- 1Department of Pharmacy, National University of Singapore, Singapore, Singapore
- 2Children’s Blood and Cancer Centre, KK Women’s and Children’s Hospital, Singapore, Singapore
- 3Duke-National University of Singapore (NUS) Medical School, Singapore, Singapore
Acute myeloid leukemia (AML) is clinically and genetically a heterogeneous disease characterized by clonal expansion of abnormal hematopoietic progenitors. Genomic approaches to precision medicine have been implemented to direct targeted therapy for subgroups of AML patients, for instance, IDH inhibitors for IDH1/2 mutated patients, and FLT3 inhibitors with FLT3 mutated patients. While next generation sequencing for genetic mutations has improved treatment outcomes, only a fraction of AML patients benefit due to the low prevalence of actionable targets. In recent years, the adoption of newer functional technologies for quantitative phenotypic analysis and patient-derived avatar models has strengthened the potential for generalized functional precision medicine approach. However, functional approach requires robust standardization for multiple variables such as functional parameters, time of drug exposure and drug concentration for making in vitro predictions. In this review, we first summarize genomic and functional therapeutic biomarkers adopted for AML therapy, followed by challenges associated with these approaches, and finally, the future strategies to enhance the implementation of precision medicine.
Introduction
Acute myeloid leukemia (AML) is a heterogenous and molecularly complex blood and bone marrow malignancy. A median age of diagnosis for AML patients is about sixty eight years (1). The prognosis in the elderly AML (>65 years) patients is worse compared to younger patients (<65 years) with a 5-year median survival of less than 20% (2, 3). There has been a significant progress in understanding the biology of AML including identification of translocations leading to aberrant fusion proteins such as t(8:21) (q22;q22), inv(16) (p13.1q22), t(9;11) (p21.3;q23.3), t(15;17) (q22;q12), t(9;11) (p22;q23), or gene-specific somatic mutations in Fms-like tyrosine kinase (FLT3), IDH1/2, P53, CEBPA, NPM1, DNMT3A, c-KIT, TET2, MLL, and others. However, the standard of care (SOC) for AML has remained unchanged for more than five decades and involves combination of cytarabine and daunorubicin for most of the patients (3). There is no further room in escalating the intensity of chemotherapy due to the associated life-threatening toxicity. Recently the therapeutic arsenal has diversified since the advent of targeted agents against FLT3, BCL-2, and HDAC. However, the optimal strategy to use these newer drugs in isolation and in combination with chemotherapeutic agents needs to be explored further to prevent relapse and resistance to treatment.
For young adult AML patients, high-dose induction chemotherapy and allogeneic hematopoietic stem cell transplantation (Allo-HSCT) have proven to be an effective cure for around 60% of patients, but chronic toxicities and eventual relapse are common (4). The treatment options are limited for patients with refractory or relapsed (R/R) AML. The advent of risk stratification and targeted therapies in AML was possible because of extensive efforts in understanding the genetic and molecular landscape of AML. This, in turn has led to the development of new generation of drugs. However, there continue to be lack of predictive biomarkers to assign patients to suitable treatment arms (5, 6).
The concept of precision oncology stemmed from the real-world successes of imatinib in patients with BCR-ABL fusion and hormonal therapy for estrogen-positive breast cancer patients. The genomic precision medicine (GPM) approach utilizes presence of specific genetic alternations or mutations to guide treatment decisions. Although GPM has shown clinical success with EGFR or ALK inhibitors in lung adenocarcinoma, BRAF inhibitors in melanoma, and c-KIT in gastrointestinal stromal tumors, the clinical benefit in a large cohort of patients still remains obscure. Emerging data from genomics guided clinical trials such as NCI-MATCH trial, SHIVA trial, SIGNATURE program basket trial has met disappointment due to either limited assignment of patients to treatment arm or due to lack of targetable mutations or due to lower benefit (17% to 7%) in those that were assigned to the treatment arms compared to existing standard of care treatments (7–13). Recent data from NCI-MATCH trial showed that while 93% of tumor biopsies were successfully sequenced, treatment match rate was less than 26% (10). In some cases where patients were successfully matched to a treatment option, objective response rate was less than 38%, for instance in BRAFV600E mutated patients receiving dabrafenib and trametinib. Another randomized multicenter Phase II trial assessing the implementation of genomic precision medicine reported only 2% objective response rate (12). Ultimately, precision medicine progress so far has informed us limited clinical benefit and blunt reality that genotype alone does not reliably inform drug responses.
Over the last decade, functional precision medicine (FPM) approach has gained lot of attention due to technological advances in functional screening technologies, high-throughput platforms for drug testing, and rapid development of patient derived avatars. In FPM guided approach, the direct exposure of tumor cells to drug of choice followed by functional readout measurement is being used to rank drug sensitivity (14). This strategy does not rely on the presence of specific genetic abnormality and hence is applicable to larger patient population. While FPM approach is more generalized compared to GPM, the clinical implementation of FPM lags behind GPM. In this review, we do a narrative summary of the clinical implementation of genomic and functional precision medicine approaches for AML patients. We then delve into the future perspectives of combining both GPM and FPM strategies to further improve treatment stratification for AML.
Genomic precision medicine approach in AML
Genomic precision medicine gained huge attention over the past two decades. With the advent of next generation sequencing (NGS), and improved RNA-sequencing, applying genomic approaches to precision medicine became cost-effective, rapid, and precise strategy to identify treatment strategies. GPM involves the identification of specific genetic mutations using NGS and assigning the right drug based on the prevalence of genetic mutation present in the patient (Figure 1). The identification of specific genetic marker is a key step in assigning the precise drug for the patient. Burd et al. published a landmark clinical trial (Beat AML), the first comprehensive clinical trial to leverage genomic precision medicine to assign therapy for AML patients based on the presence of somatic mutations. The assignment of different regimen based on genetic mutations showed that patients enrolled on Beat AML assigned treatment (n=224) group had significantly longer survival (median 12.8 months, 95% CI 10.3–14.8) compared to either standard of care, 7 + 3 combination (median 3.9 months, 95% CI 2.1–8.8) or palliative care (median 0.6 months, 95% CI 0.4–0.8) groups, but not significantly different from investigational therapy arm (15, 16). An expansion cohort with additional drugs including Ven-AZA is currently recruiting patients (17).
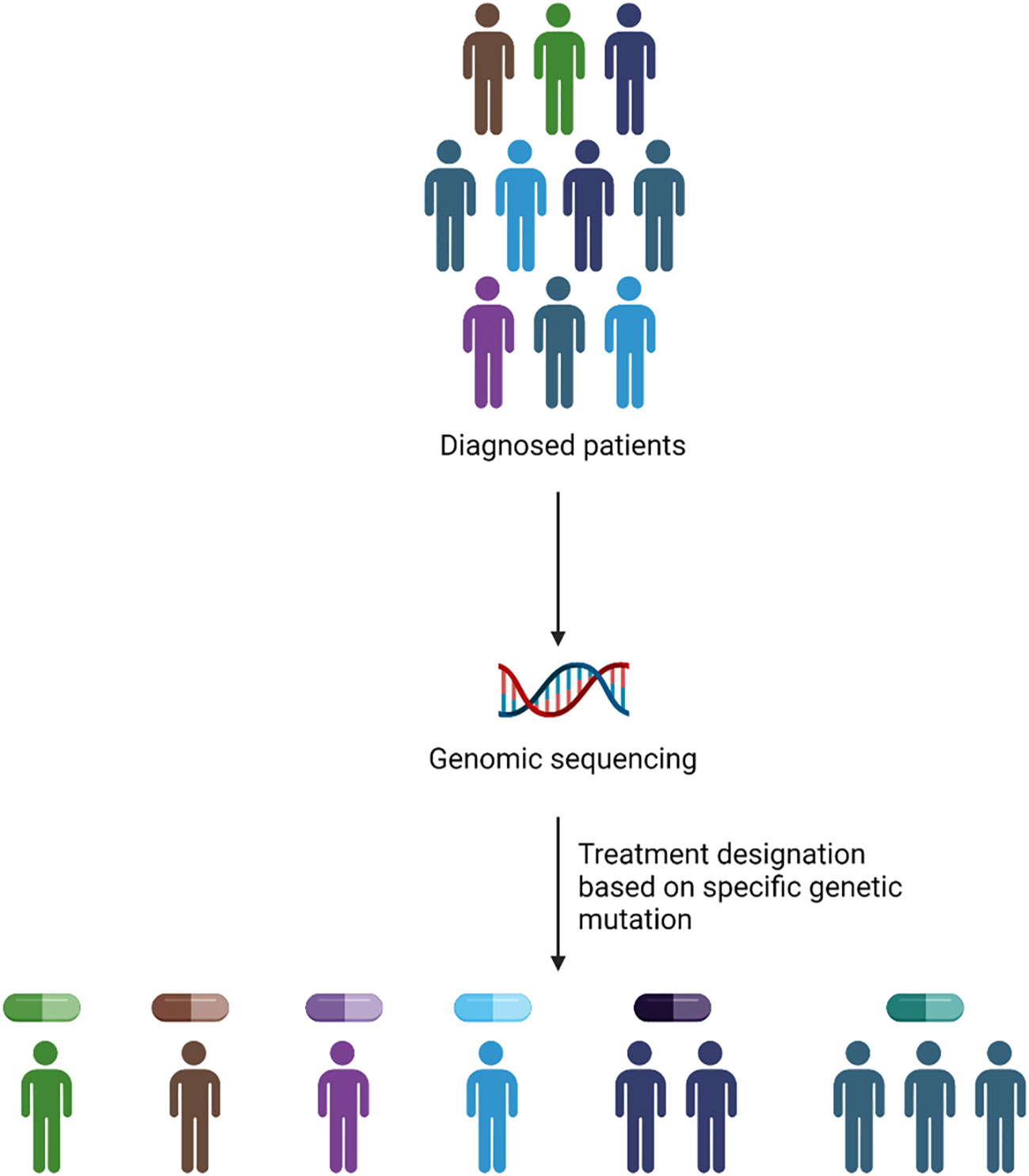
Figure 1 Methodology of GPM implementation in the clinic. The application of GPM depends upon the presence of genetic abnormality identified via genomic sequencing to assign personalized drug treatment.
In the following section, we discuss the key genetic mutations that are currently targeted for AML treatment and the associated clinical performance.
Targeting FLT3 mutations
About one-third of newly diagnosed AML patients carry genetic alterations in FLT3 gene (18). FLT3 mutations are classified into two groups: FLT3-ITD (internal tandem duplication), most frequent (10-25%), comprising duplication of nucleotide sequences with varying lengths and insertion sites, and FLT3-TKD found in 5-10% patients, comprising single-nucleotide variants in the tyrosine kinase domain (TKD) (3). Mutations in FLT3 gene leads to constitutive activation of FLT3 signaling, causing AML cell survival, proliferation, and differentiation by regulating pathways linked to PI3K/AKT/mTOR, STAT5, and RAS/MAPK (19). AML patients carrying FLT3 mutations have inferior prognosis and are at adverse risk but outcomes vary depending on the insertion site, allelic ratio and the mutational background (20). A favorable prognosis is observed in patients with FLT3-TKD when it is accompanied by co-mutations in NPM1 or core binding factor (CBF) AML patients (21). The discovery of second generation inhibitors against FLT3-ITD and FLT3-TKD has made it possible to target these mutated tyrosine kinases in AML patients.
Two classes of FLT3 inhibitors depending upon the binding conformation of FLT3 (active or inactive) are defined. RATIFY trial led to FDA approval of midostaurin, a type I inhibitor in combination with induction therapy in which the patients on combination arm showed significant improvement in event-free survival (EFS) (hazard ratio = 0.78; P = 0.002) and overall survival (OS) (hazard ratio = 0.78; P = 0.009) compared with induction therapy alone (22). Even though the RATIFY trial included only patients with age less than 60 years, the use of midostaurin is not limited to only this group of patients. The combination of midostaurin with induction or consolidation chemotherapy followed by 1-year maintenance was accessed for the efficacy in a phase III trial in FLT3-mutant AML adult patients (23). The OS and EFS were modestly improved with midostaurin (4-year OS 51% versus 44% for placebo), but approximately half of the patients did not benefit from the treatment due to which it has not been approved as a maintenance therapy.
Gilteritinib, a novel FLT3 inhibitor received FDA approval for R/R AML (24). Gilteritinib demonstrated significantly longer survival and higher remission rates as compared to salvage chemotherapy in patients with R/R AML (median OS 9.3 months vs 5.6 months in patients receiving salvage chemotherapy; P = 0.001). Gilteritinib also improved the relapse-free survival (RFS) of AML patients with FLT3-ITD after allogeneic HSCT (MJ 25). Patients with detectable minimal residual disease (MRD) showed better improvement than undetectable MRD. Crenolanib another type I FLT3 antagonist, demonstrated anti-leukemic activity in R/R FLT3 mutated AML patients in two phase 2 studies. Results from the first phase 2 trial of crenolanib with 38 patients reported a CRi rate of 23% in FLT3 inhibitor-naive patients and CRi rate of 5% in those who previously received FLT3 inhibitor. The median OS difference was also enhanced between the two groups of 55 and 13 weeks, respectively (26). The second phase 2 trial with 69 patients reported CRi rate of 39% and PR rate of 11% in relapsed FLT3-mutated FLT3 inhibitor naïve patients and CRi + PR rate of 28% in patients previously treated with FLT3 inhibitors. The median OS was 33.4 weeks in relapsed FLT3-mutated AML FLT3 inhibitor naïve patients and patients with FLT3-ITD had the longest overall survival (34 weeks) (27). The additional type II FLT3 inhibitor Quizartinib, type 2 FLT3 inhibitor was tested in recent clinical trial (QuANTUM-First) in combination with induction therapy in newly diagnosed FLT3-ITD+ AML patients. In comparison to placebo plus combination, quizartinib plus combination of induction therapy showed significant increase improvement in median OS in quizartinib arm (31·9 months (95% CI 21·0-not estimable) for quizartinib versus 15·1 months (13·2-26·2) for placebo plus induction therapy (28).
Although remarkable progress is made in the discovery of highly specific and potent FLT3 inhibitors, the emergence of relapse is unavoidable (29). FLT3 inhibitor resistance is mediated by inherent or acquired mechanisms. Higher expression of FLT3 ligand in bone marrow microenvironment and low variant allele frequency of: mutated FLT3 confer resistance to FLT3 inhibitors. Activation of MAPK/STAT5 signaling, rapid metabolism by liver CYP3A4 enzyme, CYP3A4 enzyme activity in bone marrow stromal cells, acquired FLT3-TKD secondary mutations, loss of FLT3-ITD, and activation of PI3K/AKT or RAS alternative signaling are some of the resistance mechanisms reported against FLT3 inhibitors (30–36).
Targeting IDH1/2 mutations
The frequency of IDH1/2 mutations in AML is approximately 15-20% (37, 38). IDH1 and IDH2 are different isoforms of iso-citrate dehydrogenase, a key enzyme in citrate metabolism catalyzing the conversion of isocitrate to α-ketoglutarate (αKg). Mutations in IDH1/2 causes reduction of αKg to 2-hydroxyglutarate (2-HG) which acts as a competitive inhibitor of αKg-dependent enzymes, namely TET family enzymes and lysine demethylases. The prognostic value of IDH mutations on AML prognosis is still actively being investigated, however, IDH1 mutations are generally associated with an inferior treatment outcome and IDH2 with a favorable treatment outcome (39, 40).
Multiple small molecule IDH1/2 inhibitors are currently under investigation for the treatment of AML. Ivosidenib is a first-in-class, selective, orally available, small-molecule inhibitor of IDH1. Ivosidenib reduces oncometabolite 2-HG levelsto induce differentiation of malignant cells (41). Ivosidenib received FDA approval for R/R AML in 2018 and newly diagnosed AML in 2019. The safety and efficacy of ivosidenib was evaluated in a phase 1 dose-escalation and dose-expansion study in IDH1-mutated AML R/R patients. Ivosidenib showed of 30.4% CRi(95% confidence interval [CI], 22.5 to 39.3),21.6% CR (95% CI, 14.7 to 29.8), and 41.6% ORR (95% CI, 32.9 to 50.8). The median duration of responses were 8.2 months (95% CI, 5.5 to 12.0), 9.3 months (95% CI, 5.6 to 18.3), and 6.5 months (95% CI, 4.6 to 9.3), respectively (42). Enasidenib (AG-221) is a first-in-class, oral, selective small molecule inhibitor of mutant IDH2 enzymes. AG-221 is a selective antagonist of mutant IDH2 variants R140Q, R172K, and R172S. Similar to Ivosidenib, Enasidenib also reduces of serum 2-HG level to cause myeloid differentiation (43). In 2017, enasidenib received FDA approval for IDH2 mutated R/R AML patients. The safety, pharmacokinetic and pharmacodynamic evaluation of enasidenib was evaluated in a phase 1/2, open-label study in advanced hematologic malignancy patients with IDH2 mutation. The overall response rate among patients with R/R AML was 40.3 percent, with a median response duration of 5.8 months. Responses were linked to cellular differentiation and maturation, although there was no indication of aplasia in most cases. The median overall survival in R/R patients was 9.3 months, and for the 34 patients (19.3%) who attained CR, the OS was 19.7 months (44).
Resistance to IDH inhibitor emerges through multiple mechanisms. Leukemia stemness plays a critical role in the primary resistance acquired to IDH inhibitors (45). Using LSC17 score Wang et al. observed a significantly higher LSC17 score in non- responders compared to responders (45). Other key mechanisms of primary resistance include epigenetic regulation, receptor tyrosine kinase (RTK) pathway mutation and MAPK pathway activation (46). The acquired resistance mechanisms to IDH1/2 inhibitors include mutations in RTK pathway, acquisition of secondary IDH mutations, isoform switching, adaptations in mitochondrial metabolism and clonal evolution/selection of resistant clones relying on other cell survival pathways (47).
Targeting TP53 mutations
The prevalence of TP53 mutation in de novo AML is around 5–10%. Chromosomal aneuploidies such as monosomy 5/deletion 5q (–5/5q–), –7/7q–, –17/17p–, complex and/or monosomal karyotypes and high-level DNA amplification are associated with TP53-mutant AML (48). TP53 mutant AML patients show poor prognosis and shorter response to induction therapy. TP53 mutations are commonly protein-altering missense mutations that exert a dominant-negative effect on p53 function. Targeting loss-of-function mutant p53 by small molecules to restore normal function of mutant p53 is adapted as therapeutic strategy (49). Eprenetapopt (APR-246/PRIMA-1Met) acts via covalent binding to specific cysteine residues (Cys124 and Cys277) in the protein core domain of mutant p53 to reactivate wild-type function of p53 (50) (51). Eprenetapopt was tested in combination with azacytidine in two separate phase Ib/II trials (n=11, and 18 respectively) in TP53-mutated MDS and AML patients. The trials reported CR/CRi rates of 36–45% in patients with 20–30% blasts (oligoblastic AML) and CR/CRi rate of 14% in AML patients with more than 30% blasts (52, 53). Contrary to these results, phase 3 trial showed no added benefit after combining eprenetapopt with azacitidine compared to azacitidine alone (CR rate 22.4% versus 33.3%) (54). A distinct approach of CD47 blockade via magrolimab in combination with azacytidine showed favorable outcomes in both TP53-mutant (40% CR, median OS 16.3 months) and wild-type patients with high-risk MDS (31% CR, median OS NR) (55). The ORR in AML patients was 71% (15/21) with 67% (14/21) of patients achieving CR/CRi. Despite initial promise, a recent randomized phase 3 (ENHANCE-2) trial, the combination of magrolimab plus azacitidine failed to show clinical benefit over venetoclax plus azacitidine or 7 + 3 chemotherapy in untreated TP53- mutant AML (NCT04778397).
Targeting epigenetic enzymes
Epigenetic regulome consists of a multitude set of ‘reader’, ‘writer’, and ‘eraser’ enzymes, that results in modifications such as methylation or acetylation of histones and methylation of DNA to module gene expression in irreversible manner. DNA methyl transferase 3 (DNMT3A) mutations occur in ~ 20% of AML patients, making it a potential target in this subset of AML patients. In a multicentre, randomized, open label phase III trial, azacitidine (DNMT3A inhibitor) showed improvement in MOS (10.4 months with 95% confidence interval [CI], 8.0-12.7 months) when compared with standard induction therapy (6.5 months with 95% CI, 5.0-8.6 months) (56). A phase III trial compared azacytidine in combination with gilteritinib versus azacytidine alone in newly diagnosed FLT3 mutated AML patients unfit for standard of care (57). There was no significant improvement in MOS in combination arm (9.82 months) in comparison to azacytidine alone (8.87 months) but CRc was improved (4.53 in combination vs 0.03 months in azacytidine alone). Similarly, IDH1 inhibitor ivosidenib in combination with azacytidine versus azacytidine alone was tested in newly diagnosed IDH1 mutant AML patients (58). A higher EFS and MOS in combination arm than azacytidine plus placebo was observed. A comparison of decitabine vs supportive care or low-dose cytarabine in a phase III trial revealed a non-significant increase in OS but an increment in CR/CRp of 17.8% with decitabine vs 7.8% in cytarabine or supportive care (59). Based on the results of an international phase III trial (QUAZAR AML-001 Maintenance Trial) of an orally administered azacitidine derivative CC-486, FDA approved CC-486 as maintenance therapy for adult AML patients who achieved CR/CRi after intensive induction chemotherapy and who are unfit for HSCT (60).
Other than DNMT inhibitors, histone deacetylase inhibitors such as panobinostat, pracinostat, and vorinostat and lysine demethylases inhibitors such as GSK2879552 have been evaluated in AML, but the results seem disappointing when they are combined with azacitidine (61–63). Other therapeutic options include pinometostat (DOT1L inhibitor) and FT-1101 (BRD4/BET inhibitor) (64, 65). One other potential target in epigenetic therapy is lysine specific demethylase 1 (LSD1/KDM1A). The inhibition of LSD1 has been possible by an inhibitor called Iadademstat which blocks the demethylation and scaffolding function of LSD1. Inhibition of both of these functions reduces leukemic stem cell regeneration and proliferation leading to the induction of differentiation in leukemic (66, 67). Iadademstat has been tested in combination with azacidine in a phase 2 trial in AML patients (68). The investigators reported 81% of the enrolled patients responded out of which 52% had CR/CRi and 82% of CR/CRi patients evaluated for MRD were negative by flow cytometry. We have summarized the key studies and the outcomes below in Table 1.
Challenges associated with GPM approach
Limited response rate
While GPM implementation led to a number of treatment successes in AML, only a small fraction of patients shows durable and deeper remissions from GPM assigned therapy. In FLT3 mutated patients, FLT3 inhibitors show overall response rate of ~ 49% (76). Despite promising clinical efficacy of FLT3 inhibitors, overall survival (OS) in FLT3-mutated AML vs WT is similar (77). Despite existing efforts to identify new mutations and development of targeted therapies against new targets, treatment-resistant cancers continue to emerge where non-genetic factors may also drive cancer development (78). Monotherapy targeting specific genetic abnormality is seldom effective to achieve complete response. Hence combination therapy against multiple target is generally preferred (79, 80). However, genomic sequencing alone often fails to assign combination therapy due to limited number of targetable mutations or lack of drug targeting novel genetic abnormalities.
Apart from the limited clinical benefit of genomic precision medicine, we also considered reasons that hinders the implementation of genomic precision medicine in the clinical setting. McCarthy et al. highlighted the issue of clinical validity pertaining to the use of genomic precision medicine (78). Here, clinical validity was referred as how consistent and accurate genomic testing in the clinical settings. The discovery and validation of biomarkers for each cancer type is an arduous process that requires large number of patients. The issue of identifying robust biomarker can be magnified in rare cancers with fewer number of enrolled patients (78). Another challenge with clinical implementation of GPM approach is physician’s lack of knowledge in the field of genomic precision medicine (81, 82) and even if they are well versed in the field of genomic precision medicine, there may still be uncertainties when interpreting genomic data (83–85) and choosing the most appropriate treatment. Due to the heterogeneous nature of a tumor, it can be challenging to characterize the genetic mutations in the tumor (86, 87) and to identify driver mutations that do not contribute to cancer growth (78). Interpreting these genomic data may not be easy and assigning treatment may be an issue. Whilst we appreciate that the concerns of clinical validity, lack of biomarkers have been researched in solid tumors; the concerns also apply to hematological malignancies in our opinion. Cumulatively, these factors hinder the clinical implementation of genomic precision medicine.
Co-mutations and non-targetable genetic changes
GPM success from the assigned targeted therapies relies on the presence of specific mutations. However, individual prognostic information derived from the presence or absence of a specific mutation does not take into account cooperating co-mutations that may cause altered oncogenic dependence and signaling (88). In a recent mutational spectrum study, over 90% of NRAS mutant AML patients had at least one co-mutation. Furthermore, treatment of patients with recurrent genetic mutations including FLT3-ITD, NPM1, DNMT3A, TET2 and KIT and fusion genes including AML1-ETO and MYH11-CBFβ are found to be unfavorable due to possible interplay between mutated genes (89). Adverse outcomes were observed in patients with DNMT3A and FLT3-ITD co-mutations (90). Beyond co-mutations, mutational burden of individual genes (VAF), can often play a key role in dictating responses to targeted therapy. Studies on the impact of clonal burden of TP53, TET2, DNMT3A, and NPM1 show that high VAF is associated with a shorter survival time (91). Absence of good predictive markers as well as the presence of non-targetable co-mutations are posing a big challenge to current GPM approaches.
Time to assignment of therapy
While recent technological advances has reduced the turnaround time for NGS data, it still requires several days to few weeks for genomic screening results. As opposed to indolent tumors, AML is a highly aggressive malignancy and requires immediate initiation of therapy to minimize disease-related morbidity and mortality (92). Data from a recent study that compared time from diagnosis to treatment showed no significant difference in OS based on treatment time delays (93). Hence it is still a debatable whether increase in time to treatment after diagnosis until sequencing data is obtained to enroll patients on GPM based treatment is safer option and will not affect assigned treatment outcomes. That being said having more rapid method of screening for AML-specific genetic mutations is desirable for rapid deployment of targeted therapies. Another caveat is that genomic sequencing provides a snapshot of a static event in the cell. Myeloblasts in AML bone marrow are dynamic, consisting of microenvironment as well as soluble factors. Due to this, the drug predicted by GPM often fail produce desired therapeutic effect despite of presence of genetic mutation. Often polyclonal heterogenous tumors upon relapse become oligoclonal with a complex genomic phenotype (94). Assignment of the precise drug to eradicate this complex population is a daunting task by genomic approach because of multiple co-genetic mutations, thus a combination of drugs, identified by testing multiple drugs using alternative precision medicine approaches are desired.
Functional precision medicine approach
FPM is underpinned by the idea that biological systems are exceptionally complex and predicting cellular response with initial conditions of genetic mutations can often be challenging and inaccurate. The core driving principle of functional precision medicine (FPM) relies on the perturbation of a system followed by the measurement of generated response (95).
FPM aims to revolutionize concept of precision medicine in which rather than relying on genetic biomarkers and mutations, it directly leverages tumor samples from patients to assess sensitivity to a multitude of anti-cancer agents. A functional measurement-based precision medicine provides particular advantage for cancers with poor response to drugs and high inter and intra-tumor heterogeneity, such as AML. Currently, FPM approach is implemented by using multiple approaches to identify personalized cancer therapy (Figure 2). In the subsequent session we discuss various FPM methods.
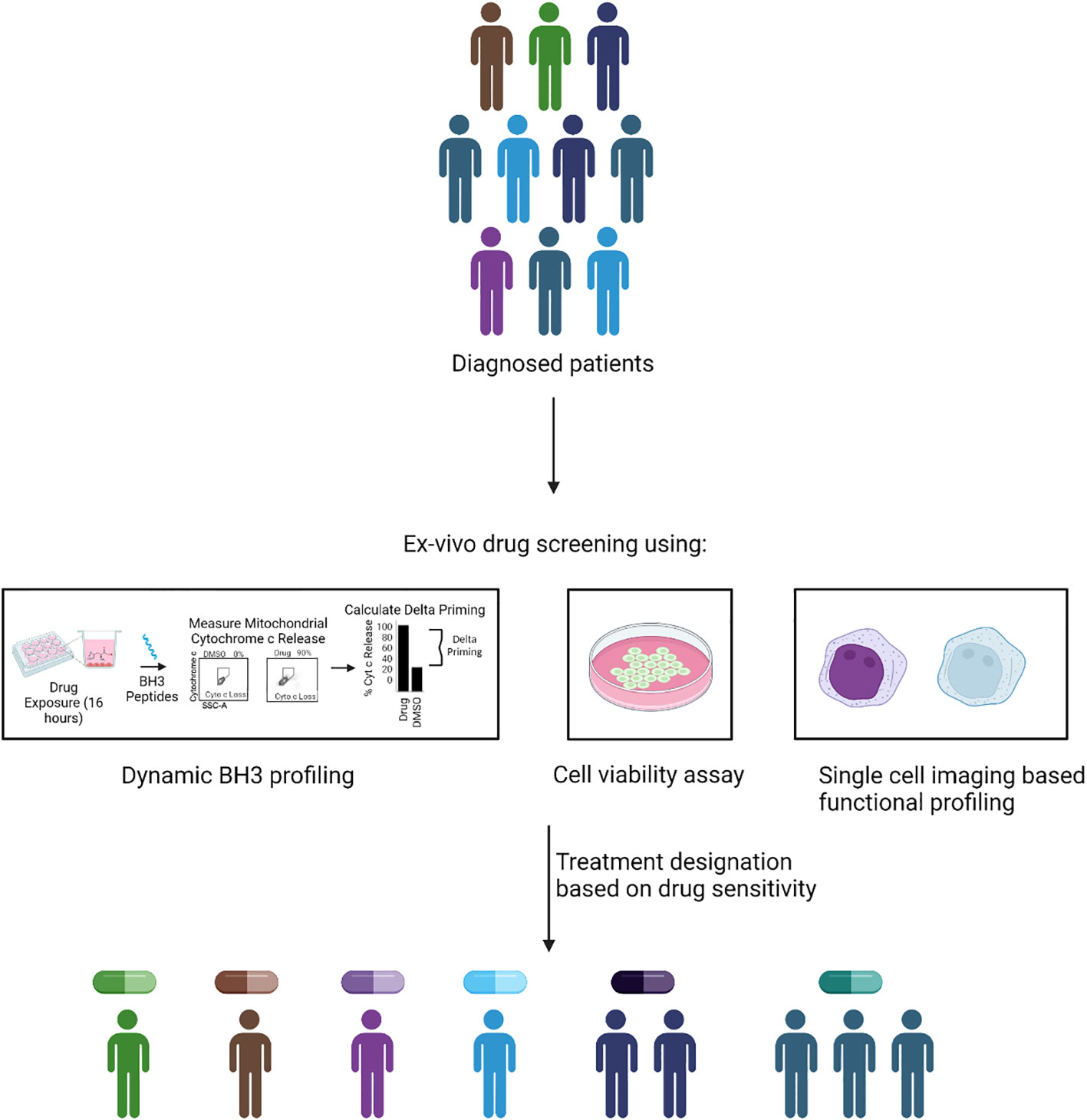
Figure 2 Methodology of FPM. In FPM approach, drug treatment is assigned based on the ex-vivo functional screening of isolated tumor cells. The functional screening could be done via different methods such as dynamic BH3 profiling, cell viability assay or imaging based functional phenotype assessment. The quantifiable read of the assay such as increase in apoptotic priming, reduced cell viability and functional change in phenotype is used assign treatment for patients based on their individual response.
Methods for FPM
Assigning BH3 mimetics therapy using BH3 profiling
Although mutations in BCL-2 was not identified as a putative genetic marker to assign therapy to AML patients, targeting BCL-2 has become one of the most successful targeted therapy in AML in current clinical practice. While evasion of apoptosis was documented as one of the hallmarks of cancer (96), its relevance in therapeutic targeting was not realized until implementation of FPM approach called BH3 profiling. BH3 profiling measures mitochondrial priming of cancer cells to determine threshold to apoptosis. Using BH3 peptides specific to proapoptotic BH3 only proteins, BH3 profiling can also infer anti-apoptotic dependence of specific cell type (97, 98). The cellular fate of survival or apoptosis is based on the balance between the BCL-2 family pro-survival proteins and the proapoptotic BH3-only proteins. Under the activation of proapoptotic BH3-only proteins, BAX and BAK oligomerizes on mitochondrial outer membrane to form pores followed by release of cytochrome c and downstream activation of caspases (99). The discovery of BCL-2 as oncogene which had anti-apoptotic activities, its binding with BH3 only pro-apoptotic proteins, and further reports of evidence showing the selection of malignant cells with high BCL-2 expression has led to the stimulus for targeting BCL2 via small molecule inhibitors such as BH3 mimetics (example venetoclax) (100, 101).
First-in-class BH3 mimetic developed to target BCL2 family protein was ABT-737 which has a broad-spectrum activity against the BCL-2, BCL-XL, BCL-XL and BCL-W. Using BH3 profiling approach, functional dependence on BCL-2 was first documented for chronic lymphocytic leukemia (CLL) which led to clinical testing of navitoclax in CLL patients (102). Despite of remarkable clinical activity, further progress was halted due to the on-target inhibition of BCL-XL that led to severe thrombocytopenia (103, 104). Further search for BH3 mimetics with BCL-2 specificity led to the discovery of highly potent BCL-2 inhibitor ABT-199 (Venetoclax) (105–108). By using BH3 profiling once again venetoclax showed on-target cytotoxicity for CLL cells at IC50 <10nM and this work led to clinical trial program that ultimately yielded multiple regulatory approval for venetoclax in CLL (102, 109). While AML cells did show evidence for functional dependence on BCL-2 using BH3 profiling, compared to CLL, AML myeloblast had heterogenous dependence (110). Clinical evaluation of venetoclax as a single agent in R/R AML patients revealed a short-lived response with a CR/CRi rate of 19% (111). The combination of venetoclax with LDAC resulted in a CR/CRi of 54% with a median time to first response of 1.4 months. The median OS was 10.1 months (95% CI, 5.7 to 14.2) (112); with HMA, the CR/CRi was 73% and 67% of all patients achieved CR (113, 114). The combination of venetoclax with IDH1 inhibitor ivosidenib in IDH1-mutant AML, with gilteritinib in FLT3 mutant AML patients, and with a MEK inhibitor has also been explored by (115–118). Stevens et al., have shown that the emergence of resistance against venetoclax and azacitidine combination is due to the upregulation of fatty acid metabolism via RAS pathway mutations or compensatory adaptations (119). The monocytic resistant clones lost the BCL-2 expression and were relying on MCL-1 for survival (120). Genomic deletion of BAX leading to venetoclax resistant MV4-11 AML cell lines have also been reported (121) as a resistance mechanism. Additional mechanisms of resistance by reduction in overall apoptotic priming in venetoclax resistant PDX cells and adaptive apoptotic rewiring via sequestration of pro-apoptotic proteins by MCL-1/BCL-XL rather than BCL-2 and/or decreased BAX expression have also been reported (122). Other than BCL-2, inhibitors targeting other specific BCL-2 family proteins like MCL-1, and BCL-XL are also being explored. (123–127).
Dynamic BH3 profiling and BH3 profiling assay
DBP method measures drug-induced apoptotic priming (delta priming) after treatment with proapoptotic BH3 peptides (128). In this approach, isolated cancer cells or tumor tissues are first exposed to drugs for 8-24 hours, followed by cell membrane permeabilization with digitonin and subsequent exposure to BH3 peptides derived for proapoptotic BH3 domains. Drug response is expressed as difference in cytochrome c release to indicate mitochondrial outer membrane permeabilization. The utility of DBP to accurately predict functional response of the cells has not been limited to hematological malignancies but was also implemented in solid tumors (129–132).
DBP approach was applied to discriminate the responders from non-responders in lenalidomide (LEN) plus MEC (mitoxantrone, etoposide, and cytarabine) combination chemotherapy inR/R AML patients. Increased mitochondrial signaling measured via DBP after a short-term ex vivo exposure of LEN to pretreatment myelobalsts was able to discriminate clinical responders from non-responders (133). To overcome resistance against venetoclax and S63845, Bhatt and colleagues utilized BH3 profiling and DBP and reported that the drug resistance is attributed to the reduction in mitochondrial apoptotic priming (122). Next they applied DBP as an FPM approach to identify the personalized treatment option for resistant models by exposing the myeloblasts isolated from AML PDX models to a panel of 40. DBP revealed that FLT3 inhibitors, SMAC mimetics, HDAC inhibitors, CDK9 inhibitors and MCL-1 inhibitors could be used to overcome the venetoclax resistance (122).
Ex vivo drug sensitivity assay
A functional screening of either single agent or combinations via measurement of cell viability has been extensively tested for efficacy in tailoring personalized medicine since decades. Using latest technologies and sophisticated culture conditions, several independent groups carried out ex vivo analysis of tumor cells to guide therapy for blood cancers (134–139). Kurtz et al. (139) applied ex vivo drug sensitivity assay to AML and CLL primary tumours and found that combinations involving kinase and MEK inhibitors were favorable in AML. They also correlated the combination ratio scores with patients’ genetics and other clinical markers and reported that certain combinations were specific to genetic markers but many combinations were effective irrespective of the genetic makeup of the patients, suggesting the relevance of FPM in identifying clinically effective combinations. Likewise, another study that utilized 151 primary leukemia samples to test panel of kinase inhibitors via in vitro cell viability assays, reported that functional vulnerability to kinase inhibitor may or may not be correlated to genetic abnormality (134). The Beat-AML study discussed in GPM also included ex-vivo drug sensitivity profiling and corelated the drug response to genomics and transcriptomics of the myeloblasts (15). The initial Beat-AML cohort was expanded with an expansion cohort with size to 805 patients was recently published by Bottomly and colleagues showing correlation between transcriptomics and AML cell differentiation state regulating the ex-vivo drug response (140). The same group has published a recent study evaluating 25 venetoclax inclusive combinations in AML to overcome resistance to venetoclax and azacitidine (141). They utilized ex vivo drug screening to test the combinations and correlated the drug response to tumor cell differentiation state.
Malani et al. implemented first of its kind ex vivo drug-sensitivity response (DSRT) assay to first time evaluate the clinical efficacy of functionally assigned therapy in AML patients. By setting up functional precision tumor board (FPTMB) for AML, Malani and colleagues integrated ex vivo monotherapy responses against 515 anticancer drugs with clinical, molecular, and genomic data for a total of 252 primary AML tumors collected from 186 patients (138). Based on the specific criteria devised in the study, four major drugs (venetoclax, BCL2i; sunitinib, VEGFR/FLT3i; dasatinib, BCR-ABL1/other kinases inhibitor, and temsirolimus mTORi) were tested for the clinical implementation of the FPMTB data. The drugs combinations (two-three drugs) were administered based on patient-specific sensitivity to single drugs and molecular data. Leveraging the FPMTB guided therapy, successful responses were recorded in 59% of R/R AML (n=29), of which 45% of patients (n=13) achieved CR/CRi. Of note, the DSRT assay provided actionable data in 3 days, faster than genomic and transcriptomic profiling, recommending rapid implementation of treatment options in patients who have failed the standard therapy and those requiring urgent alternative therapy options.
In another approach, the functional therapy assignment was leveraged based on drug responses were quantified via high-content microscopy at single-cell resolution in patient biopsy samples (137). A first-in-class prospective trial, Extended Analysis for Leukemia and Lymphoma Treatment (EXALT), was conducted to evaluate image-based single-cell functional precision medicine (scFPM) approach in guiding treatments in 143 patients with advanced aggressive hematologic cancers (137). scFPM approach assigned fifty-six patients (39%) for subsequent therapy and reported 54% patients (30 out of 56) achieved clinical benefit with PFS > 1.3 months after a median follow-up of 23.9 months. Encouragingly, scFPM-matched therapy resulted in a mean PFS of 276 days compared with 121 days on their previous treatment (P =0.0039), whereas nonmatched therapy led to a mean PFS of 96 days with a mean previous PFS of 121 days (P = 0.51).
The application of ex-vivo drug profiling or pharmacotyping has also been applied in acute lymphoblastic leukemia (ALL). Gocho et al., reported ex-vivo functional screening of 352 pediatric and adult T-ALL patients to characterize the sensitivity of dasatanib, a BCR-ABL kinase inhibitor (136). Although T-ALL patients do not show BCR-ABL fusion, the study reported that the functional screening with dasatanib revealed sensitivity in 44.4% of pediatric T-ALL patients, indicating the importance of FPM based approaches in identifying effective therapy. They further characterized dasatinib sensitivity in T-ALL patients by network-based systems pharmacology to examine the signal circuitry and reported that preTCR-LCK activation was correlated with dasatinib sensitivity. A similar approach of pharmacotyping was done in 805 pediatric ALL primary samples with 18 drugs to define whether leukemia genomics and MRD status influence drug sensitivity (142). They identified distinct genomics clusters and MRD level contributing to the drug sensitivity in B-ALL and T-ALL pediatric patients.
FPM approach has been extended to multiple myeloma (MM) to devise new combinatorial treatment. Rashid et al., reported a quadratic phenotypic optimization platform (QPOP) to identify new combinatorial treatments based on the ex-vivo screening of 114 approved drugs (135). The uniqueness of this approach lies in its methodology in which no molecular mechanisms or predetermined drug synergy data is required. This approach utilized quadratic surfaces to model the biological effects of drug combinations to identify effective drug combinations. They successfully utilized QPOP to devise new combinations in bortezomib resistant MM cell lines and xenograft mouse models.
The next major question is whether FPM is clinically feasible to guide the treatment in prospective fashion. A prospective non-interventional SMARTrial that tested the clinical feasibility and predictive powers of ex vivo drug response profiling addressed that it is indeed possible to prospectively assign therapy using FPM approach (143). SMARTrial tested end points in which the primary endpoint provided drug response in 7 days and the secondary endpoint correlated the findings of the ex-vivo response to the in vivo drug activity in patients. Investigators reported that the trial met the primary endpoint in 91.3% (95% confidence interval (95% CI) 82.8–96.4%) of all eligible participants. To associate the ex vivo drug sensitivity and in vivo response, they conducted logistic regression on ex vivo drug response profiles of individual chemotherapeutic agents to in vivo response and reported a median cross-validation area under the receiver operating characteristic curve (AUROC) of 0.84 to 0.85 with a model of 5 drugs used as prognostic features. They also regressed EFS to the ex vivo response and reported a stronger ex-vivo response was associated with extended EFS. They also validated this approach in 95 AML patients reporting a stronger correlation between in vivo response to cytarabine and daunorubicin and ex vivo drug sensitivity.
An independent clinical trial, VenEx tested the utility and predictiveness of venetoclax ex vivo sensitivity in de novo (patients ineligible for induction therapy), R/R or secondary AML patients (144). The study reported that the cell culture media also influences the ex vivo drug sensitivity profile and condition media based on RPMI with supernatant from stroma cells outperformed. The treatment response was achieved in 88% of the ex-vivo drug sensitive patients. They also reported a significant longer median survival for participants who were ex vivo-sensitive to venetoclax (14.6 months for venetoclax-sensitive patients vs. 3.5 for venetoclax-insensitive patients, P<0.001). However, ability of VenEx study to prospectively identify insensitive patients remained to be determined via ROC analysis (144).
Assigning treatment based on the mutational status of the patient is not productive as the underlying biology of the tumor is extensively complex. Therefore, a combination of genetic and functional predictive biomarkers is needed to guide the therapy decision. Some of the predictive biomarkers for specific agents are provided in Table 2.
Challenges associated with FPM approach
FPM approach provides significant advantage over GPM by excluding the inherent requirement of presence of genetic abnormality. FPM enables generalized applicability of the approach to broader population of patients. But there remain inherent challenges to FPM approach that needs to be overcome in the coming years. Firstly there is the requirement for viable tissues. For multicentre prospective clinical studies, it is imperative to optimize the storage as well as transport conditions to ensure good tissue viability. Also, since viable tissues are required, there needs to be enough cells for implementation of FPM (95). It is also difficult to optimize drug concentration and treatment duration and interval to be used for ex vivo FPM testing as the drug concentration varies across patients due to interindividual differences in pharmacokinetics (metabolism and elimination) (95). Since ex-vivo testing is done away from the tumor microenvironment, there remains a possibility that the predicted drug combinations maybe less efficacious in patients. Therefore, model used for FPM testing must be carefully considered. In case of organoid models, tumor microenvironment cells are excluded. These cells play a role in regulating drug responses (150) and therefore, this method does not truly represent the tumor and the interaction with their tumor microenvironment. PDX models may exhibit a more realistic tumor microenvironment, however, human tumor cell-stroma cell interactions are affected with the original human stromal cells gradually replaced by murine stromal cells (151). Importantly interaction with immune cells cannot be tested in PDX models as these models are derived in immunodeficient mice (152). FPM testing may take longer time in certain approaches, especially if ex vivo cell expansion is required. To truly adopt FPM as a global standard, better standardization practices pertaining to doses, time to measure perturbance, tumor mass need to be established for it to produce reliable and replicable results need to be addressed rigorously.
Looking beyond genomic and functional approach
GPM and FPM have made an impact in targeting complex tumor biology, but both approaches have inherent limitations. Because of rapid development in technology, a precision medicine approach based on omics technology (proteomics or transcriptomics) might become a possibility in future years. As deregulation of different types of proteins such as signaling or sensor proteins is usually observed at the transcriptional (in terms of RNA transcripts) or translational level in disease state, identification and targeting these specific set of vulnerabilities is a possible way of delivering precision medicine for the patients. Proteomics based precision medicine would require mass spectrometry or high throughput affinity-based methods like ELISA, aptamers or antibody labelled nucleotide arrays to quantify the proteins in individual patient samples (153). Integration of genomic with transcriptomics and proteomic data will be a better strategy to understand the significance of genomic alterations at the translational level and to better identify biomarkers for effective targeting (154). Beyond this state-of-the-art single cell RNA sequencing (scRNA-seq) may dwell upon in near future to aid personalized medicine. Single cell technology will offer a unique advantage of deconvoluting clonal heterogeneity of the bulk tumor to specific subset of tumor population. Such knowledge could enable identification of unique biomarkers in different clusters of tumor population to deliver precision medicine to target clonal heterogeneity and thus the emergence of resistance clones. A detailed description of transcriptomics based precision medicine has been reviewed elsewhere (155, 156).
Future perspectives
The evolution of different treatment strategies allude to the ever-growing nature of precision medicine. As our understanding of AML improves, so would the strategies undertaken to address it. Although a genetic approach to precision medicine has improved clinical outcomes for specific subgroup of patients carrying mutations, its ability to assist in guiding therapy for a broad spectrum of patients remains limited. This limitation becomes especially evident when used in a heterogeneous cancer like AML. In comparison, functional precision medicine allows identification of personalized regimens that do not rely on mutations or the genomic makeup of a patient, allowing for much broader implementation. However, functional approaches also have limitations that need to be addressed in coming years. These approaches require fresh tumor samples and are still in infancy due to requirement for robust standardization. However, in the coming years, field will improve on the shortcomings and will improve on the existing functional approaches to enable broader use of it as predictive biomarker.
Integrating functional approaches with genomics would not only yield greater insight into the biology of AML, as observed by Malani et al. but also allow for better treatments to be designed for AML. We posit a growing convergence of these two techniques in clinical trials over the coming years (Figure 3). This would allow the use of FPM and GPM, working in tandem to potentially confer a greater degree of benefit to patients with AML. A recent example of such case is the use of triplet therapy consisting of venetoclax, hypomethylating agent (decitabine) and FLT-3 inhibitor gilteritinib in FLT-3 mutated AML. A phase-II trial validated this combination in newly diagnosed and relapsed/refractory patients and reported the CRc rate of 92% with MRD negativity by FCM in 56% and by PCR/NGS in 91% of responders in newly diagnosed group and the CRc rate of 62% with MRD negativity rate by FCM in 63% and by PCR/NGS in 100% of responders in R/R group (157). The enormous data generated through such precision medicine approaches could help to identify and develop novel drug combinations using machine learning (ML) algorithms. ML could help in the identification of synergism between drugs and also help to predict if a specific genetic signature is correlated with a selected drug sensitivity profile to a panel of drugs. One example of such study was reported by Lee and the colleagues., in which they integrated in vitro drug sensitivity to 160 chemotherapy drugs in 14 AML cell lines and genome wide-gene expression profiles of 30 AML patients to identify molecular markers in guiding the treatment of AML patients (158). Their machine learning approach was named MERGE based on its utilization of mutation, expression hubs, known regulators, genomic CNV, and methylation data to predict the gene-drug association. They compared MERGE with existing conventional methods and compared the consistency rate (number of significant gene-drug associations predicted by each method) and reported a higher consistency rate with MERGE. They also utilized MERGE to predict the molecular markers to topoisomerase II inhibitors and reported SMARCA4 as a potential marker in AML driving the sensitivity towards topoisomerase II inhibitors. Other such studies predicting the sensitivity of a drug combination and overall application of ML has been reviewed here (159).
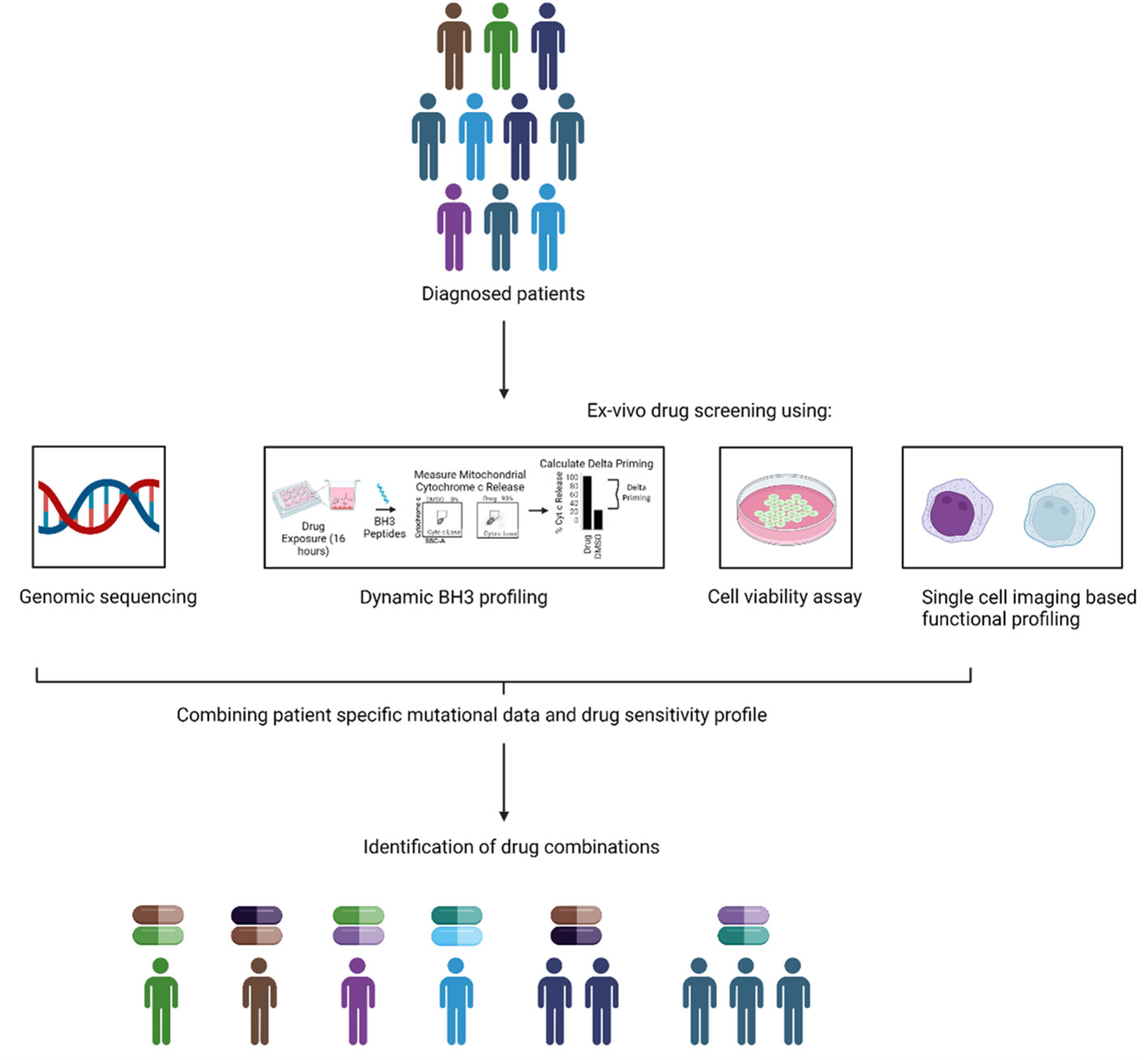
Figure 3 Combining genomic and functional precision medicine. An alternative to the current GPM and FPM based approach is to combine them together to assign therapy. In the combination approach, the genomic and functional data could be combined to guide the treatment to design new and precise combinations of the drugs for individual patients.
The success of GPM, FPM or combination based therapy would also depend on the diversity of population enrolled in clinical trials. Increasing representation of minority and underserved populations in clinical trials is important to overcome inherent bias in such studies. To improve representation, policy makers, healthcare providers and insurance providers need to work in tandem.
Extraordinary progress has been made in the understanding of predictive biomarkers as well as AML biology. This, in combination with recent approaches in integrating synergistic therapies with novel agents and the use of ML algorithms promises a successful future in improving outcomes in AML using personalized medicine approaches.
Author contributions
KB: Conceptualization, Writing – original draft, Writing – review & editing. VS: Writing – original draft. MW: Writing – original draft. PI: Conceptualization, Supervision, Writing – original draft, Writing – review & editing. SB: Conceptualization, Supervision, Writing – original draft, Writing – review & editing.
Funding
The author(s) declare that no financial support was received for the research, authorship, and/or publication of this article.
Conflict of interest
The authors declare that the research was conducted in the absence of any commercial or financial relationships that could be construed as a potential conflict of interest.
Publisher’s note
All claims expressed in this article are solely those of the authors and do not necessarily represent those of their affiliated organizations, or those of the publisher, the editors and the reviewers. Any product that may be evaluated in this article, or claim that may be made by its manufacturer, is not guaranteed or endorsed by the publisher.
References
1. Appelbaum FR, Gundacker H, Head DR, Slovak ML, Willman CL, Godwin JE, et al. Age and acute myeloid leukemia. Blood (2006) 107:3481–5. doi: 10.1182/blood-2005-09-3724
2. Thein MS, Ershler WB, Jemal A, Yates JW, Baer MR. Outcome of Older Patients with Acute Myeloid Leukemia: An analysis of SEER Data over Three Decades. Cancer (2013) 119:2720–7. doi: 10.1002/CNCR.28129
3. Kantarjian H, Kadia T, DiNardo C, Daver N, Borthakur G, Jabbour E, et al. Acute myeloid leukemia: current progress and future directions. Blood Cancer J (2021) 11:1–25. doi: 10.1038/s41408-021-00425-3
4. Döhner H, Weisdorf DJ, Bloomfield CD. Acute myeloid leukemia. N Engl J Med (2015) 373:1136–52. doi: 10.1056/NEJMRA1406184
5. Prasad V. Perspective: The precision-oncology illusion. Nature (2016) 537:S63. doi: 10.1038/537S63A
6. Letai A. Functional precision cancer medicine-moving beyond pure genomics. Nat Med (2017) 23:1028–35. doi: 10.1038/NM.4389
7. Le Tourneau C, Delord JP, Gonçalves A, Gavoille C, Dubot C, Isambert N, et al. Molecularly targeted therapy based on tumour molecular profiling versus conventional therapy for advanced cancer (SHIVA): a multicentre, open-label, proof-of-concept, randomised, controlled phase 2 trial. Lancet Oncol (2015) 16:1324–34. doi: 10.1016/S1470-2045(15)00188-6
8. Massard C, Michiels S, Ferté C, Le Deley MC, Lacroix L, Hollebecque A, et al. High-throughput genomics and clinical outcome in hard-to-treat advanced cancers: Results of the MOSCATO 01 trial. Cancer Discovery (2017) 7:586–95. doi: 10.1158/2159-8290.CD-16-1396
9. Sicklick JK, Kato S, Okamura R, Schwaederle M, Hahn ME, Williams CB, et al. Molecular profiling of cancer patients enables personalized combination therapy: the I-PREDICT study. Nat Med (2019) 25:744–50. doi: 10.1038/s41591-019-0407-5
10. Flaherty KT, Gray RJ, Chen AP, Li S, McShane LM, Patton D, et al. Molecular landscape and actionable alterations in a genomically guided cancer clinical trial: National cancer institute molecular analysis for therapy choice (NCI-MATCH). J Clin Oncol (2020) 38:3883–94. doi: 10.1200/JCO.19.03010
11. Middleton G, Fletcher P, Popat S, Savage J, Summers Y, Greystoke A, et al. The National Lung Matrix Trial of personalized therapy in lung cancer. Nat (2020) 583:807–12. doi: 10.1038/s41586-020-2481-8
12. Chen AP, Kummar S, Moore N, Rubinstein LV, Zhao Y, Williams PM, et al. Molecular profiling-based assignment of cancer therapy (NCI-MPACT): A randomized multicenter phase II trial. JCO Precis Oncol (2021) 5:133–44. doi: 10.1200/PO.20.00372
13. Van Tilburg CM, Pfaff E, Pajtler KW, Langenberg KPS, Fiesel P, Jones BC, et al. The pediatric precision oncology inform registry: Clinical outcome and benefit for patients with very high-evidence targets. Cancer Discovery (2021) 11:2764–79. doi: 10.1158/2159-8290.CD-21-0094/673845/AM/THE-PEDIATRIC-PRECISION-ONCOLOGY-INFORM-REGISTRY
14. Letai A. Functional precision cancer medicine—moving beyond pure genomics. Nat Med (2017) 23:1028–35. doi: 10.1038/nm.4389
15. Tyner JW, Tognon CE, Bottomly D, Wilmot B, Kurtz SE, Savage SL, et al. Functional genomic landscape of acute myeloid leukaemia. Nature (2018) 562:526–31. doi: 10.1038/s41586-018-0623-z
16. Burd A, Levine RL, Ruppert AS, Mims AS, Borate U, Stein EM, et al. Precision medicine treatment in acute myeloid leukemia using prospective genomic profiling: feasibility and preliminary efficacy of the Beat AML Master Trial. Nat Med (2020) 26:1852–8. doi: 10.1038/S41591-020-1089-8
17. Borate UM, Huang Y, Johnson MF, Zeidner JF, Swords RT, Koenig KL, et al. A randomized phase 2 trial of 28-day (Arm A) versus 14-day (Arm B) schedule of venetoclax + Azacitidine in newly diagnosed acute myeloid leukemia patients ≥ 60 years. Blood (2023) 142:1535. doi: 10.1182/blood-2023-187383
18. Kennedy VE, Smith CC. FLT3 mutations in acute myeloid leukemia: key concepts and emerging controversies. Front Oncol (2020) 10:612880. doi: 10.3389/fonc.2020.612880
19. Takahashi S. Downstream molecular pathways of FLT3 in the pathogenesis of acute myeloid leukemia: biology and therapeutic implications. J Hematol Oncol (2011) 4:13. doi: 10.1186/1756-8722-4-13
20. Bullinger L, Döhner K, Dohner H. Genomics of acute myeloid leukemia diagnosis and pathways. J Clin Oncol (2017) 35:934–46. doi: 10.1200/JCO.2016.71.2208
21. Voso MT, Larson RA, Jones D, Marcucci G, Prior T, Krauter J, et al. Midostaurin in patients with acute myeloid leukemia and FLT3-TKD mutations: a subanalysis from the RATIFY trial. Blood Adv (2020) 4:4945–54. doi: 10.1182/BLOODADVANCES.2020002904
22. Stone RM, Mandrekar SJ, Sanford BL, Laumann K, Geyer SM, Bloomfield CD, et al. The addition of midostaurin to standard chemotherapy decreases cumulative incidence of relapse (CIR) in the international prospective randomized, placebo-controlled, double-blind trial (CALGB 10603 / RATIFY [Alliance]) for newly diagnosed acute myeloid leukemia (AML) Patients with FLT3 Mutations. Blood (2017) 130:2580. doi: 10.1056/NEJMoa1614359
23. Stone RM, Mandrekar SJ, Sanford BL, Laumann K, Geyer S, Bloomfield CD, et al. Midostaurin plus chemotherapy for acute myeloid leukemia with a FLT3 mutation. N Engl J Med (2017) 377:454–64. doi: 10.1056/NEJMOA1614359
24. Perl AE, Martinelli G, Cortes JE, Neubauer A, Berman E, Paolini S, et al. Gilteritinib or chemotherapy for relapsed or refractory FLT3-mutated AML. N Engl J Med (2019) 381:1728–40. doi: 10.1056/NEJMOA1902688
25. Levis MJ, Hamadani M, Logan B. BMT-CTN 1506 (MORPHO): A randomized trial of the FLT3 inhibitor gilteritinib as post-transplant maintenance for FLT3-ITD AML. EHA 2023 Hybrid Congr Abstr (2023), LB271.
26. Randhawa JK, Kantarjian HM, Borthakur G, Thompson PA, Konopleva M, Daver N, et al. Results of a phase II study of crenolanib in relapsed/refractory acute myeloid leukemia patients (Pts) with activating FLT3 mutations. Blood (2014) 124:389. doi: 10.1182/BLOOD.V124.21.389.389
27. Cortes JE, Kantarjian HM, Kadia TM, Borthakur G, Konopleva M, Garcia-Manero G, et al. Crenolanib besylate, a type I pan-FLT3 inhibitor, to demonstrate clinical activity in multiply relapsed FLT3-ITD and D835 AML. J Clin Oncol (2016) 34(15_suppl):7008. doi: 10.1200/JCO.2016.34.15_suppl.7008
28. Erba HP, Montesinos P, Kim H-J, Patkowska E, Vrhovac R, Žák P, et al. Quizartinib plus chemotherapy in newly diagnosed patients with FLT3-internal-tandem-duplication-positive acute myeloid leukaemia (QuANTUM-First): a randomised, double-blind, placebo-controlled, phase 3 trial. Lancet (London England) (2023) 401:1571–83. doi: 10.1016/S0140-6736(23)00464-6
29. Daver N, Cortes J, Ravandi F, Patel KP, Burger JA, Konopleva M, et al. Secondary mutations as mediators of resistance to targeted therapy in leukemia. Blood (2015) 125:3236–45. doi: 10.1182/BLOOD-2014-10-605808
30. Piloto O, Wright M, Brown P, Kim KT, Levis M, Small D. Prolonged exposure to FLT3 inhibitors leads to resistance via activation of parallel signaling pathways. Blood (2007) 109:1643–52. doi: 10.1182/BLOOD-2006-05-023804
31. Dutreix C, Munarini F, Lorenzo S, Roesel J, Wang Y. Investigation into CYP3A4-mediated drug-drug interactions on midostaurin in healthy volunteers. Cancer Chemother Pharmacol (2013) 72:1223–34. doi: 10.1007/S00280-013-2287-6
32. Traer E, Martinez J, Javidi-Sharifi N, Agarwal A, Dunlap J, English I, et al. FGF2 from marrow microenvironment promotes resistance to FLT3 inhibitors in acute myeloid leukemia. Cancer Res (2016) 76:6471–82. doi: 10.1158/0008-5472.CAN-15-3569
33. Chang Y-T, Hernandez D, Ghiaur G, Levis MJ, Jones RJ. Bone marrow stroma protects FLT3 acute myeloid leukemia (AML) through CYP3A4-mediated drug metabolization of FLT3 tyrosine kinase inhibitors (TKIs). Blood (2017) 130:2519. doi: 10.1182/blood.V130.Suppl_1.2519.2519
34. McMahon CM, Ferng T, Canaani J, Wang ES, Morrissette JJD, Eastburn DJ, et al. Clonal selection with RAS pathway activation mediates secondary clinical resistance to selective FLT3 inhibition in acute myeloid leukemia. Cancer Discovery (2019) 9:1050–63. doi: 10.1158/2159-8290.CD-18-1453/333364/AM/CLONAL-SELECTION-WITH-RAS-PATHWAY-ACTIVATION
35. Zhang H, Savage S, Schultz AR, Bottomly D, White L, Segerdell E, et al. Clinical resistance to crenolanib in acute myeloid leukemia due to diverse molecular mechanisms. Nat Commun (2019) 10:244. doi: 10.1038/S41467-018-08263-X
36. Schmalbrock LK, Dolnik A, Cocciardi S, Sträng E, Theis F, Jahn N, et al. Clonal evolution of acute myeloid leukemia with FLT3-ITD mutation under treatment with midostaurin. Blood (2021) 137:3093–104. doi: 10.1182/BLOOD.2020007626
37. Dinardo CD, Ravandi F, Agresta S, Konopleva M, Takahashi K, Kadia T, et al. Characteristics, clinical outcome, and prognostic significance of IDH mutations in AML. Am J Hematol (2015) 90:732–6. doi: 10.1002/AJH.24072
38. Molenaar RJ, Thota S, Nagata Y, Patel B, Clemente M, Przychodzen B, et al. Clinical and biological implications of ancestral and non-ancestral IDH1 and IDH2 mutations in myeloid neoplasms. Leuk (2015) 29:2134–42. doi: 10.1038/leu.2015.91
39. Patel JP, Gönen M, Figueroa ME, Fernandez H, Sun Z, Racevskis J, et al. Prognostic relevance of integrated genetic profiling in acute myeloid leukemia. N Engl J Med (2012) 366:1079–89. doi: 10.1056/NEJMOA1112304/SUPPL_FILE/NEJMOA1112304_DISCLOSURES.PDF
40. Im AP, Sehgal AR, Carroll MP, Smith BD, Tefferi A, Johnson DE, et al. DNMT3A and IDH mutations in acute myeloid leukemia and other myeloid Malignancies: associations with prognosis and potential treatment strategies. Leuk (2014) 28:1774–83. doi: 10.1038/leu.2014.124
41. Rohle D, Popovici-Muller J, Palaskas N, Turcan S, Grommes C, Campos C, et al. An inhibitor of mutant IDH1 delays growth and promotes differentiation of glioma cells. Science (2013) 340:626–30. doi: 10.1126/SCIENCE.1236062/SUPPL_FILE/ROHLE.SM.PDF
42. DiNardo CD, Stein EM, de Botton S, Roboz GJ, Altman JK, Mims AS, et al. Durable remissions with ivosidenib in IDH1 -mutated relapsed or refractory AML. N Engl J Med (2018) 378:2386–98. doi: 10.1056/NEJMOA1716984/SUPPL_FILE/NEJMOA1716984_DISCLOSURES.PDF
43. Yen K, Travins J, Wang F, David MD, Artin E, Straley K, et al. AG-221, a first-in-class therapy targeting acute myeloid leukemia harboring oncogenic IDH2 mutations. Cancer Discovery (2017) 7:478–93. doi: 10.1158/2159-8290.CD-16-1034/333274/AM/AG-221-A-FIRST-IN-CLASS-THERAPY-TARGETING-ACUTE
44. Stein EM, DiNardo CD, Pollyea DA, Fathi AT, Roboz GJ, Altman JK, et al. Enasidenib in mutant IDH2 relapsed or refractory acute myeloid leukemia. Blood (2017) 130:722–31. doi: 10.1182/BLOOD-2017-04-779405
45. Wang F, Morita K, DiNardo CD, Furudate K, Tanaka T, Yan Y, et al. Leukemia stemness and co-occurring mutations drive resistance to IDH inhibitors in acute myeloid leukemia. Nat Commun (2021) 12:1–13. doi: 10.1038/s41467-021-22874-x
46. Yao K, Liu H, Yu S, Zhu H, Pan J. Resistance to mutant IDH inhibitors in acute myeloid leukemia: Molecular mechanisms and therapeutic strategies. Cancer Lett (2022) 533:215603. doi: 10.1016/J.CANLET.2022.215603
47. Zhuang X, Pei HZ, Li T, Huang J, Guo Y, Zhao Y, et al. The molecular mechanisms of resistance to IDH inhibitors in acute myeloid leukemia. Front Oncol (2022) 12:931462/BIBTEX. doi: 10.3389/FONC.2022.931462/BIBTEX
48. Rücker FG, Schlenk RF, Bullinger L, Kayser S, Teleanu V, Kett H, et al. TP53 alterations in acute myeloid leukemia with complex karyotype correlate with specific copy number alterations, monosomal karyotype, and dismal outcome. Blood (2012) 119:2114–21. doi: 10.1182/BLOOD-2011-08-375758
49. Bykov VJN, Eriksson SE, Bianchi J, Wiman KG. Targeting mutant p53 for efficient cancer therapy. Nat Rev (2017) 18:89–102. doi: 10.1038/nrc.2017.109
50. Lehmann S, Bykov VJN, Ali D, Andreń O, Cherif H, Tidefelt U, et al. Targeting p53 in vivo: A first-in-human study with p53-targeting compound APR-246 in refractory hematologic Malignancies and prostate cancer. J Clin Oncol (2012) 30:3633–9. doi: 10.1200/JCO.2011.40.7783
51. Zhang Q, Bykov VJN, Wiman KG, Zawacka-Pankau J. APR-246 reactivates mutant p53 by targeting cysteines 124 and 277. Cell Death Dis (2018) 9:439. doi: 10.1038/S41419-018-0463-7
52. Cluzeau T, Sebert M, Rahmé R, Cuzzubbo S, Lehmann-Che J, Madelaine I, et al. Eprenetapopt plus azacitidine in TP53-mutated myelodysplastic syndromes and acute myeloid Leukemia: A phase II study by the groupe francophone des Myélodysplasies (GFM). J Clin Oncol (2021) 39:1575–83. doi: 10.1200/JCO.20.02342
53. Sallman DA, DeZern AE, Garcia-Manero G, Steensma DP, Roboz GJ, Sekeres MA, et al. Eprenetapopt (APR-246) and azacitidine in TP53-mutant myelodysplastic syndromes. J Clin Oncol (2021) 39:1584–94. doi: 10.1200/JCO.20.02341
54. Aprea Therapeutics. Aprea therapeutics announces results of primary endpoint from phase 3 trial of eprenetapopt in TP53 mutant myelodysplastic syndromes (MDS). Aprea Ther (2020).
55. Sallman DA, Al Malki MM, Asch AS, Wang ES, Jurcic JG, Bradley TJ, et al. Magrolimab in combination with azacitidine for untreated higher-risk myelodysplastic syndromes (HR-MDS): 5F9005 phase 1b study results. J Clin Oncol (2022) 40:7017. doi: 10.1200/JCO.2022.40.16_SUPPL.7017
56. Dombret H, Seymour JF, Butrym A, Wierzbowska A, Selleslag D, Jang JH, et al. International phase 3 study of azacitidine vs conventional care regimens in older patients with newly diagnosed AML with >30% blasts. Blood (2015) 126:291–9. doi: 10.1182/BLOOD-2015-01-621664
57. Wang ES, Montesinos P, Minden MD, Lee J-H, Heuser M, Naoe T, et al. Phase 3 trial of gilteritinib plus azacitidine vs azacitidine for newly diagnosed FLT3mut+ AML ineligible for intensive chemotherapy. Blood (2022) 140:1845–57. doi: 10.1182/blood.2021014586
58. Montesinos P, Recher C, Vives S, Zarzycka E, Wang J, Bertani G, et al. Ivosidenib and azacitidine in IDH1-mutated acute myeloid leukemia. N Engl J Med (2022) 386:1519–31. doi: 10.1056/NEJMoa2117344
59. Kantarjian HM, Thomas XG, Dmoszynska A, Wierzbowska A, Mazur G, Mayer J, et al. Multicenter, randomized, open-label, phase III trial of decitabine versus patient choice, with physician advice, of either supportive care or low-dose cytarabine for the treatment of older patients with newly diagnosed acute myeloid leukemia. J Clin Oncol (2012) 30:2670–7. doi: 10.1200/JCO.2011.38.9429
60. Wei AH, Döhner H, Pocock C, Montesinos P, Afanasyev B, Dombret H, et al. The QUAZAR AML-001 maintenance trial: results of a phase III international, randomized, double-blind, placebo-controlled study of CC-486 (Oral formulation of azacitidine) in patients with acute myeloid leukemia (AML) in first remission. Blood (2019) 134:LBA–3. doi: 10.1182/BLOOD-2019-132405
61. Craddock CF, Houlton AE, Quek LS, Ferguson P, Gbandi E, Roberts C, et al. Outcome of Azacitidine Therapy in Acute Myeloid Leukemia Is not Improved by Concurrent Vorinostat Therapy but Is Predicted by a Diagnostic Molecular Signature. Clin Cancer Res (2017) 23:6430–40. doi: 10.1158/1078-0432.CCR-17-1423
62. Garcia-Manero G, Sekeres MA, Egyed M, Breccia M, Graux C, Cavenagh JD, et al. A phase 1b/2b multicenter study of oral panobinostat plus azacitidine in adults with MDS, CMML or AML with ≦̸30% blasts. Leukemia (2017) 31:2799–806. doi: 10.1038/LEU.2017.159
63. Garcia-Manero G, Abaza Y, Takahashi K, Medeiros BC, Arellano M, Khaled SK, et al. Pracinostat plus azacitidine in older patients with newly diagnosed acute myeloid leukemia: results of a phase 2 study. Blood Adv (2019) 3:508–18. doi: 10.1182/BLOODADVANCES.2018027409
64. Stein EM, Garcia-Manero G, Rizzieri DA, Tibes R, Berdeja JG, Savona MR, et al. The DOT1L inhibitor pinometostat reduces H3K79 methylation and has modest clinical activity in adult acute leukemia. Blood (2018) 131:2662–9. doi: 10.1182/BLOOD-2017-12-818948
65. Patel MR, Garcia-Manero G, Paquette R, Dinner S, Donnellan WB, Grunwald MR, et al. Phase 1 dose escalation and expansion study to determine safety, tolerability, pharmacokinetics, and pharmacodynamics of the BET inhibitor FT-1101 as a single agent in patients with relapsed or refractory hematologic malignancies. Blood (2019) 134:3907–7. doi: 10.1182/BLOOD-2019-124741
66. Maes T, Mascaró C, Tirapu I, Estiarte A, Ciceri F, Lunardi S, et al. ORY-1001, a potent and selective covalent KDM1A inhibitor, for the treatment of acute leukemia. Cancer Cell (2018) 33:495–511.e12. doi: 10.1016/J.CCELL.2018.02.002
67. Salamero O, Montesinos P, Willekens C, Pérez-Simón JA, Pigneux A, Récher C, et al. First-in-human phase I study of iadademstat (ORY-1001): A first-in-class lysine-specific histone demethylase 1A inhibitor, in relapsed or refractory acute myeloid leukemia. J Clin Oncol (2020) 38:4260–73. doi: 10.1200/JCO.19.03250
68. Salamero O, Somervaille TC, Molero A, Acuña-Cruz E, Perez-Simon JA, Coll R, et al. Iadademstat combination with azacitidine is a safe and effective treatment in first line acute myeloid leukemia. Final results of the alice trial. Blood (2022) 140:1711–3. doi: 10.1182/BLOOD-2022-168945
69. Fiedler W, Kayser S, Kebenko M, Janning M, Krauter J, Schittenhelm M, et al. A phase I/II study of sunitinib and intensive chemotherapy in patients over 60 years of age with acute myeloid leukaemia and activating FLT3 mutations. Br J Haematol (2015) 169:694–700. doi: 10.1111/BJH.13353
70. Röllig C, Serve H, Hüttmann A, Noppeney R, Müller-Tidow C, Krug U, et al. Addition of sorafenib versus placebo to standard therapy in patients aged 60 years or younger with newly diagnosed acute myeloid leukaemia (SORAML): a multicentre, phase 2, randomised controlled trial. Lancet Oncol (2015) 16:1691–9. doi: 10.1016/S1470-2045(15)00362-9
71. Ravandi F, Arana Yi C, Cortes JE, Levis M, Faderl S, Garcia-Manero G, et al. Final Report of Phase II Study of Sorafenib, Cytarabine, and Idarubicin for Initial Therapy in Younger Patients with Acute Myeloid Leukemia. Leukemia (2014) 28:1543. doi: 10.1038/LEU.2014.54
72. Ravandi F, Alattar ML, Grunwald MR, Rudek MA, Rajkhowa T, Richie MA, et al. Phase 2 study of azacytidine plus sorafenib in patients with acute myeloid leukemia and FLT-3 internal tandem duplication mutation. Blood (2013) 121:4655–62. doi: 10.1182/BLOOD-2013-01-480228
73. Cortes J, Perl AE, Döhner H, Kantarjian H, Martinelli G, Kovacsovics T, et al. Quizartinib, an FLT3 inhibitor, as monotherapy in patients with relapsed or refractory acute myeloid leukaemia: an open-label, multicentre, single-arm, phase 2 trial. Lancet Oncol (2018b) 19:889–903. doi: 10.1016/S1470-2045(18)30240-7
74. Cortes JE, Tallman MS, Schiller GJ, Trone D, Gammon G, Goldberg SL, et al. Phase 2b study of 2 dosing regimens of quizartinib monotherapy in FLT3-ITD-mutated, relapsed or refractory AML. Blood (2018a) 132:598–607. doi: 10.1182/BLOOD-2018-01-821629
75. Cortes JE, Khaled S, Martinelli G, Perl AE, Ganguly S, Russell N, et al. Quizartinib versus salvage chemotherapy in relapsed or refractory FLT3-ITD acute myeloid leukaemia (QuANTUM-R): a multicentre, randomised, controlled, open-label, phase 3 trial. Lancet Oncol (2019) 20:984–97. doi: 10.1016/S1470-2045(19)30150-0
76. Short NJ, Kantarjian H, Ravandi F, Daver N. Emerging treatment paradigms with FLT3 inhibitors in acute myeloid leukemia. Ther Adv Hematol (2019) 10:204062071982731. doi: 10.1177/2040620719827310
77. Wu M, Li C, Zhu X. FLT3 inhibitors in acute myeloid leukemia. J Hematol Oncol (2018) 11:1–11. doi: 10.1186/S13045-018-0675-4/TABLES/6
78. McCarthy JJ, McLeod HL, Ginsburg GS. Genomic medicine: a decade of successes, challenges, and opportunities. Sci Transl Med (2013) 5:189sr4. doi: 10.1126/SCITRANSLMED.3005785
79. Lv JW, Li JY, Luo LN, Wang ZX, Chen YP. Comparative safety and efficacy of anti-PD-1 monotherapy, chemotherapy alone, and their combination therapy in advanced nasopharyngeal carcinoma: Findings from recent advances in landmark trials. J Immunother Cancer (2019) 7:1–9. doi: 10.1186/S40425-019-0636-7/TABLES/2
80. Ayoub NM. Editorial: novel combination therapies for the treatment of solid cancers. Front Oncol (2021) 11:708943/BIBTEX. doi: 10.3389/FONC.2021.708943/BIBTEX
81. Bonter K, Desjardins C, Currier N, Pun J, Ashbury FD. Personalised medicine in Canada: a survey of adoption and practice in oncology, cardiology and family medicine. BMJ Open (2011) 1:e000110. doi: 10.1136/BMJOPEN-2011-000110
82. Erdmann A, Rehmann-Sutter C, Bozzaro C. Patients’ and professionals’ views related to ethical issues in precision medicine: a mixed research synthesis. BMC Med Ethics (2021) 22:116. doi: 10.1186/S12910-021-00682-8
83. McGraw SA, Garber J, Jänne PA, Lindeman N, Oliver N, Sholl LM, et al. The fuzzy world of precision medicine: deliberations of a precision medicine tumor board. Per Med (2017) 14:37. doi: 10.2217/PME-2016-0074
84. McCarthy MC, De Abreu Lourenco R, McMillan LJ, Meshcheriakova E, Cao A, Gillam L. Finding out what matters in decision-making related to genomics and personalized medicine in pediatric oncology: developing attributes to include in a discrete choice experiment. Patient (2020) 13:347–61. doi: 10.1007/S40271-020-00411-0
85. McGill BC, Wakefield CE, Hetherington K, Munro LJ, Warby M, Lau L, et al. “Balancing expectations with actual realities”: conversations with clinicians and scientists in the first year of a high-risk childhood cancer precision medicine trial. J Pers Med (2020) 10:9. doi: 10.3390/JPM10010009
86. Seoane J, De Mattos-Arruda L. The challenge of intratumour heterogeneity in precision medicine. J Intern Med (2014) 276:41–51. doi: 10.1111/JOIM.12240
87. Pectasides E, Stachler MD, Derks S, Liu Y, Maron S, Islam M, et al. Genomic heterogeneity as a barrier to precision medicine in gastroesophageal adenocarcinoma. Cancer Discovery (2018) 8:37–48. doi: 10.1158/2159-8290.CD-17-0395
88. Di Nardo CD, Cortes JE. Mutations in AML: prognostic and therapeutic implications. Hematol Am Soc Hematol Educ Progr (2016) 2016:348. doi: 10.1182/ASHEDUCATION-2016.1.348
89. Wang S, Wu Z, Li T, Li Y, Wang W, Hao Q, et al. Mutational spectrum and prognosis in NRAS-mutated acute myeloid leukemia. Sci Rep (2020) 10:1–9. doi: 10.1038/s41598-020-69194-6
90. Yu J, Li Y, Zhang D, Wan D, Jiang Z. Clinical implications of recurrent gene mutations in acute myeloid leukemia. Exp Hematol Oncol (2020) 9:1–11. doi: 10.1186/S40164-020-00161-7
91. Sasaki K, Kanagal-Shamanna R, Montalban-Bravo G, Assi R, Jabbour E, Ravandi F, et al. Impact of the variant allele frequency of ASXL1, DNMT3A, JAK2, TET2, TP53, and NPM1 on the outcomes of patients with newly diagnosed acute myeloid leukemia. Cancer (2020) 126:765–74. doi: 10.1002/CNCR.32566
92. Sekeres MA, Elson P, Kalaycio ME, Advani AS, Copelan EA, Faderl S, et al. Time from diagnosis to treatment initiation predicts survival in younger, but not older, acute myeloid leukemia patients. Blood (2009) 113:28–36. doi: 10.1182/BLOOD-2008-05-157065
93. Röllig C, Kramer M, Schliemann C, Mikesch JH, Steffen B, Krämer A, et al. Does time from diagnosis to treatment affect the prognosis of patients with newly diagnosed acute myeloid leukemia? Blood (2020) 136:823–30. doi: 10.1182/BLOOD.2019004583
94. Hackl H, Astanina K, Wieser R. Molecular and genetic alterations associated with therapy resistance and relapse of acute myeloid leukemia. J Hematol Oncol (2017) 10:51. doi: 10.1186/s13045-017-0416-0
95. Letai A, Bhola P, Welm AL. Functional precision oncology: Testing tumors with drugs to identify vulnerabilities and novel combinations. Cancer Cell (2022) 40:26–35. doi: 10.1016/J.CCELL.2021.12.004
96. Hanahan D, Weinberg RA. Hallmarks of cancer: The next generation. Cell (2011) 144:646–74. doi: 10.1016/J.CELL.2011.02.013/ATTACHMENT/3F528E16-8B3C-4D8D-8DE5-43E0C98D8475/MMC1.PDF
97. Certo M, Del Gaizo Moore V, Nishino M, Wei G, Korsmeyer S, Armstrong SA, et al. Mitochondria primed by death signals determine cellular addiction to antiapoptotic BCL-2 family members. Cancer Cell (2006) 9:351–65. doi: 10.1016/j.ccr.2006.03.027
98. Del Gaizo Moore V, Letai A. BH3 profiling – measuring integrated function of the mitochondrial apoptotic pathway to predict cell fate decisions. Cancer Lett (2013) 332:202. doi: 10.1016/J.CANLET.2011.12.021
99. Adams JM, Cory S. The BCL-2 arbiters of apoptosis and their growing role as cancer targets. Cell Death Differ (2018) 25:27–36. doi: 10.1038/CDD.2017.161
100. Vaux DL, Cory S, Adams JM. Bcl-2 gene promotes haemopoietic cell survival and cooperates with c-myc to immortalize pre-B cells. Nature (1988) 335:440–2. doi: 10.1038/335440A0
101. Campos L, Rouault JP, Sabido O, Oriol P, Roubi N, Vasselon C, et al. High expression of bcl-2 protein in acute myeloid leukemia cells is associated with poor response to chemotherapy. Blood (1993) 81:3091–6. doi: 10.1182/BLOOD.V81.11.3091.3091
102. Moore VDG, Brown JR, Certo M, Love TM, Novina CD, Letai A. Chronic lymphocytic leukemia requires BCL2 to sequester prodeath BIM, explaining sensitivity to BCL2 antagonist ABT-737. J Clin Invest (2007) 117:112–21. doi: 10.1172/JCI28281
103. Oltersdorf T, Elmore SW, Shoemaker AR, Armstrong RC, Augeri DJ, Belli BA, et al. An inhibitor of Bcl-2 family proteins induces regression of solid tumours. Nature (2005) 435:677–81. doi: 10.1038/NATURE03579
104. Mason KD, Carpinelli MR, Fletcher JI, Collinge JE, Hilton AA, Ellis S, et al. Programmed anuclear cell death delimits platelet life span. Cell (2007) 128:1173–86. doi: 10.1016/J.CELL.2007.01.037/ATTACHMENT/7A3B191E-1518-4224-AFE4-8A851E3D5DD0/MMC1.PDF
105. Park CM, Oie T, Petros AM, Zhang H, Nimmer PM, Henry RF, et al. Design, synthesis, and computational studies of inhibitors of Bcl-X L. J Am Chem Soc (2006) 128:16206–12. doi: 10.1021/JA0650347/SUPPL_FILE/JA0650347SI20061006_051355.PDF
106. Petros AM, Dinges J, Augeri DJ, Baumeister SA, Betebenner DA, Bures MG, et al. Discovery of a potent inhibitor of the antiapoptotic protein Bcl-X L from NMR and parallel synthesis. J Med Chem (2006) 49:656–63. doi: 10.1021/JM0507532/SUPPL_FILE/JM0507532SI20051031_033429.PDF
107. Bruncko M, Oost TK, Belli BA, Ding H, Joseph MK, Kunzer A, et al. Studies leading to potent, dual inhibitors of Bcl-2 and Bcl-xL. J Med Chem (2007) 50:641–62. doi: 10.1021/JM061152T
108. Souers AJ, Leverson JD, Boghaert ER, Ackler SL, Catron ND, Chen J, et al. ABT-199, a potent and selective BCL-2 inhibitor, achieves antitumor activity while sparing platelets. Nat Med (2013) 19:202–8. doi: 10.1038/nm.3048
109. Anderson MA, Deng J, Seymour JF, Tam C, Kim SY, Fein J, et al. The BCL2 selective inhibitor venetoclax induces rapid onset apoptosis of CLL cells in patients via a TP53-independent mechanism. Blood (2016) 127:3215–24. doi: 10.1182/BLOOD-2016-01-688796
110. Pan R, Hogdal LJ, Benito JM, Bucci D, Han L, Borthakur G, et al. Selective BCL-2 inhibition by ABT-199 causes on-target cell death in acute myeloid leukemia. Cancer Discovery (2014) 4:362–75. doi: 10.1158/2159-8290.CD-13-0609
111. Konopleva M, Pollyea DA, Potluri J, Chyla B, Hogdal L, Busman T, et al. Efficacy and biological correlates of response in a phase II study of venetoclax monotherapy in patients with acute myelogenous leukemia. Cancer Discovery (2016) 6:1106–17. doi: 10.1158/2159-8290.CD-16-0313
112. Wei AH, Strickland SA, Hou JZ, Fiedler W, Lin TL, Walter RB, et al. Venetoclax combined with low-dose cytarabine for previously untreated patients with acute myeloid leukemia: results from a phase ib/II study. J Clin Oncol (2019) 37:1277–84. doi: 10.1200/JCO.18.01600
113. DiNardo CD, Pratz KW, Letai A, Jonas BA, Wei AH, Thirman M, et al. Safety and preliminary efficacy of venetoclax with decitabine or azacitidine in elderly patients with previously untreated acute myeloid leukaemia: a non-randomised, open-label, phase 1b study. Lancet Oncol (2018) 19:216–28. doi: 10.1016/S1470-2045(18)30010-X
114. DiNardo CD, Pratz K, Pullarkat V, Jonas BA, Arellano M, Becker PS, et al. Venetoclax combined with decitabine or azacitidine in treatment-naive, elderly patients with acute myeloid leukemia. Blood (2019) 133:7–17. doi: 10.1182/BLOOD-2018-08-868752
115. Daver N, Pollyea DA, Yee KWL, Fenaux P, Brandwein JM, Vey N, et al. Preliminary results from a phase ib study evaluating BCL-2 inhibitor venetoclax in combination with MEK inhibitor cobimetinib or MDM2 inhibitor idasanutlin in patients with relapsed or refractory (R/R) AML. Blood (2017) 130:813–3. doi: 10.1182/BLOOD.V130.SUPPL_1.813.813
116. Perl AE, Daver NG, Pratz KW, Maly J, Hong W-J, Bahceci E, et al. Venetoclax in combination with gilteritinib in patients with relapsed/refractory acute myeloid leukemia: A phase 1b study. Blood (2019) 134:3910–0. doi: 10.1182/BLOOD-2019-127416
117. Han L, Zhang Q, Dail M, Shi C, Cavazos A, Ruvolo VR, et al. Concomitant targeting of BCL2 with venetoclax and MAPK signaling with cobimetinib in acute myeloid leukemia models. Haematologica (2020) 105:697–707. doi: 10.3324/HAEMATOL.2018.205534
118. Lachowiez CA, Borthakur G, Loghavi S, Zeng Z, Kadia TM, Masarova L, et al. A phase Ib/II study of ivosidenib with venetoclax +/- azacitidine in IDH1-mutated myeloid malignancies. Blood Cancer Discov (2023) 4(4):276–93. doi: 10.1200/JCO.2021.39.15_SUPPL.7012
119. Stevens BM, Jones CL, Pollyea DA, Culp-Hill R, D’Alessandro A, Winters A, et al. Fatty acid metabolism underlies venetoclax resistance in acute myeloid leukemia stem cells. Nat Cancer (2020) 1:1176–87. doi: 10.1038/s43018-020-00126-z
120. Pei S, Pollyea DA, Gustafson A, Stevens BM, Minhajuddin M, Fu R, et al. Monocytic subclones confer resistance to venetoclax-based therapy in patients with acute myeloid leukemia. Cancer Discovery (2020) 10:536–51. doi: 10.1158/2159-8290.CD-19-0710/333433/AM/MONOCYTIC-SUBCLONES-CONFER-RESISTANCE-TO
121. Ahmed F, Allehyani OA, Alfayez M, Schulten H-J, Alkhattabi H, Chaudhary AGA, et al. Novel genetic mechanism of venetoclax resistance in AML: BAX deletion. Blood (2019) 134:5057–7. doi: 10.1182/BLOOD-2019-131637
122. Bhatt S, Pioso MS, Olesinski EA, Yilma B, Ryan JA, Mashaka T, et al. Reduced mitochondrial apoptotic priming drives resistance to BH3 mimetics in acute myeloid leukemia. Cancer Cell (2020) 38:872. doi: 10.1016/J.CCELL.2020.10.010
123. Tao ZF, Hasvold L, Wang L, Wang X, Petros AM, Park CH, et al. Discovery of a potent and selective BCL-XL inhibitor with in vivo activity. ACS Med Chem Lett (2014) 5:1088. doi: 10.1021/ML5001867
124. Caenepeel S, Brown SP, Belmontes B, Moody G, Keegan KS, Chui D, et al. AMG 176, a selective MCL1 inhibitor, is effective in hematologic cancer models alone and in combination with established therapies. Cancer Discovery (2018) 8:1582–97. doi: 10.1158/2159-8290.CD-18-0387
125. Tron AE, Belmonte MA, Adam A, Aquila BM, Boise LH, Chiarparin E, et al. Discovery of Mcl-1-specific inhibitor AZD5991 and preclinical activity in multiple myeloma and acute myeloid leukemia. Nat Commun (2018) 9:5341. doi: 10.1038/S41467-018-07551-W
126. Szlávik Z, Ondi L, Csékei M, Paczal A, Szabó ZB, Radics G, et al. Structure-guided discovery of a selective mcl-1 inhibitor with cellular activity. J Med Chem (2019) 62:6913–24. doi: 10.1021/ACS.JMEDCHEM.9B00134
127. Wang L, Doherty GA, Judd AS, Tao ZF, Hansen TM, Frey RR, et al. Discovery of A-1331852, a first-in-class, potent, and orally-bioavailable BCL-X L inhibitor. ACS Med Chem Lett (2020) 11:1829–36. doi: 10.1021/ACSMEDCHEMLETT.9B00568
128. Montero J, Sarosiek KA, Deangelo JD, Maertens O, Ryan J, Ercan D, et al. Drug-induced death signaling strategy rapidly predicts cancer response to chemotherapy. Cell (2015) 160:977. doi: 10.1016/J.CELL.2015.01.042
129. Bhola PD, Ahmed E, Guerriero JL, Sicinska E, Su E, Lavrova E, et al. High-throughput dynamic BH3 profiling may quickly and accurately predict effective therapies in solid tumors. Sci Signal (2020) 13. doi: 10.1126/SCISIGNAL.AAY1451
130. Daniels VW, Zoeller JJ, van Gastel N, McQueeney KE, Parvin S, Potter DS, et al. Metabolic perturbations sensitize triple-negative breast cancers to apoptosis induced by BH3 mimetics. Sci Signal (2021) 14:7405. doi: 10.1126/SCISIGNAL.ABC7405/SUPPL_FILE/SCISIGNAL.ABC7405_TABLES_S1_AND_S2.ZIP
131. Potter DS, Du R, Bhola P, Bueno R, Letai A. Dynamic BH3 profiling identifies active BH3 mimetic combinations in non-small cell lung cancer. Cell Death Dis (2021) 12:1–12. doi: 10.1038/s41419-021-04029-4
132. Alcon C, Martín F, Prada E, Mora J, Soriano A, Guillén G, et al. MEK and MCL-1 sequential inhibition synergize to enhance rhabdomyosarcoma treatment. Cell Death Discovery (2022) 8(1):1–12. doi: 10.1038/s41420-022-00959-w
133. Garcia JS, Bhatt S, Fell G, Sperling AS, Burgess M, Keshishian H, et al. Increased mitochondrial apoptotic priming with targeted therapy predicts clinical response to re-induction chemotherapy. Am J Hematol (2020) 95:245–50. doi: 10.1002/AJH.25692
134. Tyner JW, Yang WF, Bankhead A, Fan G, Fletcher LB, Bryant J, et al. Kinase pathway dependence in primary human leukemias determined by rapid inhibitor screening. Cancer Res (2013) 73:285. doi: 10.1158/0008-5472.CAN-12-1906
135. Rashid MBMA, Toh TB, Hooi L, Silva A, Zhang Y, Tan PF, et al. Optimizing drug combinations against multiple myeloma using a quadratic phenotypic optimization platform (QPOP). Sci Transl Med (2018) 10:eaan0941. doi: 10.1126/SCITRANSLMED.AAN0941
136. Gocho Y, Liu J, Hu J, Yang W, Dharia NV, Zhang J, et al. Network-based systems pharmacology reveals heterogeneity in LCK and BCL2 signaling and therapeutic sensitivity of T-cell acute lymphoblastic leukemia. Nat Cancer (2021) 2:284. doi: 10.1038/S43018-020-00167-4
137. Kornauth C, Pemovska T, Vladimer GI, Bayer G, Bergmann M, Eder S, et al. Functional precision medicine provides clinical benefit in advanced aggressive hematologic cancers and identifies exceptional responders. Cancer Discovery (2022) 12:372–87. doi: 10.1158/2159-8290.CD-21-0538/673876/AM/FUNCTIONAL-PRECISION-MEDICINE-PROVIDES-CLINICAL
138. Malani D, Kumar A, Brück O, Kontro M, Yadav B, Hellesøy M, et al. Implementing a functional precision medicine tumor board for acute myeloid leukemia. Cancer Discovery (2022) 12:388–401. doi: 10.1158/2159-8290.CD-21-0410/673825/AM/IMPLEMENTING-A-FUNCTIONAL-PRECISION-MEDICINE-TUMOR
139. Kurtz SE, Eide CA, Kaempf A, Khanna V, Savage SL, Rofelty A, et al. Molecularly targeted drug combinations demonstrate selective effectiveness for myeloid- and lymphoid-derived hematologic malignancies. Proc Natl Acad Sci USA (2017) 114:E7554–E7563. doi: 10.1073/PNAS.1703094114/SUPPL_FILE/PNAS.1703094114.SD08.XLSX
140. Bottomly D, Long N, Schultz AR, Kurtz SE, Tognon CE, Johnson K, et al. Integrative analysis of drug response and clinical outcome in acute myeloid leukemia. Cancer Cell (2022) 40:850–864.e9. doi: 10.1016/J.CCELL.2022.07.002
141. Eide CA, Kurtz SE, Kaempf A, Long N, Joshi SK, Nechiporuk T, et al. Clinical correlates of venetoclax-based combination sensitivities to augment acute myeloid leukemia therapy. Blood Cancer Discovery (2023) 4:452–67. doi: 10.1158/2643-3230.BCD-23-0014
142. Lee SHR, Yang W, Gocho Y, John A, Rowland L, Smart B, et al. Pharmacotypes across the genomic landscape of pediatric acute lymphoblastic leukemia and impact on treatment response. Nat Med (2023) 29:170–9. doi: 10.1038/s41591-022-02112-7
143. Liebers N, Bruch PM, Terzer T, Hernandez-Hernandez M, Paramasivam N, Fitzgerald D, et al. Ex vivo drug response profiling for response and outcome prediction in hematologic Malignancies: the prospective non-interventional SMARTrial. Nat Cancer (2023) 4:1648–59. doi: 10.1038/s43018-023-00645-5
144. Kuusanmäki H, Kytölä S, Vänttinen I, Ruokoranta T, Ranta A, Huuhtanen J, et al. Ex vivo venetoclax sensitivity testing predicts treatment response in acute myeloid leukemia. Haematologica (2023) 108:1768–81. doi: 10.3324/HAEMATOL.2022.281692
145. Zhang H, Wilmot B, Bottomly D, Kurtz SE, Eide CA, Damnernsawad A, et al. Biomarkers predicting venetoclax sensitivity and strategies for venetoclax combination treatment. Blood (2018) 132:175. doi: 10.1182/blood-2018-175
146. Griffioen MS, de Leeuw DC, Janssen JJWM, Smit L. Targeting acute myeloid leukemia with venetoclax; biomarkers for sensitivity and rationale for venetoclax-based combination therapies. Cancers (Basel) (2022) 14:3456. doi: 10.3390/cancers14143456
147. Mosquera Orgueira A, Peleteiro Raíndo A, Cid López M, Antelo Rodríguez B, Díaz Arias JÁ, Ferreiro Ferro R, et al. Gene expression profiling identifies FLT3 mutation-like cases in wild-type FLT3 acute myeloid leukemia. PloS One (2021) 16:e0247093. doi: 10.1371/journal.pone.0247093
148. Saad JJ, Miettinen J, Tsallos D, Eldfors S, Kontro M, Wennerberg K, et al. Predictive response biomarkers for BET inhibitors in AML. Blood (2018) 132:2749. doi: 10.1182/blood-2018-99-118363
149. Vu TT, Stölzel F, Wang KW, Röllig C, Tursky ML, Molloy TJ, et al. miR-10a as a therapeutic target and predictive biomarker for MDM2 inhibition in acute myeloid leukemia. Leukemia (2021) 35:1933–48. doi: 10.1038/s41375-020-01095-z
150. Baghban R, Roshangar L, Jahanban-Esfahlan R, Seidi K, Ebrahimi-Kalan A, Jaymand M, et al. Tumor microenvironment complexity and therapeutic implications at a glance. Cell Commun Signal (2020) 18:59. doi: 10.1186/S12964-020-0530-4
151. Yoshida GJ. Applications of patient-derived tumor xenograft models and tumor organoids. J Hematol Oncol (2020) 13:1–16. doi: 10.1186/S13045-019-0829-Z
152. Choi Y, Lee S, Kim K, Kim SH, Chung YJ, Lee C. Studying cancer immunotherapy using patient-derived xenografts (PDXs) in humanized mice. Exp Mol Med (2018) 50:1–9. doi: 10.1038/S12276-018-0115-0
153. Correa Rojo A, Heylen D, Aerts J, Thas O, Hooyberghs J, Ertaylan G, et al. Towards building a quantitative proteomics toolbox in precision medicine: A mini-review. Front Physiol (2021) 12:723510/BIBTEX. doi: 10.3389/FPHYS.2021.723510/BIBTEX
154. Molendijk J, Parker BL. Proteome-wide systems genetics to identify functional regulators of complex traits. Cell Syst (2021) 12:5–22. doi: 10.1016/J.CELS.2020.10.005
155. Lilljebjörn H, Orsmark-Pietras C, Mitelman F, Hagström-Andersson A, Fioretos T. Transcriptomics paving the way for improved diagnostics and precision medicine of acute leukemia. Semin Cancer Biol (2021) 84:40–9. doi: 10.1016/J.SEMCANCER.2021.09.013
156. Mubarak G, Zahir FR. Recent major transcriptomics and epitranscriptomics contributions toward personalized and precision medicine. J Pers Med (2022) 12:199. doi: 10.3390/JPM12020199
157. Maiti A, DiNardo CD, Daver NG, Rausch CR, Ravandi F, Kadia TM, et al. Triplet therapy with venetoclax, FLT3 inhibitor and decitabine for FLT3-mutated acute myeloid leukemia. Blood Cancer J (2021) 11:25. doi: 10.1038/s41408-021-00410-w
158. Lee SI, Celik S, Logsdon BA, Lundberg SM, Martins TJ, Oehler VG, et al. A machine learning approach to integrate big data for precision medicine in acute myeloid leukemia. Nat Commun (2018) 9:1–13. doi: 10.1038/s41467-017-02465-5
Keywords: precision medicine, functional genomics, functional precision medicine, AML, precision oncology
Citation: Bhatia K, Sandhu V, Wong MH, Iyer P and Bhatt S (2024) Therapeutic biomarkers in acute myeloid leukemia: functional and genomic approaches. Front. Oncol. 14:1275251. doi: 10.3389/fonc.2024.1275251
Received: 09 August 2023; Accepted: 17 January 2024;
Published: 12 February 2024.
Edited by:
Yan-Lai Tang, First Affiliated Hospital of Sun Yat-sen University, ChinaReviewed by:
Anna M. Eiring, Texas Tech University Health Sciences Center El Paso, United StatesMagesh Muthu, Wayne State University, United States
Su-Peng Yeh, China Medical University Hospital, Taiwan
Copyright © 2024 Bhatia, Sandhu, Wong, Iyer and Bhatt. This is an open-access article distributed under the terms of the Creative Commons Attribution License (CC BY). The use, distribution or reproduction in other forums is permitted, provided the original author(s) and the copyright owner(s) are credited and that the original publication in this journal is cited, in accordance with accepted academic practice. No use, distribution or reproduction is permitted which does not comply with these terms.
*Correspondence: Prasad Iyer, cHJhc2FkLml5ZXJAc2luZ2hlYWx0aC5jb20uc2c=; Shruti Bhatt, c2hydXRpX2JoYXR0QG51cy5lZHUuc2c=
†These authors have contributed equally to this work