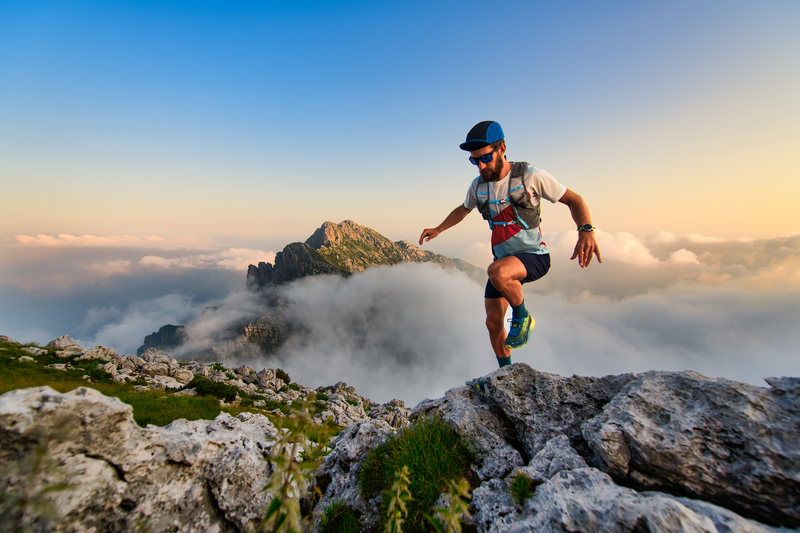
94% of researchers rate our articles as excellent or good
Learn more about the work of our research integrity team to safeguard the quality of each article we publish.
Find out more
REVIEW article
Front. Oncol. , 07 February 2024
Sec. Cancer Metabolism
Volume 14 - 2024 | https://doi.org/10.3389/fonc.2024.1331215
Liquid biopsy, a novel detection method, has recently become an active research area in clinical cancer owing to its unique advantages. Studies on circulating free DNA, circulating tumor cells, and exosomes obtained by liquid biopsy have shown great advances and they have entered clinical practice as new cancer biomarkers. The metabolism of the body is dynamic as cancer originates and progresses. Metabolic abnormalities caused by cancer can be detected in the blood, sputum, urine, and other biological fluids via systemic or local circulation. A considerable number of recent studies have focused on the roles of metabolic molecules in cancer. The purpose of this review is to provide an overview of metabolic markers from various biological fluids in the latest clinical studies, which may contribute to cancer screening and diagnosis, differentiation of cancer typing, grading and staging, and prediction of therapeutic response and prognosis.
The occurrence and development of cancer is an ever-evolving process that challenges tissue biopsy as the current gold standard for cancer diagnosis (1, 2). Compared to tissue biopsy, liquid biopsy has the advantages of non-invasiveness, high sensitivity, and repeatable sampling, which makes it a promising approach to overcome cancer heterogeneity and realize dynamic monitoring of cancer (3, 4). The exploration of circulating free DNA (5), circulating tumor cells (6), and exosomes (7) is moving from basic research to clinical applications. The initial success of liquid biopsy has also accelerated extensive research into various forms of potential biomarkers (3, 8), such as metabolites, tumor-educated platelets, immune cell components, and proteins.
Metabolic competition poses new challenges for the growth and survival of cancer cells. For one thing, cancer cells have to alter their metabolic fluxes to gain a competitive advantage in the tumor microenvironment (TME) and meet their biosynthetic and energetic requirements. For another, the abnormal accumulation of metabolites in the TME makes it necessary for cancer cells to metabolically adapt to protect themselves from harm. As a result, there is a growing appreciation for alterations in metabolites and their metabolic pathways in cancer. Metabolomics is the scientific discipline that studies the dynamics of metabolites and their mechanisms in organisms under physiological and pathological conditions (9). As a favorable complement to genomics, transcriptomics, and proteomics, the initial value of metabolomics is as a tool for identifying disease biomarkers, as changes in metabolites are regulated by upstream signaling molecules (10). Concurrently, changes in the “quality” and “quantity” of metabolic molecules will in turn affect the replication and expression of genes. Certain metabolites tend to change earlier than genes and proteins, which can reflect fluctuations in the body state in a more timely and effective manner (11). In a sense, metabolomics is also making itself noticed as a “commander.” Metabolomics can be classified into non-targeted and targeted metabolomics based on the study’s purpose. The former can provide a wide range of differential metabolite profiles, whereas the latter focuses on the absolute quantification of a specific set of metabolites (11). The two most frequently used analytical techniques in metabolomics are nuclear magnetic resonance (NMR) and mass spectrometry (MS) (12, 13). Combined MS technologies, such as liquid chromatography-mass spectrometry (LC-MS) and gas chromatography-mass spectrometry (GC-MS), are increasingly used (11, 13).
The clinical applications of metabolomics have been largely restricted to metabolic imaging (14, 15). However, the recent emergence of liquid biopsy has sparked interest in the potential role of circulating metabolic molecules, opening new avenues for cancer biomarker discovery (Figure 1). There is an ongoing search for biomarkers in circulating body fluids, mostly from blood and urine, using metabolomics. Owing to the pathophysiological characteristics of different cancers, options for biological sample types are increasingly diverse. A small number of studies have focused on metabolites from other biological samples such as sputum, cerebrospinal fluid and pleural effusion. Herein, we summarize the latest metabolic findings based on liquid biopsy in cancer population studies, intending to identify potential metabolic markers that could aid in clinical cancer diagnosis, classification, and treatment. Finally, we discuss the current limitations and future directions in the field of liquid biopsy-based cancer metabolomic biomarker research.
Figure 1 Workflow of metabolomics-based liquid biopsy in cancer. After collecting samples from various biofluids, metabolites can be detected by NMR and/or MS. Differential metabolites are then obtained through metabolomic data analysis, which can be used as biomarkers for accurate cancer diagnosis and classification as well as prognosis prediction. NMR, nuclear magnetic resonance; MS, mass spectrometry.
The early clinical symptoms of cancer are hidden, and most patients are in the middle and advanced stages once diagnosed, resulting in the loss of opportunities for radical surgery. Therefore, the key to prolonging the survival of patients with cancer is to identify high-risk groups and achieve an early diagnosis. In the search for potential metabolic markers, numerous metabolomic studies have recently compared the metabolic differences in biofluids between patients with cancer and healthy individuals or patients with benign lesions. In this section, we screen differential metabolites mentioned in at least three previous studies (Table 1) and outline how these metabolites change in patients with cancer.
Glucose is a vital nutrient that tumor cells compete whose uptake depends largely on glucose transporters (GLUTs) on the cell membranes. There are four classical GLUTs in the human body, among which GLUT1 and GLUT3 have high glucose affinities and are upregulated in multiple tumor types (80). Significant differences in circulating glucose levels between cancer-free controls and patients with cancer have been reported in recent studies (16–18). Using GC-MS techniques, two studies detected relatively low levels of glucose in the plasma of patients with diffuse large B-cell lymphoma and in urine from patients with prostate cancer (17, 18), which may potentially result from increased glucose uptake by tumor cells from the peripheral circulation. In contrast, Nizioł et al. observed higher glucose concentrations in patients with renal cancer than in healthy controls using MRI spectroscopy (16), which appears to contradict the theory of active glucose metabolism in tumor tissues. Considering the characteristics of renal blood flow regulation, it is more likely a stress glucose elevation caused by sympathetic excitation during renal ischemia (81). Taken together, these metabolomic findings suggest that changes in glucose levels in biological fluids may help in the early identification of patients with cancer.
Pyruvate synthesis relies primarily on the glycolytic pathway, and the conversion of glucose to glucose-6-phosphate catalyzed by hexokinase is the first rate-limiting step. KRAS mutation has been reported to directly regulate the enzymatic activity of hexokinase 1, thereby increasing glucose utilization by tumor cells (82). Overexpression of phosphofructokinases and pyruvate kinases in tumor tissues has also been confirmed to be involved in tumor growth and invasion (83, 84). Several studies have consistently found that patients with cancer show relatively high levels of pyruvate in the blood and urine. In addition to decreased glucose levels, Lima et al. observed a marked increase in urinary pyruvate levels in patients with prostate cancer (18). In another study, by combining differential metabolites obtained by MS with a metabolic network based on Kyoto Encyclopedia of Genes and Genomes database, Guo et al. constructed a metabolite panel (including pyruvate) specifically for non-small cell lung cancer (NSCLC) with a diagnostic accuracy of almost 98% (19). Subsequently, by applying the NMR technique, Ye et al. proposed that the combination of pyruvate and acetate presented significantly superior diagnostic performance compared to carbohydrate antigen 199 (CA199) and carcinoembryonic antigen (CEA) and could serve as a candidate marker for the diagnosis of esophageal cancer (20).
Aerobic glycolysis, known as the “Warburg effect,” is a classic example of metabolic adaptation in tumor cells; even under aerobic conditions, glucose in tumor cells is not completely oxidized but is catabolized to lactic acid, which provides a large carbon source for tumor cells while rapidly generating adenosine triphosphate (85, 86). Lactic acid transport relies on monocarboxylic acid transporters (MCTs), in which abnormal expression of MCT1 and MCT4 in different tumor types affects intracellular and extracellular lactic acid homeostasis, thereby participating in distant metastasis and recurrence (87, 88). To explore the serum metabolic profile of osteosarcoma, Lv et al. applied non-targeted metabolomics techniques to identify the metabolites that differ between patients with cancer and healthy individuals. The area under the curve (AUC) of lactic acid was 0.97 according to receiver operating characteristic (ROC) analysis (21). Using benign lesions as controls, Sato et al. demonstrated that urine lactic acid levels were significantly elevated in patients with renal cell carcinoma, which was consistent with their previous metabolomic analysis based on tumor tissue samples (22). Conversely, another metabolomic study of kidney cancer reported lower serum lactic acid levels in patients with cancer (16). Although the phenomenon discussed in the above two studies requires further confirmation, variations in inclusion criteria for controls across studies may influence the alteration of lactate levels in blood and urine.
Inositol is a class of cyclic sugar alcohols with nine isomer forms that are widely distributed throughout the body, among which myoinositol is the most stable. In addition to dietary intake, myoinositol can be synthesized in vivo by inositol-3-phosphate synthase 1 (ISYNA1) and inositol monophosphatase catalysis (89). Zhou and colleagues found a positive correlation between ISYNA1 expression and the survival of patients with pancreatic cancer, and observed increased invasiveness in ISYNA1-silenced pancreatic cancer cells (90). However, ISYNA1 plays the opposite role in bladder cancer, and its knockdown significantly promotes apoptosis of bladder cancer cells (91). Three studies reported increased myoinositol levels in prostate cancer (24), renal cell carcinoma (22) and pancreatic cancer (26), whereas two studies reported decreased myoinositol levels in kidney cancer (16) and lung cancer (25). These studies have confirmed the effectiveness of metabolic panels, including myoinositol, in the early diagnosis of cancer.
Glutamine is a crucial nutrient for sustaining cell proliferation and survival, especially in tumor cells. Solute carrier family 1 member 5 is a key protein responsible for glutamine transport, and its upregulation in tumor tissues indicates increased glutamine uptake (92). Glutamate is the breakdown product of glutamine, catalyzed by glutaminase, which can be further converted to α-ketoglutarate (α-KG) by glutamate dehydrogenase or aminotransferase. On the one hand, α-KG entering the tricarboxylic acid (TCA) cycle replenishes the intermediate products of aerobic oxidation and promotes the production of adenosine triphosphate. On the other hand, α-KG can also further enter the fatty acid synthesis pathway by converting to citric acid, which is more pronounced in the hypoxic TME (93, 94). In addition, it has been demonstrated that regulatory activation of glutaminases promotes tumor progression by producing more glutathione to assist tumor cells in resisting oxidative stress (95). Numerous studies have reported changes in glutamine and glutamate levels in the blood and urine of cancer patients, and suggested that these two metabolites could be used as potential metabolic markers to effectively distinguish cancer patients from non-cancerous individuals. Seven studies found higher levels of glutamine (21, 22, 27–31) and three studies observed lower glutamine levels in patients with cancer (32, 35, 36). These findings may be attributed to enhanced glutamine catabolism, which produces more glutamate. It is worth mentioning that in a study on esophageal squamous cell carcinoma, the authors found serum glutamine and glutamate levels were significantly higher in cancer patients compared to healthy controls (31). While only glutamate showed a statistically significant difference when patients with dysplasia were used as controls, this may indicate that glutamate and glutamine metabolism dynamically change during cancer progression. However, in two other studies based on serum metabolomics, an increase in glutamine and a decrease in glutamate levels were observed in ovarian and prostate cancers, respectively (23, 33). This implies that reproductive system tumors are likely to be less dependent on glutamine metabolism. Similarly, one study found significantly lower blood glutamate levels in patients with colon cancer than in those with colon adenomas (34), although more work is needed to verify the glutamate differences between patients with colon cancer and healthy individuals.
BCAAs refer to three non-essential amino acids, namely leucine, isoleucine, and valine, which are derived from exogenous food intake and endogenous tissue protein breakdown. The upregulation of L-type amino acid transporter 1, induced by hypoxia-inducible factor in the TME can promote more BCAAs into cells (96). The catabolism of BCAAs mediated by amino acid branched-chain transaminases is abnormally active in pancreatic malignancies (97, 98). Based on serum metabolomics, two studies observed reduced leucine levels in renal tumors and small cell lung cancer (SCLC), respectively (16, 29). Consistent with these findings, patients with pancreatic ductal adenocarcinoma also had reduced levels of two isomers of leucine: isoleucine and ortholeucine (39). Decreased levels of BCAAs have also been detected in saliva samples from patients with cancer, including oral squamous cell carcinoma, lung cancer and thyroid cancer (38, 41, 42), implying vigorous catabolism of BCAAs in cancer cells to meet the energy requirements of biological macromolecules. However, urinary metabolomics showed the opposite trend. Two studies found that patients with prostate cancer have higher levels of BCAAs in their urine than controls (18, 37). Based on MS and binary logistic regression analysis methods, Huang’s team observed a decline in urinary isoleucine in patients with gastric cancer and constructed an early diagnostic model by combining differential metabolites with age (40). It is worth noting that considering the effect of age on human metabolism, taking age into account somewhat improved the reliability of the diagnostic model in this study.
One-carbon metabolism not only provides raw materials for nucleotide synthesis and methylation reactions in tumor cells but also participates in maintaining cellular redox homeostasis, which is an important component of metabolic reprogramming in tumor cells (99).
Serine and glycine are the main sources of one-carbon units for tumor cells. In the serine-restricted TME, tumor cells can regulate the expression of phosphoglycerate dehydrogenase, allowing more 3-phosphoglycerate to enter the serine synthesis pathway (100). Published studies regarding the variation of serine content in biofluids are inconsistent. Yang et al. proposed a diagnostic model of metabolites containing serine for early-stage lung cancer with an AUC of more than 95% (35). Similarly, an MS study on prostate cancer demonstrated that differential serum metabolites (L-serine, myoinositol and decanoic acid) performed better than prostate specific antigen in distinguishing patients with prostate cancer from benign prostate hyperplasia (24). Both studies confirmed higher serine levels in patients with cancer whereas serum glycine and serine levels were inversely correlated with the risk of endometrial cancer in an observational study (47). Further studies are needed to determine whether these two metabolites are directly involved in the pathological process of endometrial cancer. Moreover, there is a notable disparity in serine levels in urine and sputum samples from individuals with cancer compared to those without cancer (40, 41). The conversion of serine to glycine requires catalysis by serine-hydroxymethyltransferase, an important target for the regulation of lung cancer metastasis (101). Several studies have consistently found relatively low levels of glycine in the circulating body fluids of cancer patients (41, 47, 48).
Tryptophan catabolism can also produce one-carbon units. Abnormalities in tryptophan metabolism in tumor cells have been extensively studied and metabolites involved in tryptophan catabolism, such as kynurenine, have emerged as targets for anti-tumor therapy (102). Alterations in tryptophan levels in the biological fluids of lung cancer patients have been observed in three studies, two of which found that patients with NSCLC had elevated blood tryptophan levels compared to healthy controls (19, 43). In contrast, a study based on sputum metabolomics observed the opposite trend in patients with lung cancer (46), and the difference in the distribution of tryptophan in blood and sputum may be the result of increased tryptophan consumption by tumor cells. In addition to lung cancer, there are significant changes in circulating blood tryptophan levels in colorectal, pancreatic, and bladder cancer (32, 44, 45).
Methionine can provide methyl groups for the synthesis of various bioactive substances, such as carnitine, choline, and creatine, through transmethylation reactions; therefore, there is intense competition for methionine in the TME. High expression of methionine transporters in tumor cells results in a stronger methionine uptake capacity than that of CD8+ T cells, thereby limiting the anti-tumor function and survival of CD8+ T cells (103). Changes in blood methionine levels have shown significance in the diagnosis of lung cancer, although they have an opposite trend in SCLC and NSCLC (19, 29), suggesting metabolic heterogeneity between the different pathological types of lung cancer. Besides, increased methionine and spermidine levels were observed in patients with oral squamous cell carcinoma in a salivary metabolomics study (38).
The transsulfuration pathway refers to the synthesis progress of cystine by serine and homocysteine under the catalysis of cystathionine β-synthase and cystathionine-γ-lyase, which is the main source of cystine synthesis in vivo. Cystathionine-γ-lyase is the key enzyme in this process and its high expression in tumor tissues has emerged as a promising target for cancer therapy (104, 105). Patients with lung adenocarcinoma (49), gastric cancer (50), and oral squamous cell carcinoma (51) show elevated cysteine levels in their blood, which also reflects the disorder of cysteine metabolism in the tumor state, demonstrating that cysteine can be used as a biomarker for cancer early detection.
As a non-essential amino acid, in addition to exogenous food intake, proline comes from the de novo synthesis pathway and collagen degradation (106). The conversion between pyrroline-5-carboxylate and proline is a reversible reaction catalyzed by pyrroline-5-carboxylate reductase and proline dehydrogenase; the former has been consistently recognized as a key oncogenic factor that promotes tumor proliferation and maintains redox homeostasis (107), while proline dehydrogenase plays a dual role in tumors by regulating apoptosis and autophagy in tumor cells (108). There are also conflicting results regarding changes in blood proline levels in patients with tumors, and two studies reported elevated proline levels compared to controls (36, 52), which may be associated with the high expression of pyrroline-5-carboxylate reductase in tumors. However, a multicenter study found that the serum proline level in patients with pancreatic cancer was lower than that in healthy controls and benign lesion groups and proposed a metabolic panel (proline, creatine and palmitic acid) that showed good sensitivity and specificity in the early diagnosis of pancreatic cancer (53). Sputum proline levels have also been reported, with two studies showing reduced proline levels in patients with thyroid and lung cancer (41, 42).
The conversion of phenylalanine to tyrosine, catalyzed by phenylalanine hydroxylase is the main metabolic pathway of phenylalanine in the human body. In addition to being the main source of catecholamine neurotransmitters, tyrosine is also converted to janosonic acid and acetoacetic acid, catalyzed by tyrosine aminotransferase, and then enters the TCA cycle and lipid metabolism pathway, respectively. Cang et al. found elevated plasma levels of phenylalanine in NSCLC and suggested that this may be due to abnormal inflammation and immune responses in tumors, which impair the function of phenylalanine hydroxylase (54). Similarly, two recent studies have reported increased levels of phenylalanine in the blood of lung cancer patients (55, 56). In another metabolomic study, patients with lung cancer showed increased sputum levels of tyrosine compared to those with benign lung lesions, and a metabolic panel combining tyrosine with diethanolamine, cytosine, and lysine was proposed for the early diagnosis of lung cancer (46). Changes in phenylalanine and tyrosine levels in other cancers have also been reported (32, 42, 57). One of these observational studies demonstrated a good positive correlation between tyrosine content and hepatocellular carcinoma based on serum metabolomics (57).
Inosine and hypoxanthine are important intermediate products of purine metabolism that are produced sequentially by the decomposition of hypoxanthine nucleotides. Hypoxanthine can enter the salvage synthesis pathway to participate in nucleotide synthesis again or be further oxidized by xanthine oxidase to form uric acid and excreted from the body. To realize the early diagnosis of esophageal squamous cell carcinoma, Zhu et al. conducted a comparative analysis of serum metabolites in 140 patients with cancer and 170 healthy controls and established a panel consisting of eight metabolites among which inosine and hypoxanthine levels were relatively low in the cancer cohort compared to those in the healthy cohort (59). In contrast, using abnormal esophageal squamous hyperplasia as a control, Zhang et al. obtained a completely different panel containing increased hypoxanthine, l-glutamate, l-aspartate and decreased 2-ketoisocaproic acid (31). Altered plasma levels of inosine and hypoxanthine were also found in patients with pancreatic and breast cancer in two other MS studies (58, 60).
Uracil is derived from the catabolism of pyrimidine nucleotides in the liver, which can be further catabolized to β-alanine, a key mediator of metabolic crosstalk between tumor and stromal cells (109). In addition to being excreted in urine, more importantly, β-alanine can be broken down into acetyl coenzyme A, providing energy and raw materials for cellular biosynthesis. There is inconclusive evidence on the changes in uracil levels among cancer patients, as both increased (38, 41, 49) and decreased (61) have been reported.
Palmitic acid is the most abundant saturated fatty acid in the human body, accounting for approximately 25% of the total fatty acids and 65% of the total saturated fatty acids in the human body (110). Interestingly, tumor cells can directly obtain fatty acids produced by adipocyte lysis in the TME, and an in vitro study proposed that adipocyte-derived palmitic acid promotes melanoma cell proliferation by activating protein kinase B (111, 112). Two studies found increased levels of palmitic acid in the blood of patients with NSCLC and pancreatic cancer and both proposed that metabolite panels containing palmitic acid could be used for the early diagnosis of diseases (19, 53). However, in another lung cancer study that included adenocarcinoma, squamous cell carcinoma, SCLC, and other pathological types, significantly lower palmitic acid levels were found in the case group than in the control group, and metabolic differences between SCLC and NSCLC may be responsible for this inconsistency with the aforementioned study (62).
As an essential fatty acid, linoleic acid can only be acquired from food and cannot be synthesized because the body lacks relevant desaturation enzymes. In addition to participating in the composition of membrane phospholipids and cell energy supply, linoleic acid can be further converted to γ-linolenic acid and arachidonic acid through desaturation and extension reactions. Arachidonic acid derivatives such as prostaglandins and leukotrienes are important mediators involved in the inflammatory response and tumor development (113). Studies have identified significantly lower blood linoleic acid levels in patients with esophageal cancer (31), gastric cancer (63), and colon cancer (44), suggesting a key role of linoleic acid metabolism in gastrointestinal tumors.
PCs and PEs are the most abundant phospholipids in biofilms, and the ratio of PEs to PCs is critical in regulating lipoprotein secretion and maintaining mitochondrial function. In one MS study, lung cancer patients had lower levels of PCs and PEs in pleural effusions than did tuberculosis patients (66). Differences in blood PCs and PEs between cancer patients and controls were also reported in four studies (28, 64, 65, 70). Interestingly, in α-fetoprotein (AFP)-negative hepatocellular carcinoma, the concentrations of PC (22:6/18:2) and PC (18:2/18:2) were increased while the concentrations of PC (16:0/16:0) were decreased, which indicated that serological alterations in PCs would help us to identify patients with hepatocellular carcinoma missed by AFP at an early stage (64). Lysophosphatidylcholines (LPCs) and lysophosphatidylethanolamines are metabolic intermediates of PCs and PEs that are hydrolyzed by phospholipases. A significant body of literature has documented that changes in their levels in biological fluids are associated with early tumor diagnosis (35, 54, 59, 63, 67–70).
SMs and CERs are not only involved in maintaining the fluidity of biological membranes, but also act as important signaling molecules to regulate different life activities. SMs mainly promote cell proliferation and migration, while CERs can induce cell apoptosis and senescence (114). Five recent studies based on blood metabolomics observed differences in SMs between cancer patients and controls, four of which observed elevated levels of SMs in the patients with cancer (47, 70–72). One study showed reduced SM 42:2 and SM42:3 in patients with laryngeal cancer compared to patients with benign laryngeal tumors and healthy volunteers (73). A prospective study of 252 cases and 252 matched controls compared the differences in circulating lipid metabolites between groups, followed all enrolled subjects for up to 23 years, and concluded that: increased SMs and CERs predicted higher ovarian cancer risks (71).
Bile acids are important products of cholesterol metabolism in hepatocytes and enter the intestine via the bile duct bile salt pump, thus contributing to lipids digestion and absorption (115). Farnesoid X receptor, the receptor of bile acids, plays a key role in maintaining bile acid balance in the liver and intestine (116). In the TME, inflammation inhibits the expression of the bile duct bile salt pump and the farnesoid X receptor, thus disrupting bile acid homeostasis and inducing an inflammatory response, ultimately creating a vicious cycle (117). GCA is the most abundant bile acid in the human body, whose changes in cancer have demonstrated its diagnostic potential. Two multicenter studies found higher levels of GCA in patients with hepatocellular carcinoma and colon cancer (57, 74). Another study proposed that the panel composed of five metabolites (creatine, inosine, β-sitosterol, sphinganine and GCA) had better diagnostic performance than carbohydrate antigen 125 and CEA (60).
Estrogens, a class of cholesterol derivatives, have been reported to be involved in the development of multiple malignancies, especially breast cancer (118, 119). In a randomized controlled trial, Dallal CM et al. found that postmenopausal women with elevated serum estradiol levels had a higher risk of breast cancer (75). Urinary estradiol levels in postmenopausal women have also been shown to be positively associated with the risk of breast cancer in two case-control studies (76, 77). Hydroxylation is the main pathway of estrogens metabolism. Based on the MS technique, studies have successively reported there is an inverse correlation between the ratio of serum 2-hydroxylation pathway metabolites to 16-hydroxylation pathway metabolites with breast cancer risk in postmenopausal women (75, 120, 121). However, due to the diversity of 16-hydroxylation pathway metabolites and the limited accuracy of specific estrogen metabolite concentrations determined by MS, the association between the ratio and breast cancer risk needs to be further verified (122). The formation of catechol estrogen quinones is a key molecular mechanism of estrogen carcinogenesis. It can further promote DNA mutation by reacting with DNA to form depurinating DNA-adducts (119). Previous studies have suggested that high-risk breast cancer patients have higher levels of depurinating DNA-adducts in circulating body fluids than healthy controls (123, 124), yet a recent study by Reding KW et al. found no correlation between urinary levels of depurinating DNA-adducts and breast cancer risk (125). In conclusion, these inconsistent findings indicate the necessity to further explore the reliability of circulating estrogens and their metabolites in predicting the risk of breast cancer.
Hippuric acid, also known as “benzoylaminoacetic acid,” is a coupling of benzoic acid and glycine. Owing to its high stability, it was initially identified as a detoxification metabolite of toluene and is still used to predict occupational exposure to toluene (126). Moreover, hippuric acid is an intestinal metabolite of phenylalanine, and its role as a host-gut microbiome co-metabolite has received increasing attention (127). Although the mechanisms underlying the altered metabolism of hippuric acid in cancer development have not been elucidated, limited experimental studies have shown that a high-salt diet produces more hippuric acid by increasing the abundance of bifidobacteria in the mouse intestine, which enhances the tumor-killing function of natural killer cells (128). In three MS studies comparing the variance in urinary metabolites between patients with cancer and controls, reduced levels of hippuric acid were consistently observed, further suggesting its potential utility in cancer diagnosis (23, 78, 79).
Extracellular vesicles (EVs) serve as important mediators of cell-to-cell communication and offer new opportunities for identifying novel tumor markers (129). In addition to circulating proteins and nucleic acids, the role of EV-derived lipid metabolites in cancer diagnosis is also gaining attention (Table 2). Phospholipids are important components of extracellular vesicles, and there is growing evidence that PCs, PEs, and SMs in EVs derived from biological fluids show significant differences between cancer patients and non-cancer controls (130–132, 134–136, 139). It has been proposed that changes in the fatty acids content of blood-derived extracellular vesicles may indicate the presence of cancer (133, 136–138, 140). Although there is currently no consensus on the diagnostic value of extracellular vesicle-derived lipid metabolites in different tumors, these findings suggest a great potential for metabolomics-based extracellular vesicle assays to advance into clinical practice.
In recent years, metabolic differences in different types of cancer have also been noted. Studies have not only identified metabolites that help to detect specific pathological types but also proposed potential metabolic markers to distinguish between the presence or absence of genetic mutations and primary or secondary tumors. In addition, the non-invasive and convenient characteristics of blood and urinary metabolomics make it a useful tool for predicting cancer grade and stage, which may instruct clinicians to develop appropriate treatment plans for patients (Table 3).
Both primary brain tumors and secondary brain metastases are important reasons for short survival and high mortality rates. Metabolites of brain tumors can be released directly into the cerebrospinal fluid; therefore, abnormal metabolic variations in cerebrospinal fluid can effectively reflect the pathophysiological changes of brain tumors. One cerebrospinal fluid metabolomics study described the metabolic characteristics of primary central nervous system lymphoma, secondary central nervous system involvement of systemic lymphoma (SCNSL), and lung adenocarcinoma with brain metastases (MBT) by liquid chromatography-quadrupole time-of-fight spectrometric and cross−contrasted the differential metabolites of patients with these three types of brain tumor (144). Compared with MBT, SCNSL had lower levels of pyruvate, a common substrate for aerobic and anaerobic oxidation. Additionally, there was a higher level of citric acid in MBT than in primary central nervous system lymphoma and SCNSL, implying a more active aerobic oxidative metabolism in MBT. Epithelial growth factor receptor (EGFR) mutations are common in lung cancer, and the identification of gene mutation types is becoming increasingly crucial because of the clinical efficacy of the latest generation of EGFR-targeted drugs. Chen et al. used GC-MS to compare the volatile metabolites of malignant pleural effusion in lung cancer patients with or without EGFR mutations (143). There was a clear separation between the clustering of patients with and without EGFR mutations from the score plot. After performing ROC analysis and bootstrapped t-test, they identified 5 and 14 volatile organic compounds related to glucose synthesis and catabolism, respectively, for the early identification of EGFR mutation.
Due to the presence of heterogeneity, significant amino acid alterations have also been observed in the biological fluids of patients with different pathological types of cancer. A study using MS analyzed the plasma metabolite differences in patients with three types of lung cancer: lung adenocarcinoma, lung squamous cell carcinoma, and SCLC (62). Ornithine has emerged as a possible biomarker, following the application of orthogonal projections to latent structures discriminant analysis (OPLS-DA). Based on previous studies (153), to further explore metabolic changes of kynurenine and tryptophan in different types of lung cancer, Mandarano et al. observed that patients with squamous cell carcinoma have a higher serum kynurenine/tryptophan ratio than patients with lung adenocarcinoma (141). Kynurenine was also highlighted in another MS study on epithelial ovarian cancer, which explored serum metabolomic alterations in four different histopathological types (145). A panel of 12 metabolites containing kynurenine were identified as potential markers for distinguishing serous carcinoma from non-serous carcinoma by ROC analysis.
Changes in lipid metabolites have been associated with the origin of malignant lung nodules. In a study conducted by Liu et al. involving 16 patients with pulmonary metastatic carcinoma and 80 patients with primary lung carcinoma, a group of lipid metabolic biomarkers consisting of L-octanoylcarnitine, retinol, and decanoylcarnitine were able to predict metastatic lung cancer (AUC > 0.95) (142). Abundant differential metabolic molecules associated with lipid metabolism between serous ovarian carcinoma and non-serous ovarian carcinoma have also been identified by Olkowicz et al., such as phosphatidic acid (38:4), monoacylglycerol (18:2), stearidonic acid, choladien-24-oic-acid and hydroxy-5-cholenoic acid (145).
One reported NMR spectroscopic metabolic panel could distinguish patients with low-grade bladder cancer (n = 54) from those with high-grade bladder cancer (n = 41) through a permutation analysis-validated OPLS-DA model comprising five metabolites (leucine, histidine, alanine, 3-methyl-2-oxovalerate, and tyrosine) (146). In addition to bladder cancer, an OPLS-DA score plot by Kurokawa et al. showed patients with meningioma were clustered according to different grades (147). Finally, Arginyl-Proline, PS (44:6), 3-O-sulfogalactosylceramide (42:2), PS (36:5) and CER (40:1) were identified as serum metabolic markers for high-grade meningiomas. Arginyl-Proline is a dipeptide formed by the dehydration condensation of arginine and proline, the increase in which indicates that high-grade meningiomas have a greater capacity for proliferation. The four remaining metabolic markers indicated the significant involvement of phospholipid metabolism in the pathological progression of high-grade meningiomas.
Attempts to use metabolomic techniques identifying biomarker for advanced renal cell carcinoma have been reported. Sato et al. reported that a panel of four metabolites, l-kynurenine, l-glutamine, fructose 6-phosphate, and butyrylcarnitine, could predict stage III/IV disease with high accuracy (sensitivity 88.5% and specificity 75.4%) (22). Higher levels of glucose-6-phosphate may indicate an increase in the energy requirements obtained through glycolysis. GLUT5, a specific fructose transporter, has recently been reported to interact with interleukin-6 to participate in tumor progression by promoting fructose uptake (154). In an MS study of NSCLC patients, 11 metabolites including D-fructose were identified that differed markedly in plasma between patients in the early and advanced stages according to partial least squares discriminant analysis (variable importance in projection ≥ 1) and Mann-Whitney test (P-value ≤ 0.05) (148). Citric acid, an intermediate product of the TCA cycle, is crucial for the interactions between glucose and lipid metabolism. In a study on prostate cancer, Buszewska-Forajta et al. observed low levels of citric acid in the blood, urine, or tissue of patients in the advanced stages, suggesting that citric acid and prostate cancer progression are closely linked (152).
In an NMR study, a metabolite panel containing seven amino acids demonstrated an AUC > 0.80 in distinguishing between stage Ta/T1 and T2 bladder cancer, and all of these amino acids were relatively reduced in stage T2 (146). Apart from D-fructose, alterations in plasma levels of intermediates in the phenylalanine metabolism pathway, such as D-phenylalanine and phenylacetic acid, have also been observed to differ in patients with early- and advanced- stage lung cancer (148). (2S,3S)-3-Methylphenylalanine, another phenylalanine derivative, was identified as a putative biomarker of esophageal squamous cell carcinoma progression using least absolute shrinkage and selection operator regularization and random forest (149).
In the absence of inexpensive and effective markers, colonoscopy and computed tomography remain the primary methods of clinically staging of colon cancer. A combination of tandem MS and logistic regression analysis of serum samples collected from 744 patients with colon cancer generated metabolomic profiles that distinguished stages III-IV from stages I-II (150). The top ten metabolites included nine PCs and SM (C18:0), indicating that phospholipid metabolism was disturbed during colorectal cancer progression. Hydroquinone and sphingomyelin, two other molecules of the lipid metabolism pathway, were identified by Rao et al. as new markers for the early detection of advanced colorectal cancer and showed a good correlation with the traditional markers CA199 and CEA (151). However, owing to the small sample size and lack of early-stage patients for comparison, further verification is required. In an untargeted plasma metabolomics study, LPC (20:5) and LPE (20:4) were identified as putative biomarkers of esophageal squamous cell carcinoma progression (59), which may be related to their involvement in pathological injury to the cell membrane. After successfully identifying plasma metabolites that help in the diagnosis of lung cancer through metabolomics, Qi et al. attempted to identify metabolic markers that could distinguish different stages of lung cancer (62). In their study, an optimized discriminant logistic regression model generated by least absolute shrinkage and selection operator regularization of five variable importance in projection-selected metabolites (palmitic acid, heptadecanoic acid, ornithine, tridecanoic acid and stearic acid) was able to effectively discriminate between early and advanced stages. Four are fatty acids, except for ornithine, implying that fatty acid biosynthesis is required for tumor growth and proliferation.
Most studies have identified metabolomic markers for predicting therapy efficacy and prognosis in blood, urine, and other biofluids from patients with cancer (Table 4), which may help clinicians screen good responders and prevent delaying the malignant condition in poor responders by adjusting their treatment strategies timely.
Table 4 Summary of biofluid metabolomic biomarkers for treatment response and prognosis prediction in cancer.
Blood metabolites collected before treatments have predictive power for therapeutic response and survival of patients with cancer. One study reported lower levels of isocitric acid and citric acid in the non-pathological complete response group than in the pathological complete response group, suggesting that these two compounds may predict patient responses to neoadjuvant chemoradiotherapy (156). Using multivariate logistic regression analysis, another MS study determined a five-metabolite panel containing lactic acid, 2-hydroxyglutaric acid, and succinic acid that could predict the recurrence of renal cell carcinoma after surgery with a sensitivity and specificity of 88.9% and 88.0%, respectively (163). Microvascular invasion is a detrimental factor affecting the surgical outcome and prognosis of patients with hepatocellular carcinoma, sensitive detection methods are still lacking because of its insidious nature. Lee et al. presented an OPLS-DA model in their NMR study that effectively differentiated between patients with and without microvascular invasion (159). Combining formate and CA199 improved the ability to predict microvascular invasion, which was confirmed in the validation cohort. Differential metabolites have also been detected in the peritoneal lavage fluids of gastric cancer patients and those with peritoneal metastasis tended to have higher glyceraldehyde-3-phosphate (161).
Plasma metabolomics measured with high-performance liquid chromatography for NSCLC patients receiving anti-programmed cell death protein 1 therapy with good survival have also been compared with those obtained from patients with poor survival and could be successfully separated in a multivariate model (concordance index = 0.775, hazard ratio = 3.23) (155). Quinolinic acid, a tryptophan intermediate metabolite mentioned in this study, was negatively correlated with the immunotherapy efficacy in NSCLC patients. Another tryptophan catabolite, 3-hydroxyanthranilic acid, was also proposed as a potential indicator of adverse clinical outcomes in NSCLC patients receiving anti-programmed cell death protein 1 therapy (43). In addition to NSCLC, abnormal tryptophan catabolism is also found in esophageal and ovarian cancers. Plasma kynurenine levels have been proposed to be negatively correlated with patient survival (157, 166). According to serum metabolomic profiles detected by NMR, the levels of three amino acids (glutamine, histidine and valine) in bladder cancer patients decreased markedly after surgery and approached those in healthy controls (164). In an untargeted study using both NMR and MS, significant increases in glycine and decreases in taurine and glutamine levels were observed in patients resistant to neoadjuvant chemotherapy (165). Monitoring changes in blood amino acids has also been reported to effectively predict chemotherapy efficacy and survival in patients with hematological malignancies (169, 170).
Recent studies have also revealed that metabolites of nucleotide metabolic pathways can be potential predictors of cancer prognosis. Jung et al. divided 18 patients with bladder cancer who received neoadjuvant chemotherapy into sensitive (n = 6) and resistant (n = 12) groups based on their therapeutic response and observed higher serum hypoxanthine levels in the resistant group (P-value < 0.05) (165). Furthermore, elevated serum levels of 1-methyladenosine (a purine nucleotide metabolite) are associated with an increased risk of prostate cancer recurrence (168).
Surgery remains the preferred treatment for resectable lung cancer. Yang et al. proposed a panel composed of six phospholipids (SM 42:2, SM 35:1, SM 38:3, PC 30:0, PC 30:1and CER 42:2) through the long-term follow-up of patients undergoing surgery, which could effectively distinguish between patients with postoperative recurrence and non-recurrence (35). Pancreatic cancer and liver cancer are highly malignant and rapidly evolving diseases for which convenient and sensitive clinical prognostic markers are still lacking. Recent researches have shown that lipids and lipid molecules in the blood are strongly related to patient survival. By establishing proportional hazards model, Wolrab et al. found LPC 18:2 and PC O-38:5 exhibited a positive and negative correlation with the overall survival of patients with pancreatic cancer, respectively (67). Similarly, liver cancer patients with low levels of retinol and retinal showed poor survival, so these two metabolites were identified as new prognostic markers superior to AFP (160).
Based on the role of oxidative stress in the pathological progression of colon cancer, a large-scale study enrolled 3361 patients with colon cancer and followed them for up to 6 years to explore the correlation between oxidative stress products and patient prognosis (162). A higher pre-treatment serum abundance of total thiol and the total thiol to diacron reactive oxygen metabolites ratio were associated with lower mortality, particularly in stage IV patients. In a pancreatic cancer study, a panel of three metabolites (trigonelline, hippurate and myoinositol) measured by 1H-labeling NMR had the potential to stratify postoperative patients into good or poor outcomes (158).
NMR is a technique that utilizes the resonance phenomenon of nuclei in a magnetic field to measure the resonance frequency and intensity of different nuclei. It has several advantages, including fast analysis, non-destructive testing, and good repeatability due to the simple sample pretreatment process. However, NMR also presents the limitations of poor sensitivity and resolution when characterizing metabolites that are present at low concentrations, are unknown, or have overlapping spectra (171). The development and application of two-dimensional (2D) NMR technology (172) and cryogenic probe 13C NMR spectroscopy (173) are the main means to improve resolution and enhance sensitivity. Mass Spectrometry (MS) is a technique that ionizes molecules by bombarding them with electrons. The ions are then separated based on their mass-to-charge ratio, and the relative peak intensities of different ions are measured (174). Higher sensitivity is the main advantage of MS compared to NMR. GC-MS and LC-MS have different applications for metabolomics studies depending on the nature of the isolated metabolites (175). LC-MS is primarily used to analyze non-volatile and unstable substances, while GC-MS is suitable for the analysis of volatile metabolites. The advent of UPLC-MS/MS technology has further improved the analytical speed and sensitivity of MS technology. In addition to separation-based MS technology, nanoparticle-enhanced laserdesorption/ionization-mass spectrometry (NPELDI-MS) has significantly advanced metabolomics due to its increased sensitivity, speed, and mass accuracy (176). A large-scale gastric cancer study combined NPELDI-MS technology with machine learning algorithms to construct a blood metabolic marker panel for the early detection of gastric cancer that significantly outperforms traditional tumor markers (176). Table 5 compared the characteristics of NMR and MS techniques.
By offering a comprehensive “fingerprint” of metabolite alterations across various biofluids, metabolomics has presented an array of potential biomarkers in cancer management. However, multiple studies have reported inconsonant changes in metabolites for a specific type of cancer. It is important to fully understand the current limitations in metabolomics research and find solutions to these challenges in future studies.
The heterogeneity of the study population is an important reason for the differences between studies. Metabolic information carried by body fluids not only comes from the internal metabolism of the entire body but also is affected by the external environment. Therefore, a patient’s metabolic profile can be affected by various factors such as age, gender, dietary habits, medications, and comorbidities, it is necessary to set up strict exclusion and control matching criteria to minimize the impact of confounding factors. Moreover, considering the existence of individual heterogeneity, expanding the sample size and establishing a validation cohort are key to improving the objectivity of the research findings.
Differences in sample selection and preparation significantly contribute to the variation in results. Various biological fluids, including blood, urine, pleural fluid, peritoneal fluid, and cerebrospinal fluid, can be analyzed for metabolomics. Peripheral blood and urine are the first choice for most metabolomics studies because of their high clinical accessibility. Selecting appropriate sample sources based on the physiological characteristics of tumors can provide more valuable information for metabolomics studies. For example, as a crucial component of the lung cancer microenvironment, pleural effusion can more directly reflect metabolic changes in lung cancer patients and is less influenced by the body’s systemic metabolism (143). Uniform collection time and appropriate storage conditions are crucial for accurate metabolomics data. Therefore, proper sample preparation is vital and should align with the research design and sample requirements (177).
Disagreements among different studies may be related to variations in analytical platforms and statistical methods. Most metabolomics studies rely on either MS or NMR techniques for metabolite detection and analysis. The lack of uniform criteria for selecting differential metabolites and the use of a single analytical platform have led to unsatisfactory comparisons between studies. Since NMR and MS technologies are different in principles and strengths, combining these two technologies is a crucial approach to fully harnessing the potential of metabolomics in cancer research (178). The selection of suitable statistical methods is also a crucial step in identifying metabolic markers specific to tumors. The multivariate analysis approach enables researchers to gain a comprehensive understanding of the contribution of various metabolites in cancer development and facilitates the establishment of more reliable metabolic models. Besides commonly used multivariate analysis methods such as Principal Component Analysis and OPLS-DA, multivariate logistic regression models that include clinicopathologic factors and contributing metabolites are a recommended option (179). Furthermore, the use of machine learning algorithms has facilitated the efficient analysis of complex metabolomics datasets (167). However, selecting appropriate algorithms and improving their generalizability remain issues for future consideration (180).
Advances in liquid biopsy are breaking new grounds for cancer diagnosis and treatment. Although liquid biopsy is not yet a complete substitute for tissue biopsy, it is a convenient, sensitive, and safe strategy for future clinical cancer management. Considering the intricate roles of metabolites and their related pathways in cancer development, metabolomic biomarkers identified in recent biofluid metabolomics studies would offer valuable information for tumor diagnosis, classification, and prognosis prediction (Figure 2). Metabolites that differ significantly in the body fluids of patients with cancer compared to those with benign disease and healthy individuals can aid clinicians in recognizing the presence of tumors early, particularly in patients who test negative for traditional tumor markers (181). Identifying the differential metabolites that vary in distinct tumor types, grades, and stages assists in understanding the pathological characteristics and malignancy of tumors and provides clues for appropriate treatment plans. Tracking metabolite changes before and after treatment enables real-time monitoring of patient responses and timely identification of patients with a favorable prognosis. However, most of the current findings in this field are limited to single-center, small-sample studies. Inter-agency collaboration or the promotion of centralized testing centers should be encouraged to ensure accurate identification of metabolomic biomarkers. Only through validation in multi-center studies and clinical trials can these metabolic markers advance to the clinical translation stage. Therefore, caution must be exercised at this time when considering the practical application of these results in the clinic. Notably, differential metabolites, especially those overlapping metabolites between studies, can guide researchers to further explore metabolic mechanisms in cancer development and provide a theoretical basis for the identification of metabolic markers. Furthermore, recent studies have shown that combining metabolomics with other omics can result in more sensitive cancer marker panels for detecting changes in a patient’s condition (182, 183). The biomolecular mechanisms underlying metabolic markers may make targeting metabolism for antitumor therapy a promising option in clinical settings.
Figure 2 Cancer metabolic markers obtained by liquid biopsy. Combining liquid biopsy with metabolomics can provide potential biomarkers for different aspects of clinical cancer management. PC, phosphatidylcholine; LPC, lysophosphatidylcholine; PE, phosphatidylethanolamine; LPE, lysophosphatidylethanolamine; LUAD, lung adenocarcinoma; LUSC, lung squamous cell carcinoma; SCLC, small cell lung cancer; PD-1, programmed cell death protein 1.
WW: Writing – original draft. SZ: Writing – original draft. YP: Writing – review & editing. LW: Conceptualization, Supervision, Writing – review & editing. YZ: Conceptualization, Funding acquisition, Supervision, Writing – review & editing.
The author(s) declare financial support was received for the research, authorship, and/or publication of this article. This work was supported by the National Key R&D Program: Intergovernmental International Science and Technology Innovation Cooperation Project (grant no. 2022YFE0141000), National Natural Science Foundation of China (grant no. 82272873), the Central Government of Henan Province Guides Local Science and Technology Development Fund Projects (Z20221343036), Medical Science and Technology Project of Henan Province (grant no. SBGJ202101010).
The authors declare that the research was conducted in the absence of any commercial or financial relationships that could be construed as a potential conflict of interest.
The author(s) declared that they were an editorial board member of Frontiers, at the time of submission. This had no impact on the peer review process and the final decision.
All claims expressed in this article are solely those of the authors and do not necessarily represent those of their affiliated organizations, or those of the publisher, the editors and the reviewers. Any product that may be evaluated in this article, or claim that may be made by its manufacturer, is not guaranteed or endorsed by the publisher.
1. Pe’er D, Ogawa S, Elhanani O, Keren L, Oliver TG, Wedge D. Tumor heterogeneity. Cancer Cell (2021) 39(8):1015–7. doi: 10.1016/j.ccell.2021.07.009
2. McGranahan N, Swanton C. Clonal heterogeneity and tumor evolution: past, present, and the future. Cell (2017) 168(4):613–28. doi: 10.1016/j.cell.2017.01.018
3. Lone SN, Nisar S, Masoodi T, Singh M, Rizwan A, Hashem S, et al. Liquid biopsy: a step closer to transform diagnosis, prognosis and future of cancer treatments. Mol Cancer (2022) 21(1):79. doi: 10.1186/s12943-022-01543-7
4. Alix-Panabières C, Pantel K. Liquid biopsy: from discovery to clinical application. Cancer Discov (2021) 11(4):858–73. doi: 10.1158/2159-8290.CD-20-1311
5. Hu S, Tao J, Peng M, Ye Z, Chen Z, Chen H, et al. Accurate detection of early-stage lung cancer using a panel of circulating cell-free DNA methylation biomarkers. biomark Res (2023) 11(1):45. doi: 10.1186/s40364-023-00486-5
6. Galanzha EI, Menyaev YA, Yadem AC, Sarimollaoglu M, Juratli MA, Nedosekin DA, et al. In vivo liquid biopsy using Cytophone platform for photoacoustic detection of circulating tumor cells in patients with melanoma. Sci Trans Med (2019) 11(496):eaat5857. doi: 10.1126/scitranslmed.aat5857
7. McKiernan J, Donovan MJ, Margolis E, Partin A, Carter B, Brown G, et al. A prospective adaptive utility trial to validate performance of a novel urine exosome gene expression assay to predict high-grade prostate cancer in patients with prostate-specific antigen 2-10ng/ml at initial biopsy. Eur Urol (2018) 74(6):731–8. doi: 10.1016/j.eururo.2018.08.019
8. Schmidt DR, Patel R, Kirsch DG, Lewis CA, Vander Heiden MG, Locasale JW. Metabolomics in cancer research and emerging applications in clinical oncology. CA: Cancer J Clin (2021) 71(4):333–58. doi: 10.3322/caac.21670
9. Wishart DS. Metabolomics for investigating physiological and pathophysiological processes. Physiol Rev (2019) 99(4):1819–75. doi: 10.1152/physrev.00035.2018
10. Rinschen MM, Ivanisevic J, Giera M, Siuzdak G. Identification of bioactive metabolites using activity metabolomics. Nat Rev Mol Cell Biol (2019) 20(6):353–67. doi: 10.1038/s41580-019-0108-4
11. Hu L, Liu J, Zhang W, Wang T, Zhang N, Lee YH, et al. Functional metabolomics decipher biochemical functions and associated mechanisms underlie small-molecule metabolism. Mass Spectrometry Rev (2020) 39(5-6):417–33. doi: 10.1002/mas.21611
12. Ayala-Lopez N. Nuclear magnetic resonance metabolomics-enabled biomarker discovery for all-cause mortality. Clin Chem (2020) 66(2):400. doi: 10.1093/clinchem/hvz014
13. Alseekh S, Aharoni A, Brotman Y, Contrepois K, D’Auria J, Ewald J, et al. Mass spectrometry-based metabolomics: a guide for annotation, quantification and best reporting practices. Nat Methods (2021) 18(7):747–56. doi: 10.1038/s41592-021-01197-1
14. Venneti S, Dunphy MP, Zhang H, Pitter KL, Zanzonico P, Campos C, et al. Glutamine-based PET imaging facilitates enhanced metabolic evaluation of gliomas in vivo. Sci Trans Med (2015) 7(274):274ra17. doi: 10.1126/scitranslmed.aaa1009
15. Fletcher JW, Djulbegovic B, Soares HP, Siegel BA, Lowe VJ, Lyman GH, et al. Recommendations on the use of 18F-FDG PET in oncology. J Nucl Med: Off Publication Soc Nucl Med (2008) 49(3):480–508. doi: 10.2967/jnumed.107.047787
16. Nizioł J, Ossoliński K, Tripet BP, Copié V, Arendowski A, Ruman T. Nuclear magnetic resonance and surface-assisted laser desorption/ionization mass spectrometry-based serum metabolomics of kidney cancer. Analytical Bioanalytical Chem (2020) 412(23):5827–41. doi: 10.1007/s00216-020-02807-1
17. Fei F, Zheng M, Xu Z, Sun R, Chen X, Cao B, et al. Plasma metabolites forecast occurrence and prognosis for patients with diffuse large B-cell lymphoma. Front Oncol (2022) 12:894891. doi: 10.3389/fonc.2022.894891
18. Lima AR, Pinto J, Barros-Silva D, Jerónimo C, Henrique R, Bastos ML, et al. New findings on urinary prostate cancer metabolome through combined GC-MS and (1)H NMR analytical platforms. Metabolomics: Off J Metabolomic Society (2020) 16(6):70. doi: 10.1007/s11306-020-01691-1
19. Guo L, Li L, Xu Z, Meng F, Guo H, Liu P, et al. Metabolic network-based identification of plasma markers for non-small cell lung cancer. Analytical Bioanalytical Chem (2021) 413(30):7421–30. doi: 10.1007/s00216-021-03699-5
20. Ye W, Lin Y, Bezabeh T, Ma C, Liang J, Zhao J, et al. (1) H NMR-based metabolomics of paired esophageal tumor tissues and serum samples identifies specific serum biomarkers for esophageal cancer. NMR Biomed (2021) 34(6):e4505. doi: 10.1002/nbm.4505
21. Lv D, Zou Y, Zeng Z, Yao H, Ding S, Bian Y, et al. Comprehensive metabolomic profiling of osteosarcoma based on UHPLC-HRMS. Metabolomics: Off J Metabolomic Society (2020) 16(12):120. doi: 10.1007/s11306-020-01745-4
22. Sato T, Kawasaki Y, Maekawa M, Takasaki S, Shimada S, Morozumi K, et al. Accurate quantification of urinary metabolites for predictive models manifest clinicopathology of renal cell carcinoma. Cancer Sci (2020) 111(7):2570–8. doi: 10.1111/cas.14440
23. Yu C, Niu L, Li L, Li T, Duan L, He Z, et al. Identification of the metabolic signatures of prostate cancer by mass spectrometry-based plasma and urine metabolomics analysis. Prostate (2021) 81(16):1320–8. doi: 10.1002/pros.24229
24. Wang W, He Z, Kong Y, Liu Z, Gong L. GC-MS-based metabolomics reveals new biomarkers to assist the differentiation of prostate cancer and benign prostatic hyperplasia. Clin Chim Acta; Int J Clin Chem (2021) 519:10–7. doi: 10.1016/j.cca.2021.03.021
25. Zheng Y, He Z, Kong Y, Huang X, Zhu W, Liu Z, et al. Combined metabolomics with transcriptomics reveals important serum biomarkers correlated with lung cancer proliferation through a calcium signaling pathway. J Proteome Res (2021) 20(7):3444–54. doi: 10.1021/acs.jproteome.0c01019
26. Sahni S, Pandya AR, Hadden WJ, Nahm CB, Maloney S, Cook V, et al. A unique urinary metabolomic signature for the detection of pancreatic ductal adenocarcinoma. Int J Cancer (2021) 148(6):1508–18. doi: 10.1002/ijc.33368
27. Zhou PC, Sun LQ, Shao L, Yi LZ, Li N, Fan XG. Establishment of a pattern recognition metabolomics model for the diagnosis of hepatocellular carcinoma. World J Gastroenterol (2020) 26(31):4607–23. doi: 10.3748/wjg.v26.i31.4607
28. Iwano T, Yoshimura K, Watanabe G, Saito R, Kiritani S, Kawaida H, et al. High-performance collective biomarker from liquid biopsy for diagnosis of pancreatic cancer based on mass spectrometry and machine learning. J Cancer (2021) 12(24):7477–87. doi: 10.7150/jca.63244
29. Pedersen S, Hansen JB, Maltesen RG, Szejniuk WM, Andreassen T, Falkmer U, et al. Identifying metabolic alterations in newly diagnosed small cell lung cancer patients. Metab Open (2021) 12:100127. doi: 10.1016/j.metop.2021.100127
30. Guida F, Tan VY, Corbin LJ, Smith-Byrne K, Alcala K, Langenberg C, et al. The blood metabolome of incident kidney cancer: A case-control study nested within the MetKid consortium. PloS Med (2021) 18(9):e1003786. doi: 10.1371/journal.pmed.1003786
31. Zhang S, Lu X, Hu C, Li Y, Yang H, Yan H, et al. Serum metabolomics for biomarker screening of esophageal squamous cell carcinoma and esophageal squamous dysplasia using gas chromatography-mass spectrometry. ACS Omega (2020) 5(41):26402–12. doi: 10.1021/acsomega.0c02600
32. Xiong Y, Shi C, Zhong F, Liu X, Yang P. LC-MS/MS and SWATH based serum metabolomics enables biomarker discovery in pancreatic cancer. Clin Chim Acta; Int J Clin Chem (2020) 506:214–21. doi: 10.1016/j.cca.2020.03.043
33. Wang X, Zhao X, Zhao J, Yang T, Zhang F, Liu L. Serum metabolite signatures of epithelial ovarian cancer based on targeted metabolomics. Clin Chim Acta; Int J Clin Chem (2021) 518:59–69. doi: 10.1016/j.cca.2021.03.012
34. Guo J, Pan Y, Chen J, Jin P, Tang S, Wang H, et al. Serum metabolite signatures in normal individuals and patients with colorectal adenoma or colorectal cancer using UPLC-MS/MS method. J Proteomics (2023) 270:104741. doi: 10.1016/j.jprot.2022.104741
35. Yang D, Yang X, Li Y, Zhao P, Fu R, Ren T, et al. Clinical significance of circulating tumor cells and metabolic signatures in lung cancer after surgical removal. J Trans Med (2020) 18(1):243. doi: 10.1186/s12967-020-02401-0
36. Breeur M, Ferrari P, Dossus L, Jenab M, Johansson M, Rinaldi S, et al. Pan-cancer analysis of pre-diagnostic blood metabolite concentrations in the European Prospective Investigation into Cancer and Nutrition. BMC Med (2022) 20(1):351. doi: 10.1186/s12916-022-02553-4
37. Pinto FG, Mahmud I, Harmon TA, Rubio VY, Garrett TJ. Rapid prostate cancer noninvasive biomarker screening using segmented flow mass spectrometry-based untargeted metabolomics. J Proteome Res (2020) 19(5):2080–91. doi: 10.1021/acs.jproteome.0c00006
38. de Sá Alves M, de Sá Rodrigues N, Bandeira CM, Chagas JFS, Pascoal MBN, Nepomuceno G, et al. Identification of possible salivary metabolic biomarkers and altered metabolic pathways in south american patients diagnosed with oral squamous cell carcinoma. Metabolites (2021) 11(10):650. doi: 10.3390/metabo11100650
39. Cao Y, Zhao R, Guo K, Ren S, Zhang Y, Lu Z, et al. Potential metabolite biomarkers for early detection of stage-I pancreatic ductal adenocarcinoma. Front Oncol (2021) 11:744667. doi: 10.3389/fonc.2021.744667
40. Huang R, Shen K, He Q, Hu Y, Sun C, Guo C, et al. Metabolic profiling of urinary chiral amino-containing biomarkers for gastric cancer using a sensitive chiral chlorine-labeled probe by HPLC-MS/MS. J Proteome Res (2021) 20(8):3952–62. doi: 10.1021/acs.jproteome.1c00267
41. Jiang X, Chen X, Chen Z, Yu J, Lou H, Wu J. High-throughput salivary metabolite profiling on an ultralow noise tip-enhanced laser desorption ionization mass spectrometry platform for noninvasive diagnosis of early lung cancer. J Proteome Res (2021) 20(9):4346–56. doi: 10.1021/acs.jproteome.1c00310
42. Zhang J, Wen X, Li Y, Zhang J, Li X, Qian C, et al. Diagnostic approach to thyroid cancer based on amino acid metabolomics in saliva by ultra-performance liquid chromatography with high resolution mass spectrometry. Talanta (2021) 235:122729. doi: 10.1016/j.talanta.2021.122729
43. Karayama M, Masuda J, Mori K, Yasui H, Hozumi H, Suzuki Y, et al. Comprehensive assessment of multiple tryptophan metabolites as potential biomarkers for immune checkpoint inhibitors in patients with non-small cell lung cancer. Clin Trans Oncol: Off Publ Fed Spanish Oncol Societies Natl Cancer Institute Mexico (2021) 23(2):418–23. doi: 10.1007/s12094-020-02421-8
44. Wang M, Long Z, Xue W, Peng C, Jiang T, Tian J, et al. Discovery of plasma biomarkers for colorectal cancer diagnosis via untargeted and targeted quantitative metabolomics. Clin Trans Med (2022) 12(4):e805. doi: 10.1002/ctm2.805
45. Lee SH, Mahendran R, Tham SM, Thamboo TP, Chionh BJ, Lim YX, et al. Tryptophan-kynurenine ratio as a biomarker of bladder cancer. BJU Int (2021) 127(4):445–53. doi: 10.1111/bju.15205
46. Takamori S, Ishikawa S, Suzuki J, Oizumi H, Uchida T, Ueda S, et al. Differential diagnosis of lung cancer and benign lung lesion using salivary metabolites: A preliminary study. Thorac Cancer (2022) 13(3):460–5. doi: 10.1111/1759-7714.14282
47. Dossus L, Kouloura E, Biessy C, Viallon V, Siskos AP, Dimou N, et al. Prospective analysis of circulating metabolites and endometrial cancer risk. Gynecol Oncol (2021) 162(2):475–81. doi: 10.1016/j.ygyno.2021.06.001
48. Ouyang T, Ma C, Zhao Y, Ye W, Zhao J, Cai R, et al. (1)H NMR-based metabolomics of paired tissue, serum and urine samples reveals an optimized panel of biofluids metabolic biomarkers for esophageal cancer. Front Oncol (2023) 13:1082841. doi: 10.3389/fonc.2023.1082841
49. Huang L, Wang L, Hu X, Chen S, Tao Y, Su H, et al. Machine learning of serum metabolic patterns encodes early-stage lung adenocarcinoma. Nat Commun (2020) 11(1):3556. doi: 10.1038/s41467-019-14242-7
50. Shu X, Cai H, Lan Q, Cai Q, Ji BT, Zheng W, et al. A prospective investigation of circulating metabolome identifies potential biomarkers for gastric cancer risk. Cancer Epidemiol Biomarkers Prevention: Publ Am Assoc Cancer Res Cosponsored By Am Soc Prev Oncol (2021) 30(9):1634–42. doi: 10.1158/1055-9965.EPI-20-1633
51. Li X, Liu L, Li N, Jia Q, Wang X, Zuo L, et al. Metabolomics based plasma biomarkers for diagnosis of oral squamous cell carcinoma and oral erosive lichen planus. J Cancer (2022) 13(1):76–87. doi: 10.7150/jca.59777
52. Xie Y, Meng WY, Li RZ, Wang YW, Qian X, Chan C, et al. Early lung cancer diagnostic biomarker discovery by machine learning methods. Trans Oncol (2021) 14(1):100907. doi: 10.1016/j.tranon.2020.100907
53. Zhao R, Ren S, Li C, Guo K, Lu Z, Tian L, et al. Biomarkers for pancreatic cancer based on tissue and serum metabolomics analysis in a multicenter study. Cancer Med (2023) 12(4):5158–71. doi: 10.1002/cam4.5296
54. Cang S, Liu R, Mu K, Tang Q, Cui H, Bi K, et al. Assessment of plasma amino acids, purines, tricarboxylic acid cycle metabolites, and lipids levels in NSCLC patients based on LC-MS/MS quantification. J Pharm Biomed Analysis (2022) 221:114990. doi: 10.1016/j.jpba.2022.114990
55. Shestakova KM, Moskaleva NE, Boldin AA, Rezvanov PM, Shestopalov AV, Rumyantsev SA, et al. Targeted metabolomic profiling as a tool for diagnostics of patients with non-small-cell lung cancer. Sci Rep (2023) 13(1):11072. doi: 10.1038/s41598-023-38140-7
56. Chen S, Li C, Qin Z, Song L, Zhang S, Sun C, et al. Serum metabolomic profiles for distinguishing lung cancer from pulmonary tuberculosis: identification of rapid and noninvasive biomarker. J Infect Dis (2023) 228(9):1154–65. doi: 10.1093/infdis/jiad175
57. Stepien M, Keski-Rahkonen P, Kiss A, Robinot N, Duarte-Salles T, Murphy N, et al. Metabolic perturbations prior to hepatocellular carcinoma diagnosis: Findings from a prospective observational cohort study. Int J Cancer (2021) 148(3):609–25. doi: 10.1002/ijc.33236
58. Wei Y, Jasbi P, Shi X, Turner C, Hrovat J, Liu L, et al. Early breast cancer detection using untargeted and targeted metabolomics. J Proteome Res (2021) 20(6):3124–33. doi: 10.1021/acs.jproteome.1c00019
59. Zhu ZJ, Qi Z, Zhang J, Xue WH, Li LF, Shen ZB, et al. Untargeted metabolomics analysis of esophageal squamous cell carcinoma discovers dysregulated metabolic pathways and potential diagnostic biomarkers. J Cancer (2020) 11(13):3944–54. doi: 10.7150/jca.41733
60. Luo X, Liu J, Wang H, Lu H. Metabolomics identified new biomarkers for the precise diagnosis of pancreatic cancer and associated tissue metastasis. Pharmacol Res (2020) 156:104805. doi: 10.1016/j.phrs.2020.104805
61. Huang Y, Du S, Liu J, Huang W, Liu W, Zhang M, et al. Diagnosis and prognosis of breast cancer by high-performance serum metabolic fingerprints. Proc Natl Acad Sci United States America (2022) 119(12):e2122245119. doi: 10.1073/pnas.2122245119
62. Qi SA, Wu Q, Chen Z, Zhang W, Zhou Y, Mao K, et al. High-resolution metabolomic biomarkers for lung cancer diagnosis and prognosis. Sci Rep (2021) 11(1):11805. doi: 10.1038/s41598-021-91276-2
63. Huang S, Guo Y, Li ZW, Shui G, Tian H, Li BW, et al. Identification and validation of plasma metabolomic signatures in precancerous gastric lesions that progress to cancer. JAMA Netw Open (2021) 4(6):e2114186. doi: 10.1001/jamanetworkopen.2021.14186
64. Lin Z, Li H, He C, Yang M, Chen H, Yang X, et al. Metabolomic biomarkers for the diagnosis and post-transplant outcomes of AFP negative hepatocellular carcinoma. Front Oncol (2023) 13:1072775. doi: 10.3389/fonc.2023.1072775
65. Zhang L, Zheng J, Ahmed R, Huang G, Reid J, Mandal R, et al. A high-performing plasma metabolite panel for early-stage lung cancer detection. Cancers (2020) 12(3):622. doi: 10.3390/cancers12030622
66. Yang Z, Song Z, Chen Z, Guo Z, Jin H, Ding C, et al. Metabolic and lipidomic characterization of Malignant pleural effusion in human lung cancer. J Pharm Biomed Analysis (2020) 180:113069. doi: 10.1016/j.jpba.2019.113069
67. Wolrab D, Jirásko R, Cífková E, Höring M, Mei D, Chocholoušková M, et al. Lipidomic profiling of human serum enables detection of pancreatic cancer. Nat Commun (2022) 13(1):124. doi: 10.1038/s41467-021-27765-9
68. Wang J, Yang WY, Li XH, Xu B, Yang YW, Zhang B, et al. Study on potential markers for diagnosis of renal cell carcinoma by serum untargeted metabolomics based on UPLC-MS/MS. Front Physiol (2022) 13:996248. doi: 10.3389/fphys.2022.996248
69. Yuan Y, Yang C, Wang Y, Sun M, Bi C, Sun S, et al. Functional metabolome profiling may improve individual outcomes in colorectal cancer management implementing concepts of predictive, preventive, and personalized medical approach. EPMA J (2022) 13(1):39–55. doi: 10.1007/s13167-021-00269-8
70. Mahajan UM, Oehrle B, Sirtl S, Alnatsha A, Goni E, Regel I, et al. Independent validation and assay standardization of improved metabolic biomarker signature to differentiate pancreatic ductal adenocarcinoma from chronic pancreatitis. Gastroenterology (2022) 163(5):1407–22. doi: 10.1053/j.gastro.2022.07.047
71. Zeleznik OA, Clish CB, Kraft P, Avila-Pacheco J, Eliassen AH, Tworoger SS. Circulating lysophosphatidylcholines, phosphatidylcholines, ceramides, and sphingomyelins and ovarian cancer risk: A 23-year prospective study. J Natl Cancer Institute (2020) 112(6):628–36. doi: 10.1093/jnci/djz195
72. Jiang N, Zhang Z, Chen X, Zhang G, Wang Y, Pan L, et al. Plasma lipidomics profiling reveals biomarkers for papillary thyroid cancer diagnosis. Front Cell Dev Biol (2021) 9:682269. doi: 10.3389/fcell.2021.682269
73. Wang H, Luo Y, Chen H, Hou H, Hu Q, Ji M. Non-targeted serum lipidomics analysis and potential biomarkers of laryngeal cancer based on UHPLC-QTOF-MS. Metabolites (2022) 12(11):1087. doi: 10.3390/metabo12111087
74. Kühn T, Stepien M, López-Nogueroles M, Damms-MaChado A, Sookthai D, Johnson T, et al. Prediagnostic plasma bile acid levels and colon cancer risk: A prospective study. J Natl Cancer Institute (2020) 112(5):516–24. doi: 10.1093/jnci/djz166
75. Dallal CM, Tice JA, Buist DS, Bauer DC, Lacey JV Jr., Cauley JA, et al. Estrogen metabolism and breast cancer risk among postmenopausal women: a case-cohort study within B~FIT. Carcinogenesis (2014) 35(2):346–55. doi: 10.1093/carcin/bgt367
76. Moore SC, Matthews CE, Ou Shu X, Yu K, Gail MH, Xu X, et al. Endogenous estrogens, estrogen metabolites, and breast cancer risk in postmenopausal chinese women. J Natl Cancer Institute (2016) 108(10):djw103. doi: 10.1093/jnci/djw103
77. Kim S, Campbell J, Yoo W, Taylor JA, Sandler DP. Systemic levels of estrogens and PGE(2) synthesis in relation to postmenopausal breast cancer risk. Cancer Epidemiol Biomarkers Prevention: Publ Am Assoc Cancer Res Cosponsored By Am Soc Prev Oncol (2017) 26(3):383–8. doi: 10.1158/1055-9965.EPI-16-0556
78. Bifarin OO, Gaul DA, Sah S, Arnold RS, Ogan K, Master VA, et al. Machine learning-enabled renal cell carcinoma status prediction using multiplatform urine-based metabolomics. J Proteome Res (2021) 20(7):3629–41. doi: 10.1021/acs.jproteome.1c00213
79. Łuczykowski K, Warmuzińska N, Operacz S, Stryjak I, Bogusiewicz J, Jacyna J, et al. Metabolic evaluation of urine from patients diagnosed with high grade (HG) bladder cancer by SPME-LC-MS method. Molecules (Basel Switzerland) (2021) 26(8):2194. doi: 10.3390/molecules26082194
80. Arponen M, Jalava N, Widjaja N, Ivaska KK. Glucose transporters GLUT1, GLUT3, and GLUT4 have different effects on osteoblast proliferation and metabolism. Front Physiol (2022) 13:1035516. doi: 10.3389/fphys.2022.1035516
81. Mahfoud F, Schlaich M, Kindermann I, Ukena C, Cremers B, Brandt MC, et al. Effect of renal sympathetic denervation on glucose metabolism in patients with resistant hypertension: a pilot study. Circulation (2011) 123(18):1940–6. doi: 10.1161/CIRCULATIONAHA.110.991869
82. Amendola CR, Mahaffey JP, Parker SJ, Ahearn IM, Chen WC, Zhou M, et al. KRAS4A directly regulates hexokinase 1. Nature (2019) 576(7787):482–6. doi: 10.1038/s41586-019-1832-9
83. Morita M, Sato T, Nomura M, Sakamoto Y, Inoue Y, Tanaka R, et al. PKM1 confers metabolic advantages and promotes cell-autonomous tumor cell growth. Cancer Cell (2018) 33(3):355–67.e7. doi: 10.1016/j.ccell.2018.02.004
84. Kam CS, Ho DW, Ming VS, Tian L, Sze KM, Zhang VX, et al. PFKFB4 drives the oncogenicity in TP53-mutated hepatocellular carcinoma in a phosphatase-dependent manner. Cell Mol Gastroenterol Hepatol (2023) 15(6):1325–50. doi: 10.1016/j.jcmgh.2023.02.004
85. Warburg O, Wind F, Negelein E. THE METABOLISM OF TUMORS IN THE BODY. J Gen Physiol (1927) 8(6):519–30. doi: 10.1085/jgp.8.6.519
86. Hui S, Ghergurovich JM, Morscher RJ, Jang C, Teng X, Lu W, et al. Glucose feeds the TCA cycle via circulating lactate. Nature (2017) 551(7678):115–8. doi: 10.1038/nature24057
87. Fan Q, Yang L, Zhang X, Ma Y, Li Y, Dong L, et al. Autophagy promotes metastasis and glycolysis by upregulating MCT1 expression and Wnt/β-catenin signaling pathway activation in hepatocellular carcinoma cells. J Exp Clin Cancer Res: CR (2018) 37(1):9. doi: 10.1186/s13046-018-0673-y
88. Fang Y, Liu W, Tang Z, Ji X, Zhou Y, Song S, et al. Monocarboxylate transporter 4 inhibition potentiates hepatocellular carcinoma immunotherapy through enhancing T cell infiltration and immune attack. Hepatol (Baltimore Md) (2023) 77(1):109–23. doi: 10.1002/hep.32348
89. Thomas MP, Mills SJ, Potter BV. The “Other” Inositols and their phosphates: synthesis, biology, and medicine (with recent advances in myo-inositol chemistry). Angewandte Chemie (International ed English) (2016) 55(5):1614–50. doi: 10.1002/anie.201502227
90. Zhou L, Sheng W, Jia C, Shi X, Cao R, Wang G, et al. Musashi2 promotes the progression of pancreatic cancer through a novel ISYNA1-p21/ZEB-1 pathway. J Cell Mol Med (2020) 24(18):10560–72. doi: 10.1111/jcmm.15676
91. Guo X, Li HH, Hu J, Duan YX, Ren WG, Guo Q, et al. ISYNA1 is overexpressed in bladder carcinoma and regulates cell proliferation and apoptosis. Biochem Biophys Res Commun (2019) 519(2):246–52. doi: 10.1016/j.bbrc.2019.08.129
92. Yoo HC, Park SJ, Nam M, Kang J, Kim K, Yeo JH, et al. A variant of SLC1A5 is a mitochondrial glutamine transporter for metabolic reprogramming in cancer cells. Cell Metab (2020) 31(2):267–83.e12. doi: 10.1016/j.cmet.2019.11.020
93. Yoo HC, Yu YC, Sung Y, Han JM. Glutamine reliance in cell metabolism. Exp Mol Med (2020) 52(9):1496–516. doi: 10.1038/s12276-020-00504-8
94. Metallo CM, Gameiro PA, Bell EL, Mattaini KR, Yang J, Hiller K, et al. Reductive glutamine metabolism by IDH1 mediates lipogenesis under hypoxia. Nature (2011) 481(7381):380–4.
95. Tong Y, Guo D, Lin SH, Liang J, Yang D, Ma C, et al. SUCLA2-coupled regulation of GLS succinylation and activity counteracts oxidative stress in tumor cells. Mol Cell (2021) 81(11):2303–16.e8. doi: 10.1016/j.molcel.2021.04.002
96. Zhang B, Chen Y, Shi X, Zhou M, Bao L, Hatanpaa KJ, et al. Regulation of branched-chain amino acid metabolism by hypoxia-inducible factor in glioblastoma. Cell Mol Life Sci: CMLS (2021) 78(1):195–206. doi: 10.1007/s00018-020-03483-1
97. Li JT, Yin M, Wang D, Wang J, Lei MZ, Zhang Y, et al. BCAT2-mediated BCAA catabolism is critical for development of pancreatic ductal adenocarcinoma. Nat Cell Biol (2020) 22(2):167–74. doi: 10.1038/s41556-019-0455-6
98. Lei MZ, Li XX, Zhang Y, Li JT, Zhang F, Wang YP, et al. Acetylation promotes BCAT2 degradation to suppress BCAA catabolism and pancreatic cancer growth. Signal Transduction Targeted Ther (2020) 5(1):70. doi: 10.1038/s41392-020-0168-0
99. Ducker GS, Rabinowitz JD. One-carbon metabolism in health and disease. Cell Metab (2017) 25(1):27–42. doi: 10.1016/j.cmet.2016.08.009
100. Ma C, Zheng K, Jiang K, Zhao Q, Sha N, Wang W, et al. The alternative activity of nuclear PHGDH contributes to tumour growth under nutrient stress. Nat Metab (2021) 3(10):1357–71. doi: 10.1038/s42255-021-00456-x
101. Bouzidi A, Magnifico MC, Paiardini A, Macone A, Boumis G, Giardina G, et al. Cytosolic serine hydroxymethyltransferase controls lung adenocarcinoma cells migratory ability by modulating AMP kinase activity. Cell Death Dis (2020) 11(11):1012. doi: 10.1038/s41419-020-03215-0
102. Wang LT, Chiou SS, Chai CY, Hsi E, Yokoyama KK, Wang SN, et al. Intestine-specific homeobox gene ISX integrates IL6 signaling, tryptophan catabolism, and immune suppression. Cancer Res (2017) 77(15):4065–77. doi: 10.1158/0008-5472.CAN-17-0090
103. Bian Y, Li W, Kremer DM, Sajjakulnukit P, Li S, Crespo J, et al. Cancer SLC43A2 alters T cell methionine metabolism and histone methylation. Nature (2020) 585(7824):277–82. doi: 10.1038/s41586-020-2682-1
104. Youness RA, Gad AZ, Sanber K, Ahn YJ, Lee GJ, Khallaf E, et al. Targeting hydrogen sulphide signaling in breast cancer. J Adv Res (2021) 27:177–90. doi: 10.1016/j.jare.2020.07.006
105. Wang YH, Huang JT, Chen WL, Wang RH, Kao MC, Pan YR, et al. Dysregulation of cystathionine γ-lyase promotes prostate cancer progression and metastasis. EMBO Rep (2019) 20(10):e45986. doi: 10.15252/embr.201845986
106. Olivares O, Mayers JR, Gouirand V, Torrence ME, Gicquel T, Borge L, et al. Collagen-derived proline promotes pancreatic ductal adenocarcinoma cell survival under nutrient limited conditions. Nat Commun (2017) 8:16031. doi: 10.1038/ncomms16031
107. Wang D, Wang L, Zhang Y, Yan Z, Liu L, Chen G. PYCR1 promotes the progression of non-small-cell lung cancer under the negative regulation of miR-488. Biomed Pharmacother Biomed Pharmacother (2019) 111:588–95. doi: 10.1016/j.biopha.2018.12.089
108. Oscilowska I, Rolkowski K, Baszanowska W, Huynh TYL, Lewoniewska S, Nizioł M, et al. Proline dehydrogenase/proline oxidase (PRODH/POX) is involved in the mechanism of metformin-induced apoptosis in C32 melanoma cell line. Int J Mol Sci (2022) 23(4). doi: 10.3390/ijms23042354
109. Sousa CM, Biancur DE, Wang X, Halbrook CJ, Sherman MH, Zhang L, et al. Pancreatic stellate cells support tumour metabolism through autophagic alanine secretion. Nature (2016) 536(7617):479–83. doi: 10.1038/nature19084
110. Carta G, Murru E, Banni S, Manca C. Palmitic acid: physiological role, metabolism and nutritional implications. Front Physiol (2017) 8:902. doi: 10.3389/fphys.2017.00902
111. Kwan HY, Fu X, Liu B, Chao X, Chan CL, Cao H, et al. Subcutaneous adipocytes promote melanoma cell growth by activating the Akt signaling pathway: role of palmitic acid. J Biol Chem (2014) 289(44):30525–37. doi: 10.1074/jbc.M114.593210
112. Yu W, Lei Q, Yang L, Qin G, Liu S, Wang D, et al. Contradictory roles of lipid metabolism in immune response within the tumor microenvironment. J Hematol Oncol (2021) 14(1):187. doi: 10.1186/s13045-021-01200-4
113. Zhao H, Wu L, Yan G, Chen Y, Zhou M, Wu Y, et al. Inflammation and tumor progression: signaling pathways and targeted intervention. Signal Transduction Targeted Ther (2021) 6(1):263. doi: 10.1038/s41392-021-00658-5
114. Lin M, Liao W, Dong M, Zhu R, Xiao J, Sun T, et al. Exosomal neutral sphingomyelinase 1 suppresses hepatocellular carcinoma via decreasing the ratio of sphingomyelin/ceramide. FEBS J (2018) 285(20):3835–48. doi: 10.1111/febs.14635
115. Felzen A, van Wessel DBE, Gonzales E, Thompson RJ, Jankowska I, Shneider BL, et al. Genotype-phenotype relationships of truncating mutations, p.E297G and p.D482G in bile salt export pump deficiency. JHEP Reports: Innovation Hepatol (2023) 5(2):100626.
116. Fu T, Coulter S, Yoshihara E, Oh TG, Fang S, Cayabyab F, et al. FXR regulates intestinal cancer stem cell proliferation. Cell (2019) 176(5):1098–112.e18. doi: 10.1016/j.cell.2019.01.036
117. Jia W, Xie G, Jia W. Bile acid-microbiota crosstalk in gastrointestinal inflammation and carcinogenesis. Nat Rev Gastroenterol Hepatol (2018) 15(2):111–28. doi: 10.1038/nrgastro.2017.119
118. Folkerd EJ, Dowsett M. Influence of sex hormones on cancer progression. J Clin Oncol: Off J Am Soc Clin Oncol (2010) 28(26):4038–44. doi: 10.1200/JCO.2009.27.4290
119. Al-Shami K, Awadi S, Khamees A, Alsheikh AM, Al-Sharif S, Ala’ Bereshy R, et al. Estrogens and the risk of breast cancer: A narrative review of literature. Heliyon (2023) 9(9):e20224. doi: 10.1016/j.heliyon.2023.e20224
120. Arslan AA, Koenig KL, Lenner P, Afanasyeva Y, Shore RE, Chen Y, et al. Circulating estrogen metabolites and risk of breast cancer in postmenopausal women. Cancer Epidemiol Biomarkers Prevention: Publ Am Assoc Cancer Res Cosponsored By Am Soc Prev Oncol (2014) 23(7):1290–7. doi: 10.1158/1055-9965.EPI-14-0009
121. Sampson JN, Falk RT, Schairer C, Moore SC, Fuhrman BJ, Dallal CM, et al. Association of estrogen metabolism with breast cancer risk in different cohorts of postmenopausal women. Cancer Res (2017) 77(4):918–25. doi: 10.1158/0008-5472.CAN-16-1717
122. Stanczyk FZ. The 2-/16α-hydroxylated estrogen ratio-breast cancer risk hypothesis: insufficient evidence for its support. J Steroid Biochem Mol Biol (2020) 201:105685. doi: 10.1016/j.jsbmb.2020.105685
123. Gaikwad NW, Yang L, Muti P, Meza JL, Pruthi S, Ingle JN, et al. The molecular etiology of breast cancer: evidence from biomarkers of risk. Int J Cancer (2008) 122(9):1949–57. doi: 10.1002/ijc.23329
124. Pruthi S, Yang L, Sandhu NP, Ingle JN, Beseler CL, Suman VJ, et al. Evaluation of serum estrogen-DNA adducts as potential biomarkers for breast cancer risk. J Steroid Biochem Mol Biol (2012) 132(1-2):73–9. doi: 10.1016/j.jsbmb.2012.02.002
125. Reding KW, Han CJ, Whittington D, Zahid M, Rogan EG, Langford D, et al. Risk of breast cancer associated with estrogen DNA adduct biomarker. Cancer Epidemiol Biomarkers Prevention: Publ Am Assoc Cancer Res Cosponsored By Am Soc Prev Oncol (2020) 29(10):2096–9. doi: 10.1158/1055-9965.EPI-20-0133
126. Oginawati K, Anka AAH, Susetyo SH, Febriana SA, Tanziha I, Prakoeswa CRS. Urinary hippuric acid level as a biological indicator of toluene exposure on batik workers. Heliyon (2021) 7(8):e07775. doi: 10.1016/j.heliyon.2021.e07775
127. Pruss KM, Chen H, Liu Y, Van Treuren W, Higginbottom SK, Jarman JB, et al. Host-microbe co-metabolism via MCAD generates circulating metabolites including hippuric acid. Nat Commun (2023) 14(1):512. doi: 10.1038/s41467-023-36138-3
128. Rizvi ZA, Dalal R, Sadhu S, Kumar Y, Kumar S, Gupta SK, et al. High-salt diet mediates interplay between NK cells and gut microbiota to induce potent tumor immunity. Sci Adv (2021) 7(37):eabg5016. doi: 10.1126/sciadv.abg5016
129. Wang Y, Wang S, Li L, Zou Y, Liu B, Fang X. Microfluidics-based molecular profiling of tumor-derived exosomes for liquid biopsy. (2023) 4(2):20220048. doi: 10.1002/VIW.20220048
130. Smolarz M, Kurczyk A, Jelonek K, Żyła J, Mielańczyk Ł, Sitkiewicz M, et al. The lipid composition of serum-derived small extracellular vesicles in participants of a lung cancer screening study. Cancers (2021) 13(14). doi: 10.3390/cancers13143414
131. Luo P, Mao K, Xu J, Wu F, Wang X, Wang S, et al. Metabolic characteristics of large and small extracellular vesicles from pleural effusion reveal biomarker candidates for the diagnosis of tuberculosis and Malignancy. J Extracellular Vesicles (2020) 9(1):1790158. doi: 10.1080/20013078.2020.1790158
132. Buentzel J, Klemp HG, Kraetzner R, Schulz M, Dihazi GH, Streit F, et al. Metabolomic profiling of blood-derived microvesicles in breast cancer patients. Int J Mol Sci (2021) 22(24). doi: 10.3390/ijms222413540
133. Liu P, Wang W, Wang F, Fan J, Guo J, Wu T, et al. Alterations of plasma exosomal proteins and motabolies are associated with the progression of castration-resistant prostate cancer. J Trans Med (2023) 21(1):40. doi: 10.1186/s12967-022-03860-3
134. Clos-Garcia M, Loizaga-Iriarte A, Zuñiga-Garcia P, Sánchez-Mosquera P, Rosa Cortazar A, González E, et al. Metabolic alterations in urine extracellular vesicles are associated to prostate cancer pathogenesis and progression. J Extracellular Vesicles (2018) 7(1):1470442. doi: 10.1080/20013078.2018.1470442
135. Skotland T, Ekroos K, Kauhanen D, Simolin H, Seierstad T, Berge V, et al. Molecular lipid species in urinary exosomes as potential prostate cancer biomarkers. Eur J Cancer (Oxford England: 1990) (2017) 70:122–32. doi: 10.1016/j.ejca.2016.10.011
136. Eylem CC, Yilmaz M, Derkus B, Nemutlu E, Camci CB, Yilmaz E, et al. Untargeted multi-omic analysis of colorectal cancer-specific exosomes reveals joint pathways of colorectal cancer in both clinical samples and cell culture. Cancer Letters (2020) 469:186–94. doi: 10.1016/j.canlet.2019.10.038
137. Bestard-Escalas J, Reigada R, Reyes J, de la Torre P, Liebisch G, Barceló-Coblijn G. Fatty acid unsaturation degree of plasma exosomes in colorectal cancer patients: A promising biomarker. Int J Mol Sci (2021) 22(10). doi: 10.3390/ijms22105060
138. Sanchez JI, Jiao J, Kwan SY, Veillon L, Warmoes MO, Tan L, et al. Lipidomic profiles of plasma exosomes identify candidate biomarkers for early detection of hepatocellular carcinoma in patients with cirrhosis. Cancer Prev Res (Philadelphia Pa) (2021) 14(10):955–62. doi: 10.1158/1940-6207.CAPR-20-0612
139. Palacios-Ferrer JL, García-Ortega MB, Gallardo-Gómez M, García M, Díaz C, Boulaiz H, et al. Metabolomic profile of cancer stem cell-derived exosomes from patients with Malignant melanoma. Mol Oncol (2021) 15(2):407–28. doi: 10.1002/1878-0261.12823
140. Paolino G, Huber V, Camerini S, Casella M, Macone A, Bertuccini L, et al. The fatty acid and protein profiles of circulating CD81-positive small extracellular vesicles are associated with disease stage in melanoma patients. Cancers (2021) 13(16). doi: 10.3390/cancers13164157
141. Mandarano M, Orecchini E, Bellezza G, Vannucci J, Ludovini V, Baglivo S, et al. Kynurenine/tryptophan ratio as a potential blood-based biomarker in non-small cell lung cancer. Int J Mol Sci (2021) 22(9). doi: 10.3390/ijms22094403
142. Liu Z, Wang L, Du M, Liang Y, Liang M, Li Z, et al. Plasm metabolomics study in pulmonary metastatic carcinoma. J Oncol (2022) 2022:9460019. doi: 10.1155/2022/9460019
143. Chen KC, Tsai SW, Zhang X, Zeng C, Yang HY. The investigation of the volatile metabolites of lung cancer from the microenvironment of Malignant pleural effusion. Sci Rep (2021) 11(1):13585. doi: 10.1038/s41598-021-93032-y
144. Wang FX, Chen K, Huang FQ, Alolga RN, Ma J, Wu ZX, et al. Cerebrospinal fluid-based metabolomics to characterize different types of brain tumors. J Neurol (2020) 267(4):984–93. doi: 10.1007/s00415-019-09665-7
145. Olkowicz M, Rosales-Solano H, Kulasingam V, Pawliszyn J. SPME-LC/MS-based serum metabolomic phenotyping for distinguishing ovarian cancer histologic subtypes: a pilot study. Sci Rep (2021) 11(1):22428. doi: 10.1038/s41598-021-00802-9
146. Ossoliński K, Ruman T, Copié V, Tripet BP, Nogueira LB, Nogueira K, et al. Metabolomic and elemental profiling of blood serum in bladder cancer. J Pharm Analysis (2022) 12(6):889–900. doi: 10.1016/j.jpha.2022.08.004
147. Kurokawa GA, Hamamoto Filho PT, Delafiori J, Galvani AF, de Oliveira AN, Dias-Audibert FL, et al. Differential plasma metabolites between high- and low-grade meningioma cases. Int J Mol Sci (2022) 24(1). doi: 10.3390/ijms24010394
148. Zhang Y, Cheng Y, Qin L, Liu Y, Huang S, Dai L, et al. Plasma metabolomics for the assessment of the progression of non-small cell lung cancer. Int J Biol Markers (2023) 38(1):37–45. doi: 10.1177/03936155221137359
149. Wang PP, Song X, Zhao XK, Wei MX, Gao SG, Zhou FY, et al. Serum metabolomic profiling reveals biomarkers for early detection and prognosis of esophageal squamous cell carcinoma. Front Oncol (2022) 12:790933. doi: 10.3389/fonc.2022.790933
150. Geijsen A, van Roekel EH, van Duijnhoven FJB, Achaintre D, Bachleitner-Hofmann T, Baierl A, et al. Plasma metabolites associated with colorectal cancer stage: Findings from an international consortium. Int J Cancer (2020) 146(12):3256–66. doi: 10.1002/ijc.32666
151. Rao J, Wan X, Tou F, He Q, Xiong A, Chen X, et al. Molecular characterization of advanced colorectal cancer using serum proteomics and metabolomics. Front Mol Biosci (2021) 8:687229. doi: 10.3389/fmolb.2021.687229
152. Buszewska-Forajta M, Monedeiro F, Gołębiowski A, Adamczyk P, Buszewski B. Citric acid as a potential prostate cancer biomarker determined in various biological samples. Metabolites (2022) 12(3). doi: 10.3390/metabo12030268
153. Huang JY, Larose TL, Luu HN, Wang R, Fanidi A, Alcala K, et al. Circulating markers of cellular immune activation in prediagnostic blood sample and lung cancer risk in the Lung Cancer Cohort Consortium (LC3). Int J Cancer (2020) 146(9):2394–405. doi: 10.1002/ijc.32555
154. Huang X, Fang J, Lai W, Hu Y, Li L, Zhong Y, et al. IL-6/STAT3 axis activates glut5 to regulate fructose metabolism and tumorigenesis. Int J Biol Sci (2022) 18(9):3668–75. doi: 10.7150/ijbs.68990
155. Azuma K, Xiang H, Tagami T, Kasajima R, Kato Y, Karakawa S, et al. Clinical significance of plasma-free amino acids and tryptophan metabolites in patients with non-small cell lung cancer receiving PD-1 inhibitor: a pilot cohort study for developing a prognostic multivariate model. J Immunother Cancer (2022) 10(5). doi: 10.1136/jitc-2021-004420
156. Zhang Y, Wang J, Dai N, Han P, Li J, Zhao J, et al. Alteration of plasma metabolites associated with chemoradiosensitivity in esophageal squamous cell carcinoma via untargeted metabolomics approach. BMC Cancer (2020) 20(1):835. doi: 10.1186/s12885-020-07336-9
157. Chen Z, Dai Y, Huang X, Chen K, Gao Y, Li N, et al. Combined metabolomic analysis of plasma and tissue reveals a prognostic risk score system and metabolic dysregulation in esophageal squamous cell carcinoma. Front Oncol (2020) 10:1545. doi: 10.3389/fonc.2020.01545
158. Hipperson L, Hadden WJ, Nahm CB, Gill AJ, Samra JS, Dona A, et al. Urinary metabolite prognostic biomarker panel for pancreatic ductal adenocarcinomas. Biochim Biophys Acta Gen Subjects (2021) 1865(11):129966. doi: 10.1016/j.bbagen.2021.129966
159. Lee CW, Yu MC, Lin G, Chiu JC, Chiang MH, Sung CM, et al. Serum metabolites may be useful markers to assess vascular invasion and identify normal alpha-fetoprotein in hepatocellular carcinoma undergoing liver resection: a pilot study. World J Surg Oncol (2020) 18(1):121. doi: 10.1186/s12957-020-01885-w
160. Han J, Han ML, Xing H, Li ZL, Yuan DY, Wu H, et al. Tissue and serum metabolomic phenotyping for diagnosis and prognosis of hepatocellular carcinoma. Int J Cancer (2020) 146(6):1741–53. doi: 10.1002/ijc.32599
161. Pan G, Ma Y, Suo J, Li W, Zhang Y, Qin S, et al. Discovering biomarkers in peritoneal metastasis of gastric cancer by metabolomics. OncoTargets Ther (2020) 13:7199–211. doi: 10.2147/OTT.S245663
162. Boakye D, Jansen L, Schöttker B, Jansen E, Schneider M, Halama N, et al. Blood markers of oxidative stress are strongly associated with poorer prognosis in colorectal cancer patients. Int J Cancer (2020) 147(9):2373–86. doi: 10.1002/ijc.33018
163. Morozumi K, Kawasaki Y, Maekawa M, Takasaki S, Sato T, Shimada S, et al. Predictive model for recurrence of renal cell carcinoma by comparing pre- and postoperative urinary metabolite concentrations. Cancer Sci (2022) 113(1):182–94. doi: 10.1111/cas.15180
164. Gupta A, Bansal N, Mitash N, Kumar D, Kumar M, Sankhwar SN, et al. NMR-derived targeted serum metabolic biomarkers appraisal of bladder cancer: A pre- and post-operative evaluation. J Pharm Biomed Analysis (2020) 183:113134. doi: 10.1016/j.jpba.2020.113134
165. Zhuang J, Yang X, Zheng Q, Li K, Cai L, Yu H, et al. Metabolic profiling of bladder cancer patients’ Serum reveals their sensitivity to neoadjuvant chemotherapy. Metabolites (2022) 12(6). doi: 10.3390/metabo12060558
166. Hishinuma E, Shimada M, Matsukawa N, Saigusa D, Li B, Kudo K, et al. Wide-targeted metabolome analysis identifies potential biomarkers for prognosis prediction of epithelial ovarian cancer. Toxins (2021) 13(7). doi: 10.3390/toxins13070461
167. Yin X, Yang J, Zhang M, Wang X, Xu W, Price CH, et al. Serum metabolic fingerprints on bowl-shaped submicroreactor chip for chemotherapy monitoring. ACS Nano (2022) 16(2):2852–65. doi: 10.1021/acsnano.1c09864
168. Kiebish MA, Cullen J, Mishra P, Ali A, Milliman E, Rodrigues LO, et al. Multi-omic serum biomarkers for prognosis of disease progression in prostate cancer. J Trans Med (2020) 18(1):10. doi: 10.1186/s12967-019-02185-y
169. Mi M, Liu Z, Zheng X, Wen Q, Zhu F, Li J, et al. Serum metabolomic profiling based on GC/MS helped to discriminate Diffuse Large B-cell Lymphoma patients with different prognosis. Leukemia Res (2021) 111:106693. doi: 10.1016/j.leukres.2021.106693
170. Zhao R, Xie Y, Yang B, Wang C, Huang Q, Han Y, et al. Identification of metabolic biomarkers to predict treatment outcome and disease progression in multiple myeloma. Am J Cancer Res (2020) 10(11):3935–46.
171. Markley JL, Brüschweiler R, Edison AS, Eghbalnia HR, Powers R, Raftery D, et al. The future of NMR-based metabolomics. Curr Opin Biotechnol (2017) 43:34–40. doi: 10.1016/j.copbio.2016.08.001
172. Nagana Gowda GA, Raftery D. NMR metabolomics methods for investigating disease. Analytical Chem (2023) 95(1):83–99. doi: 10.1021/acs.analchem.2c04606
173. Keun HC, Beckonert O, Griffin JL, Richter C, Moskau D, Lindon JC, et al. Cryogenic probe 13C NMR spectroscopy of urine for metabonomic studies. Analytical Chem (2002) 74(17):4588–93. doi: 10.1021/ac025691r
174. Zaikin VG, Borisov RS. Options of the main derivatization approaches for analytical ESI and MALDI mass spectrometry. Crit Rev Analytical Chem (2022) 52(6):1287–342. doi: 10.1080/10408347.2021.1873100
175. Alonso A, Marsal S, Julià A. Analytical methods in untargeted metabolomics: state of the art in 2015. Front Bioengineering Biotechnol (2015) 3:23. doi: 10.3389/fbioe.2015.00023
176. Xu Z, Huang Y, Hu C, Du L, Du YA, Zhang Y, et al. Efficient plasma metabolic fingerprinting as a novel tool for diagnosis and prognosis of gastric cancer: a large-scale, multicentre study. Gut (2023) 72(11):2051–67. doi: 10.1136/gutjnl-2023-330045
177. Lippa KA, Aristizabal-Henao JJ, Beger RD, Bowden JA, Broeckling C, Beecher C, et al. Reference materials for MS-based untargeted metabolomics and lipidomics: a review by the metabolomics quality assurance and quality control consortium (mQACC). Metabolomics: Off J Metabolomic Society (2022) 18(4):24. doi: 10.1007/s11306-021-01848-6
178. Gathungu RM, Kautz R, Kristal BS, Bird SS, Vouros P. The integration of LC-MS and NMR for the analysis of low molecular weight trace analytes in complex matrices. Mass Spectrometry Rev (2020) 39(1-2):35–54. doi: 10.1002/mas.21575
179. Huang HP, Chen CH, Chang KH, Lee MS, Lee CF, Chao YH, et al. Prediction of clinically significant prostate cancer through urine metabolomic signatures: A large-scale validated study. J Trans Med (2023) 21(1):714. doi: 10.1186/s12967-023-04424-9
180. Chen X, Shu W, Zhao L, Wan J. Advanced mass spectrometric and spectroscopic methods coupled with machine learning for in vitro diagnosis. (2023) 4(1):20220038. doi: 10.1002/VIW.20220038
181. Liu Z, Liu H, Chen Z, Deng C, Zhou L, Chen S, et al. Identification of a novel plasma metabolite panel as diagnostic biomarker for hepatocellular carcinoma. Clin Chim Acta; Int J Clin Chem (2023) 543:117302. doi: 10.1016/j.cca.2023.117302
182. Costantini S, Di Gennaro E, Capone F, De Stefano A, Nasti G, Vitagliano C, et al. Plasma metabolomics, lipidomics and cytokinomics profiling predict disease recurrence in metastatic colorectal cancer patients undergoing liver resection. Front Oncol (2022) 12:1110104. doi: 10.3389/fonc.2022.1110104
183. Li X, Yi Y, Wu T, Chen N, Gu X, Xiang L, et al. Integrated microbiome and metabolome analysis reveals the interaction between intestinal flora and serum metabolites as potential biomarkers in hepatocellular carcinoma patients. Front Cell Infect Microbiol (2023) 13:1170748. doi: 10.3389/fcimb.2023.1170748
Keywords: liquid biopsy, metabolomics, cancer biomarkers, diagnosis, prognosis
Citation: Wang W, Zhen S, Ping Y, Wang L and Zhang Y (2024) Metabolomic biomarkers in liquid biopsy: accurate cancer diagnosis and prognosis monitoring. Front. Oncol. 14:1331215. doi: 10.3389/fonc.2024.1331215
Received: 31 October 2023; Accepted: 26 January 2024;
Published: 07 February 2024.
Edited by:
Maria Elena Pisanu, National Institute of Health (ISS), ItalyReviewed by:
Luciana N. S. Andrade, University of São Paulo, BrazilCopyright © 2024 Wang, Zhen, Ping, Wang and Zhang. This is an open-access article distributed under the terms of the Creative Commons Attribution License (CC BY). The use, distribution or reproduction in other forums is permitted, provided the original author(s) and the copyright owner(s) are credited and that the original publication in this journal is cited, in accordance with accepted academic practice. No use, distribution or reproduction is permitted which does not comply with these terms.
*Correspondence: Liping Wang, d2xwQHp6dS5lZHUuY24=; Yi Zhang, eWl6aGFuZ0B6enUuZWR1LmNu
†These authors have contributed equally to this work and share first authorship
Disclaimer: All claims expressed in this article are solely those of the authors and do not necessarily represent those of their affiliated organizations, or those of the publisher, the editors and the reviewers. Any product that may be evaluated in this article or claim that may be made by its manufacturer is not guaranteed or endorsed by the publisher.
Research integrity at Frontiers
Learn more about the work of our research integrity team to safeguard the quality of each article we publish.