- Biomedical Device Innovation Center, Shenzhen Technology University, Shenzhen, China
X-ray mammography is currently considered the golden standard method for breast cancer screening, however, it has limitations in terms of sensitivity and specificity. With the rapid advancements in deep learning techniques, it is possible to customize mammography for each patient, providing more accurate information for risk assessment, prognosis, and treatment planning. This paper aims to study the recent achievements of deep learning-based mammography for breast cancer detection and classification. This review paper highlights the potential of deep learning-assisted X-ray mammography in improving the accuracy of breast cancer screening. While the potential benefits are clear, it is essential to address the challenges associated with implementing this technology in clinical settings. Future research should focus on refining deep learning algorithms, ensuring data privacy, improving model interpretability, and establishing generalizability to successfully integrate deep learning-assisted mammography into routine breast cancer screening programs. It is hoped that the research findings will assist investigators, engineers, and clinicians in developing more effective breast imaging tools that provide accurate diagnosis, sensitivity, and specificity for breast cancer.
1 Introduction
Breast cancer is one of the most prevalent cancers among females worldwide (1). Several factors, including gender, age, family history, obesity, and genetic mutations, contribute to the development of breast cancer (2). Early diagnosis with prompt treatment can significantly improve the 5-year survival rate of breast cancer (3). Medical imaging techniques like mammography and ultrasound are widely used for breast cancer detection (4, 5). Mammography utilizes low-dose X-rays to generate breast images that aid radiologists in identifying abnormalities like lumps, calcifications, and distortions (6). Mammography is recommended for women over 40, particularly those with a family history of breast cancer, as it effectively detects early-stage breast cancer (7). However, mammography has limitations, such as reduced sensitivity in women with dense breast tissue. To overcome these limitations, various imaging methods, such as digital breast tomosynthesis (DBT), ultrasound, magnetic resonance imaging (MRI), and positron emission tomography (PET), have been investigated as alternative tools for breast cancer screening.
DBT uses X-rays to generate three-dimensional breast images, which is particularly useful for detecting breast cancer in dense breasts (8). Compared to mammography, DBT provides higher accuracy and sensitivity in detecting breast cancer lesions. However, the interpretation of DBT images still faces inter-observer variability, which can affect its accuracy. Ultrasound imaging uses high-frequency sound waves to produce detailed images of breast tissue. Unlike mammography, ultrasound does not involve radiation, making it a safe method for detecting breast abnormalities, especially in women with dense breast tissue. Ultrasound helps evaluate abnormalities detected on a mammogram and can be used to monitor disease progression and assess treatment effectiveness (9). MRI has been recommended for women with high risks of breast cancer (10). PET utilizes a radioactive tracer to create breast images and is often used in conjunction with other imaging techniques, such as CT or MRI, to identify areas of cancer cells (11). Each of these imaging methods has its own set of advantages and disadvantages (12).
Artificial intelligence (AI) technologies have been extensively investigated to develop cancer prediction models (13, 14). AI-based models, such as machine learning (ML) algorithms, can analyze medical image datasets and patient characteristics to identify breast cancer or predict the risk of developing breast cancer. ML algorithms can extract quantitative features from medical images, such as mammograms or ultrasound images, through radiomics. AI-based prediction models can incorporate various cancer risk factors, including genetics, lifestyle, and environmental factors, to establish personalized imaging and treatment plans. In recent years, deep learning (DL) algorithms have emerged as promising AI tools to enhance the accuracy and efficiency of breast cancer detection (15). These data-driven techniques have the potential to revolutionize breast imaging by leveraging large amounts of data to automatically learn and identify complex patterns associated with malignancy.
This paper provides an overview of the recent developments in DL-based approaches and architectures used in mammography, along with their strengths and limitations. Additionally, the article highlights challenges and opportunities associated with integrating DL-based mammography to enhance breast cancer screening and diagnosis. The remaining sections of the paper are as follows: Section 2 describes the most popular medical imaging application for breast cancer detection. Section 3 discusses DL-based mammography techniques. Section 4 describes breast cancer prediction using DL techniques. Section 5 highlights the challenges and future research directions of DL approaches in mammography. Finally, Section 6 concludes the present study.
2 Medical imaging techniques for breast cancer detection
Medical imaging techniques have become essential in the diagnosis and management of breast cancer. This section provides an overview of several commonly used medical imaging techniques for breast cancer detection. Table 1 compares the most widely utilized medical imaging methods for breast cancer.
2.1 Mammography
This section presents the working principle, recent advancements, advantages, and disadvantages of mammography. Mammography is a well-established imaging modality used for breast cancer screening. It is a non-invasive technique that utilizes low-dose X-rays to generate high-resolution images of breast tissue. Mammography operates based on the principle of differential X-ray attenuation. The breast tissue is compressed between two plates, and a low-dose X-ray beam is directed through the breast to create an image. Different types of breast tissues, such as fatty, glandular, and cancerous tissue, attenuate X-rays differently. The X-rays that pass through the breast tissue are detected by a digital detector, and an image of the breast is formed. The resulting image is a two-dimensional projection of the breast tissue. In recent years, mammography has undergone significant advancements. Digital mammography has replaced film-screen mammography, leading to improved image quality and reduced radiation dose. Digital breast tomosynthesis (DBT), a 3D mammography technique, has enhanced breast cancer detection rates and reduced false positives. Automated breast ultrasound (ABUS) is another imaging modality used in conjunction with mammography for breast screening, particularly in women with dense breast tissue.
Numerous studies have investigated the effectiveness of mammography for breast cancer screening, demonstrating that it can reduce breast cancer mortality rates, especially for women aged 50-74 years. Additional screening with MRI or ultrasound may be recommended for women with higher risk of breast cancer, such as those with a family history or genetic predisposition. Several leading companies and research groups have achieved significant advancements in the past decade. For example, Hologic’s Genius 3D mammography technology provides higher-resolution 3D images, increasing detection rates while reducing false positives (20). However, it entails higher radiation exposure and higher costs compared to traditional mammography.
Other developments include GE Healthcare and Siemens Healthineers’ contrast-enhanced spectral mammography (CESM), which combines mammography with contrast-enhanced imaging to improve diagnostic accuracy (21). Artificial intelligence tools developed by companies like iCAD and ScreenPoint Medical have been utilized to enhance mammography interpretation, leading to earlier breast cancer detection (22). Gamma Medica and Dilon Technologies have introduced new breast imaging technologies, such as molecular breast imaging and breast-specific gamma imaging, which utilize different types of radiation to provide more detailed images of breast tissue (23).
The University of Chicago has made strides in contrast-enhanced mammography (CEM), which is more accurate in detecting invasive breast cancers than traditional mammography alone. CEM provides detailed images of breast tissue without ionizing radiation, though it is not widely available and may not be covered by insurance (24). The Karolinska Institute’s work on breast tomosynthesis has shown that it is more sensitive in detecting breast cancer than traditional mammography. Tomosynthesis provides a 3D image of the breast, facilitating the detection of small tumors and reducing the need for additional imaging tests. However, it exposes patients to slightly more radiation, takes longer to perform, and is more expensive (25).
Mammography has certain limitations, including limited sensitivity in women with dense breast tissue, false positives leading to unnecessary procedures, radiation exposure that accumulates over time, inability to distinguish between benign and malignant lesions, inaccuracy in detecting small cancers or cancers in certain breast regions, and limited utility in detecting specific types of breast cancer, such as inflammatory breast cancer. To address these limitations, various new imaging technologies, such as DBT, ultrasound elastography, and molecular breast imaging, have been proposed and investigated. These technologies aim to provide more accurate and reliable breast cancer detection, particularly in high-risk individuals. Future research directions for mammography include improving test accuracy, utilizing AI for image interpretation, and developing new techniques utilizing different radiation or contrast agents.
2.2 Digital breast tomosynthesis
DBT was first introduced in the early 2000s. Unlike traditional Mammography, DBT can generate three-dimensional images, leading to more accurate breast cancer detection by reducing tissue overlap. DBT is particularly effective in detecting small tumors and reducing false positive results compared to mammography (26). Additionally, it exposes patients to less radiation. However, DBT is more expensive and may not be covered by insurance for all patients. It also requires specialized equipment and training for interpretation, which may not be widely available in all areas.
2.3 Ultrasound
Ultrasound imaging is a non-invasive, relatively low-cost imaging technique that does not involve exposure to ionizing radiation. It can be used as an adjunct to mammography for breast cancer screening, especially in women with dense breast tissue. Nakano et al. (27) developed real-time virtual sonography (RVS) for breast lesion detection. RVS combines the advantages of ultrasound and MRI and can provide real-time, highly accurate images of breast lesions. However, RVS requires specialized equipment and software, and its diagnostic accuracy may depend on the operator. Standardization of RVS protocols and operator training may improve its accuracy and accessibility.
Zhang et al. (28) conducted a study on a computer-aided diagnosis (CAD) system called BIRADS-SDL for breast cancer detection using ultrasound images. BIRADS-SDL was compared with conventional stacked convolutional auto-encoder (SCAE) and semi-supervised deep learning (SDL) methods using original images as inputs, as well as an SCAE using BIRADS-oriented feature maps (BFMs) as inputs. The experimental results showed that BIRADS-SDL performed the best among the four networks, with classification accuracy of around 92.00 ± 2.38% and 83.90 ± 3.81% on two datasets. These findings suggest that BIRADS-SDL could be a promising method for effective breast ultrasound lesion CAD, particularly with small datasets. CAD systems can enhance the accuracy and efficiency of breast cancer detection while reducing inter-operator variability. However, CAD systems may produce false-positive or false-negative results, and their diagnostic accuracy may depend on the quality of the input images. Integrating CAD systems with other imaging modalities and developing algorithms to account for image quality variations may improve their accuracy and reliability (29).
GE Healthcare (USA) developed the Invenia Automated Breast Ultrasound (ABUS) 2.0, which improves breast cancer detection, especially in women with dense breasts, by providing high-resolution 3D ultrasound images (30). Siemens Healthineers (Germany) developed the ACUSON S2000 Automated Breast Volume Scanner (ABVS), which also provides high-resolution 3D ultrasound images for accurate breast cancer detection, particularly in women with dense breasts (31). These automated systems enhance breast cancer detection rates, improve workflow, and reduce operator variability.
Canon Medical Systems (Japan) developed the Aplio i-series ultrasound system with the iBreast package, which offers high-resolution breast imaging, leading to improved diagnostic performance for breast cancer detection. Invenia ABUS 2.0 and ACUSON S2000 ABVS are automated systems, while Aplio i-series with iBreast package requires manual scanning. The advantages of ABUS 2.0 and ACUSON S2000 ABVS include enhanced image quality, improved workflow, and reduced operator variability. However, they are more expensive than traditional mammography, and image interpretation may be time-consuming. Ultimately, the choice of system depends on the needs and preferences of healthcare providers and patients. Future research is likely to focus on improving the accuracy of ultrasound imaging techniques, developing new methods for detecting small calcifications, and reducing false-positive results.
2.4 Magnetic resonance imaging
MRI utilizes strong magnetic fields and radio waves to generate images of the body’s internal structures, making it one of the most important diagnostic tools. It has various applications, including the diagnosis and monitoring of neurological, musculoskeletal, cardiovascular, and oncological conditions. Its ability to image soft tissues makes it well-suited for breast imaging. Breast MRI is a non-invasive technique used for the detection and monitoring of breast cancer. It is often used in conjunction with mammography and ultrasound to provide a comprehensive evaluation of breast tissue.
Kuhl et al. (32) were the first to investigate post-contrast subtracted images and maximum-intensity projection for breast cancer screening with MRI. This approach offers advantages in terms of speed, cost-effectiveness, and patient accessibility. However, abbreviated MRI has limitations, including lower specificity and the potential for false positives. Mann et al. (33) studied ultrafast dynamic contrast-enhanced MRI for assessing lesion enhancement patterns. The use of new MRI sequences and image reconstruction techniques improved the specificity in distinguishing between malignant and benign lesions. Zhang et al. (34) explored a deep learning-based segmentation technique for breast MRI, which demonstrated accurate and consistent segmentation of breast regions. However, this method has limitations, such as its reliance on training data and potential misclassification.
MRI has several advantages, including the absence of ionizing radiation and increased accuracy in detecting small tumors within dense breast tissue. However, it is expensive, time-consuming, and associated with a higher false-positive rate. Future research directions involve developing faster and more efficient MRI techniques and utilizing AI techniques to enhance image analysis and interpretation.
Contrast-enhanced MRI (DCE-MRI) has recently become a crucial method in clinical practice for the detection and evaluation of breast cancer. Figure 1 illustrates the workflow of unsupervised analysis based on DCE-MRI radiomics features in breast cancer patients (35). Ming et al. (35) utilized DCE-MRI to calculate voxel-based percentage enhancement (PE) and signal enhancement ratio (SER) maps of each breast. This study collected two independent radiogenomics cohorts (n = 246) to identify and validate imaging subtypes. The results demonstrated that these imaging subtypes, with distinct clinical and molecular characteristics, were reliable, reproducible, and valuable for non-invasive prediction of the outcome and biological functions of breast cancer.
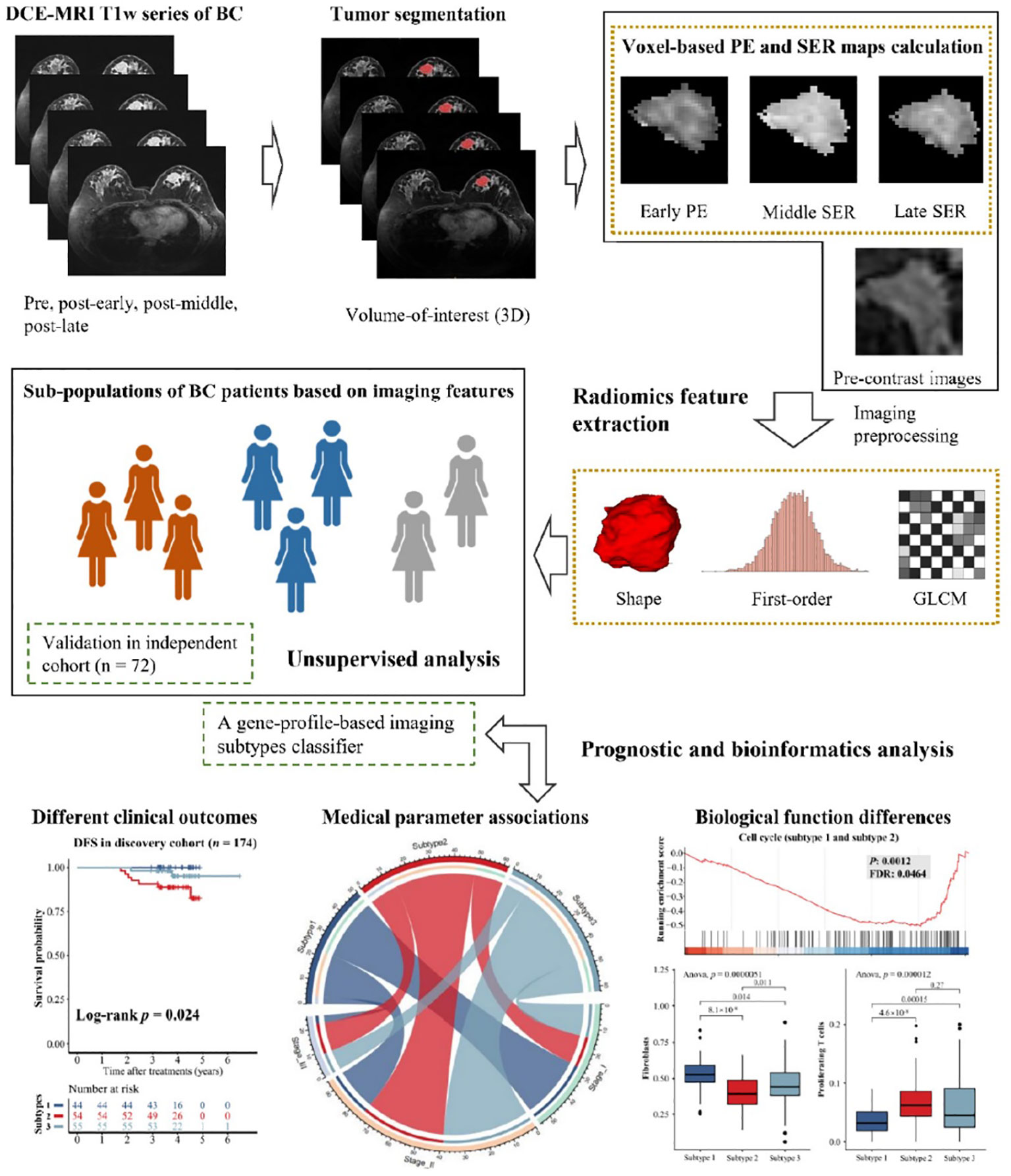
Figure 1 Workflow of unsupervised analysis based on DCE-MRI features in breast cancer patients (35).
2.5 Positron emission tomography
PET is an advanced imaging technique that has made significant contributions to the diagnosis and treatment of breast cancer. It is a non-invasive procedure that provides healthcare professionals with valuable information about the spread of cancer to other parts of the body, making it an essential tool in the fight against breast cancer. With ongoing technological advancements, PET plays a crucial role in the detection and treatment of breast cancer.
PET utilizes radiotracers to generate three-dimensional images of the interior of the body. It operates by detecting pairs of gamma rays emitted by the radiotracer as it decays within the body. PET imaging was first introduced in the early1950s, and the first PET scanner was developed in the 1970s. Since then, PET has become an indispensable tool for cancer detection. It has been commonly used to diagnose and stage cancer and assess the effectiveness of cancer treatments. It is also utilized in cardiology, neurology, breast, and psychiatry.
PET is more sensitive than mammography and ultrasound in detecting small breast tumors, and it can also distinguish between benign and malignant lesions with higher accuracy (36). The advantages of PET include its non-invasive and safety for repeated use. However, PET does have limitations, including limited availability, higher cost compared to mammography and ultrasound, a higher rate of false positives, and the requirement for radiotracer injection.
3 Deep learning-based mammography techniques
Several DL architectures, including convolutional neural networks (CNN), transfer learning (TL), ensemble learning (EL), and attention-based methods, have been developed for various applications in mammography. These applications include breast cancer detection, classification, segmentation, image restoration and enhancement, and computer-aided diagnosis (CAD) systems.
CNN is an artificial neural network with impressive results in image recognition tasks. CNN recognizes image patterns using convolutional layers that apply filters to the input image. The filters extract features from the input image, passing through fully connected layers to classify the image. Several CNN-based methods have been proposed in mammography for breast tumor detection. Wang et al. (37) applied CNN with transfer learning in ultrasound for breast cancer classification. The proposed method achieved an area under the curve (AUC) value of 0.9468 with five-folder cross-validation, for which the sensitivity and specificity were 0.886 and 0.876, respectively. Shen et al. (38) proposed a deep CNN in Mammography to classify benign and malignant and achieved an accuracy of 0.88, higher than radiologists (0.83). The study showed that CNN had a lower false-positive rate than radiologists. Yala et al. (39) developed CNN-based mammography to classify mammograms as low or high risk for breast cancer and achieved an AUC of 0.84, which was higher than that of radiologists (0.77). CNN had a lower false-positive rate than radiologists, which has shown promising results in improving the accuracy of mammography screening. CNN has several advantages over traditional mammography screening, including higher accuracy, faster processing, and the ability to identify subtle changes in mammograms. CNN requires large amounts of data to train the network and may not be able to detect all types of breast cancer. Further research is needed to investigate the use of CNN in Mammography.
CNN is an artificial neural network that has shown impressive results in image recognition tasks. It recognizes image patterns using convolutional layers that apply filters to the input image. These filters extract features from the input image, which then pass through fully connected layers to classify the image. In Mammography, several CNN-based methods, such as DenseNet, ResNet, and VGGNet, have been proposed for breast tumor detection. For example, Wang et al. (37) applied CNN with transfer learning in ultrasound for breast cancer classification, achieving an area under the curve (AUC) value of 0.9468 with five-fold cross-validation. The sensitivity and specificity were 0.886 and 0.876, respectively. Shen et al. (38) proposed a deep CNN in mammography to classify between benign and malignant tumors, achieving an accuracy of 0.88, higher than that of radiologists (0.83). Yala et al. (39) developed a CNN-based mammography system to classify mammograms as low or high risk for breast cancer, achieving an AUC of 0.84, higher than that of radiologists (0.77). These studies demonstrated that CNN had a lower false-positive rate than radiologists, showing promise in improving the accuracy of mammography screening. CNN offers advantages over traditional mammography screening, including higher accuracy, faster processing, and the ability to identify subtle changes in mammograms. However, CNN requires large amounts of data to train the network and may not be able to detect all types of breast cancer. Further research is needed to investigate the use of CNN in mammography.
TL utilizes pre-trained DL models to train on small datasets. TL-based methods have shown promising results in improving the accuracy of mammography for breast tumor detection. EL combines multiple DL models to improve the accuracy of predictions. EL-based approaches, such as stacking, boosting, and bagging, have been proposed in mammography for breast tumor detection.
Attention-based methods use attention mechanisms to focus on critical features of the image. Several attention-based methods, such as SE-Net and Channel Attention Networks (CAN), have been proposed for breast tumor detection in mammography. DL is a type of ML that uses neural networks to learn and make predictions. DL methods have gained popularity in recent years due to their ability to work with large datasets and extract meaningful patterns and insights.
DL methods have revolutionized the field of machine learning and are being used in an increasing number of applications, ranging from self-driving cars to medical imaging. As datasets and computing power continue to grow, these methods are expected to become even more powerful and prevalent in the future.
4 Breast cancer prediction using deep learning
This section presents the recent developments in DL methods for breast cancer prediction. The DL-based breast cancer prediction techniques involves the following steps:
● Data Collection: Breast datasets are obtained from various sources such as medical institutions, public repositories, and research studies. These datasets consist of mammogram images, gene expression profiles, and clinical data.
● Data Preprocessing: The collected datasets are preprocessed to eliminate noise, normalize, and standardize the data. This step involves data cleaning, feature extraction, and data augmentation.
● Model Building: DL models, such as CNNs, RNNs, DBNs, and autoencoders, are developed using the preprocessed breast cancer datasets. These models are trained and optimized using training and validation datasets.
● Model Evaluation: The trained DL models are assessed using a separate test dataset to determine their performance. Performance metrics, including sensitivity, specificity, accuracy, precision, F1 score, and AUC, are used for evaluation.
● Model Interpretation: The interpretability of the DL models is evaluated using techniques such as Grad-CAM, saliency maps, and feature visualization. These techniques help identify which features of the input data are utilized by the DL models for making predictions.
● Deployment: The DL model is deployed in a clinical setting to predict breast cancer in patients. The performance of the model is regularly monitored and updated to enhance accuracy and efficiency.
By utilizing DL techniques, breast cancer prediction can be significantly improved, leading to better detection and treatment outcomes.
4.1 Data preprocessing techniques and evaluation
4.1.1 Preprocessing techniques
When applying DL algorithms to analyze breast images, noise can have a negative impact on the accuracy of the image classifier. To address this issue, several image denoising techniques have been developed. These techniques, including the Median filter, Wiener filter, Non-local means filter, Total variation (TV) denoising, Wavelet-based denoising, Gaussian filter, anisotropic diffusion, BM3D denoising, CNN, and autoencoder, aim to reduce image noise while preserving important features and structures that are relevant for breast cancer diagnosis.
After denoising, a normalization method, such as min-max normalization, is typically employed to rescale the images and reduce the complexity of the image datasets before feeding them into the DL model. This normalization process ensures that the model can effectively learn meaningful patterns from the images and improve its ability to accurately classify them.
4.1.2 Performance metrics
Several performance metrics are utilized to evaluate DL algorithms for breast screening. The selection of a specific metric depends on the task at hand and the objectives of the model. Some of the most commonly employed metrics include:
● Accuracy: measures the proportion of correct predictions made by the model.
● Precision: measures the proportion of true positive predictions out of all positive predictions made by the model.
● Sensitivity: measures the proportion of true positive predictions out of all actual positive cases in the dataset.
● F1 score: a composite metric that balances precision and sensitivity.
● Area under the curve (AUC): distinguishes between positive and negative points across a range of threshold values.
● Mean Squared Error (MSE): measures the average squared difference between predicted and actual values in a regression task.
● Mean Absolute Error (MAE): measures the average absolute difference between the predicted and actual values in a regression task.
The commonly used equation for calculating accuracy, as stated in reference (40), is:
Where TP and TN are the numbers of true positives and true negatives, FP and FN are the numbers of false positives and false negatives, respectively.
AUC is typically computed by plotting the true positive rate against the false positive rate at different threshold values and then calculating the area under this curve.
Where ytru is the true value and ypred is the predicted value, and n is the number of samples.
Equations 1–6 provide a general idea of how performance metrics are computed, but the actual implementation may vary depending on the specific task and the software.
4.2 Datasets
Breast datasets play a crucial role in evaluating DL approaches. These datasets offer a comprehensive collection of high-quality and labelled breast images that can be utilized for training and testing DL algorithms. Table 2 presents commonly utilized publicly available breast datasets in mammography for breast screening.
4.3 Breast lesion segmentation
The Nottingham Histological Grading (NHG) system is currently the most commonly utilized tool for assessing the aggressiveness of breast cancer (50). According to this system, breast cancer scores are determined based on three significant factors: tubule formation (51), nuclear pleomorphism (52), and mitotic count (53). Tubule formation is an essential assessment factor in the NHG grading system for understanding the level of cancer. Before identifying tubule formation, detection or segmentation tasks need to be performed. Pathologists typically conduct these tasks visually by examining whole slide images (WSIs). Medical image segmentation assists pathologists in focusing on specific regions of interest in WSIs and extracting detailed information for diagnosis. Conventional and AI methods have been applied in medical image segmentation, utilizing handcrafted features such as color, shapes, and texture (54–56). Traditional manual tubule detection and segmentation techniques have been employed in medical images. However, these methods are challenging, prone to errors, exhaustive, and time-consuming (57, 58).
Table 3 provides a comparison of recently developed DL methods in mammography for breast lesion segmentation. These methods include the Conditional Random Field model (CRF) (59), Adversarial Deep Structured Net (60), Deep Learning using You-Only-Look-Once (61), Conditional Residual U-Net (CRU-Net) (62), Mixed-Supervision-Guided (MS-ResCU-Net) and Residual-Aided Classification U-Net Model (ResCU-Net) (63), Dense U-Net with Attention Gates (AGs) (64), Residual Attention U-Net Model (RU-Net) (65), Modified U-Net (66), Mask RCNN (67), Full-Resolution Convolutional Network (FrCN) (68), U-Net (69), Conditional Generative Adversarial Networks (cGAN) (70, 71), DeepLab (72), Attention-Guided Dense-Upsampling Network (AUNet) (73), FPN (74), modified CNN based on U-Net Model (76), deeply supervised U-Net (77), modified U-Net (78), and Tubule-U-Net (79). Among these DL methods, U-Net is the most commonly employed segmentation method.
Naik et al. (80) developed a likelihood method for the segmentation of lumen, cytoplasm, and nuclei based on a constraint: a lumen area must be surrounded by cytoplasm and a ring of nuclei to form a tubule. Tutac et al. (81) introduced a knowledge-guided semantic indexing technique and symbolic rules for the segmentation of tubules based on lumen and nuclei. Basavanhally et al. (82) developed the O’Callaghan neighborhood method for tubule detection, allowing for the characterization of tubules with multiple attributes. The process was tested on 1226 potential lumen areas from 14 patients and achieved an accuracy of 89% for tubule detection. In reference (83), the authors applied a k-means clustering algorithm to cluster pixels of nuclei and lumens. They employed a level-set method to segment the boundaries of the nuclei surrounding the lumen, achieving an accuracy of 90% for tubule detection. Romo-Bucheli et al. (84) developed a Convolutional Neural Network (CNN) based detection and classification method to improve the accuracy of nuclei detection in tubules, achieving an accuracy of 90% for tubule nuclei detection. Hu et al. (85) proposed a breast mass segmentation technique using a full CNN (FCNN), which showed promising results with high accuracy and speed. Abdelhafiz et al. (86) studied the application of deep CNN for mass segmentation in mammograms and found increased performance in terms of accuracy. Tan et al. (87) recently developed a tubule segmentation method that investigates geometrical patterns and regularity measurements in tubule and non-tubule regions. This method is based on handcrafted features and conventional segmentation techniques, which are not effective and efficient for tubule structures due to their complex, irregular shapes and orientations with weak boundaries.
4.4 Deep learning approaches in mammography for breast lesion detection and classification
DL approaches have garnered considerable attention in mammography for the detection and classification of breast lesions, primarily due to their ability to automatically extract high-level features from medical images. Numerous popular DL algorithms have been employed in mammography for breast screening, including convolutional neural networks (CNN), deep belief networks (DBN), recurrent neural networks (RNN), autoencoders, generative adversarial networks (GAN), capsule networks (CN), convolutional recurrent neural networks (CRNN), attention mechanisms, multiscale CNN, and ensemble learning (EL).
CNN proves highly effective in extracting and classifying image features into distinct categories. DBN is particularly advantageous in identifying subtle changes in images that may be challenging for human observers to discern. RNN utilizes feedback loops to facilitate predictions, thereby aiding in the analysis of sequential data. Autoencoders are utilized for unsupervised feature learning, which aids in the detection and classification of mammography images. GAN is exceptionally effective in generating synthetic mammography images for training DL models. CN is highly proficient in detecting and classifying mammography images. CRNN combines CNN and RNN, making it particularly useful in analyzing sequential data. Attention mechanisms focus on specific areas of mammography images, proving beneficial in detecting and classifying images that encompass intricate structures and patterns. Multiscale CNN analyzes images at multiple scales, proving invaluable in detecting and classifying images with complex structures and patterns at varying scales. EL combines multiple DL models to enhance accuracy and reduce false positives.
Table 4 analyzes the recently developed DL methods for breast lesion detection using mammography. These methods have the potential to greatly enhance the accuracy and efficiency of breast cancer diagnosis. However, it is important to note that most DL methods for biomedical imaging applications come with certain limitations. These limitations include the need for large training datasets, being limited to mass spectrometry images, and being computationally expensive.
Table 5 presents a comprehensive list of the latest DL-based mammogram models developed for breast lesion classification. DL models offer numerous benefits, including exceptional accuracy and optimal performance achieved with fewer parameters. However, it is important to acknowledge certain limitations associated with existing DL methods for breast tumor classification using mammographies. These limitations include the substantial computational power and extensive datasets required for training the models, which can be computationally expensive, intricate, and time-consuming.
5 Challenges and future research directions
The emergence of DL techniques has revolutionized medical imaging, offering immense potential to enhance the diagnosis and treatment of various diseases. DL algorithms present several advantages compared to traditional ML methods. For instance, DL algorithms can be trained using robust hardware such as graphical processing units (GPU) and tensor processing units (TPU), greatly accelerating the training process. This has enabled researchers to train large DL models with billions of parameters, yielding impressive results in diverse language tasks. However, to fully leverage the potential of DL in medical imaging, several challenges must be addressed. One of the primary challenges is the scarcity of data. DL algorithms require abundant, high-quality data for effective training. Yet, acquiring medical imaging data is often challenging, particularly for rare diseases or cases requiring long-term follow-up. Furthermore, data privacy regulations and concerns can further complicate the availability of medical imaging data. Another challenge lies in the quality of annotations. DL algorithms typically demand substantial amounts of annotated data for effective training. However, annotating medical imaging data can be subjective and time-consuming, leading to issues with annotation quality and consistency. This can significantly impact the performance of deep learning algorithms, particularly when accurate annotations are vital for diagnosing or treating specific conditions. Additionally, imbalanced classes pose another challenge in medical imaging.
In numerous instances, the occurrence of certain states may be relatively low, which can result in imbalanced datasets that have a detrimental effect on the performance of DL algorithms. This situation can pose a significant challenge, especially for rare diseases or conditions with limited data availability. Another crucial concern in medical imaging is the interpretability of models. Although DL algorithms have showcased remarkable performance across various medical imaging tasks, the lack of interpretability in these models can hinder their adoption. Clinicians frequently necessitate explanations for the predictions made by these algorithms in order to make informed decisions, but the opacity of DL models can make this task arduous.
Data privacy is a paramount concern in medical imaging. Medical images encompass confidential patient information, stringent regulations dictate the utilization and dissemination of such data. The effective training of DL necessitates substantial access to extensive medical imaging data, thereby introducing challenges concerning data privacy and security. Additionally, computational resources pose another challenge in the realm of medical imaging. DL algorithms mandate substantial computational resources for the effective training and of models. This predicament can prove particularly troublesome in medical imaging, given the size and intricacy of medical images, which can strain computing resources. DL algorithms can be vulnerable to adversarial attacks, where small perturbations to input data can cause significant changes in the model’s output. This can be particularly problematic for medical imaging, where even small changes to an image can have substantial implications for diagnosis and treatment.
Several potential strategies can be employed to address these challenges effectively. One approach involves the development of transfer learning techniques, enabling DL models to be trained on smaller datasets by leveraging information from related tasks or domains. This approach holds particular promise in medical imaging, where data scarcity poses a significant obstacle. Another approach involves placing emphasis on the development of annotation tools and frameworks that enhance the quality and consistency of annotations. This becomes important in cases where annotations play a critical role in diagnosing or treating specific conditions. Furthermore, improved data sharing and collaboration between institutions can help alleviate both data scarcity and privacy concerns. By pooling resources and sharing data, it becomes feasible to construct more extensive and diverse datasets that can be employed to train DL models with greater effectiveness. Additionally, enhancing the interpretability of DL models in medical imaging techniques stands as another critical area of research. The development of explainable AI techniques can provide clinicians with valuable insights into the underlying factors contributing to a model’s predictions. Lastly, bolstering the robustness of DL models constitutes a crucial focal point. This entails exploring adversarial training techniques, as well as leveraging ensemble methods and other strategies to enhance the overall robustness and generalizability of DL models.
DL techniques have the potential to revolutionize medical imaging. However, to fully leverage this potential, it is crucial to address several challenges. These challenges encompass data scarcity, annotation quality, imbalanced classes, model interpretability, data privacy, computational resources, and algorithm robustness. By prioritizing strategies to tackle these challenges, it becomes possible to develop DL models that are more effective and reliable for various medical imaging applications.
6 Conclusion
This paper examines the recent advancements in DL-based mammography for breast cancer screening. The authors have investigated the potential of DL techniques in enhancing the accuracy and efficiency of mammography. Additionally, they address the challenges that need to be overcome for the successful adoption of DL techniques in clinical practice.
Author contributions
LW: Conceptualization, Data curation, Formal analysis, Funding acquisition, Investigation, Methodology, Project administration, Resources, Software, Visualization, Writing – original draft, Writing – review & editing.
Funding
The author(s) declare financial support was received for the research, authorship, and/or publication of this article. This research was funded by the International Science and Technology Cooperation Project of the Shenzhen Science and Technology Innovation Committee (GJHZ20200731095804014).
Conflict of interest
The author declares that the research was conducted in the absence of any commercial or financial relationships that could be construed as a potential conflict of interest.
Publisher’s note
All claims expressed in this article are solely those of the authors and do not necessarily represent those of their affiliated organizations, or those of the publisher, the editors and the reviewers. Any product that may be evaluated in this article, or claim that may be made by its manufacturer, is not guaranteed or endorsed by the publisher.
References
1. Chon JW, Jo YY, Lee KG, Lee HS, Kweon HY. Effect of silk fibroin hydrolysate on the apoptosis of mcf-7 human breast cancer cells. Int J Ind Entomol (2013) 27(2):228–36. doi: 10.7852/ijie.2013.27.2.228
2. Habtegiorgis SD, Getahun DS, Telayneh AT, Birhanu MY, Feleke TM, Mingude AB, et al. Ethiopian women's breast cancer self-examination practices and associated factors a systematic review and meta-analysis. Cancer Epidemiol (2022) 78:102128. doi: 10.1016/j.canep.2022.102128
3. Ginsburg O, Yip CH, Brooks A, Cabanes A, Caleffi M, Dunstan Yataco JA, et al. Breast cancer early detection: A phased approach to implementation. Cancer (2020) 126:2379–93. doi: 10.1002/cncr.32887
4. Jalalian A, Mashohor SB, Mahmud HR, Saripan MIB, Ramli ARB, Karasfi B. Computer-aided detection/diagnosis of breast cancer in mammography and ultrasound: a review. Clin Imaging (2013) 37(3):420–6. doi: 10.1016/j.clinimag.2012.09.024
5. Gilbert FJ, Pinker-Domenig K. (2019). Diagnosis and staging of breast cancer: when and how to use mammography, tomosynthesis, ultrasound, contrast-enhanced mammography, and magnetic resonance imaging. In: Diseases of the chest, breast, heart and vessels 2019-2022. Springer, Cham (2019). pp. 155–66. doi: 10.1007/978-3-030-11149-6_13
6. Alghaib HA, Scott M, Adhami RR. An overview of mammogram analysis. IEEE Potentials (2016) 35(6):21–8. doi: 10.1109/MPOT.2015.2396533
7. Monticciolo DL, Newell MS, Moy L, Niell B, Monsees B, Sickles EA. Breast cancer screening in women at higher-than-average risk: recommendations from the ACR. J Am Coll Radiol (2018) 15(3):408–14. doi: 10.1016/j.jacr.2017.11.034
8. Alabousi M, Zha N, Salameh JP, Samoilov L, Sharifabadi AD, Pozdnyakov A, et al. Digital breast tomosynthesis for breast cancer detection: a diagnostic test accuracy systematic review and meta-analysis. Eur Radiol (2020) 30:2058–71. doi: 10.1007/s00330-019-06549-2
9. Brem RF, Lenihan MJ, Lieberman J, Torrente J. Screening breast ultrasound: past, present, and future. Am J Roentgenol (2015) 204(2):234–40. doi: 10.2214/AJR.13.12072
10. Heller SL, Moy L. MRI breast screening revisited. J Magnetic Resonance Imaging (2019) 49(5):1212–21. doi: 10.1002/jmri.26547
11. Schöder H, Gönen M. Screening for cancer with PET and PET/CT: potential and limitations. J Nucl Med (2007) 48(1):4S–18S. doi: 10.1016/S0148-2963(03)00075-4
12. Narayanan D, Berg WA. Dedicated breast gamma camera imaging and breast PET: current status and future directions. PET Clinics (2018) 13(3):363–81. doi: 10.1016/j.cpet.2018.02.008
13. He J, Baxter SL, Xu J, Xu J, Zhou X, Zhang K. The practical implementation of artificial intelligence technologies in medicine. Nat Med (2019) 25:30–6. doi: 10.1038/s41591-018-0307-0
14. Kim KH, Lee SH. Applications of artificial intelligence in mammography from a development and validation perspective. J Korean Soc Radiol (2021) 82(1):12. doi: 10.3348/jksr.2020.0205
15. Hamed G, Marey MAER, Amin SES, Tolba MF. (2020). Deep learning in breast cancer detection and classification. In: Proceedings of the international conference on artificial intelligence and computer vision, advances in intelligent systems and computing. Springer, Cham (2020) 1153:322–33. doi: 10.1007/978-3-030-44289-7_30
16. Wang J, Gottschal P, Ding L, Veldhuizen DAV, Lu W, Houssami N, et al. Mammographic sensitivity as a function of tumor size: a novel estimation based on population-based screening data. Breast (2021) 55:69–74. doi: 10.1016/j.breast.2020.12.003
17. Stein RG, Wollschläger D, Kreienberg R, Janni W, Wischnewsky M, Diessner J, et al. The impact of breast cancer biological subtyping on tumor size assessment by ultrasound and mammography-a retrospective multicenter cohort study of 6543 primary breast cancer patients. BMC Cancer (2016) 16(1):1–8. doi: 10.1186/s12885-016-2426-7
18. Chen HL, Zhou JQ, Chen Q, Deng YC. Comparison of the sensitivity of mammography, ultrasound, magnetic resonance imaging and combinations of these imaging modalities for the detection of small (≤2 cm) breast cancer. Medicine (2021) 100(26):e26531. doi: 10.1097/MD.0000000000026531
19. Gunther JE, Lim EA, Kim HK, Flexman M, Altoé M, Campbell JA, et al. Dynamic diffuse optical tomography for monitoring neoadjuvant chemotherapy in patients with breast cancer. Radiology (2018) 287(3):778–86. doi: 10.1148/radiol.2018161041
20. Movik E, Dalsbø TK, Fagerlund BC, Friberg EG, Håheim LL, Skår Å. (2017). Digital breast tomosynthesis with hologic 3d mammography selenia dimensions system for use in breast cancer screening: a single technology assessment. Oslo, Norway: Knowledge Centre for the Health Services at The Norwegian Institute of Public Health.
21. Marion W. Siemens Healthineers, GE HealthCare Race To Develop Next-Gen AI Solutions For Personalized Care (2023). Available at: https://medtech.pharmaintelligence.informa.com/MT147893/Siemens-Healthineers-GE-HealthCare-Race-To-Develop-NextGen-AI-Solutions-For-Personalized-Care.
22. NEWS BREAST IMAGING, ScreenPoint Medical: Transpara Breast AI Demonstrates Value in Real-world Clinical Usage (2023). Available at: https://www.itnonline.com/content/screenpoint-medical-transpara-breast-ai-demonstrates-value-real-world-clinical-usage.
23. Gong Z, Williams MB. Comparison of breast specific gamma imaging and molecular breast tomosynthesis in breast cancer detection: evaluation in phantoms. Med Phys (2015) 42(7):4250. doi: 10.1118/1.4922398
24. Łuczyńska E, Heinze-Paluchowska S, Hendrick E, Dyczek S, Ryś J, Herman K, et al. Comparison between breast MRI and contrast-enhanced spectral mammography. Med Sci Monit (2015) 21:1358–67. doi: 10.12659/MSM.893018
25. Cohen EO, Perry RE, Tso HH, Phalak KA, Leung JWT. Breast cancer screening in women with and without implants: retrospective study comparing digital mammography to digital mammography combined with digital breast tomosynthesis. Eur Radiol (2021) 31(12):9499–510. doi: 10.1007/s00330-021-08040-3
26. Gilbert FJ, Tucker L, Young KC. Digital breast tomosynthesis (DBT): a review of the evidence for use as a screening tool. Clin Radiol (2016) 71(2):141–50. doi: 10.1016/j.crad.2015.11.008
27. Nakano S, Fujii K, Yorozuya K, Yoshida M, Fukutomi T, Arai O, et al. P2-10-10: a precision comparison of breast ultrasound images between different time phases by imaging fusion technique using magnetic position tracking system. Cancer Res (2011) 71(24):P2–10-10-P2-10-10. doi: 10.1158/0008-5472.SABCS11-P2-10-10
28. Zhang E, Seiler S, Chen M, Lu W, Gu X. Birads features-oriented semi-supervised deep learning for breast ultrasound computer-aided diagnosis. Phys Med Biol (2020) 65(12):125005. doi: 10.1088/1361-6560/ab7e7d
29. Santos MK, Ferreira Júnior JR, Wada DT, Tenório APM, Barbosa MHN, Marques PMA. Artificial intelligence, machine learning, computer-aided diagnosis, and radiomics: advances in imaging towards to precision medicine. Radiol Bras (2019) 52(6):387–96. doi: 10.1590/0100-3984.2019.0049
30. GE Healthcare. Invenia ABUS 2.0. Available at: https://www.gehealthcare.com/en-ph/products/ultrasound/abus-breast-imaging/invenia-abus.
31. Siemens Healthineers. ACUSON S2000 ABVS System HELX Evolution with Touch Control. Available at: https://shop.medicalimaging.healthcare.siemens.com.sg/acuson-s2000-abvs-system-helx-evolution-with-touch-control/.
32. Kuhl CK, Schrading S, Strobel K, Schild HH, Hilgers RD, Bieling HB. Abbreviated breast magnetic resonance imaging (MRI): first postcontrast subtracted images and maximum-intensity projection-a novel approach to breast cancer screening with MRI. J Clin Oncol (2014) 32(22):2304–10. doi: 10.1200/JCO.2013.52.5386
33. Mann RM, Mus RD, van Zelst J, Geppert C, Karssemeijer NA. Novel approach to contrast-enhanced breast magnetic resonance imaging for screening: high-resolution ultrafast dynamic imaging. Invest Radiol (2014) 49(9):579–85. doi: 10.1097/RLI.0000000000000057
34. Zhang Y, Chen JH, Chang KT, Park VY, Kim MJ, Chan S, et al. Automatic breast and fibroglandular tissue segmentation in breast MRI using deep learning by a fully-convolutional residual neural network U-net. Acad Radiol (2019) 26(11):1526–35. doi: 10.1016/j.acra.2019.01.012
35. Ming W, Li F, Zhu Y, Bai Y, Gu W, Liu Y, et al. Unsupervised analysis based on DCE-MRI radiomics features revealed three novel breast cancer subtypes with distinct clinical outcomes and biological characteristics. Cancers (2022) 14(22):5507. doi: 10.3390/cancers14225507
36. Marcus C, Jeyarajasingham K, Hirsch A, Subramaniam RM. PET/CT in the management of thyroid cancers. Am Roentgen Ray Soc Annu Meeting (2014) 202:1316–29. doi: 10.2214/AJR.13.11673
37. Wang Y, Choi EJ, Choi Y, Zhang H, Jin GY, Ko SB. Breast cancer classification in automated breast ultrasound using multiview convolutional neural network with transfer learning - ScienceDirect. Ultrasound Med Biol (2020) 46(5):1119–32. doi: 10.1016/j.ultrasmedbio.2020.01.001
38. Shen L, Margolies LR, Rothstein JH, Fluder E, McBride R, Sieh W. Deep learning to improve breast cancer detection on screening mammography. Sci Rep (2019) 9:12495. doi: 10.1038/s41598-019-48995-4
39. Yala A, Lehman C, Schuster T, Portnoi T, Barzilay R. A deep learning mammography-based model for improved breast cancer risk prediction. Radiology (2019) 292(1):182716. doi: 10.1148/radiol.2019182716
40. Wang L. Deep learning techniques to diagnose lung cancer. Cancers (2022) 14:5569. doi: 10.3390/cancers14225569
41. Heath M, Bowyer K, Kopans D, Kegelmeyer P Jr, Moore R, Chang K. Current Status of the digital database for screening mammography. In: Digital mammography. computational imaging and vision. (Springer, Dordrecht) (1998) 11:457–60. doi: 10.1007/978-94-011-5318-8_75
42. Li B, Ge Y, Zhao Y, Guan E, Yan W. Benign and malignant mammographic image classification based on convolutional neural networks. In: 10th international conference on machine learning and computing. (Beijing, China: Association for Computing Machinery) (2018). pp. 11–15. doi: 10.1145/3195106.3195163
43. Inês CM, Igor A, Inês D, António C, Maria JC, Jaime SC. Inbreast: toward a full-field digital mammographic database. Acad Radiol (2012) 19:236–48. doi: 10.1016/j.acra.2011.09.014
44. Araújo T, Aresta G, Castro E, Rouco J, Aguiar P, Eloy C, et al. Classification of breast cancer histology images using convolutional neural networks. PloS One (2017) 12(6):e0177544. doi: 10.1371/journal.pone.0177544
45. Lee R, Gimenez F, Hoogi A, Miyake K, Gorovoy M, Rubin D. A curated mammography data set for use in computer-aided detection and diagnosis research. Sci Data (2017) 4:170177. doi: 10.1038/sdata.2017.177
46. Ramos-Pollán R, Guevara-López M, Suárez-Ortega C, Díaz-Herrero G, Franco-Valiente J, Rubio-del-Solar M, et al. Discovering mammography-based machine learning classifiers for breast cancer diagnosis. J Med Syst (2011) 1:11. doi: 10.1007/s10916-011-9693-
47. Heath M, Bowyer K, Kopans D, Moore R, Kegelmeyer WP. (2001). Current status of the digital database for screening mammography. In: Digital mammography. computational imaging and vision. Springer Netherlands (1998) 13:457–60. doi: 10.1007/978-94-011-5318-8_75
48. Yoon WB, Oh JE, Chae EY, Kim HH, Lee SY, Kim KG. Automatic detection of pectoral muscle region for computer-aided diagnosis using MIAS mammograms. BioMed Res Int (2016) 2016:5967580. doi: 10.1155/2016/5967580
49. Lopez MG, Posada N, Moura DC, Pollán RR, Valiente JMF, Ortega CS, et al. (2012). BCDR: a breast cancer digital repository. In: International conference on experimental mechanics. Porto, Portugal (2012). pp. 113–20.
50. Dalle J-R, Leow WK, Racoceanu D, Tutac AE, Putti TC. (2008). Automatic breast cancer grading of histopathological images. In: Annu Int Conf IEEE Eng Med Biol Soc. Vancouver, BC, Canada: IEEE (2008). pp. 3052–5. doi: 10.1109/IEMBS.2008.4649847
51. Lee S, Fu C, Salama P, Dunn K, Delp E. Tubule segmentation of fluorescence microscopy images based on convolutional neural networks with inhomogeneity correction. Int Symp Electr Imaging (2018) 30:199–1–199–8. doi: 10.2352/ISSN.2470-1173.2018.15.COIMG-199
52. Kumar N, Verma R, Sharma S, Bhargava S, Vahadane A, Sethi AA. Dataset and a technique for generalized nuclear segmentation for computational pathology. IEEE Trans Med Imaging (2017) 36(7):1550–60. doi: 10.1109/TMI.2017.2677499
53. Saha M, Chakraborty C, Racoceanu D. Efficient deep learning model for mitosis detection using breast histopathology images. Comput Med Imaging Graph (2018) 64:29–40. doi: 10.1016/j.compmedimag.2017.12.001
54. Ronneberger O, Fischer P. Brox, T. U-net: Convolutional networks for biomedical image segmentation. In: Medical image computing and computer-assisted intervention. Springer Switzerland (2015). p. 234–41. doi: 10.1007/978-3-319-24574-4_28
55. Borgli H, Thambawita V, Pia Helén S, Hicks SA, Lange TD. A comprehensive multi-class image and video dataset for gastrointestinal endoscopy. Sci Data (2020) 7:1–14. doi: 10.1038/s41597-020-00622-y
56. Mamonov AV, Figueiredo IN, Figueiredo PN, Tsai YH. Automated polyp detection in colon capsule endoscopy. IEEE Trans Med Imaging (2013) 33:1488–502. doi: 10.1109/TMI.2014.2314959
57. Bellens S, Probst GM, Janssens M, Vandewalle P, Dewulf W. Evaluating conventional and deep learning segmentation for fast X-ray CT porosity measurements of polymer laser sintered am parts. Polym Test (2022) 110:107540. doi: 10.1016/j.polymertesting.2022.107540
58. Yuan X, Yuxin W, Jie Y, Qian C, Xueding W, Carson PL. Medical breast ultrasound image segmentation by machine learning. Ultrasonics (2018) 91:1–9. doi: 10.1016/j.ultras.2018.07.006
59. Dhungel N, Carneiro G, Bradley AP. Tree RE-weighted belief propagation using deep learning potentials for mass segmentation from mammograms. In: 2015 IEEE 12th international symposium on biomedical imaging Brooklyn, NY, USA: IEEE (2015). pp. 760–3. doi: 10.1109/ISBI.2015.7163983
60. Zhu W, Xiang X, Tran TD, Hager GD, Xie X. Adversarial deep structured nets for mass segmentation from mammograms. In: International symposium on biomedical imaging. Washington, DC, USA: IEEE (2018). 847–50. doi: 10.1109/ISBI.2018.8363704
61. Al-antari MA, Al-masni MA, Choi MT, Han SM, Kim TS. A fully integrated computer-aided diagnosis system for digital x-ray mammograms via deep learning detection, segmentation, and classification. Int J Med Inform (2018) 117:44–54. doi: 10.1016/j.ijmedinf.2018.06.003
62. Li H, Chen D, Nailon WH, Davies ME, Laurenson D. Image analysis for moving organ, breast, and thoracic images. In: Improved breast mass segmentation in mammograms with conditional residual u-net. Cham: Springer (2018). p. 81–9.
63. Shen T, Gou C, Wang J, Wang FY. Simultaneous segmentation and classification of mass region from mammograms using a mixed-supervision guided deep model. IEEE Signal Process Lett (2019) 27:196–200. doi: 10.1109/LSP.2019.2963151
64. Li GDS, Dong M, Xiaomin M. Attention dense-u-net for automatic breast mass segmentation in digital mammogram. IEEE Access (2019) 7:59037–47. doi: 10.1109/ACCESS.2019.2914873
65. Abdelhafiz D, Nabavi S, Ammar R, Yang C, Bi J. Residual deep learning system for mass segmentation and classification in mammography. In: Proceedings of the 10th ACM international conference on bioinformatics. Association for Computing Machinery, New York, NY, USA: Computational Biology and Health Informatics (2019). pp. 475–84. doi: 10.1145/3307339.3342157
66. Hossain MS. Microcalcification segmentation using modified u-net segmentation network from mammogram images. J King Saud University Comput Inf Sci (2022) 34(2):86–94. doi: 10.1016/j.jksuci.2019.10.014
67. Min H, Wilson D, Huang Y, Liu S, Crozier S, Bradley AP, et al. (2020). Fully automatic computer-aided mass detection and segmentation via pseudo-color mammograms and mask R-CNN. In: 17th international symposium on biomedical imaging (ISBI), Iowa City, IA, USA: IEEE (2000). pp. 1111–5. doi: 10.1109/ISBI45749.2020.9098732
68. Al-antari MA, Al-masni MA, Kim TS. Advances in experimental medicine and biology. In: Deep learning computer-aided diagnosis for breast lesion in digital mammogram. Cham: Springer (2020). p. 59–72.
69. Abdelhafiz D, Bi J, Ammar R, Yang C, Nabavi S. Convolutional neural network for automated mass segmentation in mammography. BMC Bioinf (2020) 21(S1):1–19. doi: 10.1186/s12859-020-3521-y
70. Saffari N, Rashwan HA, Abdel-Nasser M, Singh VK, Puig D. Fully automated breast density segmentation and classification using deep learning. Diagnostics (2020) 10(11):988. doi: 10.3390/diagnostics10110988
71. Kumar Singh V, Rashwan HA, Romani S, Akram F, Pandey N, Kamal Sarker MM, et al. Breast tumor segmentation and shape classification in mammograms using generative adversarial and convolutional neural network. Expert Syst Appl (2020) 139:112855. doi: 10.1016/j.eswa.2019.112855
72. Ahmed L, Iqbal MM, Aldabbas H, Khalid S, Saleem Y, Saeed S. Images data practices for semantic segmentation of breast cancer using deep neural network. J Ambient Intell Humanized Comput (2020) 14:15227–243. doi: 10.1007/s12652-020-01680-1
73. Sun H, Cheng L, Liu B, Zheng H, Feng DD, Wang S. AUNet: attention-guided dense-upsampling networks for breast mass segmentation in whole mammograms. Phys Med Biol (2020) 65(5):55005. doi: 10.1088/1361-6560/ab5745
74. Bhatti HMA, Li J, Siddeeq S, Rehman A, Manzoor A. (2020). Multi-detection and segmentation of breast lesions based on mask RCNN-FPN. In: International conference on bioinformatics and biomedicine (BIBM). Seoul, Korea (South): IEEE (2000). pp. 2698–704. doi: 10.1109/BIBM49941.2020.9313170
75. Zeiser FA, da Costa CA, Zonta T, Marques NMC, Roehe AV, Moreno M, et al. Segmentation of masses on mammograms using data augmentation and deep learning. J Digital Imaging (2020) 33(4):858–68. doi: 10.1007/s10278-020-00330-4
76. Tsochatzidis L, Koutla P, Costaridou L, Pratikakis I. Integrating segmentation information into cnn for breast cancer diagnosis of mammographic masses. Comput Methods Programs Biomed (2021) 200:105913. doi: 10.1016/j.cmpb.2020.105913
77. Ravitha Rajalakshmi N, Vidhyapriya R, Elango N, Ramesh N. Deeply supervised u-net for mass segmentation in digital mammograms. Int J Imaging Syst Technol (2021) 31(1):59–71. doi: 10.1002/ima.22516
78. Salama WM, Aly MH. Deep learning in mammography images segmentation and classification: automated cnn approach. Alexandria Eng J (2021) 60(5):4701–9. doi: 10.1016/j.aej.2021.03.048
79. Tekin E, Yazıcı Ç, Kusetogullari H, Tokat F, Yavariabdi A, Iheme LO, et al. Tubule-U-Net: a novel dataset and deep learning-based tubule segmentation framework in whole slide images of breast cancer. Sci Rep (2023) 13:128. doi: 10.1038/s41598-022-27331-3
80. Naik S, Doyle S, Agner S, Madabhushi A, Tomaszewski J. (2008). Automated gland and nuclei segmentation for grading of prostate and breast cancer histopathology. In: International Symposium on Biomedical Imaging: From Nano to Macro. Paris, France: IEEE (2008) pp. 284–7. doi: 10.1109/ISBI.2008.4540988
81. Tutac AE, Racoceanu D, Putti T, Xiong W, Cretu V. (2008). Knowledge-guided semantic indexing of breast cancer histopathology images. In: International conference on biomedical engineering and informatics. China: IEEE (2008). pp. 107–12. doi: 10.1109/BMEI.2008.166
82. Basavanhally A, Summers RM, Van Ginneken B, Yu E, Xu J, Ganesan S, et al. Incorporating domain knowledge for tubule detection in breast histopathology using o'callaghan neighborhoods. Proc SPIE Int Soc Optical Eng (2011) 7963:796310–796310-15. doi: 10.1117/12.878092
83. Maqlin P, Thamburaj R, Mammen JJ, Nagar AK. Automatic detection of tubules in breast histopathological images. In: Proceedings of Seventh International Conference on Bio-Inspired Computing: Theories and Applications. Springer, India: Adv Intelligent Syst Comput. (2013) 202:311–21. doi: 10.1007/978-81-322-1041-2_27
84. Romo-Bucheli D, Janowczyk A, Gilmore H, Romero E, Madabhushi A. Automated tubule nuclei quantification and correlation with oncotype dx risk categories in er+ breast cancer whole slide images. Sci Rep (2016) 6:32706. doi: 10.1038/srep32706
85. Hu Y, Guo Y, Wang Y, Yu J, Li J, Zhou S, et al. Automatic tumor segmentation in breast ultrasound images using a dilated fully convolutional network combined with an active contour model. Med Phys (2019) 46(1):215–28. doi: 10.1002/mp.13268
86. Abdelhafiz D, Yang C, Ammar R, Nabavi S. Deep convolutional neural networks for mammography: advances, challenges and applications. BMC Bioinf (2019) 20:1–20. doi: 10.1186/s12859-019-2823-4
87. Tan XJ, Mustafa N, Mashor MY, Rahman KSA. A novel quantitative measurement method for irregular tubules in breast carcinoma. Eng Sci Technol an Int J (2022) 31:101051. doi: 10.1016/j.jestch.2021.08.008
88. Jiao Z, Gao X, Wang Y, Li J. A deep feature based framework for breast masses classification. Neurocomputing (2016) 197:221–31. doi: 10.1016/j.neucom.2016.02.060
89. Huynh BQ, Li H, Giger ML. Digital mammographic tumor classification using transfer learning from deep convolutional neural networks. J Med Imaging (2016) 3(3):034501. doi: 10.1117/1.JMI.3.3.034501
90. Arevalo J, Gonzalez FA, Ramos-Pollan R, Oliveira JL, Guevara Lopez MA. Representation learning for mammography mass lesion classification with convolutional neural networks. Comput Methods Programs Biomed (2016) 127:248–57. doi: 10.1016/j.cmpb.2015.12.014
91. Leod PM, Verma B. (2016). Polynomial prediction of neurons in neural network classifier for breast cancer diagnosis. In: Proceedings of the international conference on natural computation (ICNC). Zhangjiajie, China: IEEE (2015). pp. 775–80. doi: 10.1109/ICNC.2015.7378089
92. Nascimento CDL, Silva SDS, da Silva TA, Pereira WCA, Costa MGF, Costa Filho CFF. Breast tumor classification in ultrasound images using support vector machines and neural networks. Rev Bras Engenharia Biomedica (2016) 32(3):283–92. doi: 10.1590/2446-4740.04915
93. Zhang Q, Xiao Y, Dai W, Suo JF, Wang CZ, Shi J, et al. Deep learning based classification of breast tumors with shear-wave elastography. Ultrasonics (2016) 72:150–7. doi: 10.1016/j.ultras.2016.08.004
94. Sun W, Tseng TL, Zhang J, Qian W. Enhancing deep convolutional neural network scheme for breast cancer diagnosis with unlabeled data. Comput Med Imaging Graphics (2017) 57:4–9. doi: 10.1016/j.compmedimag.2016.07.004
95. Dhungel N, Carneiro G, Bradley AP. A deep learning approach for the analysis of masses in mammograms with minimal user intervention. Med Image Anal (2017) 37:114–28. doi: 10.1016/j.media.2017.01.009
96. Samala RK, Chan HP, Hadjiiski LM, Helvie MA, Cha K, Richter C. Multi-task transfer learning deep convolutional neural network: application to computer aided diagnosis of breast cancer on mammograms. Phys Med Biol (2017) 62(23):8894–908. doi: 10.1088/1361-6560/aa93d4
97. Jadoon MM, Zhang Q, Ul Haq I, Butt S, Jadoon A. Three-class mammogram classification based on descriptive CNN features. BioMed Res Int (2017) 2017:3640901. doi: 10.1155/2017/3640901
98. Antropova N, Huynh BQ, Giger ML. A deep feature fusion methodology for breast cancer diagnosis demonstrated on three imaging modality datasets. Med Phys (2017) 44(10):5162–71. doi: 10.1002/mp.12453
99. Qiu Y, Yan S, Gundreddy RR, Wang Y, Zheng B. A new approach to develop computer-aided diagnosis scheme of breast mass classification using deep learning technology. J X-Ray Sci Technol (2017) 25(5):751–63. doi: 10.3233/XST-16226
100. Gardezi SJS, Awais M, Faye I, Meriaudeau F. (2017). Mammogram classification using deep learning features. In: Proceedings of the 2017 IEEE international conference on signal and image processing applications (ICSIPA). Kuching, Malaysia: IEEE (2017). pp. 485–8. doi: 10.1109/ICSIPA.2017.8120660
101. Kumar I, Bhadauria HS, Virmani J, Thakur S. A classification framework for prediction of breast density using an ensemble of neural network classifiers. Biocybernetics Biomed Eng (2017) 37(1):217–28. doi: 10.1016/j.bbe.2017.01.001
102. Zheng Y, Jiang Z, Xie F, Zhang H, Ma Y, Shi H, et al. Feature extraction from histopathological images based on nucleus-guided convolutional neural network for breast lesion classification. Pattern Recognit (2017) 71:14–25. doi: 10.1016/j.patcog.2017.05.010
103. Han S, Kang HK, Jeong JY, Park MH, Kim W, Bang WC, et al. A deep learning framework for supporting the classification of breast lesions in ultrasound images. Phys Med Biol (2017) 62(19):7714–28. doi: 10.1088/1361-6560/aa82ec
104. Yu S, Liu LL, Wang ZY, Dai GZ, Xie YQ. Transferring deep neural networks for the differentiation of mammographic breast lesions. Sci China Technol Sci (2018) 62(3):441–7. doi: 10.1007/s11431-017-9317-3
105. Ribli D, Horv'ath A, Unger Z, Pollner P, Csabai I. Detecting and classifying lesions in mammograms with deep learning. Sci Rep (2018) 8(1):85–94. doi: 10.1038/s41598-018-22437-z
106. A l-masni MA, Al-antari MA, Park JM, Gi G, Kim TY, Rivera P, et al. Simultaneous detection and classification of breast masses in digital mammograms via a deep learning YOLO-based CAD system. Comput Methods Programs Biomed (2018) 157:85–94. doi: 10.1016/j.cmpb.2018.01.017
107. Chougrad H, Zouaki H, Alheyane Q. Deep convolutional neural networks for breast cancer screening. Comput Methods Programs Biomed: An International Journal Devoted to the Development, Implementation and Exchange of Computing Methodology and Software Systems in Biomedical Research and Medical Practice (2018) 157:19–30. doi: 10.1016/j.cmpb.2018.01.011
108. Jiao Z, Gao X, Wang Y, Li J. A parasitic metric learning net for breast mass classification based on mammography. Pattern Recognit (20118) 75:292–301. doi: 10.1016/j.patcog.2017.07.008
109. Mohamed AA, Berg WA, Peng H, Luo Y, Jankowitz RC, Wu S. A deep learning method for classifying mammographic breast density categories. Med Phys (2018) 45(1):314–21. doi: 10.1002/mp.12683
110. Ribli D, Horváth A, Unger Z, Pollner P, Csabai I. Detecting and classifying lesions in mammograms with deep learning. Sci Rep (2018) 8(1):4165. doi: 10.1038/s41598-018-22437-z
111. Mendel K, Li H, Sheth D, Giger M. Transfer learning from convolutional neural networks for computer-aided diagnosis: a comparison of digital breast tomosynthesis and full-field digital mammography. Acad Radiol (2019) 26(16):735–43. doi: 10.1016/j.acra.2018.06.019
112. Wang H, Feng J, Zhang Z, Su H, Cui L, He H, et al. Breast mass classification via deeply integrating the contextual information from multi-view data. Pattern Recognit (2018) 80:42–52. doi: 10.1016/j.patcog.2018.02.026
113. Charan S, Khan MJ, Khurshid K. (2018). Breast cancer detection in mammograms using convolutional neural network. In: Proceedings of the 2018 international conference on computing, mathematics and engineering technologies (iCoMET). Sukkur, Pakistan: IEEE (2018). pp. 1–5. doi: 10.1109/ICOMET.2018.8346384
114. Feng Y, Zhang L, Yi Z. Breast cancer cell nuclei classification in histopathology images using deep neural networks. Int J Comput Assist Radiol Surg (2018) 13(2):179–91. doi: 10.1007/s11548-017-1663-9
115. Bardou D, Zhang K, Ahmad SM. Classification of breast cancer based on histology images using convolutional neural networks. IEEE Access (2018) 6: 24680–93. doi: 10.1109/access.2018.2831280
116. Nahid AA, Kong Y. Histopathological breast-image classification using local and frequency domains by convolutional neural network. Inf (Switzerland) (2018) 9(1):19. doi: 10.3390/info9010019
117. Touahri R, AzizI N, Hammami NE, Aldwairi M, Benaida F. (2019). Automated breast tumor diagnosis using local binary patterns (LBP) based on deep learning classification. In: Proceedings of the 2019 international conference on computer and information sciences (ICCIS). Sakaka, Saudi Arabia: IEEE (2019). pp. 1–5. doi: 10.1109/ICCISci.2019.8716428
118. Abdel Rahman AS, Belhaouari SB, Bouzerdoum A, Baali H, Alam T, Eldaraa AM. (2020). Breast mass tumor classification using deep learning. In: Proceedings of the 2020 IEEE international conference on informatics, iot, and enabling technologies (ICIoT). Doha, Qatar: IEEE (2020). pp. 271–6. doi: 10.1109/ICIoT48696.2020.9089535
119. Khamparia A, Khanna A, Thanh DNH, Gupta D, Podder P, Bharati S, et al. Diagnosis of breast cancer based on modern mammography using hybrid transfer learning. Multidim Syst Sign Process (2021) 32:747–65. doi: 10.1007/s11045-020-00756-7
120. Kavitha T, Mathai PP, Karthikeyan C, Ashok M, Kohar R, Avanija J, et al. Deep learning based capsule neural network model for breast cancer diagnosis using mammogram images. Interdiscip Sci Comput Life Sci (2022) 14:113–29. doi: 10.1007/s12539-021-00467-y
121. Frazer HM, Qin AK, Pan H, Brotchie P. Evaluation of deep learning-based artificial intelligence techniques for breast cancer detection on mammograms: results from a retrospective study using a breastscreen victoria dataset. J Med Imaging Radiat Oncol (2021) 65(5):529–37. doi: 10.1111/1754-9485.13278
122. Kim KH, Nam H, Lim E, Ock CY. Development of AI-powered imaging biomarker for breast cancer risk assessment on the basis of mammography alone. J Clin Oncol (2021) 39(15):10519–9. doi: 10.1200/JCO.2021.39.15_suppl.10519
123. Li H, Chen D, Nailon WH, Davies ME, Laurenson DI. Dual convolutional neural networks for breast mass segmentation and diagnosis in mammography. IEEE Trans Med Imaging (2022) 41(1):3–13. doi: 10.1109/TMI.2021.3102622
124. Shams S, Platania R, Zhang J, Kim J, Lee K, Park SJ. (2018). Deep generative breast cancer screening and diagnosis. In: Proceedings of the international conference on medical image computing and computer-assisted intervention, Granada, Spain. Granada, Spain: Springer (2018). 11071:859–67. doi: 10.1007/978-3-030-00934-2_95
125. Shen L, Margolies LR, Rothstein JH, Fluder E, McBride R, Sieh W. Deep learning to improve breast cancer detection on screening mammography. Sci Rep (2019) 9:1–12. doi: 10.1038/s41598-019-48995-4
126. Tsochatzidis L, Costaridou L, Pratikakis I. Deep learning for breast cancer diagnosis from mammograms—A comparative study. J Imaging (2019) 5:37. doi: 10.3390/jimaging5030037
127. Agnes SA, Anitha J, Pandian SIA, Peter JD. Classification of mammogram images using multiscale all convolutional neural network (MA-CNN) J. Med Syst (2020) 44:1–9. doi: 10.1007/s10916-019-1494-z
128. Kaur P, Singh G, Kaur P. Intellectual detection and validation of automated mammogram breast cancer images by multi-class SVM using deep learning classification. Inform Med Unlocked (2019) 16:100151. doi: 10.1016/j.imu.2019.01.001
129. Ting FF, Tan YJ, Sim KS. Convolutional neural network improvement for breast cancer classification. Expert Syst Appl (2019) 120:103–15. doi: 10.1016/j.eswa.2018.11.008
130. Falconi LG, Perez M, Aguilar WG, Conci A. Transfer learning and fine tuning in breast mammogram abnormalities classification on CBIS-DDSM database. Adv Sci Technol Eng Syst (2020) 5:154–65. doi: 10.25046/aj050220
131. Ansar W, Shahid AR, Raza B, Dar AH. Breast cancer detection and localization using mobilenet based transfer learning for mammograms. In: International symposium on intelligent computing systems. Sharjah, United Arab Emirates: Springer, Cham (2020) 1187:11–21. doi: 10.1007/978-3-030-43364-2_2
132. Zhang H, Wu R, Yuan T, Jiang Z, Huang S, Wu J, et al. DE-Ada*: A novel model for breast mass classification using cross-modal pathological semantic mining and organic integration of multi-feature fusions. Inf Sci (2020) 539:461–86. doi: 10.1016/j.ins.2020.05.080
133. Shayma'a AH, Sayed MS, Abdalla MI, Rashwan MA. Breast cancer masses classification using deep convolutional neural networks and transfer learning. Multimed Tools Appl (2020) 79:30735–68. doi: 10.1007/s11042-020-09518-w
134. Al-Antari MA, Han SM, Kim TS. Evaluation of deep learning detection and classification towards a computer-aided diagnosis of breast lesions in digital X-ray mammograms. Comput Methods Programs Biomed (2020) 196:105584. doi: 10.1016/j.cmpb.2020.105584
135. El Houby EM, Yassin NI. Malignant and nonmalignant classification of breast lesions in mammograms using convolutional neural networks. Biomed Signal Process Control (2021) 70:102954. doi: 10.1016/j.bspc.2021.102954
136. Zahoor S, Shoaib U, Lali IU. Breast cancer mammograms classification using deep neural network and entropy-controlled whale optimization algorithm. Diagnostics (2022) 12(2):557. doi: 10.3390/diagnostics12020557
137. Chakravarthy SS, Rajaguru H. Automatic detection and classification of mammograms using improved extreme learning machine with deep learning. IRBM (2021) 43:49–61. doi: 10.1016/j.irbm.2020.12.004
Keywords: breast cancer, classification, X-ray mammography, artificial intelligence, machine learning, deep learning, medical imaging, radiology
Citation: Wang L (2024) Mammography with deep learning for breast cancer detection. Front. Oncol. 14:1281922. doi: 10.3389/fonc.2024.1281922
Received: 23 August 2023; Accepted: 19 January 2024;
Published: 12 February 2024.
Edited by:
Yogesh Kumar, University Medical Center Hamburg-Eppendorf, GermanyReviewed by:
Jitendra Kuldeep, Cancer Research Center of Marseille, FranceMeghal Gandhi, Charles R. Drew University of Medicine and Science, United States
Copyright © 2024 Wang. This is an open-access article distributed under the terms of the Creative Commons Attribution License (CC BY). The use, distribution or reproduction in other forums is permitted, provided the original author(s) and the copyright owner(s) are credited and that the original publication in this journal is cited, in accordance with accepted academic practice. No use, distribution or reproduction is permitted which does not comply with these terms.
*Correspondence: Lulu Wang, bHdhbmczODFAaG90bWFpbC5jb20=; d2FuZ2x1bHVAc3p0dS5lZHUuY24=