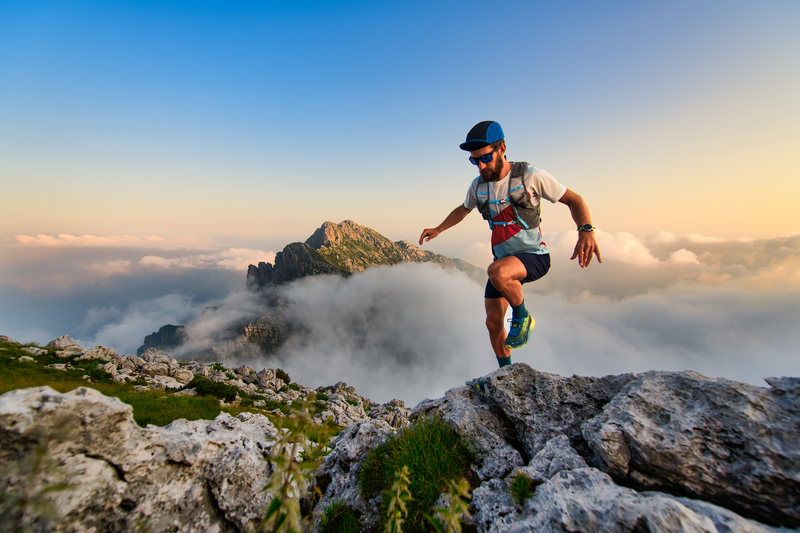
95% of researchers rate our articles as excellent or good
Learn more about the work of our research integrity team to safeguard the quality of each article we publish.
Find out more
ORIGINAL RESEARCH article
Front. Oncol. , 08 May 2024
Sec. Gynecological Oncology
Volume 14 - 2024 | https://doi.org/10.3389/fonc.2024.1397454
Purpose: To facilitate patient consultation and assist in clinical decision-making, we developed a predictive model to analyze the overall survival (OS) rate of cervical cancer patients with concurrent lung metastasis for 6 months, 1 year, or 2 years.
Methods: We extracted data on patients diagnosed with cervical cancer and concurrent lung metastasis between 2010 and 2020 from the Surveillance, Epidemiology, and End Results (SEER) database. Through a random assignment process, these patients were allocated to either a training cohort or a validation cohort, maintaining a 7:3 ratio. Utilizing both univariate and multivariate Cox regression analyses, we determined the independent prognostic factors influencing OS. To enhance predictive accuracy, we developed a nomogram model incorporating these identified independent prognostic variables. Model effectiveness was subsequently assessed using various metrics, including receiver operating characteristic (ROC) curves, calibration plots, and decision curve analysis (DCA).
Results: We gathered data on 1330 patients diagnosed with cervical cancer with lung metastases. An OS nomogram was developed, accounting for factors such as histological type, presence of metastases in other organs (brain, liver), surgical interventions, radiation therapy, and chemotherapy. The ROC curves, calibration plots, and DCA curves demonstrated the commendable predictive performance of the nomogram in assessing the prognosis of cervical cancer patients with lung metastases in both the training and validation cohorts.
Conclusion: By utilizing clinical data from the SEER database, we have effectively devised a nomogram capable of predicting the 6-month, 1-year, and 2-year survival rates of cervical cancer patients with lung metastases. The nomogram boasts high accuracy, offering precise prognostic predictions. Its implementation can guide the formulation of individualized follow-up and treatment plans for enhanced patient care.
Cervical cancer is the most prevalent malignant neoplasm of the female reproductive system. According to Globle Cancer Statistics 2020, there were 604,127 new cases of cervical cancer globally, resulting in 341831 deaths. Both the incidence and mortality rates rank fourth among malignant tumors affecting women (1). According to the latest statistics, the incidence and mortality of cervical cancer have been steadily increasing, particularly among young women in China (2). Upon initial diagnosis, approximately 13% of patients present with tumors that have already metastasized to nearby or distant organs. Research indicates that the 5-year survival rate for cervical cancer patients without metastasis is 91.5%. However, at the onset of metastasis, the 5-year survival rate decreases to 16.5%. Notably, the occurrence rate of cervical cancer with lung metastasis ranges from 2.2% to 9.1%, with patients exhibiting concomitant lung metastasis experiencing significantly decreased survival rates (3, 4). Hence, a comprehensive examination of the pathological features and prognostic factors associated with lung metastasis in cervical cancer patients represents a pivotal concern within the realm of clinical treatment.
Currently, research on patients who are diagnosed with cervical cancer lung metastasis at the time of initial diagnosis, both domestically and internationally, is predominantly characterized by small sample studies or individual case reports. There is limited exploration of factors influencing the survival of these patients, and the commonly employed TNM staging system has limitations in accurately discerning individual survival disparities. Moreover, among patients classified under the same stage, survival rates demonstrate heterogeneity. Therefore, the predictive capacity of the FIGO staging system, commonly used for prognostication, is not comprehensive and demonstrates a need for improved accuracy (5, 6). Consequently, the construction of an accurate and effective prognostic model for cervical cancer patients with lung metastasis holds paramount clinical importance. Nomograms, as tools for assessing disease risk and prognosis, have gained widespread application in clinical practice. Nomograms streamline a multitude of intricate factors into a unified numerical model, facilitating the prediction of event probabilities. In recent years, miRNAs have emerged as crucial tools in the predictive analysis and management of cancer. Thus, utilizing large and reliable datasets from the Surveillance, Epidemiology, and End Results (SEER) database, establishing a nomogram to predict the overall survival (OS) of patients with cervical cancer and lung metastasis and evaluating its predictive accuracy will contribute to guiding clinical treatment decisions and prognostic assessments.
We sourced data from the SEER database, a National Cancer Institute-supported repository, utilizing SEER Stat software (version 8.4.3; Incidence—SEER Research Data, 17 Registries, Nov 2022 Sub (2020–2022 varying)) (http://www.seer.cancer.gov/seerstat). The SEER database, which has been regularly updated since 1973, encompasses cancer diagnosis and survival information for approximately 30% of the U.S. population. Access to all SEER data is freely available with publicly accessible ethics approval.
To identify patients with malignant cervical cancer, we applied specific criteria, including the primary site labelled C53.0-C53.1 and C53.8-C53.9 according to the Site and Morphology Primary Site, the behavior recoding for analysis labelled ‘Malignant,’ and the extent of disease SEER combined sets at DX-lung labelled ‘Yes.’ Given that information on distant metastasis sites was first collected in the SEER database in 2010, we restricted the year of diagnosis to the period between 2010 and 2020. The selection criteria and research process are illustrated in Figure 1.
The variables extracted from the SEER database included diverse factors, including the year of diagnosis, age at diagnosis (categorized as ≤58 and >58 years), race (classified as white, black, and others, incorporating American Indian/Alaskan Native, Asian or Pacific Islander), marital status (grouped into married, single, which includes divorced, separated, widowed, never married or domestic partner, and unknown), primary site (endocervix, exocervix, overlapping lesion of cervix uteri, and cervix uteri), histology (such as squamous cell carcinoma, adenocarcinoma, and others), grade (Grade I, Grade II, Grade III/IV, and unknown), T stage (T1, T2, T3, T4, and Tx), N stage (N0, the N1, and Nx), metastatic site (including the bone, brain, and liver), and treatment method, involving chemotherapy, radiation, and surgery of the primary tumor. Additionally, survival months and vital signs were considered. For analytical purposes, we utilized X-tile bioinformatics software (Yale University, USA, Version 3.6.1) to categorize patients according to age into two groups: ≤58 years and >58 years (Figure 2) (7).
Our main measure of interest was OS, defined as the period from the time of cervical cancer diagnosis to the time of the last follow-up or the time of death from any cause.
All patients included in the study were randomly allocated to training or validation cohorts at a ratio of 7:3. This randomization was achieved utilizing the “create Data Partition” function within the R “caret” package to ensure the even distribution of outcome events. The training cohort was utilized for the development of a nomogram, while the validation cohort served to validate the model. Categorical variables are presented as percentages and were compared using the Chi-square test. Survival curves were generated using the Kaplan–Meier method, and the log-rank test was applied for analysis. Univariate and multivariate Cox regressions were conducted to discern the significance of variables concerning OS. In the training cohort, the covariates incorporated into the multivariate Cox proportional hazards models were determined through a backwards stepwise method based on the smallest Akaike information criterion (AIC) value. This approach was intended to identify variables that contributed minimally to the loss of prognostic information (8, 9).
Nomograms predicting 0.5-, 1-, and 2-year OS were developed utilizing independent prognostic factors. The discriminatory capacity of the nomogram was evaluated using 0.5-, 1-, and 2-year time-dependent area under the curve (AUC) values. An AUC ranging from 0.5 to 1 indicates the discriminative ability of the nomogram—higher values suggest superior discrimination. An AUC exceeding 0.7 is indicative of excellent discriminative performance.
To gauge the precision of point estimates from the nomogram-predicted survival against actual survival, calibration curves were generated. The bootstrapping method, involving 500 resamples, was applied to produce calibration curves for validating the nomogram in both the training and validation cohorts. Additionally, the net benefit was computed through decision curve analysis (DCA), which provided insights into the ability of the nomogram to predict clinical outcomes (10).
This report follows the guidelines outlined in the Strengthening the Reporting of Observational Studies in Epidemiology (STROBE) network (11). All analyses and graphical representations were executed using R software version 4.3.2 (www.r-project.org). All tests were two-sided, and a significance level of P < 0.05 was considered indicative of statistical significance.
Between 2010 and 2020, a cohort of 1550 cervical cancer patients with lung metastasis from the SEER database was initially identified. Through a meticulous selection process, 1330 eligible patients were randomly assigned to either the training cohort (N=931) or the validation cohort (N=399). Throughout the study period, the overall follow-up duration ranged from 0 to 130 months, with a median follow-up time of 6 months. In both the training and validation cohorts, the follow-up times ranged from 0 to 130 months and 0 to 117 months, respectively, with a corresponding median follow-up time of 6 months. Following the cutoff date for follow-up, a total of 1113 patients died, and remarkably, only 49 patients (3.6%) experienced mortality unrelated to cervical cancer. The detailed baseline characteristics of the patients in both the training and validation cohorts are presented in Table 1.
The demographic and clinicopathologic profiles of the patient cohort revealed that a predominant proportion were white (71.3%), unmarried or single (60.7%), diagnosed with squamous cell carcinoma (66.2%), and exhibited Grade III/IV disease (43.7%). The cervix uteri was identified as the primary site in the majority of patients (86%). Among the 1330 patients, 25.1% presented with bone metastases, 4.6% with brain metastases, and 24.4% with liver metastases. Surgical interventions were conducted in 106 (8%) women; 51% of the patients underwent radiotherapy, and 65.4% received chemotherapy. Notably, there were no significant differences in demographics or clinicopathologic characteristics between the training and validation cohorts (all P > 0.05).
We conducted both univariate and multivariate Cox regression analyses within the training cohort to ascertain the prognostic importance of the variables under consideration. In the univariate analysis, factors such as age at diagnosis, primary site, histology, presence of lymph node metastases, receipt of surgical treatment, radiotherapy, chemotherapy, and the presence of brain, liver, and bone metastases were found to be associated with OS (P<0.05, as indicated in Table 2). These significant variables were subsequently included in the multivariate analysis. Multivariate analysis revealed that histology, surgical treatment, radiotherapy, chemotherapy, brain metastasis, and liver metastasis were independent prognostic factors for OS among cervical cancer patients with lung metastases (P<0.05, as shown in Table 2). Drawing from these six independent risk factors identified through multivariate analysis, a nomogram was constructed to predict the 0.5-, 1-, and 2-year OS (Figure 3).
Table 2 Univariate and multivariate analyses of the prognostic factors for OS in the training cohort.
Figure 3 Nomogram for predicting 0.5-, 1- and 2-year OS for patients with cervical cancer and lung metastases in the training cohort.
In the training cohort, the area under the curve (AUC) values for the time-dependent receiver operating characteristic (ROC) curves were 0.811, 0.764, and 0.746 for 0.5-, 1-, and 2-year overall survival OS, respectively. In the validation cohort, these AUC values were 0.728, 0.718, and 0.683, respectively (Figure 4). These findings indicate the consistent and robust discriminatory ability of our nomogram. The calibration plots, which assessed the concordance between the nomogram predictions and the actual observations for the 0.5-, 1-, and 2-year OS in both the training and validation cohorts, exhibited favorable consistency (Figure 5). Furthermore, the decision curves depicted improved clinical applicability for predicting the overall survival of cervical cancer patients with lung metastases (Figure 6).
Figure 4 (A) nomogram ROC curves to predict 0.5-, 1-, and 2-year OS in the training cohort; (B) nomogram ROC curves to predict 0.5-, 1-, and 2-year OS in the validation cohort.
Figure 5 (A–C) nomogram calibration plots to predict 0.5-, 1-, and 2-year OS in the training cohort; (D–F) nomogram calibration plots to predict 0.5-, 1-, and 2-year OS in the validation cohort.
Figure 6 (A) DCA for predicting 6-, 12-, and 24-month OS in the training cohort; (B) DCA for predicting 6-, 12-, and 24-month OS in the validation cohort. The pink horizontal line represents that all patients in the model die, and there is no clinical benefit. The light green line, blue line and purple line represent the survival of all patients corresponding to 6, 12, and 24 month respectively, and the curve between the 2 represents the benefit of decision-making.
Considering the long-term survival of patients with cancer metastasis, it is important to clarify the prognostic factors of patients and develop reliable survival prediction models. Compared with traditional prediction tools, nomograms, as visualization tools for predicting the risk of disease occurrence and prognosis assessment, have been applied to evaluate the prognosis of patients with distant metastasis of multiple cancers, and all have demonstrated good predictive value (5, 12–15). Currently, there is a notable absence of dependable models for predicting the survival outcomes of individuals diagnosed with cervical cancer and lung metastasis, both domestically and internationally. To address this gap, our study developed a nomogram leveraging data from the SEER database. The nomogram’s performance was rigorously assessed using metrics such as the ROC curve, area under the curve, calibration plot, and DCA. Our findings collectively demonstrate that our nomogram exhibits robust discriminatory ability and accurate predictive capabilities and is of practical value. The SEER database, managed by the National Cancer Institute (NCI), is a comprehensive epidemiological repository providing invaluable insights for research in the field. The SEER database contains extensive information on cancer patients across the United States, including patient demographic characteristics, tumor diagnosis, treatment, and survival data. Due to its large sample size and long-term tracking characteristics, the SEER database has important research value in the field of cancer research. Analysis using this database will have important advantages and credibility.
Studies on the prognostic factors of lung metastasis in cervical cancer patients are the focus of clinical research, and there are obvious differences in the research results. Pulmonary metastases are secondary diseases of hematogenous dissemination and usually present as single or multiple nodules (16, 17). With the strengthening of cancer patients’ medical awareness and the continuous improvement of hospital follow-up technology, very small metastases can be detected earlier, markedly improving the detection rate of lung metastasis. Because most cervical cancer patients with lung metastases usually do not experience symptoms associated with lung metastases, it is impossible to treat metastatic lesions in a timely manner. Cervical cancer patients with lung metastasis exhibit favorable outcomes under three specific conditions: 1) absence of metastasis to other organs beyond the lung, regardless of lymph node involvement; 2) presence of ipsilateral lung metastasis; and 3) ≤4 lung metastases (18).From the analysis it is evident that patients who received surgery or radiotherapy to the primary tumor had a better prognosis. This would suggest that patients who developed lung metastases after locoregional treatment have a better prognosis than those presenting with de novo metastases.
The influence of pathological type on the prognosis of cervical cancer patients is still controversial. Some researchers believe that the pathological type of adenocarcinoma is an independent risk factor for the prognosis of cervical cancer patients with lung metastasis and is also a risk factor for lung metastasis of cervical cancer (19). Studies have shown that histopathological type, age, isolated lung metastasis, tumor size, and lymph node metastasis are prognostic factors affecting survival after lung metastasis in patients with cervical cancer. Patients with 3 or 4 lung metastases had lower 5-year survival rates than those with 1 or 2 lung metastases (42.2% vs. 0%, P = 0.0003) (20). Cox multivariate regression analysis revealed that histology, surgical intervention, radiotherapy, chemotherapy, brain metastases, and liver metastases were independent risk factors influencing overall survival OS in cervical cancer patients with lung metastasis. This finding is also in general agreement with previous studies.
This study showed that the survival period of patients with cervical cancer with lung metastasis was relatively short, so the treatment of metastatic cervical cancer is a major clinical difficulty. The nomogram showed that chemotherapy is the best choice for stage IVB patients with lung metastasis of primary cervical cancer without surgical indications. This finding is consistent with those of previous studies. The rapid development of targeted therapy and immunotherapy has revolutionized the treatment of many cancers. There have also been breakthroughs in the development of targeted drugs, including antiangiogenic drugs, tyrosinase inhibitors, and epidermal growth factor receptor blockers. The National Comprehensive Cancer Network (NCCN) guidelines recommend bevacizumab combined with systemic chemotherapy as the standard treatment for patients with distant metastasis of cervical cancer (21). Clinical studies have demonstrated that cisplatin plus paclitaxel with bevacizumab or tolopotecan plus paclitaxel with bevacizumab is effective in treating stage cervical cancer patients and extends their survival (22, 23). Bevacizumab combined with chemotherapy improves the survival rate of patients with recurrent or metastatic cervical cancer and is listed as the first-line therapy for recurrent, metastatic cervical cancer (24, 25). With the increasing use of immune and targeted therapies for treating patients with cervical cancer with lung metastasis, incorporating relevant data into prognostic nomograms will become more accurate and useful.
Nonetheless, it is important to acknowledge certain limitations in this study. First, as the study was retrospective, there was potential for selection bias during the patient selection process. Additionally, due to the limited clinical information available in the SEER database, several valuable clinical factors were not considered in the analysis, such as the absence of tumor markers, HPV infection status, imaging data, and details regarding the treatment of pulmonary metastases, the details of the cycles and doses of chemotherapy could not be obtained. Furthermore, the database primarily comprised Caucasian patients, necessitating external validation and adjustment of the model in diverse populations to ensure its generalizability. Finally, external validation was not performed due to current constraints in experimental conditions. To mitigate this limitation, we adopted a 7:3 ratio for study population allocation, with 30% earmarked for internal validation. The robustness demonstrated by the internal validation results supports the reliability of the model.
Based on the SEER database, this study identified independent prognostic factors for patients with cervical cancer lung metastasis and successfully constructed a survival prediction model for such patients which has good accuracy and clinical application value. Future studies will continue to expand the sample size, include multicenter native patients for validation, and explore the inclusion of other potential predictors to further improve the prediction accuracy and generalizability of the model.
In summary, our study effectively devised a nomogram capable of predicting the 6-month, 1-year, and 2-year survival rates of cervical cancer patients with lung metastases utilizing clinical data from the SEER database. This nomogram, constructed during our research, demonstrates notable advantages, shows high accuracy and has substantial clinical application value.
The original contributions presented in the study are included in the article/supplementary material. Further inquiries can be directed to the corresponding author.
Ethical consent was waived due to the SEER database contains anonymous patient information. All data from the SEER database were open access.
LC: Writing – original draft. KZ: Writing – review & editing.
The author(s) declare that no financial support was received for the research, authorship, and/or publication of this article.
We would like to thank the National Cancer Institute for providing the Surveillance, Epidemiology, and End Results (SEER) database.
The authors declare that the research was conducted in the absence of any commercial or financial relationships that could be construed as a potential conflict of interest.
All claims expressed in this article are solely those of the authors and do not necessarily represent those of their affiliated organizations, or those of the publisher, the editors and the reviewers. Any product that may be evaluated in this article, or claim that may be made by its manufacturer, is not guaranteed or endorsed by the publisher.
OS, overall survival; SEER, Surveillance, Epidemiology, and End Results; ROC, receiver operating characteristic; DCA, decision curve analysis; FIGO, The International Federation of Gynecology and Obstetrics; AIC, Akaike information criterion; AUC, area under the curve; STROBE, Strengthening the Reporting of Observational Studies in Epidemiology; NCI, National Cancer Institute; NCCN, National Comprehensive Cancer Network.
1. Sung H, Ferlay J, Siegel RL, Laversanne M, Soerjomataram I, Jemal A, et al. Global cancer statistics 2020: GLOBOCAN estimates of incidence and mortality Worldwide for 36 cancers in 185 countries. CA Cancer J Clin. (2021) 71:209–49. doi: 10.3322/caac.21660
2. Han B, Zheng R, Zeng H, Wang S, Sun K, Chen R, et al. Cancer incidence and mortality in China, 2022. J Nati Cancer Cent. (2024) 4(1):47–53. doi: 10.1016/j.jncc.2024.01.006
3. Henglian L, Jiajun W, Caixia W, Gang L, Min X. Analysis of related risk factors of lung metastasis after laparoscopic radical hysterectomy of cervical cancer. Med (Baltimore). (2021) 100:e24480. doi: 10.1097/md.0000000000024480
4. Ki EY, Lee KH, Park JS, Hur SY. A clinicopathological review of pulmonary metastasis from uterine cervical cancer. Cancer Res Treat. (2016) 48:266–72. doi: 10.4143/crt.2014.206
5. Wan G, Gao F, Chen J, Li Y, Geng M, Sun L, et al. Nomogram prediction of individual prognosis of patients with hepatocellular carcinoma. BMC Cancer. (2017) 17:91. doi: 10.1186/s12885-017-3062-6
6. Wang C, Yang C, Wang W, Xia B, Li K, Sun F, et al. A prognostic nomogram for cervical cancer after surgery from SEER database. J Cancer. (2018) 9:3923–8. doi: 10.7150/jca.26220
7. Camp RL, Dolled-Filhart M, Rimm DL. X-tile: a new bio-informatics tool for biomarker assessment and outcome-based cut-point optimization. Clin Cancer Res. (2004) 10:7252–9. doi: 10.1158/1078-0432.ccr-04-0713
8. Posada D, Buckley TR. Model selection and model averaging in phylogenetics: advantages of akaike information criterion and bayesian approaches over likelihood ratio tests. Syst Biol. (2004) 53:793–808. doi: 10.1080/10635150490522304
9. Wagenmakers E-J, Farrell S. AIC model selection using akaike weights. Psychon Bull Rev. (2004) 11:192–6. doi: 10.3758/BF03206482
10. Fitzgerald M, Saville BR, Lewis RJ. Decision curve analysis. JAMA. (2015) 313:409–10. doi: 10.1001/jama.2015.37
11. von Elm E, Altman DG, Egger M, Pocock SJ, Gøtzsche PC, Vandenbroucke JP. Strengthening the reporting of observational studies in epidemiology (STROBE) statement: guidelines for reporting observational studies. BMJ. (2007) 335:806–8. doi: 10.1136/bmj.39335.541782.AD
12. Liang W, Zhang L, Jiang G, Wang Q, Liu L, Liu D, et al. Development and validation of a nomogram for predicting survival in patients with resected non-small-cell lung cancer. J Clin Oncol. (2015) 33:861–9. doi: 10.1200/jco.2014.56.6661
13. Wang Y, Li J, Xia Y, Gong R, Wang K, Yan Z, et al. Prognostic nomogram for intrahepatic cholangiocarcinoma after partial hepatectomy. J Clin Oncol. (2013) 31:1188–95. doi: 10.1200/jco.2012.41.5984
14. Zhang S, Wang X, Li Z, Wang W, Wang L. Score for the overall survival probability of patients with first-diagnosed distantly metastatic cervical cancer: a novel nomogram-based risk assessment system. Front Oncol. (2019) 9:1106. doi: 10.3389/fonc.2019.01106
15. Zhang L, Dong D, Li H, Tian J, Ouyang F, Mo X, et al. Development and validation of a magnetic resonance imaging-based model for the prediction of distant metastasis before initial treatment of nasopharyngeal carcinoma: a retrospective cohort study. EBioMedicine. (2019) 40:327–35. doi: 10.1016/j.ebiom.2019.01.013
16. Li H, Wu X, Cheng X. Advances in diagnosis and treatment of metastatic cervical cancer. J Gynecol Oncol. (2016) 27:e43. doi: 10.3802/jgo.2016.27.e43
17. Balachandran VP, Gonen M, Smith JJ, DeMatteo RP. Nomograms in oncology: more than meets the eye. Lancet Oncol. (2015) 16:e173–80. doi: 10.1016/s1470-2045(14)71116-7
18. Mukai Y, Koike I, Matsunaga T, Yokota NR, Takano S, Sugiura M, et al. Radiation therapy for uterine cervical cancer with lung metastases including oligometastases. In Vivo. (2019) 33:1677–84. doi: 10.21873/invivo.11655
19. Chen X, Chen L, Zhu H, Tao J. Risk factors and prognostic predictors for cervical cancer patients with lung metastasis. J Cancer. (2020) 11:5880–9. doi: 10.7150/jca.46258
20. Yamamoto K, Yoshikawa H, Shiromizu K, Saito T, Kuzuya K, Tsunematsu R, et al. Pulmonary metastasectomy for uterine cervical cancer: a multivariate analysis. Ann Thorac Surg. (2004) 77:1179–82. doi: 10.1016/j.athoracsur.2003.06.023
21. Tewari KS, Sill MW, Penson RT, Huang H, Ramondetta LM, Landrum LM, et al. Bevacizumab for advanced cervical cancer: final overall survival and adverse event analysis of a randomized, controlled, open-label, phase 3 trial (gynecologic oncology group 240). Lancet. (2017) 390:1654–63. doi: 10.1016/s0140-6736(17)31607-0
22. Qian S, Ye L, Tian YH, Wang LG, Huang ZP, Li F, et al. Californium-252 neutron brachytherapy combined with external pelvic radiotherapy plus concurrent chemotherapy for cervical cancer: a retrospective clinical study. Chin J Cancer. (2017) 36:24. doi: 10.1186/s40880-017-0191-x
23. He X, Liu J, Xiao L, Zhao M, Su T, Liu T, et al. Cisplatin-based chemotherapy with or without bevacizumab for Chinese postmenopausal women with advanced cervical cancer: a retrospective observational study. BMC Cancer. (2020) 20:381. doi: 10.1186/s12885-020-06854-w
24. Kumar L, Harish P, Malik PS, Khurana S. Chemotherapy and targeted therapy in the management of cervical cancer. Curr Probl Cancer. (2018) 42:120–8. doi: 10.1016/j.currproblcancer.2018.01.016
Keywords: cervical cancer, lung metastasis, nomogram, overall survival, SEER
Citation: Chang L and Zhao K (2024) Construction and validation of an innovative prognostic nomogram for overall survival in cervical cancer patients with lung metastasis: an analysis utilizing the SEER database. Front. Oncol. 14:1397454. doi: 10.3389/fonc.2024.1397454
Received: 07 March 2024; Accepted: 22 April 2024;
Published: 08 May 2024.
Edited by:
Lucely Cetina-Pérez, National Institute of Cancerology (INCAN), MexicoReviewed by:
Nuradh Joseph, Governement of Sri Lanka, Sri LankaCopyright © 2024 Chang and Zhao. This is an open-access article distributed under the terms of the Creative Commons Attribution License (CC BY). The use, distribution or reproduction in other forums is permitted, provided the original author(s) and the copyright owner(s) are credited and that the original publication in this journal is cited, in accordance with accepted academic practice. No use, distribution or reproduction is permitted which does not comply with these terms.
*Correspondence: Kangkang Zhao, NDY5NjQwMjExQHFxLmNvbQ==
Disclaimer: All claims expressed in this article are solely those of the authors and do not necessarily represent those of their affiliated organizations, or those of the publisher, the editors and the reviewers. Any product that may be evaluated in this article or claim that may be made by its manufacturer is not guaranteed or endorsed by the publisher.
Research integrity at Frontiers
Learn more about the work of our research integrity team to safeguard the quality of each article we publish.