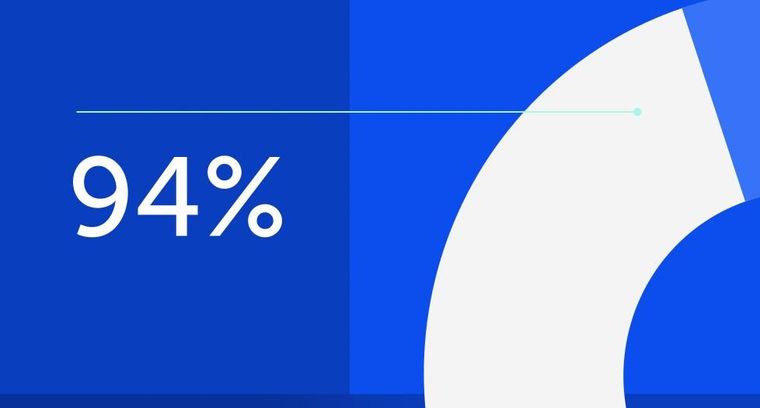
94% of researchers rate our articles as excellent or good
Learn more about the work of our research integrity team to safeguard the quality of each article we publish.
Find out more
ORIGINAL RESEARCH article
Front. Oncol., 11 April 2024
Sec. Cancer Epidemiology and Prevention
Volume 14 - 2024 | https://doi.org/10.3389/fonc.2024.1383864
Background: Several previous studies found a positive relationship between metabolic syndrome (MetS) and thyroid cancer (TC) risk. However, there is no research that has studied the relationship between the metabolic score for insulin resistance (METS-IR), a novel surrogate marker for IR, and TC incidence. Thus, we designed this retrospective cohort study to evaluate the relationship between the incidence of TC and METS-IR.
Method: We analyzed a cohort of 314,321 Korean adults aged over 40 years who participated in the National Health Screening Program from 2009 to 2010. The individuals were divided into four groups based on METS-IR quartiles. Follow-up was until the diagnosis of TC or death, or until December 31, 2019, if neither. The relationship between METS-IR and TC incidence was analyzed using the Cox proportional-hazards model with multi-variable adjustments.
Results: A total of 4,137 participants (1.3%) were diagnosed with TC during a mean follow-up of 9.5 ± 1.5 years. The population with Q1 METS-IR scores showed higher disease-free probabilities than those with Q4 METS-IR scores (p <0.001). The hazard ratio (95% confidential interval) for TC incidence in Q2, Q3, and Q4 METS-IR value were 1.14 (1.05 to 1.25), 1.21 (1.11 to 1.33), and 1.30 (1.18 to 1.42) compared with Q1 of METS-IR, respectively. The incidence of TC tended to increase with increasing METS-IR values in the total population, especially the male population in the restricted cubic spline. In subgroup analysis, the TC risk was more pronounced in the subgroups under 65 and with a BMI < 25 kg/m2.
Conclusion: METS-IR was positively correlated with TC incidence in Korea.
Thyroid cancer (TC) is the third most common cancer, following lung and stomach cancer, and the highest age-standardized incidence rate in Korea in 2020 (1). In women, it has the second highest crude and age-standardized incidence rates following breast cancer (1). In the past several decades, the incidence of TC has increased rapidly, in part due to improved detection technology and generalized fine-needle aspiration biopsies (2, 3). However, other risk factors for the development of TC cannot be ignored (4).
Metabolic syndrome (MetS) includes a cluster of metabolic disorders, including overweight, dysglycemia, hypertension, and dyslipidemia, and is significantly associated with an increased risk of developing diabetes mellitus and cardiovascular disease (5–7). Insulin resistance (IR) is known as one of the major mechanisms of MetS (7). Several models, such as hyperinsulinemic-euglycemic glucose clamp technique, homeostatic model assessment (HOMA), has been developed for determining insulin sensitivity or resistance (8, 9). However, these methods have some problems, including that is labor- and time-intensive or clinically less useful (8, 9). Thus, with the increasing interest for IR assessment in clinical settings, easier and more clinically practical markers have been in need. In 2018, the novel score, metabolic score for IR (METS-IR) was developed, which was calculated based on fasting blood glucose (FBG) levels, fasting triglyceride (TG) levels, body mass index (BMI), and high-density lipoprotein cholesterol (HDL-C) levels (10). As METS-IR demonstrated the better diagnostic and/or predicting performance to determine MetS compared to other makers, it has been used as surrogate marker for IR, which is valuable in predicting the development of MetS (10–13).
IR was reported to impact the incidence of several solid tumors, including colorectal, lung, and pancreatic cancer (14–17). A previous study reported that thyroid volumes were larger, and the risk of thyroid nodules was higher in IR patients (18). The relationship between MetS and TC has been suggested in several studies (19, 20), and it was also reported in a systemic review and meta-analysis (21). However, the study did not use METS-IR as a predictive value for TC and had a limitation due to differences in IR definitions between the studies analyzed (21).
The aim of this large retrospective cohort study was to evaluate the relationship between METS-IR, a novel score for insulin sensitivity, and the incidence of TC, especially in Korean individuals who participated in the National Health Screening Program (NHSP).
This was a retrospective population-based cohort study that used claims data obtained from the National Health Insurance Service-National Health Screening Cohort (NHIS-HealS) in Korea. The NHIS is the sole government insurer, providing a mandatory universal insurance system for the Korean population. It maintains a database of demographics, inpatient and outpatient claims, medical procedures, prescription drugs, and diagnoses according to the International Classification of Diseases, 10th edition (ICD-10). Additionally, the NHSP is provided to all insured individuals aged over 40 years every two years. This program includes anthropometric measurements, laboratory test results, and self-reported questionnaires about lifestyle behaviors. The NHIS-HealS database was established using data from NHSP participants.
We analyzed a cohort of Korean adults aged over 40 years (n = 362,285) who participated in the NHSP from 2009 to 2010, utilizing data from the NHIS-HealS database. The index date was set to the NHSP. We excluded individuals from this cohort who had TC before the index date (n = 2,874) and individuals who were diagnosed with cancers other than TC during the follow-up period (n = 26,356). We also excluded subjects with missing data (n = 3,850) and subjects with extreme METS-IR values (< 1% or > 99%) (n = 14,884). Finally, 314,321 subjects were analyzed in this study. Follow-up was until TC diagnosis or death, or until December 31, 2019, if neither (Figure 1).
METS-IR was defined as ln [(2 × FBG (mg/dL) + serum TG level (mg/dL)) × BMI (kg/m2)]/ln [serum HDL-C level (mg/dL)]. After removing extreme METS-IR values (< 1 or > 99 percentile), the participants were divided into four groups based on METS-IR quartiles (Q1, Q2, Q3, and Q4). We utilized NHIS data to identify individuals newly diagnosed with TC for data analysis with a primary focus on insurance claims. Patients with TC were identified based on the ICD-10 diagnostic code C73 (Supplementary Table 1).
Income level was categorized into quantiles, and residence was divided into urban and rural. The definitions of hypertension, diabetes, and dyslipidemia are described in Supplementary Table 1. The Charlson comorbidity index (CCI), a method of predicting mortality by classifying or weighting comorbid conditions, was calculated using diagnoses within one year before index data in the claims data (22). BMI was calculated as body weight in kilograms divided by height in meters squared (kg/m2). Obesity was defined as a BMI of ≥ 25 kg/m2 according to the guidelines provided by the World Health Organization considering the Asia-Pacific perspective. The laboratory results included hemoglobin levels (mg/dL) and estimated glomerular filtration rate (eGFR) (ml/min/1.73m2). Self-reported questionnaires were used to collect data on current smoking, alcohol drinking, and regular exercise habits.
Variables are reported as means with frequencies and percentages or standard deviations. Disease-free probability between the METS-IR and TC was visualized using a Kaplan–Meier curve and evaluated using log-rank test. The Cox proportional hazards model was used to evaluate the risk of TC with adjustments for multiple covariates, including sex, age, income level, residence, underlying disease, and health screening items. However, we adjusted the model for METS-IR for the aforementioned factors, excluding BMI. Hazard ratios (HRs) and the corresponding 95% confidence intervals (CIs) were used to determine the association between surrogate markers of IR and TC. The Schoenfeld residuals test with the logarithm of the cumulative hazard function based on Kaplan-Meier estimates was used to evaluate proportional hazard assumptions. No disturbance to the assumption that risk was proportional over time were seen. We also employed restricted cubic splines to examine HR trends along with their 95% CIs for TC, according to METS-IR. We used four knots at the 5th, 35th, 65th, and 95th percentiles according to METS-IR values, and the mean METS-IR values were selected as reference values in the restricted cubic spline. For additional sensitivity analysis, we used 1:1 propensity score matching in comparing Q1 and Q4. Matching variables included sex, age, income level, residence, underlying diseases including CCI, and health screening items including smoking, alcohol drinking, and exercise status. Propensity score matching was performed using the nearest neighbor matching method, and the caliper was set to less than 0.01. Statistical analyses were performed using SAS (version 9.4, SAS Institute Inc., Cary, NC, USA) and R 3.6.0 (R Foundation for Statistical Computing, Vienna, Austria) to ensure the accuracy and reliability of the results. P-values of less than 0.05 were considered statistically significant.
The study protocol was thoroughly reviewed and approved by the Institutional Review Board of Dong-A University Hospital (DAUHIRB-EXP-23-177), and the study was conducted in accordance with the Declaration of Helsinki. As this study used data from the National Health Insurance Service-National Health Screening Cohort in Korea, formal consent was not required.
The baseline characteristics of the study population, including demographics, underlying disease, and health screening, and quartiles based on METS-IR values are described in Table 1. The baseline characteristics of men and women are described in Supplementary Tables 2 and 3, respectively. The mean METS-IR values for the Q1 and Q4 are 2.1 and 2.5, respectively.
A total of 4,137 participants (1.3%) were diagnosed with TC during a mean follow-up of 9.5 ± 1.5 years. In the Kaplan-Meier curves, the population with Q1 METS-IR scores showed higher disease-free probabilities than those with Q4 METS-IR scores (Figure 2A). However, for women, the curves of the graph were relatively narrower than for men, despite statistically significant differences (p <0.001) (Figures 2B, C).
The crude and adjusted HRs with 95% CIs for the relationship between METS-IR quartiles and TC risk are described in Table 2. In the crude model, the total population and male population in Q2, Q3, and Q4 had significantly increased risks of TC compared to those with Q1 METS-IR values. In women, those in Q2 showed a tendency toward increased TC risk compared to those in Q1, although not statistically significant (p = 0.08). However, females in Q3 and Q4 had a statistically increased risk of TC compared with those in Q1 (p = 0.03 and 0.01, respectively). In the adjusted model, the total population and female population showed significant differences in TC incidence between Q1 and Q2, Q3, and Q4. In men, there were significant differences in TC incidence between the Q1 population and the Q3 and Q4 populations. However, the TC incidence of those in Q2 was not significantly different compared to those in Q1.
Table 2 Hazard ratio and 95% confidence interval for the incidence of thyroid cancer according to METS-IR values.
The restricted cubic spline, a mathematical function employed to model nonlinear relationships between a continuous predictor variable and an outcome variable, such as adjusted HRs with 95% CIs, is presented in Figure 3. The incidence of TC tended to increase with increasing METS-IR values in the total population, especially the male population (Figures 3A, B). In women, as METS-IR values increased beyond a certain point, the incidence of TC tended to decrease (Figure 3C).
Figure 3 Restricted cubic spline of hazard ratio with 95% confidence intervals for thyroid cancer according to METS-IR values.
Subgroup analysis according to age and BMI is described in Figure 4. In the subgroup under 65 years of age, those with Q1 METS-IR scores showed higher disease-free probabilities than those with Q4 METS-IR scores. However, the difference in disease-free probabilities between the Q1 and Q4 populations was not statistically significant. Also, a trend toward increased TC risk was seen in the BMI < 25 kg/m2 subgroup with higher METS-IR scores, but this trend was not seen in the BMI ≥ 25 kg/m2 subgroup.
Baseline characteristics of the study population before and after propensity score matching were described in Supplementary Table 4. After propensity score matching between Q1 and Q4 groups, there were 69,426 subjects with no significant differences in baseline characteristics. After adjustments, the HRs with 95% CIs for TC risk compared Q4 to Q1 were 1.31 (1.15 to 1.49). Regarding sex, the HRs with 95% CIs for TC risk compared Q4 to Q1 were 1.59 (1.19 to 2.13) in males and 1.25 (1.08 to 1.44) in females, respectively.
MetS refers to the presence of a cluster of risk factors specific to cardiovascular disease. According to the National Heart, Lung, and Blood Institute, the cluster of metabolic factors includes abdominal obesity, high blood pressure, impaired fasting blood glucose levels, high TG levels, and low HDL-C levels (23). MetS has been reported to possibly increase the risk of cancers, such as liver, colorectum, and mammary tissue cancer, as well as cardiovascular disease (16). MetS changes the microenvironment of the organism and is accompanied by insulin resistance, both of which are related to tumor formation (19). As with these malignancies, previous studies showed that the incidence of thyroid cancer was associated with MetS (19–21).
Son et al. suggested that obesity was positively correlated with the risk of TC after an average follow-up of seven years in Koreans aged over 20 years (24). Another prospective cohort study found a positive association between BMI and thyroid cancer, especially in women (20). Kown et al. concluded that weight gain significantly increased the incidence of TC, but weight loss was a protective factor for TC (25). Like obesity, high blood pressure and dyslipidemia have been positively linked to TC (21, 26). High FBG levels and diabetes mellitus suggest insulin resistance, but their impact on TC risk is still controversial (19, 27). A prospective cohort study showed that glucose was associated with an increase in TC risk in men. In contrast, an inverse association was seen between glucose and TC risk in women (20). In another matched study, history and the duration of diabetes mellitus were not associated with TC risk, but women with diabetes mellitus for fewer than five years had a reduced risk of TC (27).
In Korea, TC was expected to be the most common form of solid tumor in the 15–34 age group in both sexes, and the incidence of TC is higher in women compared to men (1). In women, the age-specific incidence of TC, along with breast cancer, is expected to level off after the age of 40 years (1). Similar differences in the incidence of TC between the sexes worldwide have been reported (28). In a previous cohort study and meta-analysis, gender disparity was mostly confined to the detection of small subclinical papillary TC (PTC) (29), which has the best prognosis of any subtype of TC. While TC incidence differs between the sexes, the difference in mortality due to TC is not significant (28), which could also be explained by the higher frequency of PTC in women than in men. The reasons for the gender disparity in TC incidence are not fully understood, but female sex hormones possibly play a role (30). Several risk factors for TC, including thyroid-stimulating hormone (TSH), adipokines, and angiotensin II, as well as MetS and estrogen, have been identified (19). A previous study concluded that the aggressiveness of PTC was associated with MetS, which was still significant after adjusting for age, sex, TSH, and BMI (31). However, less is known about the relationship between other thyroid cancer subtypes and MetS.
Given this, a causal relationship between METS-IR and TC incidence would be expected to be stronger in women. As expected, in this study, the female population showed a clearer association between METS-IR values and TC incidence in the adjusted model. However, in the crude model, the relationship was clear in men (Table 2). In women, as METS-IR values increased beyond a certain point, the incidence of TC tended to decrease (Figure 3C). We suspect that the risk factors used in the adjustment affected the results. Thus, further research is needed to determine which risk factors have an impact, especially if the METS-IR value is above a certain level. Also, the diagnosis of other cancers, such as breast cancer, before TC diagnosis might affect the incidence of TC in the female population with high METS-IR values.
In subgroup analysis, the association between METS-IR scores and TC risk was stronger in younger patients (< 65 years old) and in patients with low BMI (BMI < 25 kg/m2). The relationship between METS-IR scores and TC risk was much clearer, with statistical significance in the subgroup < 65 years old. However, in the subgroup ≥ 65 years old, there were no statistically significant differences between METS-IR scores and TC risk (Figure 4). Based on this, it is thought that age and BMI play a more important role than other MetS factors in the development of TC, although further study is needed.
This study had some limitations. First, whereas TCs are known as heterogenous in histologic and genetics and have different prognoses according to the subtypes (32), this study could not include information on heterogeneity. Second, information about hormones, such as TSH and estrogen, and other hidden covariates that could affect the incidence of TC was lacking. Also, as this study was retrospective, it utilized only TG, FBG, HDL-C, and BMI measurements at the time of health examination. Thus, we could not assess the extent of changes in these metrics and their potential impact on TC incidence. Despite these limitations, we think that this study is valuable in several ways. To the best of our knowledge, this was the first large cohort study to compare the association between METS-IR and TC incidence. Also, it is thought that this study could be representative of the Korean population because it used nationwide population-based data. Furthermore, we explored the relationship between METS-IR values and TC incidence using various analytic methods.
Despite the high incidence of TC, thyroid ultrasonography is still not included in basic health screening in Korea. One of the reasons for this is concern about overdiagnosis of TC (33), because differentiated TC, including PTC and follicular TC, has good prognosis (34). However, there is a concern that many cases of TC might exist but remain undetected. Also, in the clinic, some advanced TC afflicts many patients and increases socioeconomic costs. Thus, selective screening could be implemented for people at high risk of TC. Also, when the relationship between the aggressiveness of PTC and MetS is additionally considered (31), the results of this study are expected to provide information for which population should be screened for TC. As various targeted therapies have been developed and used in cancer treatment, including TC (35, 36), it would be worthwhile to further investigate the correlation of METS-IR and molecular genetic alterations in TC.
METS-IR was positively correlated with TC incidence in Korea.
The datasets generated during and/or analyzed during the current study are available from the corresponding author on reasonable request. Requests to access these datasets should be directed to Hye Ryeon Kim, aHllcnllb24xM0BkYXUuYWMua3I=.
The studies involving humans were approved by Institutional Review Board of Dong-A University Hospital (DAUHIRB-EXP-23-177). The studies were conducted in accordance with the local legislation and institutional requirements. Written informed consent for participation was not required from the participants or the participants’ legal guardians/next of kin in accordance with the national legislation and institutional requirements.
HK: Writing – original draft, Writing – review & editing. MS: Conceptualization, Data curation, Formal analysis, Methodology, Writing – original draft, Writing – review & editing. SH: Writing – review & editing. SM: Writing – review & editing. HM: Writing – review & editing. YK: Writing – review & editing. MK: Writing – review & editing. JL: Conceptualization, Writing – review & editing.
The author(s) declare financial support was received for the research, authorship, and/or publication of this article. This study was supported by Dong-A University Research fund 2024.
The authors declare that the research was conducted in the absence of any commercial or financial relationships that could be construed as a potential conflict of interest.
All claims expressed in this article are solely those of the authors and do not necessarily represent those of their affiliated organizations, or those of the publisher, the editors and the reviewers. Any product that may be evaluated in this article, or claim that may be made by its manufacturer, is not guaranteed or endorsed by the publisher.
The Supplementary Material for this article can be found online at: https://www.frontiersin.org/articles/10.3389/fonc.2024.1383864/full#supplementary-material
1. Jung KW, Won YJ, Hong S, Kong HJ, Lee ES. Prediction of cancer incidence and mortality in Korea, 2020. Cancer Res Treat. (2020) 52:351–8. doi: 10.4143/crt.2020.203
2. Davies L, Welch HG. Increasing incidence of thyroid cancer in the United States, 1973-2002. JAMA. (2006) 295:2164–7. doi: 10.1001/jama.295.18.2164
3. Zevallos JP, Hartman CM, Kramer JR, Sturgis EM, Chiao EY. Increased thyroid cancer incidence corresponds to increased use of thyroid ultrasound and fine-needle aspiration: a study of the Veterans Affairs health care system. Cancer. (2015) 121:741–6. doi: 10.1002/cncr.29122
4. Morris LG, Myssiorek D. Improved detection does not fully explain the rising incidence of well-differentiated thyroid cancer: a population-based analysis. Am J Surg. (2010) 200:454–61. doi: 10.1016/j.amjsurg.2009.11.008
5. Schlaich M, Straznicky N, Lambert E, Lambert G. Metabolic syndrome: a sympathetic disease? Lancet Diabetes Endocrinol. (2015) 3:148–57. doi: 10.1016/S2213-8587(14)70033-6
6. Xiao J, Shen C, Chu MJ, Gao YX, Xu GF, Huang JP, et al. Physical activity and sedentary behavior associated with components of metabolic syndrome among people in rural China. PloS One. (2016) 11:e0147062. doi: 10.1371/journal.pone.0147062
7. Fahed G, Aoun L, Bou Zerdan M, Allam S, Bou Zerdan M, Bouferraa Y, et al. Metabolic syndrome: updates on pathophysiology and management in 2021. Int J Mol Sci. (2022) 23. doi: 10.3390/ijms23020786
8. Singh B, Saxena A. Surrogate markers of insulin resistance: A review. World J Diabetes. (2010) 1:36–47. doi: 10.4239/wjd.v1.i2.36
9. Matthews DR, Hosker JP, Rudenski AS, Naylor BA, Treacher DF, Turner RC. Homeostasis model assessment: insulin resistance and beta-cell function from fasting plasma glucose and insulin concentrations in man. Diabetologia. (1985) 28:412–9. doi: 10.1007/BF00280883
10. Bello-Chavolla OY, Almeda-Valdes P, Gomez-Velasco D, Viveros-Ruiz T, Cruz-Bautista I, Romo-Romo A, et al. METS-IR, a novel score to evaluate insulin sensitivity, is predictive of visceral adiposity and incident type 2 diabetes. Eur J Endocrinol. (2018) 178:533–44. doi: 10.1530/EJE-17-0883
11. Wu Z, Cui H, Zhang Y, Liu L, Zhang W, Xiong W, et al. The impact of the metabolic score for insulin resistance on cardiovascular disease: a 10-year follow-up cohort study. J Endocrinol Invest. (2022) 46:523–33. doi: 10.1007/s40618-022-01925-0
12. Widjaja NA, Irawan R, Hanindita MH, Ugrasena I, Handajani R. METS-IR vs. HOMA-AD and metabolic syndrome in obese adolescents. J Med Invest. (2023) 70:7–16. doi: 10.2152/jmi.70.7
13. Han KY, Gu J, Wang Z, Liu J, Zou S, Yang CX, et al. Association between METS-IR and prehypertension or hypertension among normoglycemia subjects in Japan: A retrospective study. Front Endocrinol (Lausanne). (2022) 13:851338. doi: 10.3389/fendo.2022.851338
14. Esposito K, Chiodini P, Capuano A, Bellastella G, Maiorino MI, Rafaniello C, et al. Colorectal cancer association with metabolic syndrome and its components: a systematic review with meta-analysis. Endocrine. (2013) 44:634–47. doi: 10.1007/s12020-013-9939-5
15. Son M, Moon SY, Koh M, Kang Y, Lee JY. Association between surrogate markers of insulin resistance and the incidence of colorectal cancer in Korea: A nationwide population-based study. J Clin Med. (2024) 13. doi: 10.3390/jcm13061628
16. Esposito K, Chiodini P, Colao A, Lenzi A, Giugliano D. Metabolic syndrome and risk of cancer: a systematic review and meta-analysis. Diabetes Care. (2012) 35:2402–11. doi: 10.2337/dc12-0336
17. Rosato V, Tavani A, Bosetti C, Pelucchi C, Talamini R, Polesel J, et al. Metabolic syndrome and pancreatic cancer risk: a case-control study in Italy and meta-analysis. Metabolism. (2011) 60:1372–8. doi: 10.1016/j.metabol.2011.03.005
18. Ayturk S, Gursoy A, Kut A, Anil C, Nar A, Tutuncu NB. Metabolic syndrome and its components are associated with increased thyroid volume and nodule prevalence in a mild-to-moderate iodine-deficient area. Eur J Endocrinol. (2009) 161:599–605. doi: 10.1530/EJE-09-0410
19. Li LR, Song JL, Liu HQ, Chen C. Metabolic syndrome and thyroid Cancer: risk, prognosis, and mechanism. Discovery Oncol. (2023) 14:23. doi: 10.1007/s12672-022-00599-7
20. Almquist M, Johansen D, Bjorge T, Ulmer H, Lindkvist B, Stocks T, et al. Metabolic factors and risk of thyroid cancer in the Metabolic syndrome and Cancer project (Me-Can). Cancer Causes Control. (2011) 22:743–51. doi: 10.1007/s10552-011-9747-2
21. Yin DT, He H, Yu K, Xie J, Lei M, Ma R, et al. The association between thyroid cancer and insulin resistance, metabolic syndrome and its components: A systematic review and meta-analysis. Int J Surg. (2018) 57:66–75. doi: 10.1016/j.ijsu.2018.07.013
22. Quan H, Li B, Couris CM, Fushimi K, Graham P, Hider P, et al. Updating and validating the Charlson comorbidity index and score for risk adjustment in hospital discharge abstracts using data from 6 countries. Am J Epidemiol. (2011) 173:676–82. doi: 10.1093/aje/kwq433
23. Grundy SM, Cleeman JI, Daniels SR, Donato KA, Eckel RH, Franklin BA, et al. Diagnosis and management of the metabolic syndrome: an American Heart Association/National Heart, Lung, and Blood Institute Scientific Statement. Circulation. (2005) 112:2735–52. doi: 10.1161/CIRCULATIONAHA.105.169404
24. Son H, Lee H, Kang K, Lee I. The risk of thyroid cancer and obesity: A nationwide population-based study using the Korea National Health Insurance Corporation cohort database. Surg Oncol. (2018) 27:166–71. doi: 10.1016/j.suronc.2018.03.001
25. Kwon H, Han KD, Park CY. Weight change is significantly associated with risk of thyroid cancer: A nationwide population-based cohort study. Sci Rep. (2019) 9:1546. doi: 10.1038/s41598-018-38203-0
26. Park JH, Choi M, Kim JH, Kim J, Han K, Kim B, et al. Metabolic syndrome and the risk of thyroid cancer: A nationwide population-based cohort study. Thyroid. (2020) 30:1496–504. doi: 10.1089/thy.2019.0699
27. Wang M, Gong WW, Lu F, Hu RY, He QF, Yu M. The association between diabetes and thyroid cancer risk: a hospital-based case-control study in China. BMC Endocr Disord. (2021) 21:21. doi: 10.1186/s12902-021-00684-y
28. Siegel RL, Miller KD, Wagle NS, Jemal A. Cancer statistics, 2023. CA Cancer J Clin. (2023) 73:17–48. doi: 10.3322/caac.21763
29. LeClair K, Bell KJL, Furuya-Kanamori L, Doi SA, Francis DO, Davies L. Evaluation of gender inequity in thyroid cancer diagnosis: differences by sex in US thyroid cancer incidence compared with a meta-analysis of subclinical thyroid cancer rates at autopsy. JAMA Intern Med. (2021) 181:1351–8. doi: 10.1001/jamainternmed.2021.4804
30. Negri E, Dal Maso L, Ron E, La Vecchia C, Mark SD, Preston-Martin S, et al. A pooled analysis of case-control studies of thyroid cancer. II. Menstrual and reproductive factors. Cancer Causes Control. (1999) 10:143–55. doi: 10.1023/A:1008880429862
31. Song JL, Li LR, Yu XZ, Zhan L, Xu ZL, Li JJ, et al. Association between metabolic syndrome and clinicopathological features of papillary thyroid cancer. Endocrine. (2022) 75:865–71. doi: 10.1007/s12020-021-02940-6
32. Araque KA, Gubbi S, Klubo-Gwiezdzinska J. Updates on the management of thyroid cancer. Horm Metab Res. (2020) 52:562–77. doi: 10.1055/a-1089-7870
33. Ahn HS, Kim HJ, Welch HG. Korea's thyroid-cancer "epidemic"–screening and overdiagnosis. N Engl J Med. (2014) 371:1765–7. doi: 10.1056/NEJMp1409841
34. Schmidbauer B, Menhart K, Hellwig D, Grosse J. Differentiated thyroid cancer-treatment: state of the art. Int J Mol Sci. (2017) 18. doi: 10.3390/ijms18061292
35. Cabanillas ME, Ryder M, Jimenez C. Targeted therapy for advanced thyroid cancer: kinase inhibitors and beyond. Endocr Rev. (2019) 40:1573–604. doi: 10.1210/er.2019-00007
Keywords: thyroid cancer, incidence, metabolic syndrome, insulin resistance, METS-IR
Citation: Kim HR, Son M, Huh SJ, Moon SY, Moon H, Kang YW, Koh M and Lee JY (2024) Relationship between METS-IR and thyroid cancer incidence in Korea: a nationwide population-based study. Front. Oncol. 14:1383864. doi: 10.3389/fonc.2024.1383864
Received: 09 February 2024; Accepted: 26 March 2024;
Published: 11 April 2024.
Edited by:
So Hee Kwon, Yonsei University, Republic of KoreaReviewed by:
Xiaoliang Shao, The Third Affiliated Hospital of Soochow University, ChinaCopyright © 2024 Kim, Son, Huh, Moon, Moon, Kang, Koh and Lee. This is an open-access article distributed under the terms of the Creative Commons Attribution License (CC BY). The use, distribution or reproduction in other forums is permitted, provided the original author(s) and the copyright owner(s) are credited and that the original publication in this journal is cited, in accordance with accepted academic practice. No use, distribution or reproduction is permitted which does not comply with these terms.
*Correspondence: Jong Yoon Lee, bGp5aGF0ZW9AZ21haWwuY29t
†These authors share first authorship
Disclaimer: All claims expressed in this article are solely those of the authors and do not necessarily represent those of their affiliated organizations, or those of the publisher, the editors and the reviewers. Any product that may be evaluated in this article or claim that may be made by its manufacturer is not guaranteed or endorsed by the publisher.
Research integrity at Frontiers
Learn more about the work of our research integrity team to safeguard the quality of each article we publish.