- Department of Thoracic Surgery, Qilu Hospital of Shandong University, Jinan, China
Background: This study aimed to construct a clinical prediction model and nomogram to differentiate invasive from non-invasive pulmonary adenocarcinoma in solitary pulmonary nodules (SPNs).
Method: We analyzed computed tomography and clinical features as well as preoperative biomarkers in 1,106 patients with SPN who underwent pulmonary resection with definite pathology at Qilu Hospital of Shandong University between January 2020 and December 2021. Clinical parameters and imaging characteristics were analyzed using univariate and multivariate logistic regression analyses. Predictive models and nomograms were developed and their recognition abilities were evaluated using receiver operating characteristic (ROC) curves. The clinical utility of the nomogram was evaluated using decision curve analysis (DCA).
Result: The final regression analysis selected age, carcinoembryonic antigen, bronchus sign, lobulation, pleural adhesion, maximum diameter, and the consolidation-to-tumor ratio as associated factors. The areas under the ROC curves were 0.844 (95% confidence interval [CI], 0.817–0.871) and 0.812 (95% CI, 0.766–0.857) for patients in the training and validation cohorts, respectively. The predictive model calibration curve revealed good calibration for both cohorts. The DCA results confirmed that the clinical prediction model was useful in clinical practice. Bias-corrected C-indices for the training and validation cohorts were 0.844 and 0.814, respectively.
Conclusion: Our predictive model and nomogram might be useful for guiding clinical decisions regarding personalized surgical intervention and treatment options.
1 Introduction
Solitary pulmonary nodules (SPNs) are single well-defined imaging opacities ≤ 30 mm in diameter surrounded by lung parenchyma without pulmonary atelectasis, mediastinal lymph node enlargement, or pleural effusion (1). Solitary pulmonary nodules ≤ 20 mm are defined as small (2). The present dominant technique for lung cancer screening is high-resolution computed tomography (CT), which has substantially increased detection rates of isolated, particularly, of small SPNs (3–5). In clinical practice, overall survival is similar for lesions ≤2 cm removed by sublobar resection and lobectomy. The choice of sublobar or lobectomy is debatable. Adenocarcinoma is the most prevalent histological type of lung cancer and its incidence has recently increased (6–12). The World Health Organization (WHO) classification of lung tumors (2021) has categorized lung adenocarcinomas as preinvasive lesions that include atypical adenomatous hyperplasia (AAH), adenocarcinoma in situ (AIS), minimally invasive (MIA) and invasive (IAC) adenocarcinoma (13).
A recently proposed pathological classification is that lung adenocarcinoma should be categorized as pre-invasive pulmonary adenocarcinoma (IPA) and IPA. Pre-IPA lesions comprise AAH, AIS, and MIA (14, 15). Clinical treatment tends to differ between pre-IPA and IPA; sublobar resection might be reasonable for pre-IPA lesions because the 5-year survival rate after complete resection is ~ 100%, whereas standard lobectomy and lymph node dissection coverage might be suitable for IPA (15, 16). However, to distinguish pre-IPA from IPA lesions is difficult in the absence of complete preoperative histological sampling, which limits optimal treatment planning (17). Therefore, an effective preoperative risk prediction model is needed to predict IPA risk.
Numerous prediction models, including the most well-known Mayo model, the Brock University model, the Peking University People’s (PKUPH) model, the VA model, and others, have been developed to date for SPN diagnosis. Over 80% of these models have demonstrated diagnostic accuracy. Every model, in the meantime, has flaws of its own and requires more optimization.
A nomogram is a reliable tool for creating simple visual graphs of statistical predictive models to quantify the risk of clinical events such as cancer (18, 19). The high incidence of lung adenocarcinoma prompted us to develop a risk prediction model to differentiate IPA from pre-IPA in patients with isolated lung nodules and to establish a nomogram combining CT and clinical features to determine IPA risk in patients with SPNs to support clinicians’ treatment recommendations.
2 Materials and methods
2.1 Patient selection
The Ethics Committee of Qilu Hospital, Shandong University approved this single-center study (registration number: KYLL-202008–023-1) and waived the need for written informed consent due to its retrospective design. All procedures complied with the principles enshrined in the Declaration of Helsinki (2013 amendment).
This study included patients with small SPNs with clear pathology who underwent minimally invasive pulmonary resection between January 2020 and December 2021 at the Department of Thoracic Surgery, Qilu Hospital, Shandong University. Inclusion criteria comprised: a single intrapulmonary nodule suggested by chest CT within 1 month before surgery, SPN diameter ≤ 20 mm, absent pulmonary atelectasis and active lung inflammation, surgical resection to obtain definitive pathological findings. Asymptomatic at diagnosis, and no preoperative treatment. Exclusion criteria comprised age < 18 years, open thoracic surgery, incomplete perioperative data, history of malignant disease within 5 years, and metastatic tumors. All those who met the criteria were randomly assigned using a random split sample method to training and validation cohorts in a 7:3 ratio to respectively develop and verify the performance of a prediction nomogram.
2.2 Data collection and variable definitions
We downloaded the following information about the patients from the Qilu Hospital database: demographic data: sex, age, smoking history, body mass index (BMI), preoperative comorbidities (hypertension, diabetes, and chronic obstructive pulmonary disease); preoperative assessment outcomes (American Society of Anesthesiologists scores, % predicted forced expiratory volume in one second, % predicted maximal voluntary ventilation), laboratory blood findings [blood type, blood sugar, serum complement C1q, serum 5’-nucleotidase, lactate dehydrogenase, serum amyloid, albumin, neutrophils, lymphocytes, basophils, eosinophils, monocytes, erythrocytes, hemoglobin, platelets, prognostic nutritional index (PNI), neutrophil-to-lymphocyte ratio (NLR), platelet-to-lymphocyte ratio (PLR), monocyte-to-lymphocyte ratio (MLR), derived neutrophil-to-lymphocyte ratio (dNLR), neutrophil-to-lymphocyte and platelet ratio (NLPR), aggregate index of systemic inflammation (AISI), systemic inflammatory response syndrome (SIRS), systemic inflammation index (SII), pan-immune-inflammation value (PIV)]; lung cancer tumor markers (cytokeratin 19-fragments, squamous cell carcinoma antigen, pro-gastrin-releasing peptide, carcinoembryonic antigen (CEA), carcinoma antigen 125, and neuron-specific enolase); imaging features (shape regular or irregular), location (central or peripheral), spiculation (sunburst appearance), calcification, pleural adhesions, lobulation, cavitation, vascular penetration, lymph node enlargement, bronchus and, pleural effusion signs, maximum tumor diameter, consolidation-to-tumor ratio (CTR) and pathology data: postoperative pathological malignant SPN ≤ 2 cm).
AISI, [(neutrophils × monocytes × platelets)/lymphocytes].
dNLR, [neutrophils/(leukocytes - neutrophils)].
MLR, monocytes/lymphocytes.
NLPR, [Neutrophils/(lymphocytes × platelets)].
NLR, neutrophils/lymphocytes.
PIV, [(neutrophils × platelets × monocytes)/lymphocytes].
PLR, platelets/lymphocytes.
PNI, serum albumin (g/L) + 5×total lymphocyte count (×109/L).
SII, [(neutrophils x platelets)/lymphocytes)].
SIRI, [(neutrophils × monocytes)/lymphocytes)].
The study’s blood collection time was standardised, and on the morning of the second hospital day, all patients had their blood drawn while fasting and in a peaceful state. Results from blood tests were obtained for each patient no later than one week before to surgery.
All scans were performed with Iopromide injection 300 contrast enhancement from the base to the apex of the lung using either a 64-slice multi-detector CT (Aquilion 64; Toshiba Medical Systems) or a 16-slice multi-detector CT (Somatom Definition AS, Siemens Healthcare, Erlangen, Germany). The patients were lying supine when the scans were obtained at the conclusion of inspiration. The scanning parameters were 50 mA, 1 mm collimation, 1.5:1 pitch, and 120 kVp. With filtered back projection, a 2 mm slice thickness, and a 2 mm increment, the data were recreated using a smooth convolution kernel (Siemens B30f or Toshiba FC02). Computed tomography images of the entire chest during deep inspiration and breath-holding were acquired from supine patients. Two radiologists with > 5 years of experience in chest radiology independently measured each imaging feature, and another with >20 years of experience in chest radiology reassessed discrepancies. Disagreements were resolved by consensus. Centrality was defined nodules in the bronchi, lobar bronchi, or lung segmental bronchi. Peripheral location was defined as nodules found below the tertiary bronchus. Spiculation was defined as the spread of strands from the nodal margins into the lung parenchyma without contacting the pleural surface. Calcification signs on CT images were defined as stratification, central nodule, bronchi, diffusion, or popcorn. Cavitation signs were defined as gas-filled spaces that are considered as transparent or low-attenuation regions. Vascular penetration was assumed when a pulmonary artery crossed a node. Pleural adhesion was defined as linear attenuation of the pleura or a major or minor fissure from the SPN. The bronchial sign indicated direct bronchial involvement of the nodules. Lobulation was defined as a wavy or fan-shaped portion of the lesion surface, with strands extending from the nodal margins into the lung parenchyma. Pleural effusion was defined as blunting of the rib-diaphragm angle. Mediastinal lymph node enlargement was noted. The CTR is the ratio of the diameter of the solid component of a lung nodule to its maximum diameter.
All pathological specimens were fixed in formalin, stained with hematoxylin and eosin, and histologically evaluated by two experienced lung pathologists using a light microscope. All specimens were categorized according to the International Association for the Study of Lung Cancer/American Thoracic Society/European Respiratory Society classification of lung adenocarcinoma (20).
We assigned patients with SPN diameters ≤ 2 cm to pre-IPA and IPA groups. The pre-IPA group included patients with AAH, AIS, MIA, and benign lesions.
2.3 Statistical analysis
All data were statistically analyzed using SPSS (version 26.0; IBM Corp., Armonk, NY, USA). Normally distributed continuous variables are expressed as means ± standard deviation (SD) and compared using Student t-tests. Non-normally distributed continuous variables are expressed as medians with interquartile ranges (IQRs) and two groups were compared using Mann-Whitney U tests. Categorical variables were compared using Pearson chi-square or Fisher exact tests. The statistical significance of differences was defined at P < 0.05. All risk factors affecting the probability of IPA in the training cohort were evaluated using univariate analysis, then all those with p < 0.05 in were included in multivariate logistic regression analysis using R statistical software (Windows version 4.2.1, http://www.r-project.org/). A predictive model for SPN was constructed based on the results of multiple logistic regression analyses. The area under the receiver operating characteristic (ROC) curves (AUC) was determined. Scores for each variable were calculated using a regression model, and the predictive probability of IPA was derived by adding the scores for each variable. Nomograms were built and calibration curves were generated using the regression modeling strategies (rms) package in R. The ROC curves were plotted using the pROC package in R.
2.4 Nomogram performance
The performance of the predictive nomogram was assessed based on discriminatory power, calibration, and clinical utility. The ability of a model to correctly distinguish between events and non-events is called discrimination. to We evaluated the recognition efficiency of the predictive nomograms using ROC curves (21). Calibration measures the extent to which the predicted probabilities matched the actual results. We assessed calibration capability using Hosmer–Lemeshow tests with p > 0.05 indicating satisfactory calibration (22). A nomogram map was created to further evaluate the calibration. Internal verification proceeded by bootstrapping samples1,000 times (23). The clinical effectiveness of the predictive nomograms was evaluated based on the net benefit of different threshold probabilities using decision curve analysis (24). The optimal cutoff value was determined when the Youden index (sensitivity + specificity-1was maximal based on the results of ROC curve analyses of the training cohort.
3 Results
3.1 Characteristics of the patients
2213 original patients who had surgery at our institution between January 2020 and December 2021 were included in our research. The initial patients were not chosen; they were all sequential. Following a series of screening steps, 1,106 suitable patients were eventually enrolled in our research. Figure 1 shows the process of identifying and selecting 1,106 eligible patients, among whom 163, 188, 233, and 522 had benign nodules and AAH, AIS, MIA, and IPA, respectively. All patients were assigned to pre-IPA (n = 584) and IPA (n = 522) groups based on nodule invasiveness. The patients were then randomly assigned to training (n = 776) or validation (n = 330) cohort at a 7:3 ratio. with No variables significantly differed between the cohorts (Table 1). The training cohort comprised 406 and 370 patients with pre-IPA and IPA nodules and the validation cohort comprised 178 and 152 patients with pre-IPA and IPA nodules, respectively. Table 2 shows he characteristics of the patients in the training and validation groups.
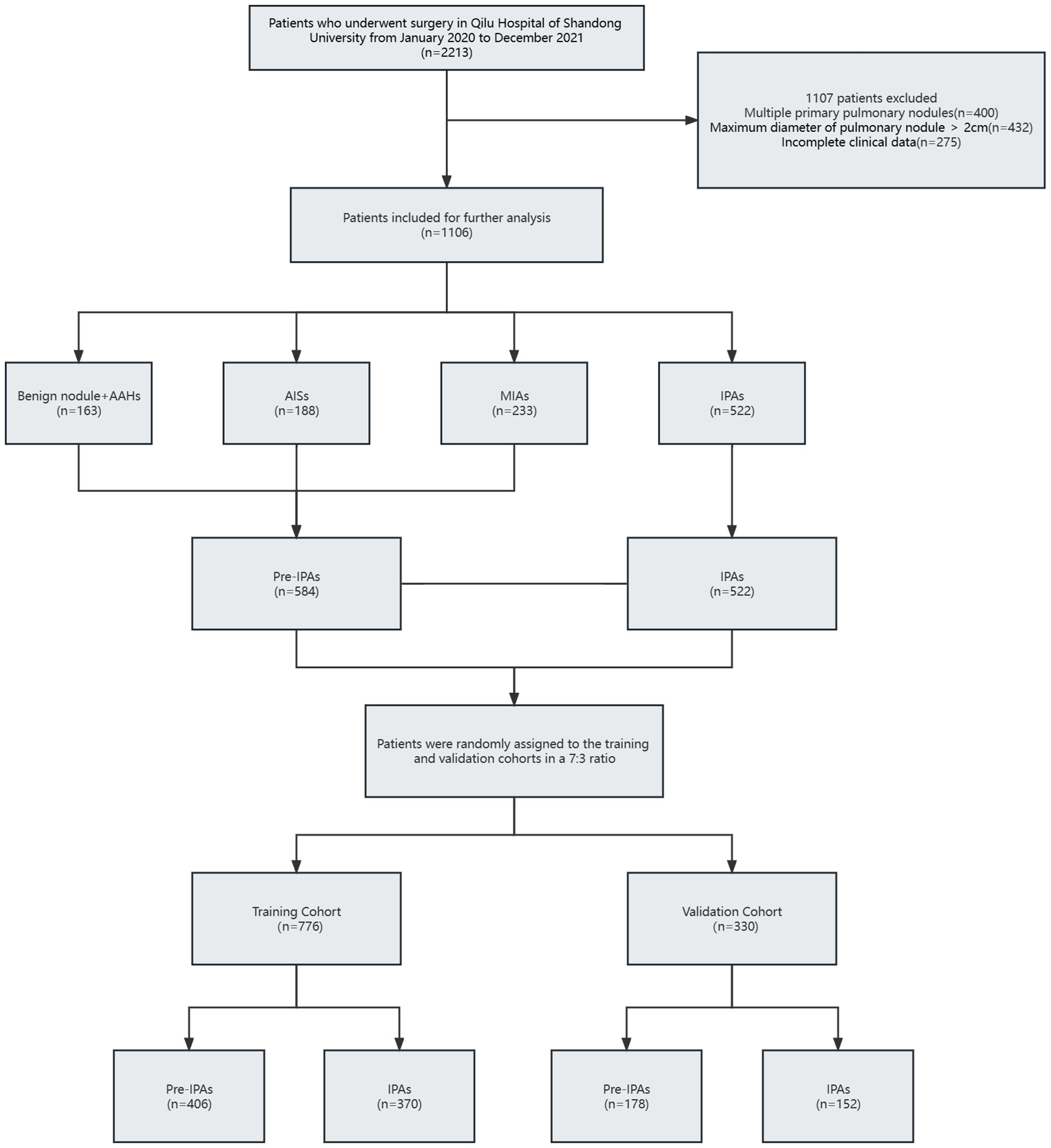
Figure 1 Flow diagram of patient selection process. AAH, atypical adenomatous hyperplasia; AIS, adenocarcinoma in situ; IPA, invasive pulmonary adenocarcinoma.; MIA, microinvasive adenocarcinoma.
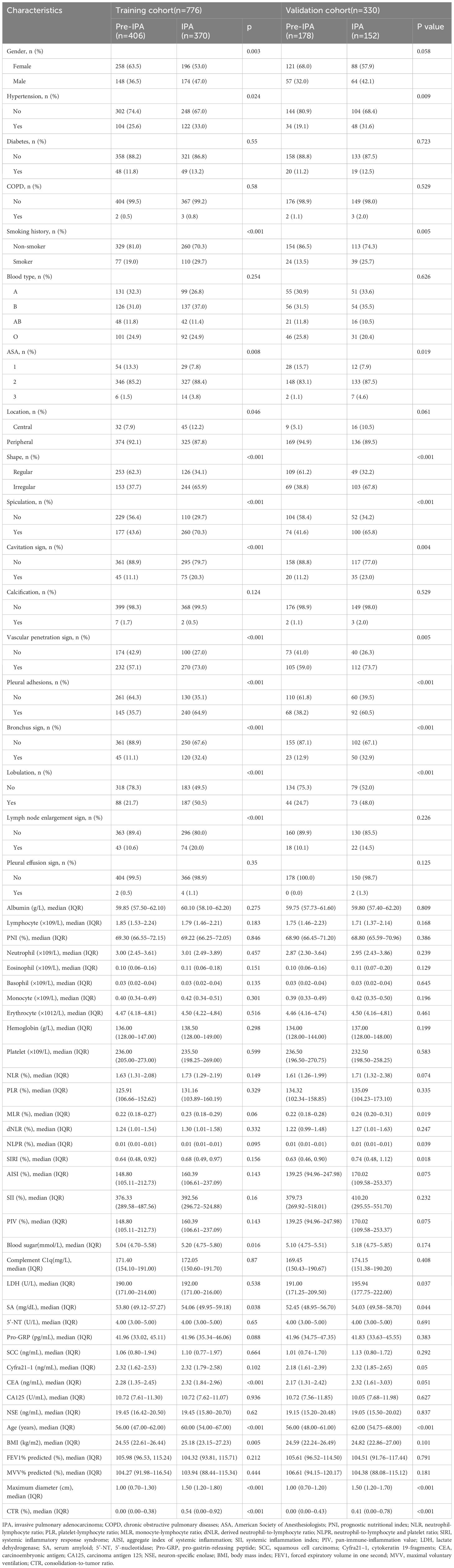
Table 2 Clinical characteristics of patients with IPA and pre-IPA in the training and validation cohorts.
3.2 Identification of risk factors for SPN aggressiveness measuring ≤ 2 cm
We identified independent risk factors for IPA in the training cohort using univariate and multivariate logistic regression analyses (Table 3) Univariate analysis revealed 21 potential risk factors for IPA within 2 cm (p < 0.05). These 21 factors (p < 0.05) were further analyzed using multivariate logistic regression, and the following indicators were selected: age (odds ratio [OR)], 1.030; 95% confidence interval [CI], 1.009–1.052; p = 0.005); CEA (OR,1.267; 95% CI, 1.097–1.486; p = 0.002); bronchus involvement (yes vs. no; OR, 1.802; 95% CI, 1.103–2.972; p = 0.02); lobulation (yes vs. no; OR, 1.772; 95% CI, 1.167–2.696; p = 0.007); pleural adhesions (yes vs. no; OR, 1.813; 95% CI, 1.250–2.632; p = 0.002); maximum tumor diameter (OR, 7.848; 95% CI: 4.834–13.003; p < 0.001); and CTR (OR, 1.644; 95% CI, 1.011–2.671; p = 0.045; Figure 2, forest plot).
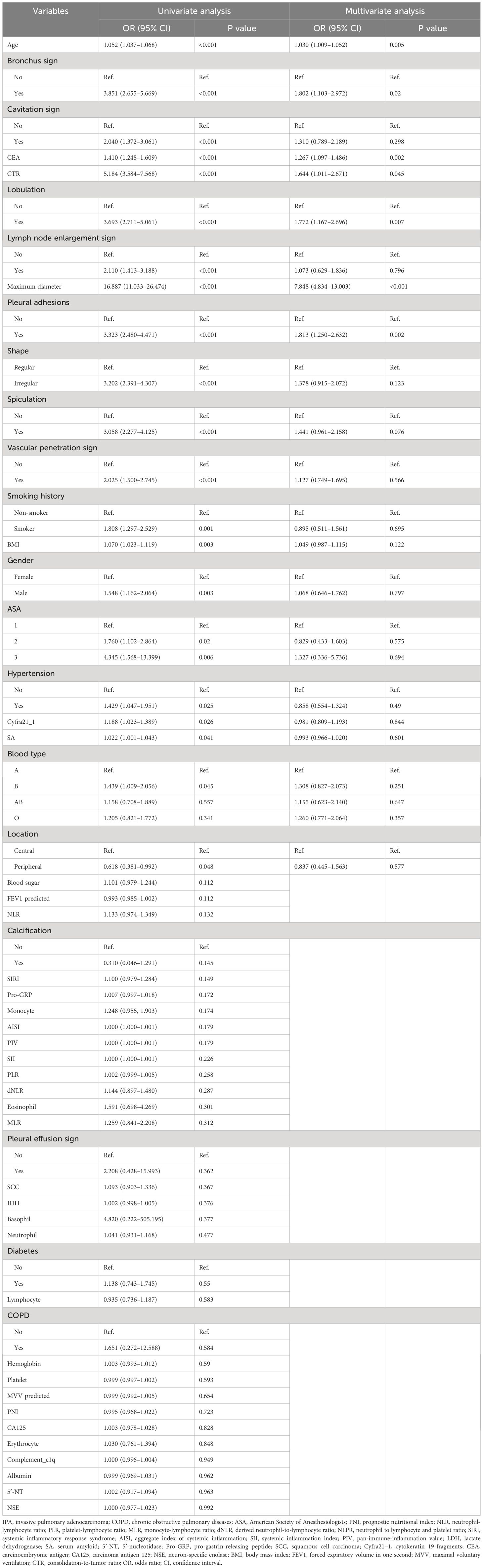
Table 3 Univariate and multivariate logistic regression analysis of IPA factors of SPN ≤2 cm in the training cohort.
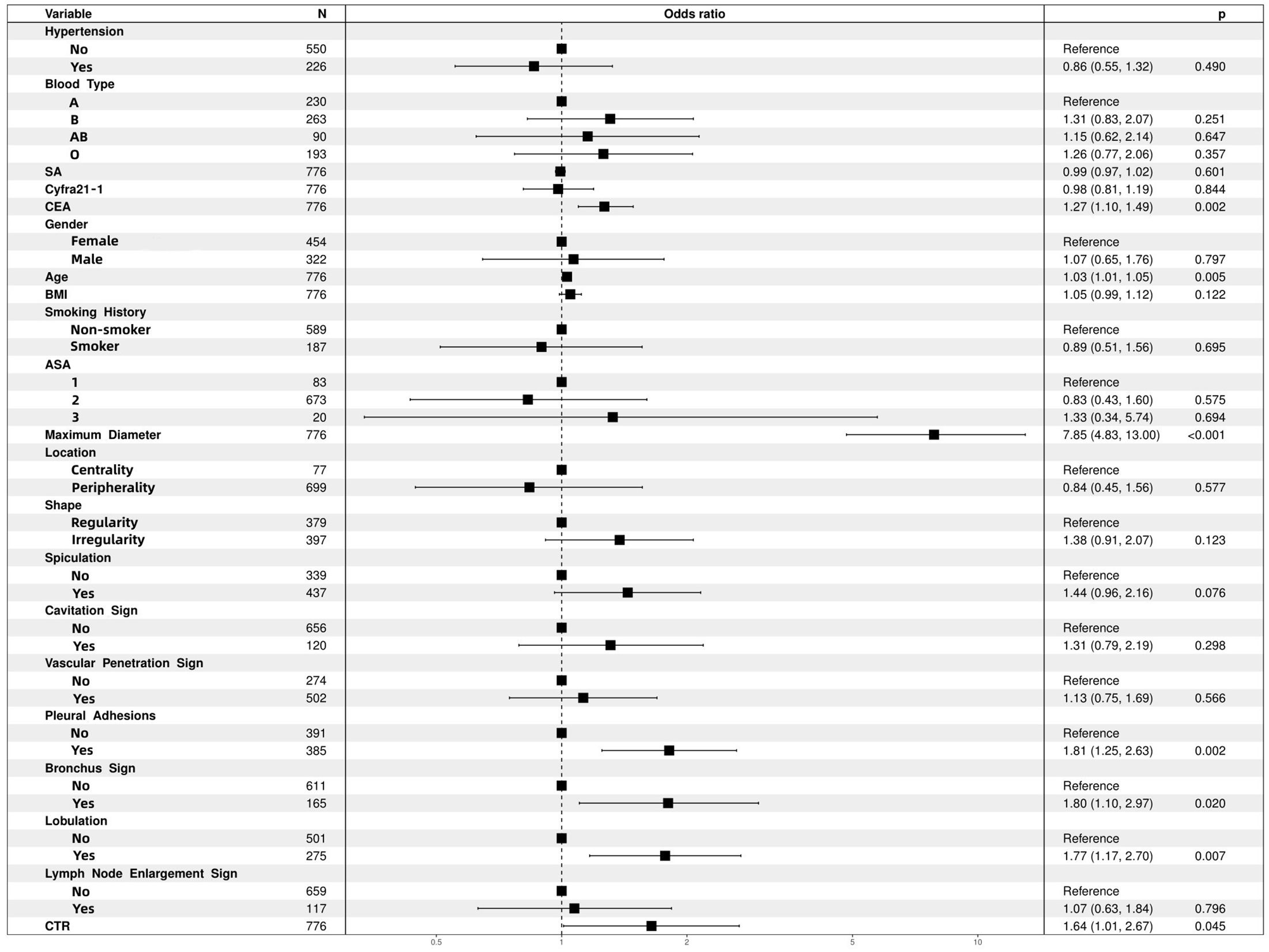
Figure 2 Multifactor logistic regression analysis of forest plots. ASA, American Society of Anesthesiologists; BMI, body mass index; CEA, carcinoembryonic antigen; CTR, consolidation-to-tumor ratio; SA, serum amyloid.
3.3 Nomogram construction
The seven independent risk factors were modeled using logistic regression. Table 4 shows details of the predictive models. Thus, invasiveness of SPN ≤ 2 cm was predicted as follows:
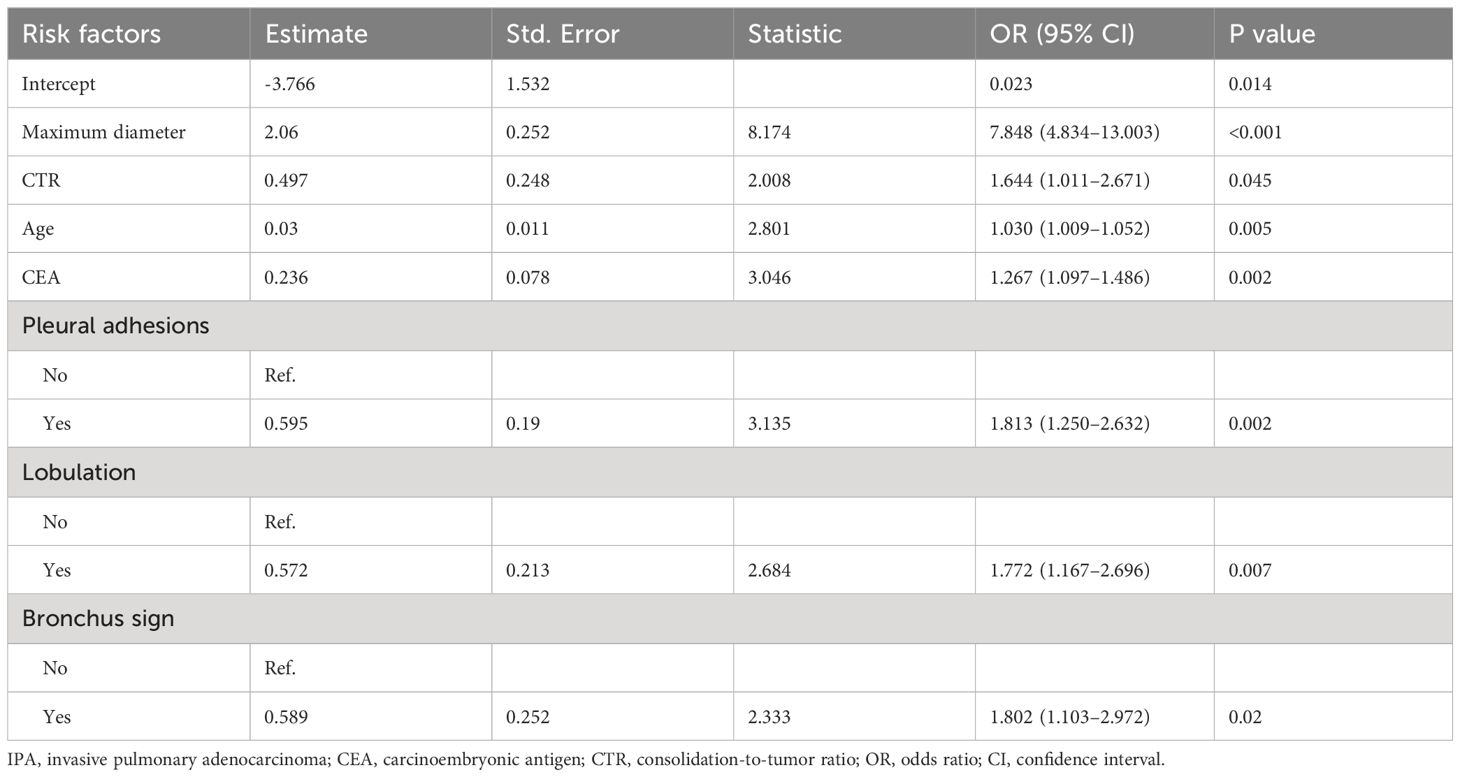
Table 4 Details of the predictive model used to calculate the probability of IPA for SPN measuring ≤2 cm in diameter.
Predicted IPA = ex/(1 + ex), x = 2.06 × maximum diameter + 0.497 × CTR + 0.03×age + 0.236 × CEA + 0.595 × pleural adhesions (no = 0; yes = 1) + 0.572 × lobulation (no = 0; yes = 1) + 0.589 × bronchus sign (no = 0; yes = 1)-3.766.
Where e is the natural logarithmic base, e = 2.718 281 828, and x is the logistic regression coefficient. The units of maximum diameter, age, and CEA are cm, years, and ng/mL, respectively.
Based on the coefficients of the multiple logistic regression model, a nomogram predicting the IPA of SPN ≤ 2 cm was drawn using the rms package in R (Figure 3). This nomogram comprised 10 axes, of which axes 2–8 represent the seven variables in the prediction model. By plotting a line perpendicular to the highest point axis, the estimated score for each risk factor was calculated and summed to obtain the total score. The total point axis was used to predict the probability of preoperative IPA of SPNs measuring ≤ 2 cm. An appropriate surgical method could then be selected.
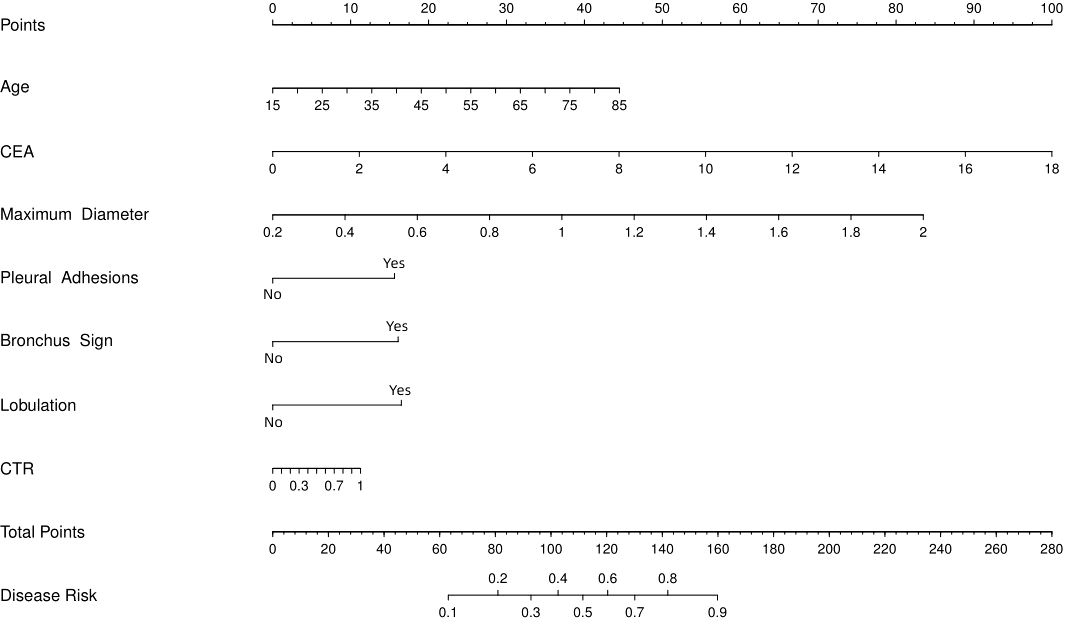
Figure 3 Nomogram to predict probability of IPA for SPN ≤ 2 cm. CEA, carcinoembryonic antigen; CTR, consolidation-to-tumor ratio.
3.4 Predictive performance and validation of the nomogram
We assessed the discriminative power of the prediction model and nomogram using ROC curves. The AUCs of the ROC curve were 0.844 (95% CI, 0.817–0.871 and 0.812 (95% CI: 0.766–0.857) for the training and validation cohorts, respectively, indicating that the predictive accuracy of the nomogram was relatively good (Figure 4). Nevertheless, overfitting might have caused the high AUC values. The ROC curve truncation value for the training cohort was 0.432, with sensitivity and specificity of 0.803 and 0.724, respectively (Figure 5; Table 5).
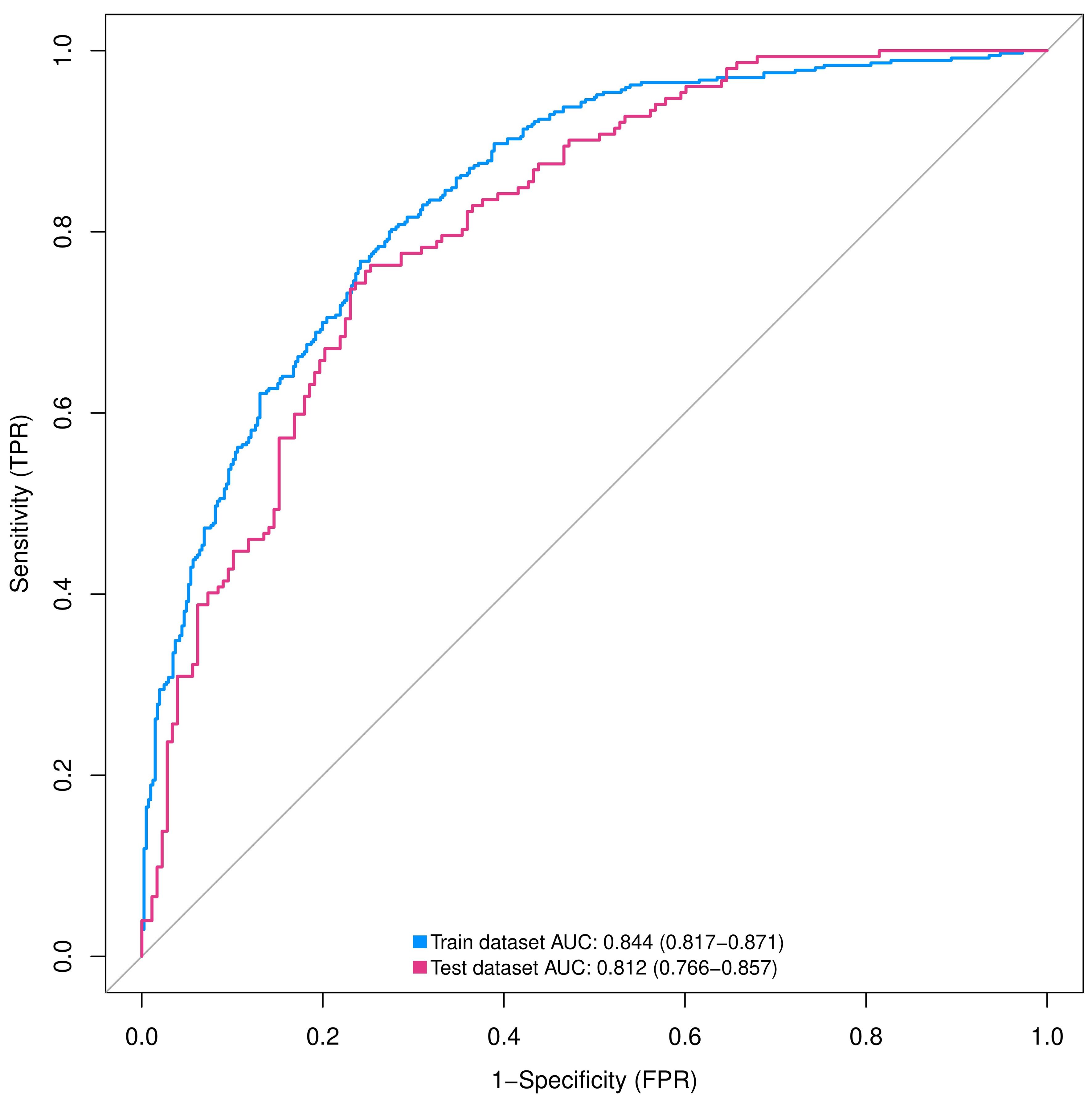
Figure 4 ROC curves of nomograms predicting IPA in training and validation groups. AUC, area under the ROC curve; ROC, receiver operating characteristic; SPN, solitary pulmonary nodule.
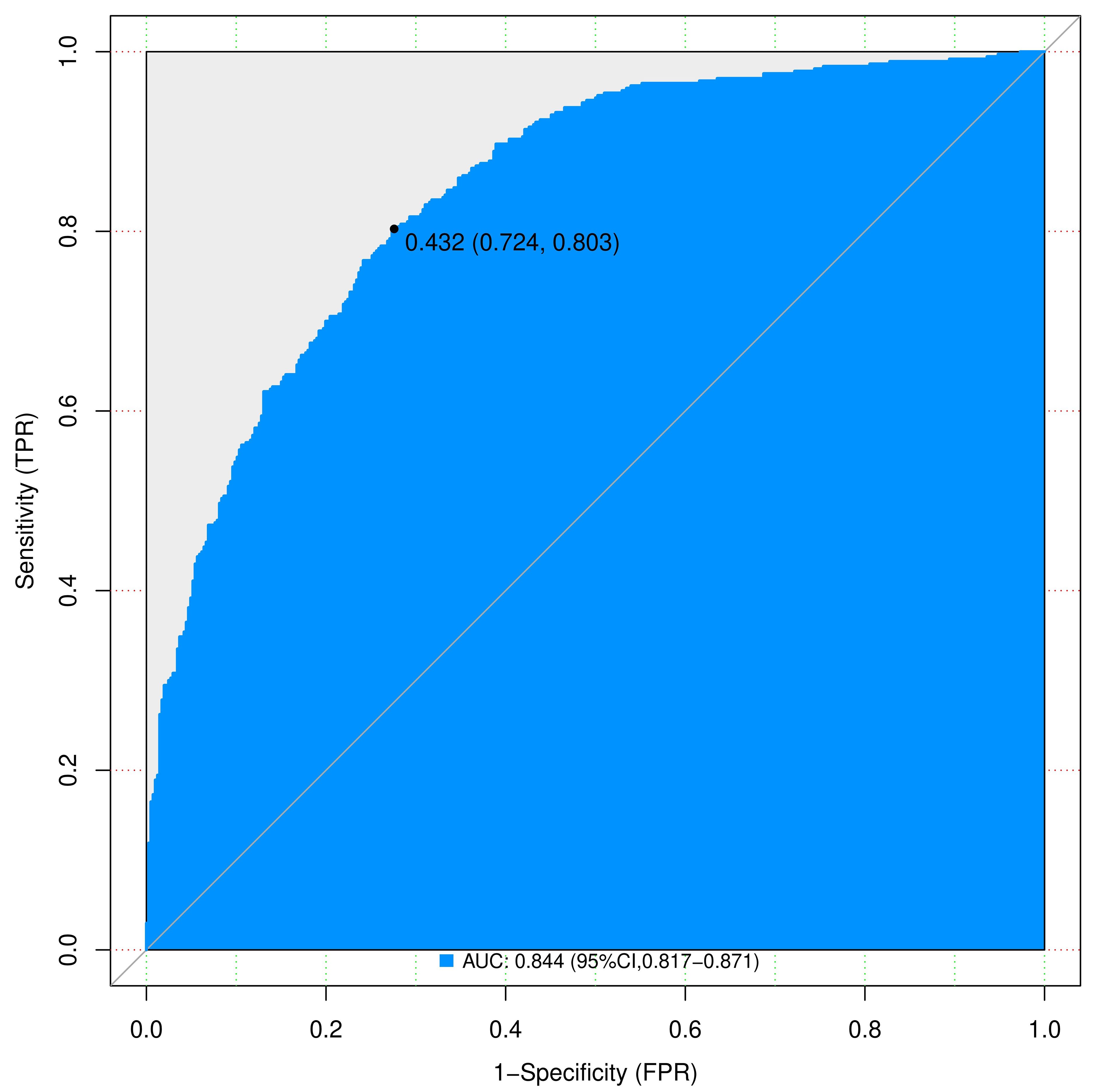
Figure 5 Complex ROC curves for nomograms to predicting IPA of SPN ≤ 2 cm in the training cohort. AUC, area under the ROC curve; ROC, receiver operating characteristic; SPN, solitary pulmonary nodule.
Calibration power was evaluated using Hosmer-Lemeshow tests and calibration plots. The values for p in the Hosmer-Lemeshow test were 0.068 and 0.290 in the training and validation cohorts, respectively, indicating no significant differences between the predicted and actual probabilities. Good calibration of the predicted nomogram was also supported by calibration plots of the training (Figure 6A) and validation (Figure 6B) cohorts. The bias-corrected C-indices in the training and validation cohorts were 0.844 and 0.814, respectively.
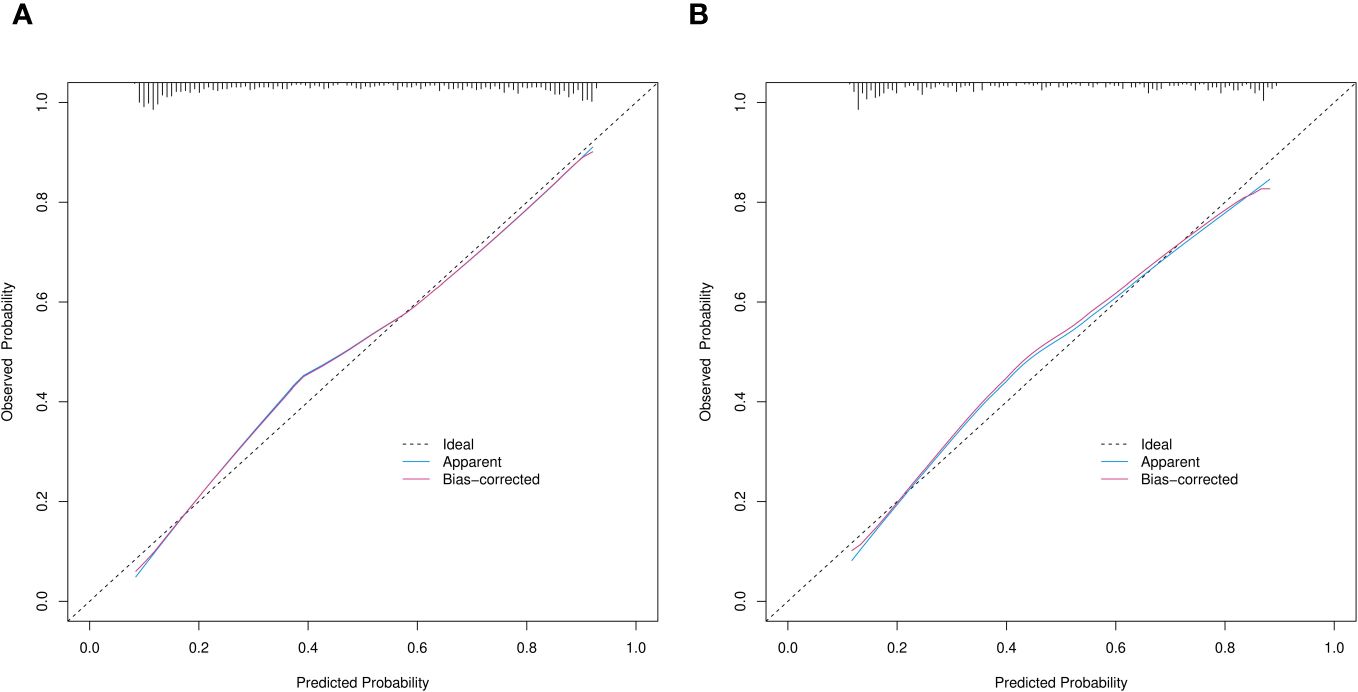
Figure 6 Calibration curves of prediction nomogram in training (A) and validation (B) cohorts. X and y axes respectively represent probability predicted by nomogram and actual probability of SPN ≤ 2 cm being IPA. Black dashed, blue and red solid lines, ideal, apparent (uncorrected), and deviation (corrected) curves the bootstrap method (B = 1,000 samplings). SPN, solitary pulmonary nodule.
3.5 Clinical utility of the predictive nomogram
We assessed the clinical utility of the nomograms using decision curve analysis. The nomograms in Figures 7A, B, provided greater net benefit and broader threshold probabilities for predicting the risk of IPA of SPN ≤ 2 cm in diameter in the training and validation cohorts, indicating that nomograms were clinically useful. We also created clinical impact curves (Figure 8) to enable surgeons to make better clinical decisions.
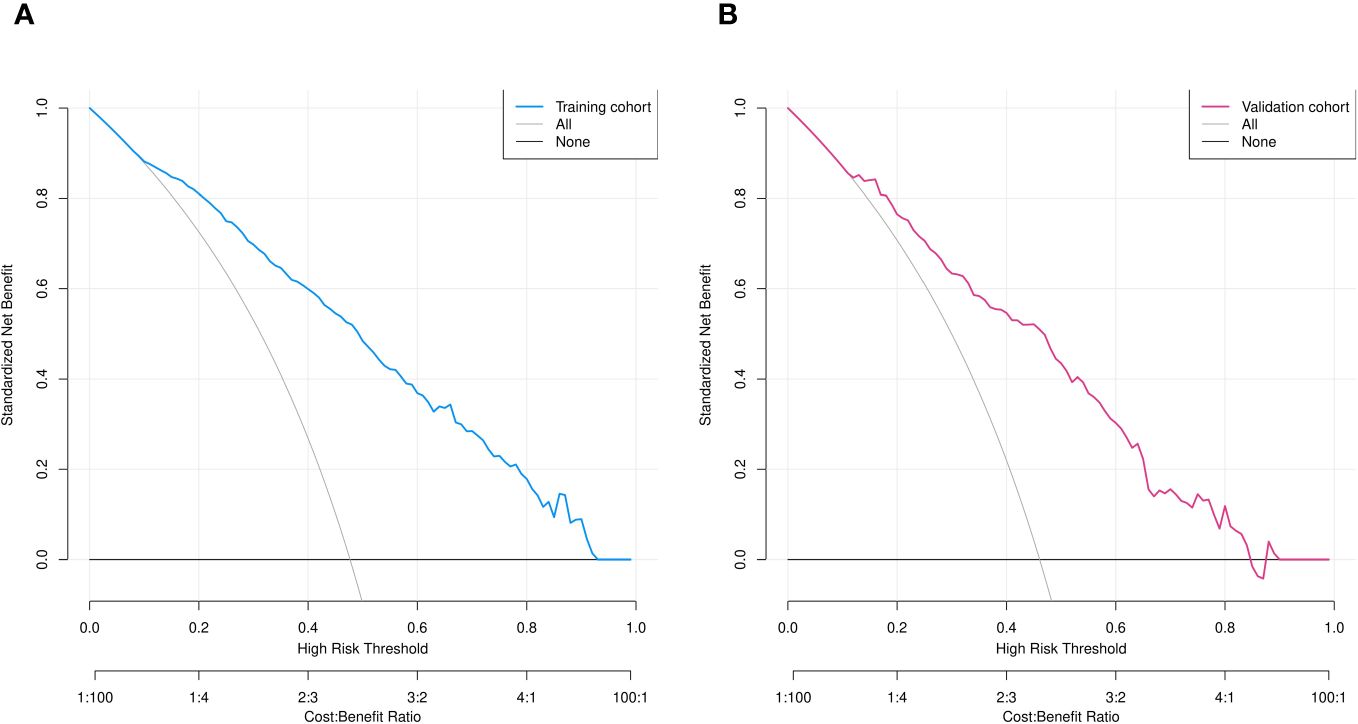
Figure 7 Decision curve analysis of predicted nomogram in training (A) and validation (B) cohorts. Y axis, net gain; black and grey lines, hypotheses that SPNs with diameter ≤ 2 cm are pre-IPA in nature and that SPNs ≤2 cm in diameter are IPAs. respectively. Blue (A) and red (B) lines, training and validation cohorts, respectively.
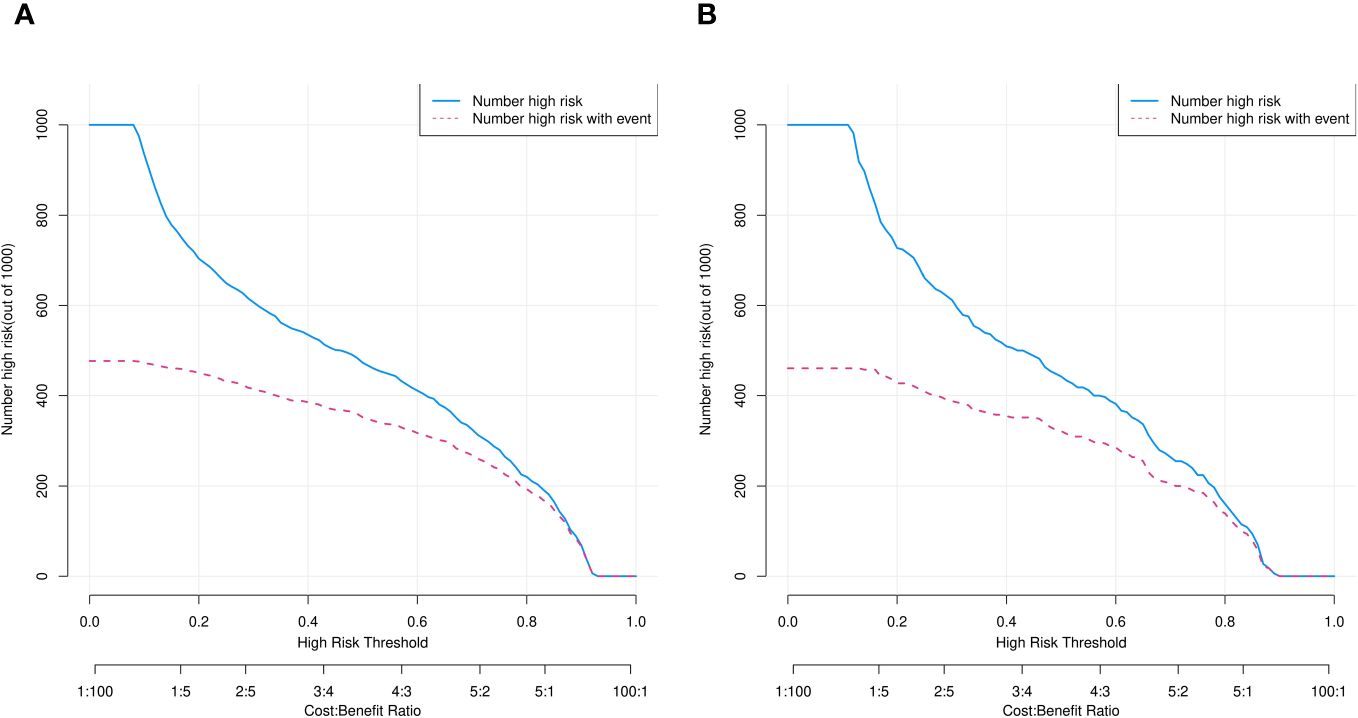
Figure 8 Clinical impact curves of predicted nomogram in training (A) and validation (B) cohorts. Horizontal and vertical coordinates, probability threshold and numbers of people, respectively. Numbers of individuals with SPNs judged by model as IPAs (blue line) and as IPAs and nodal true IPAs (red line) at different probability thresholds. Bottom, loss-to-benefit ratio at different probability thresholds.
4 Discussion
Optimal management of patients with SPN is a growing clinical concern. Pathological IPA of persistent SPNs is important to assess because clinical management strategies for pre-IPA and IPA lesions are variable. We developed a clinical prediction model and visual diagnostic nomogram for individualized preoperative prediction of IPA of SPN with diameters ≤ 2 cm by retrospectively analyzing the hematological indices, imaging characteristics, and general clinical information of 776 patients in the training cohort. We identified age, CEA values, bronchial signs, lobulation, pleural adhesions, maximum tumor diameter, and CTRs as independent predictors of IPA. Our nomogram predicted patient-specific IPA probability with excellent discrimination and outstanding calibration.
Age is an important clinical factor. The capacity of cells to renew and repair epithelial damage caused by carcinogens decreases, whereas tumor malignancy increases with advancing age (25–27). Although we found that age correlated with IPA, it was the least influential factor.
Carcinoembryonic antigen is a polysaccharide protein complex involved in cell adhesion, which is usually absent or minimal in healthy adult blood and it might be linked to the poor prognosis of tumors (28). Elevated serum CEA levels are significant predictive markers of early relapse (29), progression (30), and treatment outcomes. Our findings showed that CEA can predict the IPA of SPN, which was consistent with these previous studies.
Lobular signs are more prevalent in invasive than pre-infiltrative lesions (31). Bronchial changes can predict IPA (32). These morphological features are associated with active fibroblast proliferation in adenocarcinomas and are caused by fibrous tissue contraction (33). This has been confirmed by others, suggesting that activated fibroblast proliferation in adenocarcinoma is associated with aggressive tumor growth (34). In addition, the insignificance of spiculations here might be attributable to their low abundance. Subpleural nodules or tumors in contact with the visceral pleura or linear clouding, which is vertical and intersects the visceral pleura, might result in pleural adhesion (35). Pleural adhesions are associated with tumor invasiveness and a poor prognosis (36–38). Our findings suggested that lobar, bronchial, and pleural adhesions are more likely features of invasive lung adenocarcinoma.
The size of nodules increases in parallel as lung adenocarcinoma becomes more invasive (39, 40). Our findings confirmed this. Moreover, the maximum nodule diameter was the most influential factor for IPA in the present study.
The CTR is an imaging feature of small lung adenocarcinomas and is the ratio of the diameter of solid tumors to that of the total tumor (41–43). It is an established radiological parameter used to identify pathologically noninvasive tumors on CT images (43, 44). We found that the CTR positively correlated with IPA. Thus, a higher proportion of solid components is associated with more invasive SPNs.
We used data from Qilu Hospital to develop and validate a new predictive model and clinical prediction nomogram that can help thoracic surgeons use preoperative information to assess risk of IPA in patients with SPNs. Patients with high scores underwent curative lobectomy, whereas those with low scores underwent sublobar resection. Consequently, modeling to distinguish between IPA and pre-IPA in patients with SPNs can improve their management and prognosis.
The PKUPH model was said to be better than conventional models, whereas the Mayo model was the most often used model for predicting malignant SPN. A more precise forecasting technique based on CT scans and descriptions of clinical data is the Brock model. Nevertheless, clinical indicators were not incorporated in these models. Chinese mainland populations are not a good fit for foreign prediction models. Certain prediction models integrate more complex and quantitative imaging data into their evaluations, such as tumor diameter growth rates and CT attenuation. However, due to their difficulty in obtaining, conducting, and standardising, these imaging data are rarely recognised and utilised by physicians. Unlike previous studies (45), we introduced benign tumors and combined them with the pre-IPA group. This grouping method is useful for predicting the prognosis of patients and it has value in guiding clinical decisions. This is because the possibility of benign tumors cannot be completely excluded from clinical SPNs. Moreover, we incorporated basic clinical patient information, imaging features, and hematological findings to establish a clinical prediction model with comprehensive preoperative information. The combination of preoperative clinical predictive results and rapid intraoperative pathological findings allows accurate and safe realization of nodal aggressiveness and the development of treatment strategies that are specific for individual patients. In cases where predictive modelling suggested, before surgery, that there was a high likelihood the nodule was invasive, we operated on the patient and performed a lobectomy. If preoperative predictive modelling suggested that the nodule was likely non-invasive, we performed a sublobar excision to maintain the patient’s lung function. Each patient therefore receives a customised diagnosis and course of care.
This study had several limitations. We included only patients who underwent surgical resection in our department. Those who did not undergo surgical resection were excluded, which represents selection bias. The subjectivity of radiologists might have led to different judgments of the CT images of pulmonary nodules. Our model was limited by the retrospective design of study. Our data were derived from a single center with a relatively small sample size. The predictive model has only been validated internally, and further validation involving multiple centers and sufficient samples are needed. Although the validation of the model showed good discriminatory and calibration capabilities, the generalizability of nomograms to new patient populations remains a major issue. However, the nomogram requires further external validation.
5 Conclusions
We developed and validated a novel and easy-to-use nomogram for predicting the risk of IPA in patients with SPN ≤ 2 cm in diameter. With excellent differentiation and calibration, clinicians and surgeons can accurately develop specific treatment strategies for each patient.
Data availability statement
The raw data supporting the conclusions of this article will be made available by the authors, without undue reservation.
Ethics statement
The Ethics Committee of Qilu Hospital, Shandong University approved this single-center study (registration number: KYLL-202008-023-1). The studies were conducted in accordance with the local legislation and institutional requirements. Written informed consent for participation was not required from the participants or the participants’ legal guardians/next of kin in accordance with the national legislation and institutional requirements.
Author contributions
MX: Writing – review & editing, Writing – original draft, Visualization, Validation, Supervision, Software, Resources, Project administration, Methodology, Investigation, Formal analysis, Data curation, Conceptualization. RL: Writing – original draft, Visualization, Supervision, Software, Resources, Project administration, Methodology, Investigation, Formal analysis. JL: Writing – review & editing, Resources, Project administration, Methodology, Investigation. ML: Writing – original draft, Visualization, Validation, Supervision, Software, Resources, Project administration, Methodology, Investigation, Formal analysis, Data curation, Conceptualization. ZL: Writing – review & editing, Funding acquisition, Formal analysis, Data curation, Conceptualization. HZ: Writing – review & editing, Resources, Project administration, Methodology, Investigation. HT: Writing – review & editing, Writing – original draft, Visualization, Validation, Supervision, Software, Resources, Project administration, Methodology, Investigation, Funding acquisition, Formal analysis, Data curation, Conceptualization.
Funding
The author(s) declare financial support was received for the research, authorship, and/or publication of this article. This work was funded by National Key Research and Development Program (2021YFC2500904, and 2021YFC2500905) and Natural Science Foundation of Shandong Province (ZR2021LSW006).
Acknowledgments
We would like to thank Editage for English language editing. We thank the imaging physicians and pathologists at Qilu Hospital for their contributions to this study.
Conflict of interest
The authors declare that the research was conducted in the absence of any commercial or financial relationships that could be construed as a potential conflict of interest.
Publisher’s note
All claims expressed in this article are solely those of the authors and do not necessarily represent those of their affiliated organizations, or those of the publisher, the editors and the reviewers. Any product that may be evaluated in this article, or claim that may be made by its manufacturer, is not guaranteed or endorsed by the publisher.
Supplementary material
The Supplementary Material for this article can be found online at: https://www.frontiersin.org/articles/10.3389/fonc.2024.1334504/full#supplementary-material
References
1. She Y, Zhao L, Dai C, Ren Y, Jiang G, Xie H, et al. Development and validation of a nomogram to estimate the pretest probability of cancer in Chinese patients with solid solitary pulmonary nodules: A multi-institutional study. J Surg Oncol. (2017) 116:756–62. doi: 10.1002/jso.24704
2. Yankelevitz DF, Henschke CI. Small solitary pulmonary nodules. Radiol Clin North Am. (2000) 38:471–8. doi: 10.1016/S0033-8389(05)70177-9
3. Khan AN, Al-Jahdali HH, Irion KL, Arabi M, Koteyar SS. Solitary pulmonary nodule: A diagnostic algorithm in the light of current imaging technique. Avicenna J Med. (2011) 1:39–51. doi: 10.4103/2231-0770.90915
4. Choromańska A, Macura KJ. Evaluation of solitary pulmonary nodule detected during computed tomography examination. Pol J Radiol. (2012) 77:22–34. doi: 10.12659/PJR.882967
5. Horeweg N, van Rosmalen J, Heuvelmans MA, van der Aalst CM, Vliegenthart R, Scholten ET, et al. Lung cancer probability in patients with CT-detected pulmonary nodules: a prespecified analysis of data from the NELSON trial of low-dose CT screening. Lancet Oncol. (2014) 15:1332–41. doi: 10.1016/S1470-2045(14)70389-4
6. Kuhn E, Morbini P, Cancellieri A, Damiani S, Cavazza A, Comin CE. Adenocarcinoma classification: patterns and prognosis. Pathologica. (2018) 110:5–11.
7. Wu YJ, Liu YC, Liao CY, Tang EK, Wu FZ. A comparative study to evaluate CT-based semantic and radiomic features in preoperative diagnosis of invasive pulmonary adenocarcinomas manifesting as subsolid nodules. Sci Rep. (2021) 11:66. doi: 10.1038/s41598-020-79690-4
8. Chen X, Feng B, Chen Y, Liu K, Li K, Duan X, et al. A CT-based radiomics nomogram for prediction of lung adenocarcinomas and granulomatous lesions in patient with solitary sub-centimeter solid nodules. Cancer Imaging. (2020) 20:45. doi: 10.1186/s40644-020-00320-3
9. Tang EK, Chen CS, Wu CC, Wu MT, Yang TL, Liang HL, et al. Natural history of persistent pulmonary subsolid nodules: long-term observation of different interval growth. Heart Lung Circ. (2019) 28:1747–54. doi: 10.1016/j.hlc.2018.08.015
10. Hsu HT, Tang EK, Wu MT, Wu CC, Liang CH, Chen CS, et al. Modified lung-RADS improves performance of screening LDCT in a population with high prevalence of non-smoking-related lung cancer. Acad Radiol. (2018) 25:1240–51. doi: 10.1016/j.acra.2018.01.012
11. Wu FZ, Chen PA, Wu CC, Kuo PL, Tsao SP, Chien CC, et al. Semiquantative visual assessment of sub-solid pulmonary nodules ≦3 cm in differentiation of lung adenocarcinoma spectrum. Sci Rep. (2017) 7:15790. doi: 10.1038/s41598-017-16042-9
12. Wu FZ, Huang YL, Wu CC, Tang EK, Chen CS, Mar GY, et al. Assessment of selection criteria for low-dose lung screening CT among asian ethnic groups in Taiwan: from mass screening to specific risk-based screening for non-smoker lung cancer. Clin Lung Cancer. (2016) 17:e45–56. doi: 10.1016/j.cllc.2016.03.004
13. Travis WD, Brambilla E, Nicholson AG, Yatabe Y, Austin JHM, Beasley MB, et al. The 2015 world health organization classification of lung tumors: impact of genetic, clinical and radiologic advances since the 2004 classification. J Thorac Oncol. (2015) 10:1243–60. doi: 10.1097/JTO.00000000000000630
14. Travis WD, Brambilla E, Noguchi M, Nicholson AG, Geisinger KR, Yatabe Y, et al. International association for the study of lung cancer/american thoracic society/european respiratory society international multidisciplinary classification of lung adenocarcinoma. J Thorac Oncol. (2011) 6:244–85. doi: 10.1097/JTO.0b013e318206a221
15. Liu S, Wang R, Zhang Y, Li Y, Cheng C, Pan Y, et al. Precise diagnosis of intraoperative frozen section is an effective method to guide resection strategy for peripheral small-sized lung adenocarcinoma. J Clin Oncol. (2016) 34:307–13. doi: 10.1200/JCO.2015.63.4907
16. Kodama K, Higashiyama M, Takami K, Oda K, Okami J, Maeda J, et al. Treatment strategy for patients with small peripheral lung lesion(s): intermediate-term results of prospective study. Eur J Cardiothorac Surg. (2008) 34:1068–74. doi: 10.1016/j.ejcts.2008.07.044
17. Van Schil PE, Asamura H, Rusch VW, Mitsudomi T, Tsuboi M, Brambilla E, et al. Surgical implications of the new IASLC/ATS/ERS adenocarcinoma classification. Eur Respir J. (2012) 39:478–86. doi: 10.1183/09031936.00027511
18. Zhang Y, Sun Y, Xiang J, Zhang Y, Hu H, Chen H. A clinicopathologic prediction model for postoperative recurrence in stage Ia non-small cell lung cancer. J Thorac Cardiovasc Surg. (2014) 148:1193–9. doi: 10.1016/j.jtcvs.2014.02.064
19. Liang W, Zhang L, Jiang G, Wang Q, Liu L, Liu D, et al. Development and validation of a nomogram for predicting survival in patients with resected non-small-cell lung cancer. J Clin Oncol. (2015) 33:861–9. doi: 10.1200/JCO.2014.56.6661
20. Travis WD, Brambilla E, Noguchi M, Nicholson AG, Geisinger K, Yatabe Y, et al. International Association for the Study of Lung Cancer/American Thoracic Society/European Respiratory Society: international multidisciplinary classification of lung adenocarcinoma: executive summary. Proc Am Thorac Soc. (2011) 8:381–5. doi: 10.1513/pats.201107-042ST
21. Obuchowski NA, Bullen JA. Receiver operating characteristic (ROC) curves: review of methods with applications in diagnostic medicine. Phys Med Biol. (2018) 63:07tr1. doi: 10.1088/1361-6560/aab4b1
22. Nattino G, Pennell ML, Lemeshow S. Assessing the goodness of fit of logistic regression models in large samples: A modification of the Hosmer-Lemeshow test. Biometrics. (2020) 76:549–60. doi: 10.1111/biom.13249
23. Rosenfeld JP, Donchin E. Resampling (bootstrapping) the mean: A definite do. Psychophysiology. (2015) 52:969–72. doi: 10.1111/psyp.12421
24. Vickers AJ, Holland F. Decision curve analysis to evaluate the clinical benefit of prediction models. Spine J. (2021) 21:1643–8. doi: 10.1016/j.spinee.2021.02.024
25. MacMahon H, Austin JH, Gamsu G, Herold CJ, Jett JR, Naidich DP, et al. Guidelines for management of small pulmonary nodules detected on CT scans: a statement from the Fleischner Society. Radiology. (2005) 237:395–400. doi: 10.1148/radiol.2372041887
26. MacMahon H, Naidich DP, Goo JM, Lee KS, Leung ANC, Mayo JR, et al. Guidelines for management of incidental pulmonary nodules detected on CT images: from the fleischner society 2017. Radiology. (2017) 284:228–43. doi: 10.1148/radiol.2017161659
27. Swensen SJ, Silverstein MD, Edell ES, Trastek VF, Aughenbaugh GL, Ilstrup DM, et al. Solitary pulmonary nodules: clinical prediction model versus physicians. Mayo Clin Proc. (1999) 74:319–29. doi: 10.4065/74.4.319
28. Grunnet M, Sorensen JB. Carcinoembryonic antigen (CEA) as tumor marker in lung cancer. Lung Cancer. (2012) 76:138–43. doi: 10.1016/j.lungcan.2011.11.012
29. Sakao Y, Tomimitsu S, Takeda Y, Natsuaki M, Itoh T. Carcinoembryonic antigen as a predictive factor for postoperative tumor relapse in early-stage lung adenocarcinoma. Eur J Cardiothorac Surg. (2004) 25:520–2. doi: 10.1016/j.ejcts.2004.01.029
30. Kashiwabara K, Saeki S, Sasaki J, Nomura M, Kohrogi H. Combined evaluation of postoperative serum levels of carcinoembryonic antigen less than or equal to 2.5 ng/ml and absence of vascular invasion may predict no recurrence of stage I adenocarcinoma lung cancer. J Thorac Oncol. (2008) 3:1416–20. doi: 10.1097/JTO.0b013e31818dda85
31. Chu ZG, Li WJ, Fu BJ, Lv FJ. CT characteristics for predicting invasiveness in pulmonary pure ground-glass nodules. AJR Am J Roentgenol. (2020) 215:351–8. doi: 10.2214/AJR.19.22381
32. Zhang Y, Qiang JW, Shen Y, Ye JD, Zhang J, Zhu L. Using air bronchograms on multi-detector CT to predict the invasiveness of small lung adenocarcinoma. Eur J Radiol. (2016) 85:571–7. doi: 10.1016/j.ejrad.2015.12.014
33. Sakurai H, Maeshima A, Watanabe S, Suzuki K, Tsuchiya R, Maeshima AM, et al. Grade of stromal invasion in small adenocarcinoma of the lung: histopathological minimal invasion and prognosis. Am J Surg Pathol. (2004) 28:198–206. doi: 10.1097/00000478-200402000-00007
34. Noguchi M, Morikawa A, Kawasaki M, Matsuno Y, Yamada T, Hirohashi S, et al. Small adenocarcinoma of the lung. Histologic characteristics and prognosis. Cancer. (1995) 75:2844–52. doi: 10.1002/1097-0142(19950615)75:12<2844::AID-CNCR2820751209>3.0.CO;2-#
35. Han J, Xiang H, Ridley WE, Ridley LJ. Pleural tail sign: pleural tags. J Med Imaging Radiat Oncol. (2018) 62 Suppl 1:37. doi: 10.1111/1754-9485.24_12785
36. Kim HJ, Cho JY, Lee YJ, Park JS, Cho YJ, Yoon HI, et al. Clinical significance of pleural attachment and indentation of subsolid nodule lung cancer. Cancer Res Treat. (2019) 51:1540–8. doi: 10.4143/crt.2019.057
37. Meniga IN, Tiljak MK, Ivankovic D, Aleric I, Zekan M, Hrabac P, et al. Prognostic value of computed tomography morphologic characteristics in stage I non-small-cell lung cancer. Clin Lung Cancer. (2010) 11:98–104. doi: 10.3816/CLC.2010.n.013
38. Moon Y, Sung SW, Lee KY, Sim SB, Park JK. Pure ground-glass opacity on chest computed tomography: predictive factors for invasive adenocarcinoma. J Thorac Dis. (2016) 8:1561–70. doi: 10.21037/jtd
39. Jones KD. Whence lepidic?: the history of a Canadian neologism. Arch Pathol Lab Med. (2013) 137:1822–4. doi: 10.5858/arpa.2013-0144-HP
40. Wu F, Tian SP, Jin X, Jing R, Yang YQ, Jin M, et al. CT and histopathologic characteristics of lung adenocarcinoma with pure ground-glass nodules 10 mm or less in diameter. Eur Radiol. (2017) 27:4037–43. doi: 10.1007/s00330-017-4829-5
41. Aberle DR, Adams AM, Berg CD, Black WC, Clapp JD, Fagerstrom RM, et al. Reduced lung-cancer mortality with low-dose computed tomographic screening. N Engl J Med. (2011) 365:395–409. doi: 10.1056/NEJMoa1102873
42. Aokage K, Yoshida J, Ishii G, Matsumura Y, Haruki T, Hishida T, et al. Identification of early t1b lung adenocarcinoma based on thin-section computed tomography findings. J Thorac Oncol. (2013) 8:1289–94. doi: 10.1097/JTO.0b013e31829f6d3b
43. Hattori A, Matsunaga T, Takamochi K, Oh S, Suzuki K. Importance of ground glass opacity component in clinical stage IA radiologic invasive lung cancer. Ann Thorac Surg. (2017) 104:313–20. doi: 10.1016/j.athoracsur.2017.01.076
44. Eguchi T, Kadota K, Park BJ, Travis WD, Jones DR, Adusumilli PS. The new IASLC-ATS-ERS lung adenocarcinoma classification: what the surgeon should know. Semin Thorac Cardiovasc Surg. (2014) 26:210–22. doi: 10.1053/j.semtcvs.2014.09.002
Keywords: solitary pulmonary nodules, diagnosis, prediction, logical model, invasive pulmonary adenocarcinoma
Citation: Xue M, Li R, Liu J, Lu M, Li Z, Zhang H and Tian H (2024) Nomogram for predicting invasive lung adenocarcinoma in small solitary pulmonary nodules. Front. Oncol. 14:1334504. doi: 10.3389/fonc.2024.1334504
Received: 07 November 2023; Accepted: 10 June 2024;
Published: 01 July 2024.
Edited by:
Giuseppe Cardillo, San Camillo Forlanini Hospital, ItalyReviewed by:
Yuting Ke, Massachusetts Institute of Technology, United StatesCui Youbin, First Affiliated Hospital of Jilin University, China
Copyright © 2024 Xue, Li, Liu, Lu, Li, Zhang and Tian. This is an open-access article distributed under the terms of the Creative Commons Attribution License (CC BY). The use, distribution or reproduction in other forums is permitted, provided the original author(s) and the copyright owner(s) are credited and that the original publication in this journal is cited, in accordance with accepted academic practice. No use, distribution or reproduction is permitted which does not comply with these terms.
*Correspondence: Hui Tian, tianhuiql@email.sdu.edu.cn