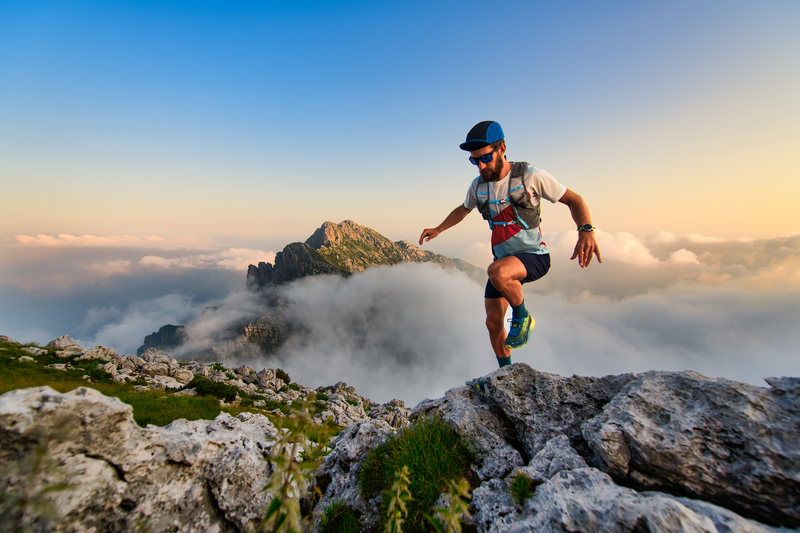
95% of researchers rate our articles as excellent or good
Learn more about the work of our research integrity team to safeguard the quality of each article we publish.
Find out more
ORIGINAL RESEARCH article
Front. Oncol. , 20 August 2024
Sec. Surgical Oncology
Volume 14 - 2024 | https://doi.org/10.3389/fonc.2024.1393990
Purpose: To construct and validate nomograms for predicting lung metastasis probability in patients with malignant primary osseous spinal neoplasms (MPOSN) at initial diagnosis and predicting cancer-specific survival (CSS) in the lung metastasis subgroup.
Methods: A total of 1,298 patients with spinal primary osteosarcoma, chondrosarcoma, Ewing sarcoma, and chordoma were retrospectively collected. Least absolute shrinkage and selection operator (LASSO) and multivariate logistic analysis were used to identify the predictors for lung metastasis. LASSO and multivariate Cox analysis were used to identify the prognostic factors for 3- and 5-year CSS in the lung metastasis subgroup. Receiver operating characteristic (ROC) curves, calibration curves, and decision curve analyses (DCA) were used to estimate the accuracy and net benefits of nomograms.
Results: Histologic type, grade, lymph node involvement, tumor size, tumor extension, and other site metastasis were identified as predictors for lung metastasis. The area under the curve (AUC) for the training and validating cohorts were 0.825 and 0.827, respectively. Age, histologic type, surgery at primary site, and grade were identified as the prognostic factors for the CSS. The AUC for the 3- and 5-year CSS were 0.790 and 0.740, respectively. Calibration curves revealed good agreements, and the Hosmer and Lemeshow test identified the models to be well fitted. DCA curves demonstrated that nomograms were clinically useful.
Conclusion: The nomograms constructed and validated by us could provide clinicians with a rapid and user-friendly tool to predict lung metastasis probability in patients with MPOSN at initial diagnosis and make a personalized CSS evaluation for the lung metastasis subgroup.
Malignant primary osseous spinal neoplasms (MPOSN) are very rare and make up less than 5% of all bone tumors (1). MPOSN mainly consist of osteosarcoma (35.1%), chondrosarcoma (25.8%), Ewing sarcoma (16.0%), and chordoma (8.4%) (2–4). Malignant primary osseous neoplasms occur primarily in extremities and rarely in the spine (5–7). However, when it occurs in the spine, the prognosis is poor. Extensive excision is effective and recommended for primary malignant bone tumors in the extremities, but extensive excision is more difficult and challenging in the spine, and is often dangerous (8). Moreover, previous studies have reported higher rates of lung metastasis of malignant tumors in the spine compared to other sites such as the extremities (9, 10). Zhang et al. suggested that occurring at the axial site, such as the spine, was significantly related to lung metastasis of malignant bone tumors such as osteosarcoma and chondrosarcoma (9). Although medical technology has developed increasingly in recent years, the survival rate of the patients with distant metastasis still remained poor (11, 12). It was worth noticing that the lungs have been proven as the most metastasized site, and the incidence of lung metastasis at initial diagnosis was approximately 10%–40% in malignant primary osseous neoplasms (13–16). Previous studies have demonstrated that the patients with MPOSN benefited greatly from the early diagnosis of lung metastasis (17–19). Considering the micro-metastases in malignant tumors and the moderate performance of conventional lung CT scan in the detection of small lung nodules (20, 21), combining clinicopathologic characteristics with imaging features may help to improve the accuracy of initial diagnosis. For those with MPOSN who have already presented lung metastasis, the early identification of survival rate can also help provide individual adjuvant therapies or trial options.
Previous literatures have reported many potential predictors for lung metastasis or survival rate in spine tumors (22, 23). However, the role of only a single variable may be limited. A predictive tool, which can integrate multiple significant risk features to make an individual prediction is urgently needed. The nomogram has been confirmed to provide a superior individual disease risk estimation and promote the decision management of treatment (22). To our limited knowledge, a nomogram for predicting cancer-specific survival (CSS) of lung metastasis subgroup in patients with MPOSN has not yet been reported. Moreover, the nomogram to predict lung metastasis probability in MPOSN at initial diagnosis was also rare and needs further large-sample investigation (10).
In the current study, the corresponding data from the Surveillance, Epidemiology, and End Results (SEER) database, which originates from 17 geographically variable cancer registries and represents approximately 26% of the US population were collected (24). The purpose of this study was to construct and validate nomograms for predicting lung metastasis probability in patients with MPOSN at initial diagnosis and predicting CSS in the lung metastasis subgroup.
The inclusion criteria were as follows: (1) diagnosed with the most common types of MPOSN (osteosarcoma, chondrosarcoma, Ewing sarcoma, and chordoma) in the SEER database between 2004 to 2015; (2) the primary sites of tumors were the spine; (3) microscopically confirmed, positive histology confirmed, or positive exfoliative cytology confirmed; and (3) known survival months and status.
The exclusion criteria were as follows: (1) unknown metastasis status; (2) unknown race; (3) unknown tumor size and unknown tumor extension; and (3) unknown surgery in primary site, unknown radiotherapy, and unknown lymph nodes removed.
Clinicopathologic features were as follows: (1) demographics (age, race, sex, year of birth, reporting source, insurance, and marriage); (2) tumor characteristics (tumor size, tumor extension, histologic type, grade, original laterality, lymph node involvement, and metastasis status); and (3) therapies (surgery at primary site, lymph nodes removed, radiotherapy, and chemotherapy) and survival data were identified.
The collected data (n = 1,298) were randomly assigned into a training cohort (n = 910) and a validation cohort (n = 388). The baseline clinicopathologic features between the two groups were compared via Chi-square test. Least absolute shrinkage and selection operator (LASSO) regression was performed to initially select the most significant predictive features and ensure that the multiple factor models were not over fitting. Multivariate logistic regression was used to identify the ultimate independent risk factors for lung metastasis prediction. In the lung metastasis subgroup, the ultimate prognostic factors for CSS were identified by multivariate Cox regression.
Based on the ultimate selected variables, the nomogram for lung metastasis prediction was constructed and internally validated in the training cohort and externally validated in the validation cohort. In the lung metastasis subgroup, the nomogram for the CSS was constructed and internally validated in the cancer-specific cases. The evaluation for the predictive discrimination of nomograms were performed via receiver operating characteristic (ROC) curves and the areas under the curves (AUC). Decision curve analyses (DCAs) were utilized to assess the clinical usefulness and net benefits of the prediction models (25, 26). Meanwhile, calibration curves and Hosmer–Lemeshow tests were used to validate the concordance of nomograms. Kaplan–Meier curves were plotted to perform to construct cumulative survival curves. The statistical significance was evaluated by Log-rank test.
All of these statistical analyses and graphics were performed by SPSS statistics software version 22.0 (IBM Corporation, Armonk, NY, USA), R software (3.6.3), and Rstudio software (1.2.5033). Two-sided p value <0.05 was defined to have statistical significance.
A total of 2,168 patients based on the inclusion criteria were selected from the SEER database during the period of 2004–2015. In total, 870 patients were excluded according to the exclusion criteria. Ultimately, 1,298 cases were determined and randomly divided into the training cohort (n = 980) and the validation cohort (n = 338). There were no significant differences between the two cohorts (Table 1, p > 0.05). In the lung metastasis subgroup, 4 cases who died due to causes other than cancer were excluded, and 122 cases were determined ultimately.
Because the exact time of therapies were unknown, we first excluded surgery at the primary site, lymph node removal, radiotherapy, and chemotherapy from the prediction for lung metastasis. LASSO regression analysis initially selected the significant factors, including age, race, year of birth, grade, histologic type, original laterality, tumor size, tumor extension, lymph node involvement, other site metastasis, insurance, and marriage (Figures 1A, B). The variables, including grade, histologic type, tumor size, tumor extension, lymph node involvement, and other site metastasis were identified as the ultimate risk factors for lung metastasis via multivariate logistic regression (Table 2, p < 0.05).
Figure 1. The results of the least absolute shrinkage and selection operator (LASSO) regression. The LASSO regression analysis initially selected age, race, year of birth, grade, histology type, origin laterality, tumor size, tumor extension, lymph nodes involvement, other site metastasis, insurance and marriage as the risk factors for lung metastasis (A, B), and age, histology type, other metastasis, grade, surgery at primary site, lymph nodes removed and chemotherapy as prognostic factors for the prediction of OS in the lung metastasis subgroup (C, D). LASSO, Least absolute shrinkage and selection operator; OS, Overall survival.
Table 2. Multivariate logistic regression for analyzing the lung metastasis-associated factors in the training cohort (n = 910).
Moreover, LASSO regression analysis initially selected variables, including age, histologic type, grade, other site metastasis, surgery at primary site, lymph node removed, and chemotherapy (Figures 1C, D) in the lung metastasis subgroup. Further, the variables, including age, histologic type, grade, and surgery at the primary site, were identified as the ultimate prognostic factors for the CSS via multivariate Cox regression analysis (Table 3, p < 0.05).
Table 3. Multivariate Cox regression for analyzing the prognosis-associated factors in the metastasis subgroup (n = 122).
Nomograms were constructed as follows to predict the probability of lung metastasis (Figure 2A, nomogram A) and the 3- and 5-year CSS in the lung metastasis subgroup (Figure 2B, nomogram B). The calibration curves for the two nomograms approached the ideal match straight line indicating that they are well calibrated (Figures 3A–C). The Hosmer and Lemeshow test identified the models as well fitted. The AUC of training and validating cohorts in the nomogram A were 0.825 and 0.827, respectively (Figures 4A, B). The AUC of 3- and 5-year CCS in the nomogram B were 0.790 and 0.740, respectively (Figures 4C, D), appearing with good predictive discrimination.
Figure 2. Nomograms for predicting the probability of lung metastasis (A) in patients with malignant primary osseous spinal neoplasms (MPOSN), and the 3- and 5-year cancer-specific survival (CSS) in the lung metastasis subgroups (B) (Grade I, well differentiated; Grade II, moderately differentiated; Grade III, poorly differentiated; Grade IV, undifferentiated).
Figure 3. Calibration curves showed the presentable accuracy of nomograms by comparing nomogram predictions with actual endpoints. (1) Calibration curve for nomogram A (A). (2) Calibration curves for nomogram B (B, C).
Figure 4. Receiver operating characteristic (ROC) curve of the nomograms. The areas under the curves (AUC) of training and validating cohorts in nomogram A were 0.825 and 0.827, respectively (A, B). The AUC of 3- and 5-year CCS in nomogram B were 0.790 and 0.740, respectively (C, D) appearing as good predictive discrimination.
DCA showed that the nomograms provided clinical usefulness and net benefits (Figures 5A, B). Kaplan–Meier curves and log-rank analyses demonstrated that advanced age (p < 0.001, Figure 6A), histologic type (p < 0.001, Figure 6B), high tumor grade (p = 0.009, Figure 6C), and those who did not undergo surgery at the primary site (p < 0.038, Figure 6D) were associated with worse prognoses.
Figure 5. Decision curve analyses (DCA) showed that the nomograms provided clinical usefulness and net benefits. (1) DCA for nomogram A (A). (2) DCA for nomogram B (B).
Figure 6. Kaplan–Meier curves and log-rank analyses demonstrated that advanced age (A), histologic type (B), high tumor grade (C), and those who did not undergo surgery at primary site (D) were associated with worse prognoses in the lung metastasis subgroup.
In recent years, nomograms have generally been used as a predictive tool for individual diagnosis or survival outcome (27, 28). For patients with MPOSN, nomograms can meet our desire for improving the diagnostic rate of lung metastasis and identifying the high-risk patients in the lung metastasis subgroup at an early stage.
In the present study, six characteristics were identified as the independent risk factors for lung metastasis, including grade, histologic type, other site metastasis, tumor size, tumor extension, and lymph node involvement. Similar results have been shown in the previous literatures. Xie et al. (22) investigated data of 4,459 patients with malignant primary osseous neoplasms and found variables, including histology type (osteosarcoma and Ewing sarcoma), lager tumors, or higher tumor grade were associated with higher possibility of lung metastasis. Fan et al. (10) reported that higher American Joint Committee on Cancer (AJCC) T stage, higher AJCC N stage, and tumor extension beyond the periosteum independently contributed to lung metastasis in MPOSN. Besides, LASSO regression and multivariate logistic regression analyses in our study demonstrated that other site metastasis was a novel risk factor to predict lung metastasis. The reason may be that the lungs are the most common site of metastasis in MPOSN, so once distant metastases occurred, whether it was detected or not, the occurrence of metastasis to the lungs was theoretically high.
In the lung metastasis subgroup, our survival analysis revealed that advanced age was related to poorer prognosis. A possible explanation is that older patients tended to have higher pathological grades and larger tumor sizes, which have been reported to correlate with survival of bone tumors (29). In our result, the possibility of histologic type for predicting lung metastasis from high to low were, respectively, osteosarcoma, chondrosarcoma, Ewing sarcoma, and chordoma. Previous studies have showed that 5-year survival rates were approximately 20%–30% in osteosarcoma patients with lung metastasis (30), and the rates were respectively 50% in Ewing sarcoma (11) and 45.7% in chondrosarcoma (31). Compared to the above, chordoma is a type of relatively slow-growing and low-grade malignancy, the 5-year overall survival was between 50% and 75%, and distant metastasis is rare (32, 33).
Mukherjee et al. (12) reported that independent of other factors, patients undergoing surgical resection of primary spinal chordoma, chondrosarcoma, Ewing sarcoma, or osteosarcoma all showed prolonged survival. They also found an interesting result that adjuvant radiotherapy can improve the survival only in patients with osteosarcoma and chordomas who underwent surgical resection. Similarly, radiotherapy and chemotherapy were not identified as independent prognostic factors in our study. This phenomenon may be due to some subclassifications of radiotherapy and chemotherapy in the SEER database, which were unknown due to the unavailable information. Meanwhile, different tumors have different responses to radiotherapy and chemotherapy (34, 35). Last but not the least, the effects of adjuvant radiotherapy and chemotherapy may be associated with surgical resection to some extent (36–41).
Based on the identified variables, we constructed and validated nomograms A and B (Figures 2A, B). For instance, a patient was diagnosed as having chondrosarcoma with beyond periosteum tumor extension and liver metastasis, and he did not undergo surgery at the primary site, and his tumor grade was III (poorly differentiated). Besides, the tumor size was 12 cm, and he had lymph node involvement. To use nomogram A (Figure 2A), we draw a perpendicular line from each predictive factor to obtain the corresponding points. By adding up each point, he gets approximately 435 total points, and we rapidly conclude his lung metastasis probability is approximately 65%. Based on the result, if the conventional lung CT scan revealed nothing, we may suggest the patient take further detection such as high-resolution CT or PET-CT.
There are also some limitations in the present study. First, this is a retrospective study, which may contain a latent risk of bias. Second, the internal and external validations of nomogram A were based on the same center. It may be more reliable to validate nomograms in different centers. Third, due to fewer sample data, we have to analyze the four malignancies together. We expect that more cases would be included in further prospective studies, and the cancer-specific survival analysis of lung metastasis would be carried out separately for four tumor types. Moreover, we did not have external validation of nomogram B. Last but not the least, because the factors, such as pathologic fracture, genetic, and epigenetic factors, were not found in the SEER database, they were not included in the study.
The nomograms constructed and validated by us could provide clinicians with a rapid and user-friendly tool to predict lung metastasis probability in patients with MPOSN at initial diagnosis and make a personalized CCS evaluation for the lung metastasis subgroup.
The original contributions presented in the study are included in the article/supplementary material. Further inquiries can be directed to the corresponding authors.
Ethical approval was not required for the study involving humans in accordance with the local legislation and institutional requirements. Written informed consent to participate in this study was not required from the participants or the participants’ legal guardians/next of kin in accordance with the national legislation and the institutional requirements.
YJ: Writing – original draft. YZ: Writing – original draft. YD: Writing – review & editing. XL: Writing – review & editing.
The author(s) declare that no financial support was received for the research, authorship, and/or publication of this article.
All the authors were directly involved in the whole process.
The authors declare that the research was conducted in the absence of any commercial or financial relationships that could be construed as a potential conflict of interest.
All claims expressed in this article are solely those of the authors and do not necessarily represent those of their affiliated organizations, or those of the publisher, the editors and the reviewers. Any product that may be evaluated in this article, or claim that may be made by its manufacturer, is not guaranteed or endorsed by the publisher.
1. Pickle LW, Hao Y, Jemal A, Zou Z, Tiwari RC, Ward E, et al. A new method of estimating United States and state-level cancer incidence counts for the current calendar year. CA: Cancer J Clin. (2007) 57:30–42. doi: 10.3322/canjclin.57.1.30
2. Biermann JS, Chow W, Reed DR, Lucas D, Adkins DR, Agulnik M, et al. NCCN guidelines insights: bone cancer, version 2.2017. J Natl Compr Cancer Network: JNCCN. (2017) 15:155–67. doi: 10.6004/jnccn.2017.0017
4. Sundaresan N, Rosen G, Boriani S. Primary Malignant tumors of the spine. Orthopedic Clinics North America. (2009) 40:21–36. doi: 10.1016/j.ocl.2008.10.004
5. Wang D, Liu F, Li B, Xu J, Gong H, Yang M, et al. Development and validation of a prognostic model for overall survival in patients with primary pelvis and spine osteosarcoma: A population-based study and external validation. J Clin Med. (2023) 12:2521. doi: 10.3390/jcm12072521
6. Pennington Z, Ehresman J, Pittman PD, Ahmed AK, Lubelski D, McCarthy EF, et al. Chondrosarcoma of the spine: a narrative review. Spine J. (2021) 21:2078–96. doi: 10.1016/j.spinee.2021.04.021
7. Sharafuddin MJ, Haddad FS, Hitchon PW, Haddad SF, el-Khoury GY. Treatment options in primary Ewing's sarcoma of the spine: report of seven cases and review of the literature. Neurosurgery. (1992) 30:610–8; discussion 618-9.
8. Schwab J, Gasbarrini A, Bandiera S, Boriani L, Amendola L, Picci P, et al. Osteosarcoma of the mobile spine. Spine (Phila Pa 1976). (2012) 37:E381–6. doi: 10.1097/BRS.0b013e31822fb1a7
9. Zhang C, Guo X, Xu Y, Han X, Cai J, Wang X, et al. Lung metastases at the initial diagnosis of high-grade osteosarcoma: prevalence, risk factors and prognostic factors. A large population-based cohort study. Sao Paulo Med J. (2019) 137:423–9. doi: 10.1590/1516-3180.2018.0381120619
10. Fan Y, Cai M, Xia L. Distinction and potential prediction of lung metastasis in patients with Malignant primary osseous spinal neoplasms. Spine (Phila Pa 1976). (2020) 45:921–9. doi: 10.1097/BRS.0000000000003421
11. Gaspar N, Hawkins DS, Dirksen U, Lewis IJ, Ferrari S, Le Deley MC, et al. Ewing sarcoma: current management and future approaches through collaboration. J Clin Oncol. (2015) 33:3036–46. doi: 10.1200/JCO.2014.59.5256
12. Mukherjee D, Chaichana KL, Parker SL, Gokaslan ZL, McGirt MJ. Association of surgical resection and survival in patients with Malignant primary osseous spinal neoplasms from the Surveillance, Epidemiology, and End Results (SEER) database. Eur Spine J. (2013) 22:1375–82. doi: 10.1007/s00586-012-2621-4
13. Kager L, Zoubek A, Potschger U, Kastner U, Flege S, Kempf-Bielack B, et al. Primary metastatic osteosarcoma: presentation and outcome of patients treated on neoadjuvant Cooperative Osteosarcoma Study Group protocols. J Clin Oncol. (2003) 21:2011–8. doi: 10.1200/JCO.2003.08.132
14. Pochanugool L, Subhadharaphandou T, Dhanachai M, Hathirat P, Sangthawan D, Pirabul R, et al. Prognostic factors among 130 patients with osteosarcoma. Clin orthopaedics related Res. (1997) 345):206–14. doi: 10.1097/00003086-199712000-00030
15. Jaffe N, Jaffe DM. Tumor size and prognosis in aggressively treated osteosarcoma. J Clin Oncol. (1996) 14:2399–400. doi: 10.1200/JCO.1996.14.8.2399
16. Wu PK, Chen WM, Chen CF, Lee OK, Haung CK, Chen TH. Primary osteogenic sarcoma with pulmonary metastasis: clinical results and prognostic factors in 91 patients. Japanese J Clin Oncol. (2009) 39:514–22. doi: 10.1093/jjco/hyp057
17. Kaste SC, Pratt CB, Cain AM, Jones-Wallace DJ, Rao BN. Metastases detected at the time of diagnosis of primary pediatric extremity osteosarcoma at diagnosis: imaging features. Cancer. (1999) 86:1602–8. doi: 10.1002/(ISSN)1097-0142
18. Bruns J, Elbracht M, Niggemeyer O. Chondrosarcoma of bone: an oncological and functional follow-up study. Ann Oncol. (2001) 12:859–64. doi: 10.1023/A:1011162118869
19. Luzzati F, Giusti EM, Scotto GM, Perrucchini G, Cannavò L, Castelnuovo G, et al. Quality of life, pain, and psychological factors in patients undergoing surgery for primary tumors of the spine. Supportive Care Cancer. (2020) 28:1385–93. doi: 10.1007/s00520-019-04965-0
20. Meybaum C, Graff M, Fallenberg EM, Leschber G, Wormanns D. Contribution of CAD to the sensitivity for detecting lung metastases on thin-section CT - A prospective study with surgical and histopathological correlation. RoFo: Fortschr auf dem Gebiete der Rontgenstrahlen und der Nuklearmedizin. (2020) 192:65–73. doi: 10.1055/a-0977-3453
21. Ciccarese F, Bazzocchi A, Ciminari R, Righi A, Rocca M, Rimondi E, et al. The many faces of pulmonary metastases of osteosarcoma: Retrospective study on 283 lesions submitted to surgery. Eur J Radiol. (2015) 84:2679–85. doi: 10.1016/j.ejrad.2015.09.022
22. Xie L, Huang W, Wang H, Zheng C, Jiang J. Risk factors for lung metastasis at presentation with Malignant primary osseous neoplasms: a population-based study. J Orthop Surg Res. (2020) 15:32. doi: 10.1186/s13018-020-1571-5
23. Huang X, Zhao J, Bai J, Shen H, Zhang B, Deng L, et al. Risk and clinicopathological features of osteosarcoma metastasis to the lung: A population-based study. J Bone Oncol. (2019) 16:100230. doi: 10.1016/j.jbo.2019.100230
24. Doll KM, Rademaker A, Sosa JA. Practical guide to surgical data sets: surveillance, epidemiology, and end results (SEER) database. JAMA Surg. (2018) 153:588–9. doi: 10.1001/jamasurg.2018.0501
25. Rousson V, Zumbrunn T. Decision curve analysis revisited: overall net benefit, relationships to ROC curve analysis, and application to case-control studies. BMC Med Inf decision making. (2011) 11:45. doi: 10.1186/1472-6947-11-45
26. Vickers AJ, Elkin EB. Decision curve analysis: a novel method for evaluating prediction models. Med decision making. (2006) 26:565–74. doi: 10.1177/0272989X06295361
27. Albert JM, Liu DD, Shen Y, Pan IW, Shih YC, Hoffman KE, et al. Nomogram to predict the benefit of radiation for older patients with breast cancer treated with conservative surgery. J Clin Oncol. (2012) 30:2837–43. doi: 10.1200/JCO.2011.41.0076
28. Balachandran VP, Gonen M, Smith JJ, DeMatteo RP. Nomograms in oncology: more than meets the eye, The Lancet. Oncology. (2015) 16:e173–80. doi: 10.1016/S1470-2045(14)71116-7
29. Huang R, Xian S, Shi T, Yan P, Hu P, Yin H, et al. Evaluating and predicting the probability of death in patients with non-metastatic osteosarcoma: A population-based study. Med Sci monitor. (2019) 25:4675–90. doi: 10.12659/MSM.915418
30. Meazza C, Scanagatta P. Metastatic osteosarcoma: a challenging multidisciplinary treatment. Expert Rev Anticancer Ther. (2016) 16:543–56. doi: 10.1586/14737140.2016.1168697
31. Nakamura T, Matsumine A, Yamada S, Tsukushi S, Kawanami K, Ohno T, et al. Oncological outcome after lung metastasis in patients presenting with localized chondrosarcoma at extremities: Tokai Musculoskeletal Oncology Consortium study. OncoTargets Ther. (2016) 9:4747–51. doi: 10.2147/OTT
32. van Wulfften Palthe ODR, Tromp I, Ferreira A, Fiore A, Bramer JAM, van Dijk NC, et al. Sacral chordoma: a clinical review of 101 cases with 30-year experience in a single institution. Spine J. (2019) 19:869–79. doi: 10.1016/j.spinee.2018.11.002
33. Le Charpentier Y, Bellefqih S, Boisnic S, Roy-Camille R. [Chordomas]. Annales pathologie. (1988) 8:25–32.
34. Sandberg AA, Bridge JA. Updates on the cytogenetics and molecular genetics of bone and soft tissue tumors: chondrosarcoma and other cartilaginous neoplasms. Cancer Genet cytogenetics. (2003) 143:1–31. doi: 10.1016/S0165-4608(03)00002-5
35. Vogin G, Helfre S, Glorion C, Mosseri V, Mascard E, Oberlin O, et al. Local control and sequelae in localised Ewing tumours of the spine: a French retrospective study. Eur J Cancer (Oxford Engl. (2013) 1990) 49:1314–23. doi: 10.1016/j.ejca.2012.12.005
36. Sheth DS, Yasko AW, Johnson ME, Ayala AG, Murray JA, Romsdahl MM. Chondrosarcoma of the pelvis. Prognostic factors for 67 patients treated with definitive surgery. Cancer. (1996) 78:745–50. doi: 10.1002/(ISSN)1097-0142
37. George B, Bresson D, Bouazza S, Froelich S, Mandonnet E, Hamdi S, et al. [Chordoma]. Neuro-Chirurgie. (2014) 60:63–140. doi: 10.1016/j.neuchi.2014.02.003
38. Ruosi C, Colella G, Di Donato SL, Granata F, Di Salvatore MG, Fazioli F. Surgical treatment of sacral chordoma: survival and prognostic factors. Eur Spine J. (2015) 24 Suppl 7:912–7. doi: 10.1007/s00586-015-4276-4
39. Leddy LR, Holmes RE. Chondrosarcoma of bone. Cancer Treat Res. (2014) 162:117–30. doi: 10.1007/978-3-319-07323-1_6
40. Biazzo A, De Paolis M. Multidisciplinary approach to osteosarcoma. Acta orthopaedica Belgica. (2016) 82:690–8.
Keywords: spinal tumors, SEER database, lung metastasis, survival analysis, nomogram
Citation: Jiang Y, Zhu Y, Ding Y and Lu X (2024) Nomograms to predict lung metastasis in malignant primary osseous spinal neoplasms and cancer-specific survival in lung metastasis subgroup. Front. Oncol. 14:1393990. doi: 10.3389/fonc.2024.1393990
Received: 31 March 2024; Accepted: 30 July 2024;
Published: 20 August 2024.
Edited by:
Zhimin Tao, Jiangsu University, ChinaReviewed by:
Gianluca Mascianà, Campus Bio-Medico University Hospital, ItalyCopyright © 2024 Jiang, Zhu, Ding and Lu. This is an open-access article distributed under the terms of the Creative Commons Attribution License (CC BY). The use, distribution or reproduction in other forums is permitted, provided the original author(s) and the copyright owner(s) are credited and that the original publication in this journal is cited, in accordance with accepted academic practice. No use, distribution or reproduction is permitted which does not comply with these terms.
*Correspondence: Yongli Ding, Y2RkZ3MyMDAyQDE2My5jb20=; Xinchang Lu, bHVjOTk5QDE2My5jb20=
Disclaimer: All claims expressed in this article are solely those of the authors and do not necessarily represent those of their affiliated organizations, or those of the publisher, the editors and the reviewers. Any product that may be evaluated in this article or claim that may be made by its manufacturer is not guaranteed or endorsed by the publisher.
Research integrity at Frontiers
Learn more about the work of our research integrity team to safeguard the quality of each article we publish.