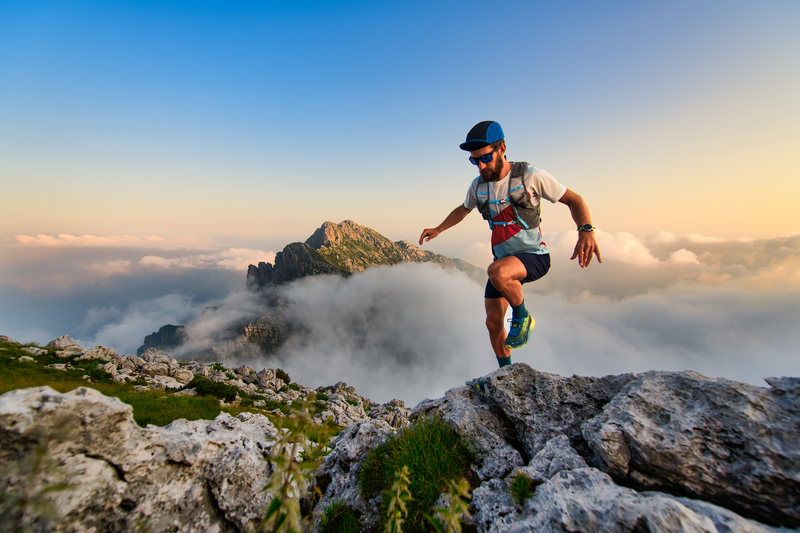
94% of researchers rate our articles as excellent or good
Learn more about the work of our research integrity team to safeguard the quality of each article we publish.
Find out more
GENERAL COMMENTARY article
Front. Oncol. , 09 February 2024
Sec. Gastrointestinal Cancers: Colorectal Cancer
Volume 14 - 2024 | https://doi.org/10.3389/fonc.2024.1337576
This article is a commentary on:
An artificial intelligence prediction model outperforms conventional guidelines in predicting lymph node metastasis of T1 colorectal cancer
by Piao ZH, Ge R and Lu L (2023) Front. Oncol. 13:1229998. doi: 10.3389/fonc.2023.1229998
Approximately 10% of T1 colorectal cancers (CRCs) harbor lymph node metastasis (LNM), necessitating surgical resection with lymph node dissection for curative intent (1). Accurate preoperative assessment of LNM risk following endoscopic treatment of T1 CRC is critical to determine the need for additional bowel resection. Present guidelines stipulate risk factors for LNM and indications for subsequent surgery post-endoscopic resection, including submucosal invasion depth ≥1000 µm, positive lymphovascular invasion, poor differentiation adenocarcinoma, signet-ring cell carcinoma, mucinous carcinoma, and tumor budding grade (grade 2 or 3) (2). Nevertheless, the current guidelines yield approximately 10% accuracy for LNM prediction, highlighting the necessity for a more precise model to prevent excessive treatment. Although various prediction models for LNM have been proposed, a consensus on the essential predictors and their number for effective clinical application remains a subject for discussion (3–6).
Piao et al. crafted an artificial intelligence (AI) model employing a classification and regression tree algorithm that outperformed both the Japanese Society for Cancer of the Colon and Rectum in Japan (AUC=0.588) and National Comprehensive Cancer Network guidelines in the US (AUC=0.850), achieving superior discriminative ability (AUC=0.960) (7). This model incorporated a comprehensive array of 12 clinicopathological variables: patient age and sex, tumor size, location, and morphology, histologic differentiation, lymphovascular invasion, tumor budding, poorly differentiated clusters, and the width, depth, and area of submucosal invasion. Their model incorporated a larger number of variables compared to previous models (Table 1).
Table 1 Variable selection of the predicting model for lymph node metastasis in T1 colorectal cancer.
The selection of variables is crucial to enhance the model’s predictive accuracy and to circumvent overfitting. Three core considerations are paramount in this domain. Firstly, the correlation between the variables and LNM is essential. Along with the established pathological risk factors, recent extensive Japanese multicenter studies have identified a correlation between LNM and variables such as patient age and sex, as well as tumor location and size (5, 6). Notably, Piao’s study highlighted the significant contribution of patient age and tumor size to LNM risk. Secondly, the generality of the information is vital; it must be readily available at any medical facility. While patient age and sex, tumor location and size, along with guideline-specified pathological risk factors, are commonly accessible, the width and area of submucosal invasion, though critical to their AI model, may not be universally available. Lastly, the predictive model’s reproducibility warrants attention. Pathological variables are subject to inter-pathologist variability, unlike stable clinical variables such as age and sex. The interobserver reliability for depth of submucosal invasion, lymphovascular invasion, differentiation, and tumor budding is reported to be relatively low, with kappa values ranging between 0.10 and 0.68 (1). In addition, pathological diagnostic criteria can vary between countries, and the application of immunostaining may differ among institutions.
Piao’s investigation illustrates that an AI-assisted predictive model, integrating clinical with pathological factors, surpasses the accuracy of the current guidelines. The impending challenge lies in discerning the appropriate factors for inclusion and ensuring reproducibility in routine clinical practice.
KI: Conceptualization, Writing – original draft. S-EK: Writing – review & editing. KY: Writing – review & editing.
The author(s) declare that no financial support was received for the research, authorship, and/or publication of this article.
The authors declare that the research was conducted in the absence of any commercial or financial relationships that could be construed as a potential conflict of interest.
All claims expressed in this article are solely those of the authors and do not necessarily represent those of their affiliated organizations, or those of the publisher, the editors and the reviewers. Any product that may be evaluated in this article, or claim that may be made by its manufacturer, is not guaranteed or endorsed by the publisher.
1. Ichimasa K, Kudo SE, Miyachi H, Kouyama Y, Mochizuki K, Takashina Y, et al. Current problems and perspectives of pathological risk factors for lymph node metastasis in T1 colorectal cancer: Systematic review. Dig Endosc (2022) 34:901–12. doi: 10.1111/den.14220
2. Hashiguchi Y, Muro K, Saito Y, Ito Y, Ajioka Y, Hamaguchi T, et al. Japanese Society for Cancer of the Colon and Rectum (JSCCR) guidelines 2019 for the treatment of colorectal cancer. Int J Clin Oncol (2020) 25:1–42. doi: 10.1007/s10147-019-01485-z
3. Oh JR, Park B, Lee S, Han KS, Youk EG, Lee DH, et al. Nomogram development and external validation for predicting the risk of lymph node metastasis in T1 colorectal cancer. Cancer Res Treat (2019) 51:1275–84. doi: 10.4143/crt.2018.569
4. Ahn JH, Kwak MS, Lee HH, Cha JM, Shin HP, Jeon JW, et al. Development of a novel prognostic model for predicting lymph node metastasis in early colorectal cancer: Analysis based on the surveillance, epidemiology, and end results database. Front Oncol (2021) 11:614398. doi: 10.3389/fonc.2021.614398
5. Kudo SE, Ichimasa K, Villard B, Mori Y, Misawa M, Saito S, et al. Artificial intelligence system to determine risk of T1 colorectal cancer metastasis to lymph node. Gastroenterology (2021) 160:1075–1084.e1072. doi: 10.1053/j.gastro.2020.09.027
6. Kajiwara Y, Oka S, Tanaka S, Nakamura T, Saito S, Fukunaga Y, et al. Nomogram as a novel predictive tool for lymph node metastasis in T1 colorectal cancer treated with endoscopic resection: A nationwide, multicenter study. Gastrointest Endosc (2023) 97:1119–1128.e1115. doi: 10.1016/j.gie.2023.01.022
Keywords: T1 colorectal cancer, lymph node metastasis, artificial intelligence, risk factor, variable selection algorithm
Citation: Ichimasa K, Kudo S-e and Yeoh KG (2024) Commentary: An artificial intelligence prediction model outperforms conventional guidelines in predicting lymph node metastasis of T1 colorectal cancer. Front. Oncol. 14:1337576. doi: 10.3389/fonc.2024.1337576
Received: 13 November 2023; Accepted: 22 January 2024;
Published: 09 February 2024.
Edited by:
Tadahiko Masaki, Kyorin University, JapanReviewed by:
Yasuhiko Mizuguchi, National Cancer Centre, JapanCopyright © 2024 Ichimasa, Kudo and Yeoh. This is an open-access article distributed under the terms of the Creative Commons Attribution License (CC BY). The use, distribution or reproduction in other forums is permitted, provided the original author(s) and the copyright owner(s) are credited and that the original publication in this journal is cited, in accordance with accepted academic practice. No use, distribution or reproduction is permitted which does not comply with these terms.
*Correspondence: Katsuro Ichimasa, aWNoaW1hc2FAbnVzLmVkdS5zZw==
Disclaimer: All claims expressed in this article are solely those of the authors and do not necessarily represent those of their affiliated organizations, or those of the publisher, the editors and the reviewers. Any product that may be evaluated in this article or claim that may be made by its manufacturer is not guaranteed or endorsed by the publisher.
Research integrity at Frontiers
Learn more about the work of our research integrity team to safeguard the quality of each article we publish.