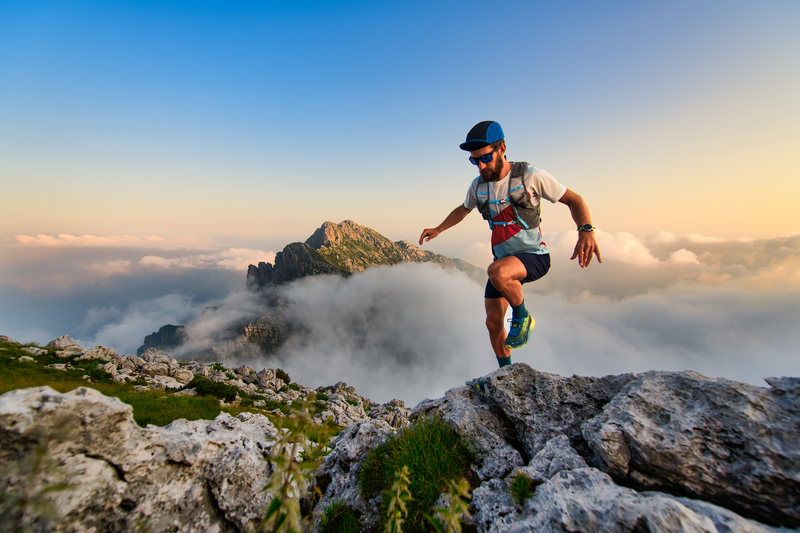
94% of researchers rate our articles as excellent or good
Learn more about the work of our research integrity team to safeguard the quality of each article we publish.
Find out more
ORIGINAL RESEARCH article
Front. Oncol. , 05 February 2024
Sec. Cancer Epidemiology and Prevention
Volume 14 - 2024 | https://doi.org/10.3389/fonc.2024.1305684
Aim: This research aimed to explore the causal impact of blood metabolites on oral cancer using a two-sample Mendelian randomization (MR) analysis. The study endeavored to identify potential biomarkers for oral cancer’s clinical management.
Materials and methods: Based on the large individual-level datasets from UK Biobank as well as GWAS summary datasets, we first constructed genetic risk scores (GRSs) of 486 human blood metabolites and evaluated the effect on oral cancer. Various statistical methods, including inverse variance weighted (IVW), MR-Egger, and weighted median, among others, were employed to analyze the potential causal relationship between blood metabolites and oral cancer. The sensitivity analyses were conducted using Cochran’s Q tests, funnel plots, leave-one-out analyses, and MR-Egger intercept tests.
Results: 29 metabolites met the stringent selection criteria. Out of these, 14 metabolites demonstrated a positive association with oral cancer risk, while 15 metabolites indicated a protective effect against oral cancer. The IVW-derived estimates were significant, and the results were consistent across different statistical methodologies. Both the Cochran Q test and the MR-Egger intercept test indicated no heterogeneity and pleiotropy.
Conclusion: This MR study offers evidence of the role specific blood metabolites play in oral cancer, pinpointing several with potential risk or protective effects. These findings could be helpful for new diagnostic tools and treatments for oral cancer. While the results are promising, additional research is necessary to fully validate and refine these conclusions. This study serves as a foundational step towards more comprehensive understandings in the future.
Oral cancer, classified under head and neck malignancies, stands as the world’s sixth most common cancer, with oral squamous cell carcinoma constituting over 90% of these cases (1, 2). Several oral lesions, such as lichen planus, leukoplakia, erythroplakia, and oral sub-mucous fibrosis, have been recognized as potential precursors to malignant oral disorders (3). With limited understanding of oral cancer’s pathophysiology, emphasizing early detection is crucial to enhance survival rates.
Recently, the merger of metabolomics into systems biology has provided a novel approach to understanding disease processes. Through metabolomics, it’s possible to discern the biological complexity of diseases by identifying changes in metabolites and metabolic routes. Metabolic reprogramming is a distinctive feature of cancer cells, as many studies have highlighted (4–7). Interestingly, these cells prefer glycolysis for their energy needs, even in oxygen-rich conditions, a shift known as the Warburg effect or aerobic glycolysis (5). This metabolic shift contributes to both the initiation and progression of cancer. Besides the Warburg effect, cancer cells undergo diverse metabolic transformations, including shifts in lipid and amino acid pathways, such as glutaminolysis (4, 6, 7). Nonetheless, a thorough understanding of oral cancer’s metabolic traits remains elusive. Investigating these metabolic changes linked to oral cancer could lead to the discovery of new biomarkers and a deeper comprehension of the disease’s development and advancement. Recent advancements have combined metabolomics with extensive genotyping, enabling the examination of the relationship between genetic variations and metabolic phenotypes through genome-wide association studies (GWASs) (8, 9). Numerous genetic locations connected to metabolic phenotypes have been identified through these studies (8). By utilizing extensive GWAS data, genetic risk scores (GRSs) and Mendelian randomization (MR) have become instrumental in unraveling the etiology of complex diseases by effectively managing unidentified variables (10).
MR, an emerging analytical tool, uses genetic variations associated with exposures to assess the potential causal effects of these exposures on observed outcomes (11). Notably, MR is often likened to a “natural” randomized controlled trial (RCT) because alleles are randomly distributed during gamete production, possibly minimizing confounders and biases due to reverse causality (12). The enduring nature of genetic influences, together with the extensive GWAS data on oral diseases (13–19), makes MR studies an effective alternative to conventional, long-term clinical trials focused on preventing oral cancer, avoiding their significant costs and lengthy monitoring periods.
MR design has been applied to identify the potential mediators of oral cancer and related cancer. Gormley et al.’s study showed strong evidence of smoking’s causal effect on oral/oropharyngeal cancer and suggested an underestimated effect of alcohol when adjusted for smoking (14). Also, Chen et al. identified a possible causal link between inflammatory bowel disease and oral cavity cancer (15). For the relationship between cancers and blood metabolomic, Guo et al. applied two-sample MR to identify blood metabolites associated with lacunar stroke, finding 15 known and 14 unknown metabolites with potential implications in disease pathogenesis (20). Liu et al. utilized bidirectional Mendelian randomization on Chinese individuals, revealing 58 causal relationships between the gut microbiome and blood metabolites, with a notable link between fecal Oscillibacter and Alistipes and decreased triglyceride concentration (21).
However, to our knowledge, there is no MR study to assess the causal role of blood metabolites on oral cancer. In this study, we performed the first two-sample MR analysis of the GWAS summary data containing blood metabolites and oral cancer, revealed the causal impact of blood metabolites on oral cancer, provided new biomarkers for the clinical management of oral cancer.
MR study adheres to three fundamental instrumental variable (IV) assumptions: (1) the genetic variants must correlate with the exposure; (2) these variants should be free from confounding factors; and (3) they should only affect the outcome via the exposure (Figure 1). Data summaries for the blood metabolites and oral cancer used in this research were sourced from publicly accessible GWASs, primarily based on cohorts of European descent (8). This MR study followed the guidelines of Strengthening the Reporting of Observational Studies in Epidemiology using Mendelian Randomization (STROBE-MR).
Figure 1 Diagram of the MR analysis. Assumption 1, genetic instruments are strongly associated with the exposures of interest; Assumption 2, genetic instruments are independent of confounding factors; Assumption 3, genetic instruments are not associated with outcome and affect outcome only via exposures. IVW, inverse variance weighted; LD, linkage disequilibrium; LOO analysis, leave-one-out analysis; MR-PRESSO, MR-Pleiotropy RESidual sum and outlier; WMedine, weighted medine; SNPs, single nucleotide polymorphisms; WM, weighted mode; SMode, simple mode.
Blood metabolite genetic data were retrieved from the metabolomics GWAS server (https://metabolomics.helmholtz-muenchen.de/gwas/), which represents the most comprehensive study on blood metabolite genetic loci presently (Supplementary Table S1). The research by Shin et al. pinpointed around 2.1 million SNPs connected with 486 human metabolites by integrating Genome-wide association scans with high-throughput metabolic profiling (8), with metabolites prefixed by ‘X-’ having unidentified chemical properties. This research involved 7,824 Europeans: 1,768 from Germany’s KORA F4 study and 6,056 from the UK Twin Study. Among the 486 metabolites, 107 remain undefined chemically, while 309 have undergone chemical validation and are classified into eight primary metabolic groups, as cited in the Kyoto Encyclopedia of Genes and Genomes (KEGG) database. These groups encompass amino acid, carbohydrate, cofactors and vitamin, energy, lipid, nucleotide, peptide, and xenobiotic metabolism.
The genetic association data for oral cancer originated from the UK Biobank’s GWAS (22, 23). This initiative registered close to 500,000 participants aged between 40 and 69 (24) (Supplementary Table S2). The UK Biobank’s medical records utilized the International Classification of Diseases, Tenth Revision (ICD-10-CM), and Ninth Revision (ICD-9-CM). The discussed GWAS comprised a sample of 372,373 European-descent individuals, including 7.72 million SNPs. This data is accessible via the IEU GWAS database at https://gwas.mrcieu.ac.uk/. A linear mixed model (LMM) association method was applied, facilitated by BOLT-LMM (v2.3) (25). Addressing the population structure involved SNP selection post-filtration based on multiple criteria, including MAF > 0.01, genotyping rate > 0.015, a Hardy-Weinberg equilibrium p-value below 0.0001, and LD pruning to an limit of 0.1 using PLINKv2.00.
For the MR evaluation, SNPs linked to blood metabolites at a genome-wide significance threshold from prior GWASs were utilized. These SNPs were chosen ensuring no linkage disequilibrium (LD) with other SNPs, maintaining an r2 below 0.1 within a 500 kb clumping radius. In cases where SNPs exceeded the r2 = 0.1 limit, only the SNP with the strongest association (smallest P value) with the metabolite was selected. This selection strategy is consistent with methods commonly employed in earlier studies (26, 27). To counteract potential bias from inferior instruments, the R2 and F statistics for each SNP were determined using the following formulas:
Here, β indicates the genetic variant’s effect size; EAF represents its effect allele frequency; se(β) is the standard error for this effect size; N denotes the sample size for the exposure; and k is the count of SNPs. SNPs with an F value under 10 were deemed weak and subsequently discarded. The subsequent procedure extracted outcome-associated SNPs, discarding those correlated with the outcome . Exposure and outcome SNP harmonization followed, with the removal of palindromic and allele inconsistent SNPs. The third assumption was satisfied by excluding outcome-associated SNPs with . The analysis culminated in an MR study on metabolites represented by over two SNPs (28).
The potential causal relationship between blood metabolites and oral cancer was explored using multiple techniques in this research. These methodologies included inverse variance weighted (IVW), MR-Egger, weighted median, weighted mode, MR-PRESSO, and simple model strategies. The overarching impact of blood metabolites on oral cancer was determined using a meta-analysis approach, combined with Wald estimates for each SNP via the IVW method. In the scenario where horizontal pleiotropy is absent, IVW results remain unbiased (29). The MR-Egger regression, based on the InSIDE assumption that instrument strength is not linked to a direct effect, gauges pleiotropy via its intercept term. An intercept term of zero in the MR-Egger regression indicates alignment with IVW results, implying an absence of horizontal pleiotropy (30). The weighted median method can provide accurate causal inferences, even if as many as 50% of the IVs are considered invalid (31). In instances where the InSIDE assumption is challenged, the weighted mode estimate offers enhanced power, reduced bias, and a lower type I error rate compared to MR-Egger regression (31). The MR-PRESSO approach identifies and corrects for horizontal pleiotropy by eliminating significant outliers. Yet, the MR-PRESSO outlier test is contingent on InSIDE premises and mandates that a majority of the genetic markers serve as valid instruments (32). While the simple mode method may exhibit lower precision, it typically presents a diminished bias relative to alternative techniques (31).
To corroborate significant outcomes, tests for heterogeneity and horizontal pleiotropy were undertaken using meta-analytical methods, including the modified Cochran Q statistic and the MR Egger intercept test for deviation (33). To alleviate the impact of horizontal pleiotropy attributed to a single SNP, a leave-one-out analysis was performed, systematically omitting one SNP at a time. The “TwoSampleMR” (34) and “MRPRESSO” R packages, version 4.1.3, facilitated these evaluations.
Furthermore, the Steiger test was executed to counteract potential biases from reverse causality (35). The causal inference direction could be erroneous if the explained variance of IVs in oral cancer surpasses that of blood metabolites.
Upon stringent quality control of IVs, 486 metabolites were included in the MR analysis. These IVs comprised SNPs ranging from 4 to 136 (with X-14056 genetically represented by 4 SNPs and tryptophan having the highest representation with 136 SNPs. The F statistics of all SNPs associated with metabolites were greater than 10 (Supplementary Table S3). Subsequent IVW analysis, paired with supplemental and sensitivity evaluations, pinpointed 29 metabolites that satisfied the stringent selection criteria as potential candidates (Figure 2). These consisted of 15 identified metabolites and 14 with unknown chemical identities. The identified metabolites were chemically categorized into Amino Acid, Vitamin, Alkaloid, Steroid, Lipid, Peptide, Nucleoside and Xenobiotics.
Figure 2 Forest plot of Mendelian randomization estimates between blood metabolites and oral cancer. The figure showed the IVW estimates of significantly oral cancer-associated blood metabolites. The blue dots represent the IVW estimates, and the blue bars represent the 95% confidence intervals of IVW estimates of protective factors. The red dots represent the IVW estimates, and the red bars represent the 95% confidence intervals of IVW estimates of risk factors.
The positive association between the risk of oral cancer and 14 blood metabolites were as follow (Figure 2): tryptophan (OR 1.0032, 95% CI: 1.0005–1.0059, p=0.0183), pentadecanoate (15:0) (OR 1.0031, 95% CI: 1.0001–1.0061, p=0.0427), laurate (12:0) (OR 1.0032, 95% CI: 1.0002–1.0063, p=0.0378), X-03088 (OR 1.0030, 95% CI: 1.0009–1.0052, p=0.0059), X-03094 (OR 1.0036, 95% CI: 1.0008–1.0064, p=0.0107), 1-oleoylglycerol (1-monoolein) (OR 1.0019, 95% CI: 1.0001–1.0037, p=0.0397), X-10510 (OR 1.0023, 95% CI: 1.0000–1.0046, p=0.0479), N-(2-furoyl)glycine (OR 1.0004, 95% CI: 1.0000–1.0008, p=0.0396), X-03056 (OR 1.0026, 95% CI: 1.0006–1.0047, p=0.0121), X-12435 (OR 1.0006, 95% CI: 1.0001–1.0012, p=0.0181), X-12680 (OR 1.0030, 95% CI: 1.0000–1.0060, p=0.0481), X-12786 (OR 1.0020, 95% CI: 1.0003–1.0038, p=0.0230), 1-palmitoylglycerophosphoethanolamine (OR 1.0028, 95% CI: 1.0005–1.0052, p=0.0185), palmitoyl sphingomyelin (OR 1.0031, 95% CI: 1.0004–1.0057, p=0.0234). This suggests that these blood metabolites may increase the risk of oral cancer.
On the other hand, we found that 15 blood metabolites were associated with a reduced risk of oral cancer (Figure 2): uridine (OR 0.9954, 95% CI: 0.9909–1.0000, p=0.0485), allantoin (OR 0.9982, 95% CI: 0.9964–1.0000, p=0.0467), alpha-tocopherol (OR 0.9959, 95% CI: 0.9928–0.9991, p=0.0109), X-03003 (OR 0.9929, 95% CI: 0.9880–0.9978, p=0.0046), X-06350 (OR 0.9963, 95% CI: 0.9938–0.9987, p=0.0031), saccharin (OR 0.9991, 95% CI: 0.9982–1.0000, p=0.0487), pyridoxate (OR 0.9971, 95% CI: 0.9944–0.9997, p=0.0279), X-06126 (OR 0.9979, 95% CI: 0.9960–0.9998, p=0.0285), X-11905 (OR 0.9989, 95% CI: 0.9979–1.0000, p=0.0403), 1-methylxanthine (OR 0.9984, 95% CI: 0.9970–0.9999, p=0.0324), pro-hydroxy-pro (OR 0.9968, 95% CI: 0.9936–0.9999, p=0.0430), X-13429 (OR 0.9990, 95% CI: 0.9980–0.9999, p=0.0351), X-14056 (OR 0.9958, 95% CI: 0.9917–1.0000, p=0.0479), X-14541 (OR 0.9988, 95% CI: 0.9978–0.9999, p=0.0343), 4-androsten-3beta,17beta-diol disulfate 2* (OR 0.9974, 95% CI: 0.9955–0.9993, p=0.0070). This suggests that these blood metabolites may have a protective effect against oral cancer.
In summation, IVW-derived estimates were significant (p<0.05), and there was consistency in direction and magnitude across IVW, MR-Egger, Weighted median, Weighted mode and Simple mode estimates (Figure 3; Supplementary Table S4). Scatter plots for identified metabolites across various tests are displayed in Figure 4. Both the Cochran Q test (p>0.05) and the MR-Egger intercept test (p>0.05) strongly supported the lack of heterogeneity and pleiotropy (Table 1). Leave-one-out analysis affirmed that no individual SNP introduced bias into the MR estimation (Supplementary Figure S1). The funnel plots were showed on Supplementary Figure S2. In addition, the Steiger test revealed that the causality between genetically proxied metabolites and oral cancer was not violated by reverse causal effects (Supplementary Table S5).
Figure 3 Preliminary MR analyses for the associations between blood metabolites and the risk of oral cancer. The circle from the outer to the inner represented the IVW, MR-Egger, weighted medine, simple mode and weighted mode, respectively. Blood metabolites was classified in order, Amino Acid, Nucleoside, Alkaloid, Vitamin, Xenobiotics, Lipid, Peptide, Steroid, Unknown. The shades of color were reflections of the magnitude of the p-value as the label inside the circle. (MR, Mendelian randomization; IVW, inverse variance-weighted; WMedine, weighted median; SMode, simple mode; WMode, weighted mode.).
Figure 4 Scatter plots of the MR estimates for the significant causality of blood metabolites and the risk of oral cancer. The causal effect of the 15 identified metabolites on oral cancer. The lines implying positive correlations moved diagonally upward from left to right, indicating a facilitative effect of metabolites on oral cancer. The horizontal and vertical lines indicated each correlation’s 95% confidence interval. The lines implying negative correlations move diagonally downward from left to right, indicating the inhibitory effect of blood metabolites on oral cancer. (MR, Mendelian randomization; SNPs, single nucleotide polymorphisms).
In recent decades, extensive research has emphasized the significant roles of metabolic reprogramming and energy metabolism in the proliferation and metastasis of oral cancer cells. Within the realm of normal cellular function, alterations in metabolism not only bolster cellular proliferation and division but also predispose cells to oncogenic transformation, a critical aspect in the context of oral cancer. In the course of conducting a Mendelian randomization study on the impact of blood metabolites on oral cancer, a multitude of blood metabolites were identified to be significantly associated with the risk of oral cancer. Our experimental results revealed that metabolites such as tryptophan, uridine, allantoin, pentadecanoate, α-tocopherol, and laurate were correlated with the risk of oral cancer.
Amino acids play a crucial role in oral cancer by providing essential building blocks and energy for tumor cell proliferation and are intimately involved in metabolic processes linked to tumor growth and survival (36). Gupta et al. discerned that metabolites including glutamine, propionate, acetone, and choline could accurately differentiate oral cancer from healthy controls, partially resonating with our findings (37). Synchronous luminescence spectroscopy has been employed to distinguish oral cancer tissues. Notable observations were made concerning peak shifts and broadening for tryptophan, NADH, and FAD in oral cancer tissues (38). These findings suggest substantial biochemical and micro-environmental alterations at the cellular level. When we pair these observations with our positive data, especially that of tryptophan (OR 1.0032, 95% CI: 1.0005–1.0059, p=0.0183), it underscores the significance of tryptophan as a crucial endogenous fluorophore in oral cancer. The changes in tryptophan not only echo the biochemical shifts within cells but might also be indicative of the progression and development of the cancer. However,. Our study also observed a down-regulation in the levels of pro-hydroxy-pro (OR 0.9968, 95% CI: 0.9936–0.9999, p=0.0430) in oral cancer patients, a finding corroborated by Yonezawa et al., who reported a down-regulation in the serum levels of several amino acids in head and neck cancer patients (39). Further analysis aligns our findings with the study by Kong et al., who through NMR analysis found that the up-regulation of lactate, choline, and glucose and the down-regulation of proline, valine, isoleucine, aspartate, and 2-hydroxybutyric acid may contribute to oral cancer development (40). This further affirms the significance of plasma metabolites as potential metabolic biomarkers for oral carcinogenesis.
Our observation is the consistent demonstration of the anticancer activity of α-tocopherol across multiple studies, especially regarding its therapeutic potential for oral cancer (41–43). α-tocopherol acts by inducing apoptosis to inhibit the growth of cancer cells. Moreover, it serves as a scavenger of free radicals, combating cancer cells through oxidative stress. While its antitumor activity may be perceived as weaker compared to cisplatin, indications of apoptosis onset appear earlier for α-tocopherol. Its lack of cytotoxicity further illustrates its potential as an antitumor agent (41). Alpha-tocopherol has displayed effective antitumor activity against ORL-48 in experiments (43). Alongside elevated serum levels of retinol, it’s been associated with a decreased risk of oral cancer. These findings suggest that alpha-tocopherol might serve as a promising therapeutic agent for oral cancer. Variations in tryptophan concentrations hint at biochemical and micro-environmental changes occurring in oral cancer tissues. In our Mendelian experiment, α-tocopherol has an OR value of 0.9959, with a 95% CI of 0.9928-0.9991 and p=0.0109, indicating a significant association with a specific condition or phenotype. This bolsters its potential therapeutic implications.
In our study, we initially linked allantoin to oral cancer with a down-regulation (OR 0.9982, 95% CI: 0.9964–1.0000, p=0.0467). While focusing on oral cancer, broader research suggests allantoin’s effects might extend to other cancers, indicating a need to understand its systemic impact beyond the oral cavity. Allantoin could increases under stress conditions, has shown cytotoxicity against various age-related cancers, especially in environments simulating oxidative stress. In our study, 1-palmitoylglycerophosphoethanolamine (OR 1.0028, 95% CI: 1.0005–1.0052, p=0.0185), has an up-regulation in oral cancer patients. In an Alpha-Tocopherol, Beta-Carotene Cancer Prevention Study, 1-palmitoylglycerophosphoethanolamine has reported a significant association with retinol levels, suggesting its potential role in the metabolic pathways influenced by vitamin A (44, 45). Randomized trials and meta-analyses indicated a potential increased risk for certain cancers with high serum retinol concentrations or after supplementation (46). Both cases highlight the importance of studying these metabolites in a wider cancer context.
Our study also unveiled results that diverge from previous research. For example, our research found a significant negative correlation between the levels of uridine and pyridoxate and the risk of oral cancer, a finding not reported in previous studies. This may suggest a diverse role of these metabolites in the onset and progression of oral cancer. Additionally, our study discovered new metabolites related to oral cancer risk, such as X-03003 and X-03088, which have not been previously reported to be associated with oral cancer risk. These novel findings may contribute to a more comprehensive understanding of the metabolic mechanisms of oral cancer and offer new potential biomarkers for the early diagnosis and prevention of oral cancer.
Several limitations exist within this study. Firstly, the exposure of interest at the genome-wide level had a restricted number of SNPs. This was addressed by applying slightly relaxed thresholds for the MR analysis, mirroring practices in previous research. Nonetheless, with F-statistic values for the chosen SNPs surpassing 10, the robustness of our IVs is indicated. The Steiger test results further affirm the validity of our threshold approach by consistently supporting the causal direction. Secondly, our MR analysis exclusively utilized GWAS data from European ancestry individuals, limiting ethnic variability. Consequently, the applicability of these findings to diverse populations necessitates further investigation and validation. Thirdly, the MR estimation’s precision is inherently tied to sample size, emphasizing the need to augment the sample size for result validation. While MR analysis sheds light on disease etiology, it’s imperative to corroborate our findings through rigorous RCTs and foundational research before clinical integration.
This MR study offers evidence of the role specific blood metabolites play in oral cancer, pinpointing several with potential risk or protective effects. These findings could be helpful for new diagnostic tools and treatments for oral cancer. While the results are promising, additional research is necessary to fully validate and refine these conclusions. This study serves as a foundational step towards more comprehensive understandings in the future.
The original contributions presented in the study are included in the article/Supplementary Material, further inquiries can be directed to the corresponding author/s.
All the data used in this research can be found in public databases. No additional ethical approval was required.
ZH: Conceptualization, Formal analysis, Methodology, Project administration, Resources, Software, Supervision, Visualization, Writing – original draft, Writing – review & editing. ZX: Data curation, Investigation, Resources, Writing – original draft. QY: Data curation, Investigation, Resources, Writing – original draft. XP: Data curation, Investigation, Resources, Writing – original draft. PS: Data curation, Investigation, Resources, Writing – original draft. DZ: Data curation, Investigation, Resources, Writing – original draft. JZ: Data curation, Investigation, Resources, Writing – original draft. ZL: Conceptualization, Funding acquisition, Project administration, Writing – review & editing. RD: Conceptualization, Investigation, Project administration, Writing – original draft.
The author(s) declare financial support was received for the research, authorship, and/or publication of this article. This work was supported by the National Natural Science Foundation of China (Nos., 82201135), General project of Jiangsu Provincial Health Commission (No. M2021077), “2015” Cultivation Program for Reserve Talents for Academic Leaders of Nanjing Stomatological School, Medical School of Nanjing University (No., 0223A204)
We express our gratitude to Shin et al.’s study for providing open access to the summary association statistics data. Additionally, our heartfelt appreciation goes out to all the investigators and participants of the UK Biobank for making individual-level datasets publicly accessible and for their invaluable contributions to these studies.
The authors declare that the research was conducted in the absence of any commercial or financial relationships that could be construed as a potential conflict of interest.
All claims expressed in this article are solely those of the authors and do not necessarily represent those of their affiliated organizations, or those of the publisher, the editors and the reviewers. Any product that may be evaluated in this article, or claim that may be made by its manufacturer, is not guaranteed or endorsed by the publisher.
The Supplementary Material for this article can be found online at: https://www.frontiersin.org/articles/10.3389/fonc.2024.1305684/full#supplementary-material
1. Sung H, Ferlay J, Siegel RL, Laversanne M, Soerjomataram I, Jemal A, et al. Global cancer statistics 2020: GLOBOCAN estimates of incidence and mortality worldwide for 36 cancers in 185 countries. CA Cancer J Clin (2021) 71(3):209–49. doi: 10.3322/caac.21660
2. Vered M, Wright JM. Update from the 5th edition of the world health organization classification of head and neck tumors: Odontogenic and maxillofacial bone tumours. Head Neck Pathol (2022) 16(1):63–75. doi: 10.1007/s12105-021-01404-7
3. Chen X, Zhao Y. Human papillomavirus infection in oral potentially Malignant disorders and cancer. Arch Oral Biol (2017) 83:334–9. doi: 10.1016/j.archoralbio.2017.08.011
4. Yang L, Venneti S, Nagrath D. Glutaminolysis: A hallmark of cancer metabolism. Annu Rev BioMed Eng (2017) 19:163–94. doi: 10.1146/annurev-bioeng-071516-044546
5. Yu L, Chen X, Sun X, Wang L, Chen S. The glycolytic switch in tumors: How many players are involved? J Cancer (2017) 8(17):3430–40. doi: 10.7150/jca.21125
6. Nakagawa H, Hayata Y, Kawamura S, Yamada T, Fujiwara N, Koike K. Lipid metabolic reprogramming in hepatocellular carcinoma. Cancers (Basel) (2018) 10(11):447. doi: 10.3390/cancers10110447
7. Sun L, Suo C, Li ST, Zhang H, Gao P. Metabolic reprogramming for cancer cells and their microenvironment: Beyond the Warburg Effect. Biochim Biophys Acta Rev Cancer (2018) 1870(1):51–66. doi: 10.1016/j.bbcan.2018.06.005
8. Shin SY, Fauman EB, Petersen AK, Krumsiek J, Santos R, Huang J, et al. An atlas of genetic influences on human blood metabolites. Nat Genet (2014) 46(6):543–50. doi: 10.1038/ng.2982
9. Kettunen J, Demirkan A, Würtz P, Draisma HH, Haller T, Rawal R, et al. Genome-wide study for circulating metabolites identifies 62 loci and reveals novel systemic effects of LPA. Nat Commun (2016) 7:11122. doi: 10.1038/ncomms11122
10. Smith GD, Ebrahim S. 'Mendelian randomization': can genetic epidemiology contribute to understanding environmental determinants of disease? Int J Epidemiol (2003) 32(1):1–22. doi: 10.1093/ije/dyg070
11. Lawlor DA, Harbord RM, Sterne JA, Timpson N, Davey Smith G. Mendelian randomization: using genes as instruments for making causal inferences in epidemiology. Stat Med (2008) 27(8):1133–63. doi: 10.1002/sim.3034
12. Hemani G, Bowden J, Davey Smith G. Evaluating the potential role of pleiotropy in Mendelian randomization studies. Hum Mol Genet (2018) 27(R2):R195–r208. doi: 10.1093/hmg/ddy163
13. Dudding T, Johansson M, Thomas SJ, Brennan P, Martin RM, Timpson NJ. Assessing the causal association between 25-hydroxyvitamin D and the risk of oral and oropharyngeal cancer using Mendelian randomization. Int J Cancer (2018) 143(5):1029–36. doi: 10.1002/ijc.31377
14. Gormley M, Dudding T, Sanderson E, Martin RM, Thomas S, Tyrrell J, et al. A multivariable Mendelian randomization analysis investigating smoking and alcohol consumption in oral and oropharyngeal cancer. Nat Commun (2020) 11(1):6071. doi: 10.1038/s41467-020-19822-6
15. Chen G, Xie J, Liang T, Wang Y, Liao W, Song L, et al. Inflammatory Bowel disease promote oral cancer and pharyngeal cancer: new evidence of Mendelian randomization. Acta Otolaryngol (2022) 142(2):191–6. doi: 10.1080/00016489.2022.2035431
16. Gormley M, Dudding T, Kachuri L, Burrows K, Chong AHW, Martin RM, et al. Investigating the effect of sexual behaviour on oropharyngeal cancer risk: a methodological assessment of Mendelian randomization. BMC Med (2022) 20(1):40. doi: 10.1186/s12916-022-02233-3
17. Chen G, Xie J, Liu D, Zhang X, Tang A. Causal effects of education attainment on oral and oropharyngeal cancer: New evidence from a meta-analysis and Mendelian randomization study. Front Public Health (2023) 11:1132035. doi: 10.3389/fpubh.2023.1132035
18. Gormley M, Dudding T, Thomas SJ, Tyrrell J, Ness AR, Pring M, et al. Evaluating the effect of metabolic traits on oral and oropharyngeal cancer risk using Mendelian randomization. Elife (2023) 12:e82674. doi: 10.7554/eLife.82674.sa2
19. Gui L, He X, Tang L, Yao J, Pi J. Obesity and head and neck cancer risk: a mendelian randomization study. BMC Med Genomics (2023) 16(1):200. doi: 10.1186/s12920-023-01634-4
20. Guo MN, Hao XY, Tian J, Wang YC, Li JD, Fan Y, et al. Human blood metabolites and lacunar stroke: A Mendelian randomization study. Int J Stroke (2023) 18(1):109–16. doi: 10.1177/17474930221140792
21. Liu X, Tong X, Zou Y, Lin X, Zhao H, Tian L, et al. Mendelian randomization analyses support causal relationships between blood metabolites and the gut microbiome. Nat Genet (2022) 54(1):52–61. doi: 10.1038/s41588-021-00968-y
22. Bycroft C, Freeman C, Petkova D, Band G, Elliott LT, Sharp K, et al. The UK Biobank resource with deep phenotyping and genomic data. Nature (2018) 562(7726):203–9. doi: 10.1038/s41586-018-0579-z
23. Yang S, Zhou X. Accurate and scalable construction of polygenic scores in large biobank data sets. Am J Hum Genet (2020) 106(5):679–93. doi: 10.1016/j.ajhg.2020.03.013
24. Elsworth B, Lyon M, Alexander T, Liu Y, Matthews P, Hallett J, et al. The MRC IEU OpenGWAS data infrastructure. bioRxiv (2020). doi: 10.1101/2020.08.10.244293
25. Loh PR, Tucker G, Bulik-Sullivan BK, Vilhjálmsson BJ, Finucane HK, Salem RM, et al. Efficient Bayesian mixed-model analysis increases association power in large cohorts. Nat Genet (2015) 47(3):284–90. doi: 10.1038/ng.3190
26. Choi KW, Chen CY, Stein MB, Klimentidis YC, Wang MJ, Koenen KC, et al. Assessment of bidirectional relationships between physical activity and depression among adults: A 2-sample mendelian randomization study. JAMA Psychiatry (2019) 76(4):399–408. doi: 10.1001/jamapsychiatry.2018.4175
27. Yang J, Yan B, Zhao B, Fan Y, He X, Yang L, et al. Assessing the causal effects of human serum metabolites on 5 major psychiatric disorders. Schizophr Bull (2020) 46(4):804–13. doi: 10.1093/schbul/sbz138
28. Gill D, Brewer CF, Monori G, Trégouët DA, Franceschini N, Giambartolomei C, et al. Effects of genetically determined iron status on risk of venous thromboembolism and carotid atherosclerotic disease: A mendelian randomization study. J Am Heart Assoc (2019) 8(15):e012994. doi: 10.1161/JAHA.119.012994
29. Burgess S, Dudbridge F, Thompson SG. Combining information on multiple instrumental variables in Mendelian randomization: Comparison of allele score and summarized data methods. Stat Med (2016) 35(11):1880–906. doi: 10.1002/sim.6835
30. Bowden J, Davey Smith G, Burgess S. Mendelian randomization with invalid instruments: effect estimation and bias detection through Egger regression. Int J Epidemiol (2015) 44(2):512–25. doi: 10.1093/ije/dyv080
31. Hartwig FP, Davey Smith G, Bowden J. Robust inference in summary data Mendelian randomization via the zero modal pleiotropy assumption. Int J Epidemiol (2017) 46(6):1985–98. doi: 10.1093/ije/dyx102
32. Verbanck M, Chen CY, Neale B, Do R. Detection of widespread horizontal pleiotropy in causal relationships inferred from Mendelian randomization between complex traits and diseases. Nat Genet (2018) 50(5):693–8. doi: 10.1038/s41588-018-0099-7
33. Burgess S, Thompson SG. Interpreting findings from Mendelian randomization using the MR-Egger method. Eur J Epidemiol (2017) 32(5):377–89. doi: 10.1007/s10654-017-0255-x
34. Hemani G, Zheng J, Elsworth B, Wade KH, Haberland V, Baird D, et al. The MR-Base platform supports systematic causal inference across the human phenome. Elife (2018) 7:e34408. doi: 10.7554/eLife.34408
35. Hemani G, Tilling K, Davey Smith G. Orienting the causal relationship between imprecisely measured traits using GWAS summary data. PloS Genet (2017) 13(11):e1007081. doi: 10.1371/journal.pgen.1007081
36. Vettore L, Westbrook RL, Tennant DA. New aspects of amino acid metabolism in cancer. Br J Cancer (2020) 122(2):150–6. doi: 10.1038/s41416-019-0620-5
37. Gupta A, Gupta S, Mahdi AA. ¹H NMR-derived serum metabolomics of leukoplakia and squamous cell carcinoma. Clin Chim Acta (2015) 441:47–55. doi: 10.1016/j.cca.2014.12.003
38. Gnanatheepam E, Kanniyappan U, Dornadula K, Prakasarao A, Singaravelu G. Synchronous luminescence spectroscopy as a tool in the discrimination and characterization of oral cancer tissue. J Fluoresc (2019) 29(2):361–7. doi: 10.1007/s10895-018-02343-3
39. Yonezawa K, Nishiumi S, Kitamoto-Matsuda J, Fujita T, Morimoto K, Yamashita D, et al. Serum and tissue metabolomics of head and neck cancer. Cancer Genomics Proteomics (2013) 10(5):233–8.
40. Kong X, Yang X, Zhou J, Chen S, Li X, Jian F, et al. Analysis of plasma metabolic biomarkers in the development of 4-nitroquinoline-1-oxide-induced oral carcinogenesis in rats. Oncol Lett (2015) 9(1):283–9. doi: 10.3892/ol.2014.2619
41. Chitra S, Shyamaladevi CS. Modulatory action of α-tocopherol on erythrocyte membrane adenosine triphosphatase against radiation damage in oral cancer. J Membr Biol (2011) 240(2):83–8. doi: 10.1007/s00232-011-9346-x
42. Athirajan V, Razak IA, Thurairajah N, Ghani WM, Ching HN, Yang YH, et al. High serum level of retinol and α-tocopherol affords protection against oral cancer in a multiethnic population. Asian Pac J Cancer Prev (2014) 15(19):8183–9. doi: 10.7314/APJCP.2014.15.19.8183
43. Zulkapli R, Abdul Razak F, Zain RB. Vitamin E (α-tocopherol) exhibits antitumour activity on oral squamous carcinoma cells ORL-48. Integr Cancer Ther (2017) 16(3):414–25. doi: 10.1177/1534735416675950
44. Ioannidis JP. Contradicted and initially stronger effects in highly cited clinical research. Jama (2005) 294(2):218–28. doi: 10.1001/jama.294.2.218
45. Huang J, Panagiotou OA, Anic GM, Mondul AM, Liao LM, Derkach A, et al. Metabolomic profiling of serum retinol in the alpha-tocopherol, beta-carotene cancer prevention (ATBC) study. Sci Rep (2017) 7(1):10601. doi: 10.1038/s41598-017-09698-w
46. Fortmann SP, Burda BU, Senger CA, Lin JS, Whitlock EP. Vitamin and mineral supplements in the primary prevention of cardiovascular disease and cancer: An updated systematic evidence review for the U.S. Preventive Services Task Force. Ann Intern Med (2013) 159(12):824–34. doi: 10.7326/0003-4819-159-12-201312170-00729
Keywords: Mendelian randomization, blood metabolites, oral cancer, genome-wide association study, SNPs, metabolomics
Citation: Hu Z, Xu Z, Yue Q, Pan X, Shi P, Zhang D, Zhang J, Deng R and Lin Z (2024) The role of blood metabolites in oral cancer: insights from a Mendelian randomization approach. Front. Oncol. 14:1305684. doi: 10.3389/fonc.2024.1305684
Received: 09 October 2023; Accepted: 02 January 2024;
Published: 05 February 2024.
Edited by:
So Hee Kwon, Yonsei University, Republic of KoreaReviewed by:
Aishwarya Jala, Northwestern University, United StatesCopyright © 2024 Hu, Xu, Yue, Pan, Shi, Zhang, Zhang, Deng and Lin. This is an open-access article distributed under the terms of the Creative Commons Attribution License (CC BY). The use, distribution or reproduction in other forums is permitted, provided the original author(s) and the copyright owner(s) are credited and that the original publication in this journal is cited, in accordance with accepted academic practice. No use, distribution or reproduction is permitted which does not comply with these terms.
*Correspondence: Ziyang Hu, cHl4MTEyMjMzQDE2My5jb20=; Runzhi Deng, ZG9jdG9yZEAxNjMuY29t; Zitong Lin, bGlueml0b25nXzcxMEAxNjMuY29t
Disclaimer: All claims expressed in this article are solely those of the authors and do not necessarily represent those of their affiliated organizations, or those of the publisher, the editors and the reviewers. Any product that may be evaluated in this article or claim that may be made by its manufacturer is not guaranteed or endorsed by the publisher.
Research integrity at Frontiers
Learn more about the work of our research integrity team to safeguard the quality of each article we publish.