for EBRT and 3 mm for BT, respectively. The clinical target volume (CTV) of EBRT was the gross tumor volume (GTV), the entire uterus and cervix, the lymph nodal region at risk (GTVnd), and part of the bladder and rectum. A margin of 5 mm was applied around the CTV and GTVnd to create the planning target volume (PTV) and planning GTVnd (PGTVnd). The PTV was prescribed with 45 Gy or 50 Gy, and the PGTVnd was boosted by 10 Gy to 15 Gy. Normal tissues, including the rectum, bladder, intestine, colon, sigmoid, pelvic bone, and right and left femur head, were also delineated for planning and dose evaluation. The EBRT VMAT plans were designed using Pinnacle v9.1–16.2 (Philips Radiation Oncology Systems, Fitchburg, WI, USA) and delivered using double full arcs with 6-MV X-rays. All plans were optimized to ensure that at least 95% of the target volumes received the prescription dose (PD) while minimizing doses to OARs. To manage the deformation caused by different organ filling, each patient was requested to empty their bladder and rectum, and drink 800 mL of water 40 min before undergoing treatment. Cone-beam CT was used to verify their anatomical situation.
The target volume and OARs for BT were delineated on the planning CT according to Groupe Européen de Curiethérapie (GEC)- European SocieTy for Radiotherapy & Oncology (ESTRO) recommendations, including the high-risk CTV (HR-CTV), rectum, bladder, sigmoid, and bowel. MRI at diagnosis acquired before the first BT fraction was served as a reference. All BT plans were designed using Oncentra Brachy v4.6 (Elekta Brachytherapy, Veneedal, The Netherlands) and delivered using tandem/ovoid (T/O) applicators or T/O applicators with interstitial needles. A Flexitron afterloader unit with an 192Ir source was used for the BT treatment. The activation step was 2 mm. The source dwell times for BT plans were optimized by inverse planning simulated annealing and then manually adjusted on the basis of OAR dose constraints. The fractional PD was prescribed to cover 90% of the HR-CTV and normalized to 6 Gy for a high-precision dose prediction.
2.2 Method description
The flowchart shown in Figure 1 illustrates the method of this study. It can be divided into three steps: dose accumulation, dose prediction, and plan redesign.
Step 1: Dose accumulation
To accumulate the dose distributions from EBRT and BT, all doses were converted into equivalent doses in 2 Gy fraction (EQD2) using the linear quadratic model with α/β = 10 Gy for tumors and α/β = 3 Gy for normal tissues. Due to limited conditions of our hospital, CT-guided BT was only performed for the first fraction. Thus, we could only magnify the first fractional BT dose four times to simulate the total BT dose. Commercially available DIR software MIM Maestro (MIM Software, Cleveland, OH, USA) was used to deform and sum the BT dose to the referenced EBRT dose to obtain the accumulated dose distribution. For a high DIR accuracy, we adopted a hybrid DIR method combining contour-based and intensity-based DIR. The contours used for DIR were the bladder, rectum, and the whole uterus and vagina (U + V). The purpose of delineating the U + V is to include the BT applicator and packing material. The CT numbers of these contours were, respectively, overridden to a certain value to ensure consistent intensities in both images. For the purpose of dose prediction, all accumulated doses were interpolated into the same pixel size with corresponding EBRT CT images.
Step 2: Dose prediction
The pixel-wise dose distribution prediction used a ResNet-101–based deep learning model, which has been introduced in our previous study (39). In this study, we also used combined anatomic maps as three-channel inputs, including the planning CT images of EBRT, corresponding structure maps, and distance maps. In the structure maps, the voxels in each target and OAR were assigned with a unique label. For voxels in the overlap, their label values were summed to ensure uniqueness. The voxels in the rest of the body and out of the body were labeled as 1 and 0, respectively. To improve dose prediction accuracy, we introduced a distance map as an input for the model. It was defined as the minimum distance from the PTV surface to each voxel of normal tissue outside the PTV, called the distance-to-target volume (DTT). In each slice of the DTT map, each voxel corresponded to a label value of DTT, and voxels inside the PTV and outside the body were labeled as 0. The outputs for the deep learning model were the corresponding accumulated dose maps. Due to the limited number of available datasets, a fivefold cross-validation was used to test prediction model performance. The prediction performance was quantitatively evaluated by (1) the voxel-wise mean absolute error (MAE) and (2) the dice similarity coefficient (DSC) of isodose volumes. Isodoses from 10 Gy to 160 Gy were evaluated with a 10-Gy interval.
Step 3: Plan redesign
The predicted accumulated dose distribution was used as a reference to redesign EBRT VMAT plans. As the BT dose is distributed within the cervix, the accumulated dose region is contained in the EBRT target. In terms of the region outside the EBRT target, the result of accumulated dose prediction would be similar to that of pure EBRT dose prediction. Therefore, the dosimetric parameters obtained from the predicted accumulated dose distribution could be used as optimization objectives for most normal tissues. However, as the bladder and rectum are partly included in the EBRT target, they are usually close to the high-dose region. As shown in Figure 2, the overlaps between the predicted isodose volume of 70 Gy and the bladder/rectum were created as a contour. For the redesigned plans, we minimized the dosimetric hotspots from occurring in this contour.
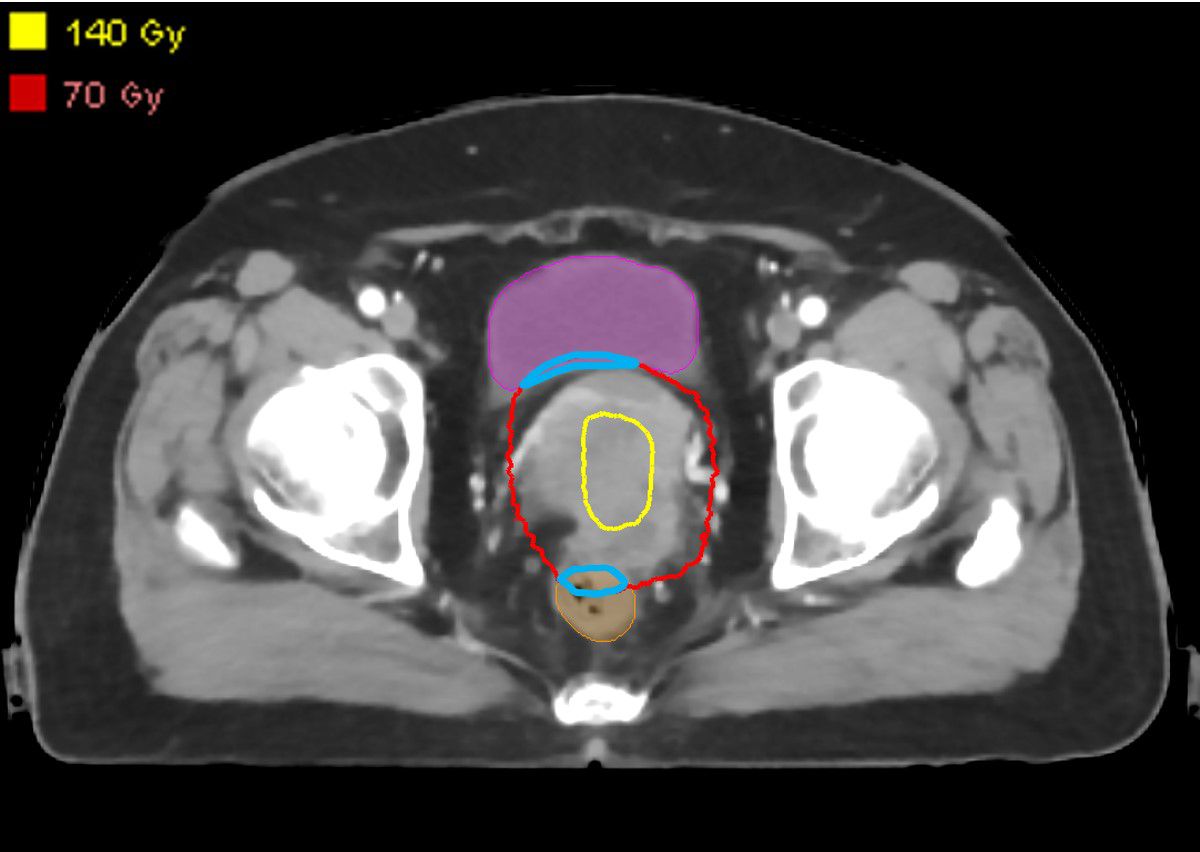
Figure 2 Overlaps (blue contours) between the predicted isodose volume of 70 Gy and the bladder/rectum.
2.3 Clinical validation
To test the role of the predicted accumulated dose in clinic, one physicist designed an EBRT VMAT plan using the conventional method without knowing the predicted dose and then redesigned a new EBRT VMAT plan using the proposed method. The two resulting EBRT dose distributions were separately accumulated with the BT dose for comparison. Critical dosimetric parameters of the bladder and rectum (V50, V60, and D2cc) were recorded. Normal tissue complication probability (NTCP) values were calculated using the Lyman–Kutcher–Burman model. The estimated parameter values for the rectum and bladder were D50 = 80 Gy, m = 0.15, and n = 0.12 and D50 = 80 Gy, m = 0.11, and n = 0.50, respectively (40). The statistical significance of the results was proven with a Wilcoxon signed-rank test at 5% level significance.
3 Results
3.1 Dose prediction performance
The mean MAE between predicted and actual dose distributions were as follows: 3.73 ± 1.58 Gy for the whole body, 3.27 ± 1.06 Gy for the whole body without PTV, 6.55 ± 3.10 Gy for the bladder, 8.05 ± 4.95 Gy for the rectum, 4.10 ± 1.88 Gy for the intestine, 3.67 ± 2.01 Gy for the colon, 5.06 ± 3.10 Gy for the sigmoid, 3.48 ± 1.28 Gy for the pelvic bone, 2.71 ± 1.52 Gy for the femur head, 4.96 ± 3.32 Gy for the cord, and 2.75 ± 1.56 Gy for the kidney. Because the bladder, rectum, and sigmoid were close to the high-dose region, their mean MAE was larger than that of other OARs. Table 1 presents the results of the DSC of isodose volumes between predicted and actual dose distributions. For an isodose less than or equal to the PD of EBRT, the mean DSC was high, ranging from 0.87 to 0.92, whereas the mean DSC of isodose volumes higher than the PD of EBRT was low and decreased with increasing isodose, ranging from 0.73 to 0.49. For the isodose volume of the summed PD of EBRT and BT (76.25/82 GyEQD2), the mean DSC was approximately 0.7. Compared to the actual dose distribution, the predicted dose distribution exhibits characteristics of a more homogeneous dose in the target, lower dose gradient, and indistinct boundaries between the target and normal tissues. Consequently, the predicted volumes for low isodoses were slightly larger, whereas those for high isodoses were smaller compared to the actual volumes. This observation is also evident in Figure 3 that illustrates a comparison between the predicted and actual DVHs for all OARs. Furthermore, the predicted and actual spatial locations of the high-dose regions of the bladder and rectum were similar. For the bladder, the high-dose region often occurs in the trigonum vesicae. In the rectum, it is often located near the upper cervix segment.
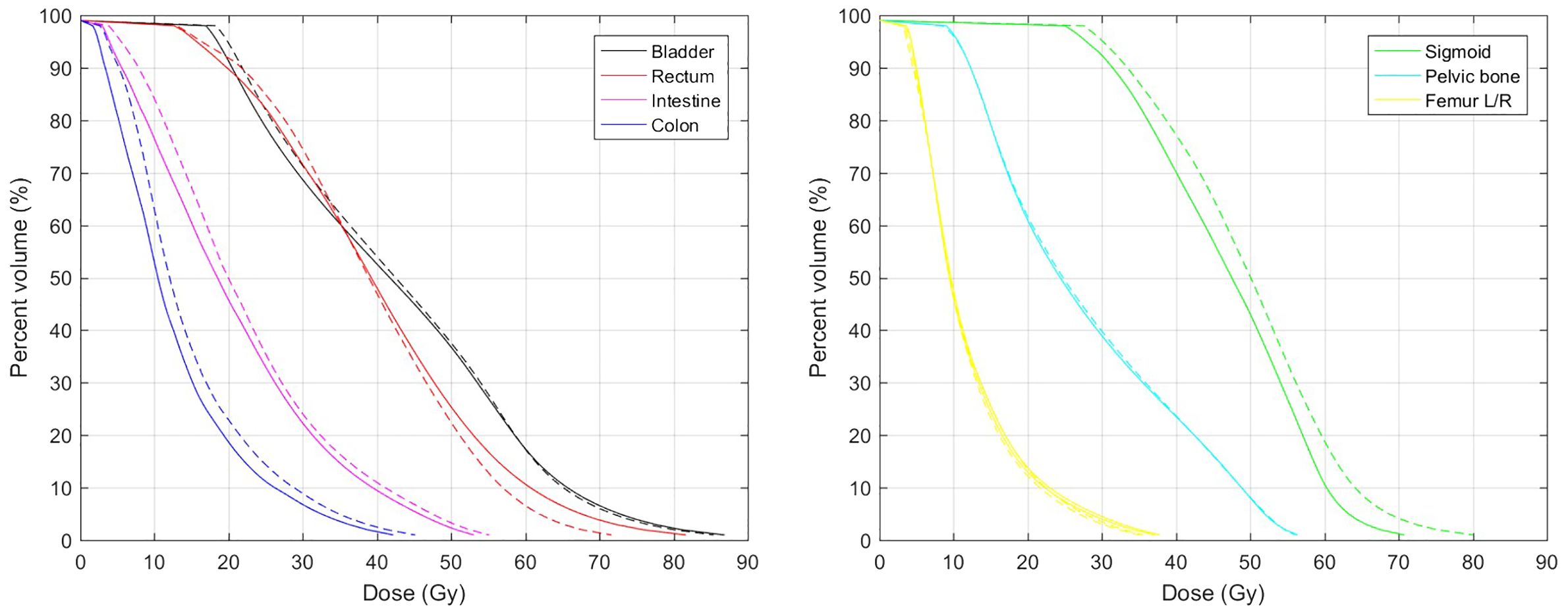
Figure 3 Mean DVHs of OARs. Solid and dashed lines represent actual and predicted DVHs, respectively.
3.2 Redesigned plan evaluation
Table 2 shows the dosimetric parameters of the bladder and rectum for the conventional and redesigned EBRT + BT. Compared with the conventional EBRT + BT, the mean V50 and V60 of the redesigned EBRT + BT for the bladder decreased by 3.02% and 1.71%, respectively, whereas those for the rectum decreased by 4.82% and 1.97%, respectively. All values showed significant differences (p < 0.001). The mean D2cc of the bladder and rectum for the redesigned EBRT + BT were also lower than those for the conventional EBRT + BT (−1.19 Gy and −4.13 Gy, respectively). The rectal D2cc showed statistical significance (p < 0.001). Figure 4 shows typical dose distributions and DVHs of the conventional and redesigned EBRT + BT for a single patient.
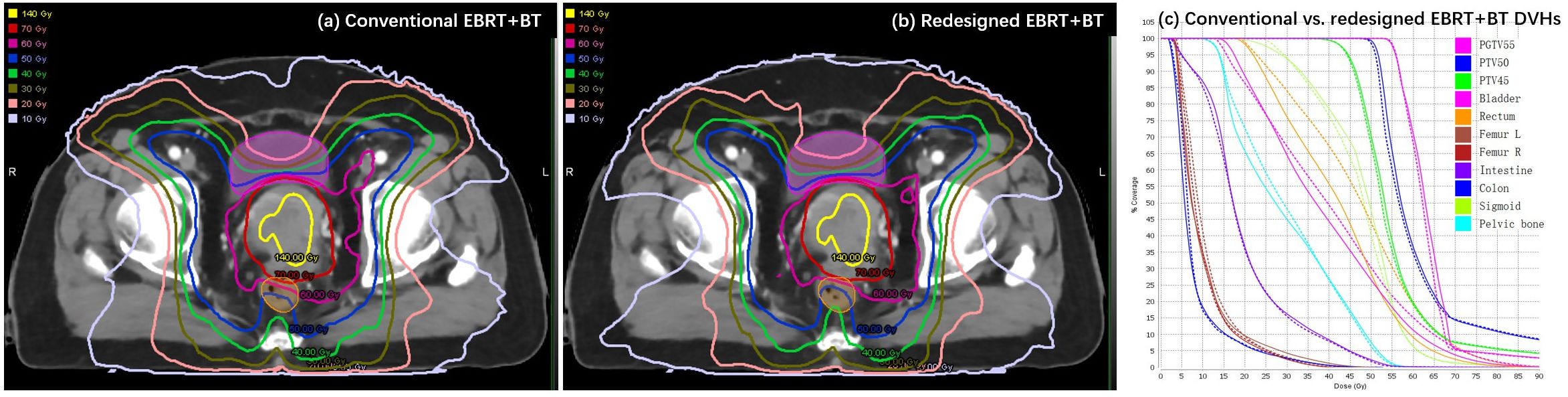
Figure 4 Example of (A, B) dose distributions and (C) DVHs for the conventional (dashed lines) and redesigned (solid lines) EBRT + BT.
3.3 NTCP results
When considering NTCP, the redesigned accumulated doses resulted in a decrease of NTCP for the bladder (0.04 ± 0.06‰ vs. 0.06 ± 0.09‰, P = 0.004) and rectum (1.21 ± 1.39% vs.2.19 ± 2.41%, P < 0.001). Figure 5 compares bladder and rectum NTCP values for conventional and redesigned EBRT + BT for each patient.
4 Discussion
To address dose optimization and evaluation difficulties associated with separate implementation of EBRT and BT, we predicted the accumulated dose distribution using a combination of DIR and deep learning techniques. High-precision DIR is particularly challenging in the pelvic region due to large and complex deformations caused by tumor shrinkage, different organ filling, bowel gas, and the presence of a BT applicator and vaginal packing. Thus, we adopted an advanced hybrid DIR method, which could minimize both the intensity differences between the two images and the differences between corresponding contour surfaces. Additionally, we delineated the U + V and used it as a registration contour. As an important anatomy in the whole pelvis, it could not only solve the issue of inconsistent intensity between the EBRT and BT images but also help to improve the DIR accuracy. After the DIR, the mean DSC for all contours reached 0.8–0.9, which satisfied the accuracy requirement recommended by AAPM TG-132 report (41).
Although dose prediction has been successfully realized in studies, the accumulated dose prediction faces new challenges. The input data for deep learning were all based on EBRT. However, the output data were accumulated in dose maps correlated with both EBRT and BT. This led to an uncertain location of the predicted accumulated dose region in the EBRT targets. Consequently, the DSC of the isodose volume for the summed PD was relatively low for our initial dose prediction. To solve this problem, we added the U + V to the structure maps. Although it is an extra delineated structure used for DIR, it could specify the location of the BT dose. With this structure, the DSC of the isodose volumes was increased by approximately 0.05 for the 100%, 150%, and 200% summed PD.
However, the accuracy of the accumulated dose prediction is still affected by many factors, including the DIR method, the number of training data, and the various types of applicators used in BT. It can be observed from Table 1 that there was a significant decrease in mean DSC when the isodose exceeded the PD of EBRT. This suggests that, while the current model performs well in predicting the EBRT dose part, improvements are required for accurately predicting the BT dose part. Enhancing accuracy in BT dose prediction may be achieved through adopting a more powerful DIR method, increasing the training data, and using a consistent BT applicator. Another limitation of this study is that we used the dose of the first BT fraction to represent the total BT dose due to the limited clinical data. This led to a deviation from the actual BT dose indeed. However, the treatment planning only needs the general scope of high-dose region as a reference, which is confirmed to be basically consistent among BT fractions (42) and could be provided by the first BT fraction. Moreover, multiple DIRs need to be performed if accumulating multiple BT doses. Our approach could avoid multiple errors caused by multiple DIR. The intra-fraction variations in the tumor and OARs were also not taken into account in this study. In fact, these variations will result in a deviation between the predicted dose and the actual dose (43, 44). The impact of these variations to different degrees on the actual effectiveness of the dose prediction model needs to be specifically studied in the future.
Treatment planning is a complex process with a large amount of optimization parameters to adjust based on the skill and experience of the planner. In recent years, KBP is increasingly used to improve plan quality and consistency. In previous literatures, the dose predictions used for KBP mainly focused on EBRT or BT alone (19–32). Li et al. applied a commercial KBP system to control EBRT plan quality in a clinical trial. They reported that the mean NTCP for gastrointestinal toxicity was lower for KBP plans compared to validation-set plans (48.7% vs. 53.8%, P < 0.001). Chen et al. used CNN to predict a patient-specific set of IMRT objectives based on overlap volume histograms (OVHs). They showed that the V40 of the bladder and rectum decreased by 6.3% and 12.3% compared to that of manual plans. In addition to EBRT, a research group applied knowledge-based dose prediction to BT (19, 24, 25). Their latest results showed that the differences between actual and predicted D2cc were −0.17± 0.67 Gy, −0.04 ± 0.46 Gy, and 0.00 ± 0.44 Gy for the bladder, rectum, and sigmoid, respectively. Reijtenbagh et al. also used machine learning models to predict D2cc to OARs using OVHs for identifying plans that may require further optimization. The models achieved mean squared errors ranging from 0.13 Gy to 0.40 Gy (31). Furthermore, dose prediction models have also been used to assess intra-fractional dose variations in OARs (27). To our knowledge, the accumulated dose of EBRT and BT is currently used only for predicting toxicity prediction and not for KBP (45, 46). However, for this combined radiotherapy, dose prediction for EBRT alone is not sufficient to guide EBRT treatment planning and BT doses should be taken into account to minimize the overall risk of toxicity. However, the problem is that EBRT is usually implemented before BT and the BT dose is unknown. This study realized accumulated dose prediction without knowing the BT dose, thereby allowing the BT dose to be taken into account in EBRT treatment planning. With the predicted dose, planners could set optimization objectives more appropriately to improve the consistency of plan quality. More importantly, the prediction could indicate an overlap between the high-dose region and OARs. This could help planners further decrease the EBRT dose to OARs, leave more OAR dosimetric space for BT, lower the overall OAR dose, and achieve an optimal accumulated dose distribution. For the redesigned EBRT plans, we minimized the hotspots occurred in the overlaps between the predicted high-dose region and the bladder/rectum. The results verified the redesigned EBRT + BT had lower total doses to the bladder and rectum. However, this study did not consider the sigmoid and bowel as they are too complex to be accurately registered. Furthermore, we only used the predicted accumulated dose for the EBRT planning. Ideally, it can be helpful for both EBRT and BT planning. This may be our next research direction.
Accumulated dose prediction could also be helpful to ROs. In current clinical practice, the actual accumulated dose for combined radiotherapy is not entirely clear or visible. Usually, tumor dosages are determined on the basis of the guidelines and combined with personal experience. Total OAR doses are evaluated on the worse case assumption. Accumulated dose prediction provides a final dose distribution before treatment initiation. It could help ROs, especially young and inexperienced ROs, in making treatment decisions, delineating targets, guiding the insertion of the BT applicator, and predicting the risk of toxicity. This effect could be further explored in future work.
5 Conclusion
In this study, we realized accumulated dose prediction for combined cervical cancer radiotherapy without knowing the BT dose. EBRT treatment planning referencing the predicted accumulated dose distribution could improve accumulated dose distribution and decrease OAR NTCP values. The predicted dose could also help ROs estimate the total doses to OARs and make better treatment decisions.
Data availability statement
The raw data supporting the conclusions of this article will be made available by the authors, without undue reservation.
Ethics statement
The studies involving humans were approved by the Ethics Committee of National Cancer Center/Cancer Hospital, Chinese Academy of Medial Sciences and Peking Union Medical College (protocol code 2023020809270002 and 8 February 2023). The studies were conducted in accordance with the local legislation and institutional requirements. Written informed consent for participation was not required from the participants or the participants’ legal guardians/next of kin in accordance with the national legislation and institutional requirements.
Author contributions
QF: Writing – original draft, Writing – review & editing, Conceptualization, Investigation, Methodology, Project administration, Data curation, Formal analysis, Visualization. XC: Conceptualization, Data curation, Investigation, Methodology, Software, Writing – original draft, Formal analysis, Resources. YL: Writing – original draft, Formal analysis, Investigation, Resources, Software. JZ: Writing – original draft, Data curation, Formal analysis, Investigation. YX: Writing – original draft, Methodology, Supervision, Validation. XY: Data curation, Supervision, Validation, Writing – original draft. MH: Data curation, Supervision, Validation, Writing – original draft. KM: Conceptualization, Funding acquisition, Project administration, Supervision, Writing – original draft. JD: Project administration, Supervision, Writing – original draft.
Funding
The author(s) declare financial support was received for the research, authorship, and/or publication of this article. This work was supported by the National Natural Science Foundation of China (12175312, 11975313, and 12005302), CAMS Innovation Fund for Medical Sciences (2020-I2M-C&T-B-073 and 2021-I2M-C&T-A-016), the Beijing Nova Program (Z201100006820058), and Beijing Hope Run Special Fund of Cancer Foundation of China (LC2021A15).
Conflict of interest
The authors declare that the research was conducted in the absence of any commercial or financial relationships that could be construed as a potential conflict of interest.
Publisher’s note
All claims expressed in this article are solely those of the authors and do not necessarily represent those of their affiliated organizations, or those of the publisher, the editors and the reviewers. Any product that may be evaluated in this article, or claim that may be made by its manufacturer, is not guaranteed or endorsed by the publisher.
References
1. Zhen X, Chen J, Zhong Z, Hrycushko B, Zhou L, Jiang S, et al. Deep convolutional neural network with transfer learning for rectum toxicity prediction in cervical cancer radiotherapy: A feasibility study. Phys Med Biol. (2017) 62:8246. doi: 10.1088/1361-6560/aa8d09
2. Men K, Chen X, Yang B, Zhu J, Yi J, Wang S, et al. Automatic segmentation of three clinical target volumes in radiotherapy using lifelong learning. Radiother Oncol. (2021) 157:1–7. doi: 10.1016/j.radonc.2020.12.034
3. Orlando N, Gillies DJ, Gyacskov I, Romagnoli C, D’Souza D, Fenster A. Automatic prostate segmentation using deep learning on clinically diverse 3D transrectal ultrasound images. Med Phys. (2020) 47:2413–26. doi: 10.1002/mp.14134
4. Wang F, Xing L, Bagshaw H, Buyyounouski M, Han B. Deep learning applications in automatic needle segmentation in ultrasound guided prostate brachytherapy. Med Phys. (2020) 47:3797–805. doi: 10.1002/mp.14328
5. Han X. MR-based synthetic CT generation using a deep convolutional neural network method. Med physics. (2017) 44:1408–19. doi: 10.1002/mp.12155
6. Tomori S, Kadoya N, Takayama Y, Kajikawa T, Shima K, Narazaki K, et al. A deep learning-based prediction model for gamma evaluation in patient-specific quality assurance. Med Phys. (2018) 45:4055–65. doi: 10.1002/mp.13112
7. Mardani M, Dong P, Xing L. Deep-learning based prediction of achievable dose for personalizing inverse treatment planning. Int J Radiat Oncol Biol Phys. (2016) 96:E419–20. doi: 10.1016/j.ijrobp.2016.06.1685
8. Kearney V, Chan JW, Haaf S, Descovich M, Solberg TD. Dose Net: a volumetric dose prediction algorithm using 3D fully convolutional neural networks. Phys Med Biol. (2018) 63:235022. doi: 10.1088/1361-6560/aaef74
9. Fan J, Wang J, Chen Z, Hu C, Zhang Z, Hu W. Automatic treatment planning based on three-dimensional dose distribution predicted from deep learning technique. Med Phys. (2019) 46:370–81. doi: 10.1002/mp.13271
10. Mao X, Pineau J, Keyes R, Enger SA. RapidBrachyDL: rapid radiation dose calculations in brachytherapy via deep learning. Int J Radiat Oncol Biol Phys. (2020) 108:802–12. doi: 10.1016/j.ijrobp.2020.04.045
11. Akhavanallaf A, Mohammadi R, Shiri I, Salimi Y, Arabi H, Zaidi H. Personalized brachytherapy dose reconstruction using deep learning. Comput Biol Med. (2021) 136:104755. doi: 10.1016/j.compbiomed.2021.104755
12. Ma M, Kidd E, Fahimian BP, Han B, Niedermayr TR, Hristov D, et al. Dose prediction for cervical cancer brachytherapy using 3-D deep convolutional neural network. IEEE Trans Radiat Plasma Med Sci. (2021) 6:214–21. doi: 10.1016/10.1109/TRPMS.2021.3098507
13. Mcintosh C, Welch M, Mcniven A, Jaffray DA, Purdie TG. Fully automated treatment planning for head and neck radiotherapy using a voxel-based dose prediction and dose mimicking method. Phys Med Biol. (2017) 62:5926–44. doi: 10.1088/1361-6560/aa71f8
14. Good D, Lo J, Lee WR, Wu QJ, Yin FF, Das SK. A knowledge-based approach to improving and homogenizing intensity modulated radiation therapy planning quality among treatment centers: an example application to prostate cancer planning. Int J Radiat Oncol Biol Phys. (2013) 87:176–81. doi: 10.1016/j.ijrobp.2013.03.015
15. Moore KL, Brame RS, Low DA, Mutic S. Experience-based quality control of clinical intensity-modulated radiotherapy planning. Int J Radiat Oncol Biol Phys. (2011) 81:545–51. doi: 10.1016/j.ijrobp.2010.11.030
16. Ge Y, Wu QJ. Knowledge-based planning for intensity-modulated radiation therapy: a review of data-driven approaches. Med Phys. (2019) 46:2760–75. doi: 10.1002/mp.13526
17. Appenzoller LM, Michalski JM, Thorstad WL, Mutic S, Moore KL. Predicting dose volume histograms for organs-at-risk in IMRT planning. Med Phys. (2012) 39:7446–61. doi: 10.1118/1.4761864
18. Shiraishi S, Tan J, Olsen LA, Moore KL. Knowledge-based prediction of plan quality metrics in intracranial stereotactic radiosurgery: Knowledge-based prediction of plan quality metrics in SRS. Med Phys. (2015) 42:908–17. doi: 10.1118/1.4906183
19. Yusufaly TI, Meyers SM, Mell LK, Moore KL. Knowledge-based planning for intact cervical cancer. Semin Radiat Oncol. (2020) 30:328–39. doi: 10.1016/j.semradonc.2020.05.009
20. Li N, Carmona R, Sirak I, Kasaova L, Followill D, Michalski J, et al. Highly efficient training, refinement, and validation of a knowledge-based planning quality-control system for radiation therapy clinical trials. Int J Radiat Oncol Biol Phys. (2017) 97:164–72. doi: 10.1016/j.ijrobp.2016.10.005
21. Jihong C, Penggang B, Xiuchun Z, Chen K, Chen W, Dai Y, et al. Automated intensity modulated radiation therapy treatment planning for cervical cancer based on convolution neural network. Technol Cancer Res Treat. (2020) 19:1–8. doi: 10.1177/1533033820957002
22. Tang H, Chen Y, Jiang J, Li K, Zeng J, Hu Z, et al. Dose prediction models based on geometric and plan optimization parameter for adjuvant radiotherapy planning design in cervical cancer radiotherapy. J Healthcare Eng. (2021) 2021. doi: 10.1155/2021/7026098
23. Shen C, Gonzalez Y, Klages P, Qin N, Jung H, Chen L, et al. Intelligent inverse treatment planning via deep reinforcement learning, a proof-of-principle study in high dose-rate brachytherapy for cervical cancer. Phys Med Biol. (2019) 64:115013. doi: 10.1088/1361-6560/ab18bf
24. Yusufaly TI, Kallis K, Simon A, Mayadev J, Yashar CM, Einck JP, et al. A knowledge-based organ dose prediction tool for brachytherapy treatment planning of patients with cervical cancer. Brachytherapy. (2020) 19:624–34. doi: 10.1016/j.brachy.2020.04.008
25. Cortes KG, Kallis K, Simon A, Mayadev J, Meyers SM, Moore KL. Knowledge-based three-dimensional dose prediction for tandem-and-ovoid brachytherapy. Brachytherapy. (2022) 21:532–42. doi: 10.1016/j.brachy.2022.03.002
26. Pu G, Jiang S, Yang Z, Hu Y, Liu Z. Deep reinforcement learning for treatment planning in high-dose-rate cervical brachytherapy. Phys Med. (2022) 94:1–7. doi: 10.1016/j.ejmp.2021.12.009
27. Jaberi R, Siavashpour Z, Aghamiri MR, Kirisits C, Ghaderi R. Artificial neural network based gynaecological image-guided adaptive brachytherapy treatment planning correction of intra-fractional organs at risk dose variation. J Contemp Brachyther. (2017) 9:508–18. doi: 10.5114/jcb.2017.72567
28. Akhavanallaf A, Mohammadi R, Shiri I, Salimi Y, Arabi H, Zaidi H. Personalized brachytherapy dose reconstruction using deep learning. Comput Biol Med. (2021) 136:104755. doi: 10.1016/j.compbiomed.2021.104755
29. Mao X, Pineau J, Keyes R, Enger SA. RapidBrachyDL: Rapid radiation dose calculations in brachytherapy via deep learning. Int J Radiat Oncol Biol Phys. (2020) 108:802–12. doi: 10.1016/j.ijrobp.2020.04.045
30. Villa M, Bert J, Valeri A, Schick U, Visvikis D. Fast Monte Carlo-based inverse planning for prostate brachytherapy by using deep learning. IEEE Trans Radiat Plasma Med Sci. (2022) 6:182–8. doi: 10.1109/TRPMS.2021.3060191
31. Reijtenbagh D, Godart J, de Leeuw A, Seppenwoolde Y, Jürgenliemk-Schulz I, Mens JW, et al. Multi-center analysis of machine-learning predicted dose parameters in brachytherapy for cervical cancer. Radiother Oncol. (2022) 170:169–75. doi: 10.1016/j.radonc.2022.02.022
32. Li Z, Yang Z, Lu J, Zhu Q, Wang Y, Zhao M, et al. Deep learning-based dose map prediction for high-dose-rate brachytherapy. Phys Med Biol. (2023) 68:175015. doi: 10.1088/1361-6560/acecd2
33. Kim H, Lee YC, Benedict SH, Dyer B, Price M, Rong Y, et al. Dose summation strategies for external beam radiation therapy and brachytherapy in gynecologic Malignancy: A review from the NRG oncology and NCTN medical physics subcommittees. Int J Radiat Oncol Biol Phys. (2021) 111:999–1010. doi: 10.1016/j.ijrobp.2021.06.019
34. Swamidas J, Kirisits C, Brabandere MD, Hellebust TP, Siebert FA, Tanderup K. Image registration, contour propagation and dose accumulation of external beam and brachytherapy in gynecological radiotherapy. Radiother Oncol. (2019) 143:1–11. doi: 10.1016/j.radonc.2019.08.023
35. Abe T, Tamaki T, Makino S, Ebara T, Hirai R, Miyaura K, et al. Assessing cumulative dose distributions in combined radiotherapy for cervical cancer using deformable image registration with pre-imaging preparations. Radiat Oncol. (2014) 20:293. doi: 10.1186/s13014-014-0293-4
36. Teo BK, Bonner Millar LP, Ding X, Lin LL. Assessment of cumulative external beam and intracavitary brachytherapy organ doses in gynecologic cancers using deformable dose summation. Radiother Oncol. (2015) 115:195–202. doi: 10.1016/j.radonc.2015.04.002
37. Heerden LE, Houweling AC, Koedooder K, Kesteren Z, Wieringen N, Rasch CRN, et al. Structure-based deformable image registration: added value for dose accumulation of external beam radiotherapy and brachytherapy in cervical cancer. Radiother Oncol. (2017) 123:319–24. doi: 10.1016/j.radonc.2017.03.015
38. Chapman CH, Polan D, Vineberg K, Jolly S, Maturen KE, Brock KK, et al. Deformable image registration based contour propagation yields clinically acceptable plans for MRI based cervical cancer brachytherapy planning. Brachytherapy. (2018) 17:360–7. doi: 10.1016/j.brachy.2017.11.019
39. Chen X, Men K, Li Y, Yi J, Dai J. A feasibility study on an automated method to generate patient-specific dose distributions for radiotherapy using deep learning. Med Phys. (2019) 46:56–64. doi: 10.1002/mp.13262
40. Burman C, Kutcher GJ, Emami B, Goitein M. Fitting of normal tissue tolerance to an analytic function. Int J Radiat Oncol Biol Phys. (1991) 21:123–35. doi: 10.1016/0360-3016(91)90172-Z
41. Brock KK, Mutic S, McNutt TR, Li H, Kessler ML. Use of image registration and fusion algorithms and techniques in radiotherapy: report of the AAPM radiation therapy committee task group no. 132. Med Phys. (2017) 44:e43–76. doi: 10.1002/mp.2017.44.issue-7
42. Jamema SV, Mahantshetty U, Tanderup K, Malvankar D, Sharma S, Engineer R, et al. Inter-application variation of dose and spatial location of D(2cm(3)) volumes of OARs during MR image based cervix brachytherapy. Radiother. Oncol. (2013) 107:58–62. doi: 10.1016/j.radonc.2013.01.011
43. Simha V, Patel FD, Sharma SC, Rai B, Oinam AS, Krishnatry R, et al. Evaluation of intrafraction motion of the organs at risk in image-based brachytherapy of cervical cancer. Brachytherapy. (2014) 13:562–7. doi: 10.1016/j.brachy.2014.05.016
44. Nomden CN, de Leeuw AA, Roesink JM, Tersteeg RJHA, Westerveld H, Jürgenliemk-Schulz IM. Intra-fraction uncertainties of MRI guided brachytherapy in patients with cervical cancer. Radiother Oncol. (2014) 112:217–20. doi: 10.1016/j.radonc.2014.08.010
45. Zhen X, Chen J, Zhong Z, Hrycushko B, Zhou L, Jiang S, et al. Deep convolutional neural network with transfer learning for rectum toxicity prediction in cervical cancer radiotherapy: a feasibility study. Phys Med Biol. (2017) 62:8246–63. doi: 10.1088/1361-6560/aa8d09
Keywords: cervical cancer, combined radiotherapy, accumulated dose, deep learning, NTCP
Citation: Fu Q, Chen X, Liu Y, Zhang J, Xu Y, Yang X, Huang M, Men K and Dai J (2024) Improvement of accumulated dose distribution in combined cervical cancer radiotherapy with deep learning–based dose prediction. Front. Oncol. 14:1407016. doi: 10.3389/fonc.2024.1407016
Received: 26 March 2024; Accepted: 17 June 2024;
Published: 08 July 2024.
Edited by:
Gene A. Cardarelli, Brown University, United StatesReviewed by:
Ruijie Yang, Peking University Third Hospital, ChinaEric Carver, Brown University, United States
Copyright © 2024 Fu, Chen, Liu, Zhang, Xu, Yang, Huang, Men and Dai. This is an open-access article distributed under the terms of the Creative Commons Attribution License (CC BY). The use, distribution or reproduction in other forums is permitted, provided the original author(s) and the copyright owner(s) are credited and that the original publication in this journal is cited, in accordance with accepted academic practice. No use, distribution or reproduction is permitted which does not comply with these terms.
*Correspondence: Kuo Men, bWVua3VvMTI2QDEyNi5jb20=
†These authors have contributed equally to this work and share first authorship