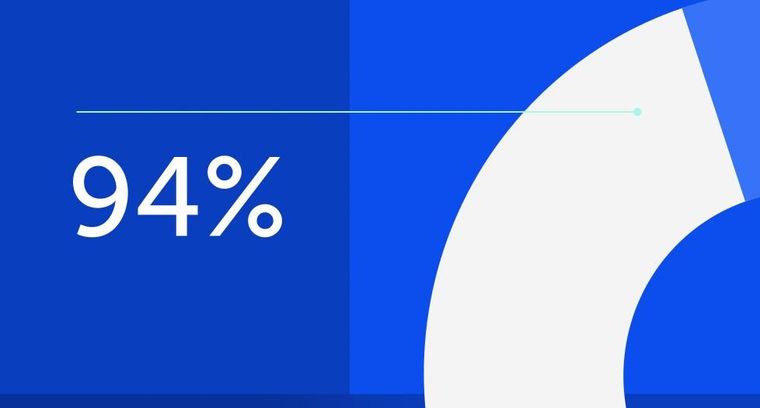
94% of researchers rate our articles as excellent or good
Learn more about the work of our research integrity team to safeguard the quality of each article we publish.
Find out more
REVIEW article
Front. Oncol., 06 December 2023
Sec. Hematologic Malignancies
Volume 13 - 2023 | https://doi.org/10.3389/fonc.2023.1330977
This article is part of the Research TopicReviews in Hematologic Malignancies: 2023View all 15 articles
Acute lymphoblastic leukemia (ALL) poses a significant health challenge, particularly in pediatric cases, requiring precise and rapid diagnostic approaches. This comprehensive review explores the transformative capacity of deep learning (DL) in enhancing ALL diagnosis and classification, focusing on bone marrow image analysis. Examining ten studies conducted between 2013 and 2023 across various countries, including India, China, KSA, and Mexico, the synthesis underscores the adaptability and proficiency of DL methodologies in detecting leukemia. Innovative DL models, notably Convolutional Neural Networks (CNNs) with Cat-Boosting, XG-Boosting, and Transfer Learning techniques, demonstrate notable approaches. Some models achieve outstanding accuracy, with one CNN reaching 100% in cancer cell classification. The incorporation of novel algorithms like Cat-Swarm Optimization and specialized CNN architectures contributes to superior classification accuracy. Performance metrics highlight these achievements, with models consistently outperforming traditional diagnostic methods. For instance, a CNN with Cat-Boosting attains 100% accuracy, while others hover around 99%, showcasing DL models’ robustness in ALL diagnosis. Despite acknowledged challenges, such as the need for larger and more diverse datasets, these findings underscore DL’s transformative potential in reshaping leukemia diagnostics. The high numerical accuracies accentuate a promising trajectory toward more efficient and accurate ALL diagnosis in clinical settings, prompting ongoing research to address challenges and refine DL models for optimal clinical integration.
Acute lymphoblastic leukemia (ALL) encompasses a range of lymphoid neoplasms that originate from precursor cells of both B-lineage and T-lineage cells (1). These neoplasms may primarily manifest as an extensive leukemic process involving both the bone marrow and peripheral blood, or they can display localized tissue infiltration with limited bone marrow involvement, termed lymphoblastic lymphoma (LBL) (2). Although ALL and LBLs exhibit distinct clinical features, they appear to represent a continuous biological spectrum. The current classification by the World Health Organization categorizes these conditions as B- or T-lymphoblastic leukemia/lymphoma (3). ALL is the most common pediatric malignancy, with pediatric ALL constituting approximately 80% of cases (4, 5). However, when it arises in adults, ALL takes on a particularly different clinical presentation. Notably, in the recent era of novel agents, not all cases of adult ALL have a poor prognosis; in fact, some individuals now experience good prognoses (6). In the United States, the estimated occurrence of ALL is about 1.6 cases per 100,000 individuals (7, 8). Research conducted among children has pinpointed genetic conditions that make a fraction of ALL cases more likely to occur including Down syndrome, Fanconi anemia, Bloom syndrome, and Ataxia Telangiectasia (9–11).
The initial phase of the diagnostic process for ALL, particularly to distinguish it from acute myeloid leukemia (AML) involves examining the bone marrow. This is crucial because ALL, as per its definition, invariably manifests with bone marrow participation (12, 13). Additional specialized tests are used to complement bone marrow evaluation such as peripheral blood smear (PBS) assessment and flowcytometric immunophenotyping (14, 15). However, bone marrow aspiration and biopsy remains the gold standard for ALL diagnostic confirmation, which provides a complete examination of cellular structure and appearance which could help indicate prognosis and evolution of the disease later on (16). While this approach allows for more precise classification and subtyping, it is an invasive process that can be painful, especially in pediatric patients, and getting appropriate samples can be difficult. Peripheral blood smears, on the other hand, require studying blood samples under a microscope to analyze blood cell morphology. Although they provide a rapid and non-invasive method of detecting blasts, their diagnostic depth may not be as extensive as bone marrow analysis.
Artificial intelligence (AI) and machine learning (ML) breakthroughs have sparked a revolution in medical image analysis and hematological diseases as previously explored by our group (17–21). Deep learning (DL) is a subset of ML that uses artificial neural networks to learn from data. Convolutional neural networks (CNNs) are one type of DL algorithm that has been particularly successful in image classification tasks (22). CNNs are designed to recognize patterns in images by using a series of convolutional layers that extract features from the input image. These features are then passed through a series of fully connected layers that classify the image based on the extracted features (22). CNNs have demonstrated exceptional ability in evaluating and interpreting medical images, including microscopic bone marrow images (23, 24).
In addition to the strides made in deep learning-based approaches, it is essential to acknowledge recent non-DL-based works that have contributed to the field of hematological disorder detection (25). Despite their contributions, these non-deep learning methods often face limitations in handling the complexity and variability present in hematological images. They may struggle to adapt to diverse morphologies and may require extensive manual tuning for optimal performance. DL methods, with their ability to automatically learn hierarchical features and patterns, offer a promising alternative that can potentially overcome some of these limitations, providing a more adaptive and robust solution for hematological disorder detection.
Although DL models in ALL diagnosis are widely studied, the focus has primarily been on peripheral blood smear (PBS) samples, neglecting the crucial bone marrow aspirates and biopsies, which are the gold standard for leukemia diagnosis. Recent reviews have also missed the majority of studies involving digital image analysis of microscopic bone marrow images (26, 27). Therefore, the goal of this review is to investigate the uses of DL in redefining ALL diagnosis and categorization using bone marrow images, possibly leading to the development of automated systems that assist healthcare personnel in making precise and timely ALL diagnoses. Performance metrics of several DL models and architectures in the detection and/or classification of ALL will be discussed. Furthermore, we will discuss the possible limitations and benefits of applying these models.
We developed our search strategy on the 11th of June 2023 in the PubMed/MEDLINE database. To ensure a broad search strategy, we used many terms, such as ‘acute lymphoblastic leukemia’, ‘acute lymphocytic leukemia’, ‘acute lymphoid leukemia’, ‘ALL’, ‘artificial intelligence’, ‘machine learning’, ‘deep learning’, and ‘neural network’. The search was not restricted by language or time frame. The developed search strategy was transferred to Scopus, Embase, and Web of Science databases using the Polyglot translator (28). The studies were then transferred to EndNote X9, where duplicates were detected and omitted.
The review will encompass studies that meet specific inclusion criteria: (1) utilization of human ALL samples, (2) publication in English, (3) employment of DL techniques for diagnosing/classifying ALL, (4) utilization of bone marrow samples, and (5) reporting of performance metrics. Studies not meeting these criteria will be excluded, ensuring a focused and relevant analysis.
After applying our search strategy to the mentioned databases, studies were transferred to EndNote X9, where duplicates were identified and removed. Remaining articles were uploaded to the Rayyan platform for additional screening (29). In Rayyan, titles and abstracts were screened for preliminary eligibility by two reviewers, and any discrepancies were settled by consensus. The whole texts of the papers that had been determined to be eligible were then acquired and independently double-screened for inclusion or exclusion using the mentioned criteria, with discrepancies resolved through screening by a third member if needed.
The data extraction process involved the extraction of pertinent information from included studies, comprising the last name of the primary author and the publication year, country of origin, dataset utilized, the targeted outcome under investigation, the applied validation methodologies, the employed models, and their corresponding performance metrics including accuracy, precision, sensitivity (recall), specificity, and F1 score. Furthermore, the strengths and limitations associated with each model were noted. Two investigators examined and obtained data from the eligible study independently. When they were unable to reach an agreement, they held a meeting with all team members. Only if it was agreed upon in the team meeting was the study included in the final review.
This review aims to provide an extensive examination of contemporary DL algorithms employed for the diagnosis and classification of ALL, with a specific emphasis on bone marrow samples. The principal objective entails a comprehensive evaluation of the performance of the diverse DL models featured in each study. Concurrently, a secondary aim involves the analysis of the relative merits and constraints of individual models in comparison to others.
The PRISMA flow diagram, shown in Figure 1, depicts the process of selecting studies for this review. Initially, our database search yielded 496 results, with an additional article found through manual extraction. After removing 282 duplicates with EndNote and Rayyan, we evaluated the remaining 215 items based on their titles and abstracts. Through this screening process, we excluded 195 articles not eligible for further screening and were left with 20 for full-text screening. We retrieved and examined the complete texts of the 20 studies and based on a variety of reasons listed in Figure 1, we eliminated 10 more articles. Ultimately, 10 studies were included in our review.
Table 1 presents the attributes and data gathered from the studies included in this analysis. It evaluates the effectiveness of deep learning models implemented for the diagnosis and categorization of ALL through bone marrow imagery. For a more comprehensive understanding, the specific metrics for accuracy (ACC), precision (PRE), sensitivity (SEN), specificity (SPE), and F1-score are provided for each model. In summary, the studies covered were published between 2013 and 2023, predominantly originating from India (n = 5), China (n = 3), KSA (n = 1), and Mexico (n = 1). Among these, five studies utilized the SN-AM dataset, comprising microscopic bone marrow aspirate images from patients diagnosed with B-cell ALL and Multiple Myeloma (MM) (40).
Notably, Yang et al. employed the SN-AM dataset in conjunction with the ALL-IDB1 database of peripheral blood smear images for external validation (38). Their model’s training and testing employed bone marrow samples from patients representing diverse leukemia families and subtypes. Zhou et al. introduced a novel “AI-cell platform” database for white blood cell (WBC) classification using bone marrow images, externally validating their model on authentic clinical samples of ALL and acute myeloid leukemia (AML) (39). The remaining four studies retrospectively sourced bone marrow aspirate images from hospital records. In terms of validation methods, only two studies conducted both external and internal validation, specifically utilizing a Train-Test Split approach. The remaining eight studies solely relied on internal validation via Train-Test Split (6) and k-fold cross-validation (2). Most of the studies employed CNNs as their classifier model, incorporating various layers, optimizations, and supplementary algorithms. The architecture of CNNs, as adapted from Kavitha et al, is depicted in Figure 2. A singular study deviated by utilizing radial basis function neural networks with a fuzzy logic algorithm in place of CNNs. The prevalence of CNNs, transfer learning, gradient boosting algorithms, and other elements typically associated with supervised learning tasks underscores these models’ intent for tasks involving labeled training data. Most models exhibited remarkable performance in their designated tasks. Notably, the models achieved high accuracy, with some reaching 100%, demonstrating the robustness of DL in ALL diagnosis.
Table 2 provides an overview of the strengths and limitations of each DL model discussed in the review. Each model’s outcomes, including feature selection, boosting algorithms, and optimized hyperparameters, are highlighted as strengths. However, limitations such as the lack of external validation, dependency on image quality, and computational complexity are also discussed. These strengths and limitations are crucial in evaluating the practical applicability and potential challenges associated with each DL model. For instance, the discussion on the lack of external validation emphasizes the need for further validation on diverse datasets to ensure the generalizability of the models. Moreover, the acknowledgment of computational complexities and interpretability issues provides insights into areas where improvements and future research could be directed.
Section 3.3 is a discussion of studies that focused on developing specialized CNN designs, including the integration of specific enhancements, additional layers, and boosting algorithms to increase classification accuracy. It will be divided into studies using the SN-AM dataset and studies using retrospectively collected hospital bone marrow samples.
B-ALL and MM are hematologic malignancies that arise from various stages of B-cell development. Despite their evident clinical and pathological distinctions, certain resemblances exist in their morphological attributes and molecular characteristics, rendering their differentiation challenging. This subsection focuses on a series of studies that employ the SN-AM dataset, containing bone marrow images from patients with B-ALL and MM, to develop specialized CNN designs for accurate classification. In the four studies, the model was evaluated using internal validation through train-test split, partitioning data into training, validation, and testing sets with no external validation.
The article by Devi et al. addresses the segmentation and classification of white blood cancer cells within bone marrow microscopic images. The research methodology starts with data preprocessing, effectively eliminating dataset anomalies. Following that, dataset diversity and comprehensiveness are augmented through data augmentation techniques. The proposed model then utilizes the Convolutional Leaky RELU with CatBoost and XGBoost (CLR-CXG) algorithm for image segmentation and feature extraction, which are key processes for accurate classification. Binary classification is executed through CNN, accompanied by gradient boosting using CatBoost and XGBoost algorithms individually. The interaction between CNN and boosting algorithms mainly occurs in the classification phase. The features extracted by the CNN are used as inputs to the boosting algorithms, which refine the classification decision. This combination allows for a more accurate and efficient classification of blood cancer cells. This CLR-CXG approach aims to minimize bias and amplify accuracy in cancer cell classification, primarily discerning between B-ALL and MM. Internal validation is achieved by partitioning the dataset into train, test, and validate sets. CNNs play a pivotal role in image classification, recognizing important features within images through weight and bias assignment. To address challenges like input-output consistency and GPU expenses, the CLR-CXG model introduces modifications into the CNN architecture. A novel element is the incorporation of the Leaky RELU activation function, elevating the architecture’s capabilities. The results impeccably show that CatBoost and XGBoost algorithms enhance accuracy and computational efficiency. The CLRC algorithm achieves an impressive 100% accuracy, precision, and specificity in cancer cell classification, complemented by a sensitivity (recall) of 99.9% and an F1 score of 100. Meanwhile, CLRXG attains 97.12% accuracy, alongside precision, sensitivity (recall), and specificity values of 98.5%, 99%, and 97.2%, correspondingly. Despite these achievements, the article has many limitations including absence of information regarding resource allocation, memory usage, and energy efficiency.
Moreover, the study by Ikechukwu et al. introduces a novel deep CNN model named “i-Net” for classification of ALL using microscopic images. The proposed approach utilizes data from the SN-AM and ALL-IDB datasets, both sourced from the cancer imaging archive (TCIA) repository. Initially, augmentation balanced limited data. Data preprocessing involved grayscale conversion, contrast enhancement, and resizing. For segmentation, they used a UNet model with InceptionV2 architecture, while a custom CNN was designed for image classification. The authors employed two well-known pre-trained deep learning networks, ResNet-50 and VGG-19. However, they adapted the weights and learning parameters instead of using pre-existing ones. An upgraded CNN model, “i-Net,” was introduced, adding convolutional layers and fine-tuning hyperparameters for better classification accuracy. To prevent overfitting during training, the authors used data augmentation, dropout regularization, and batch normalization techniques. The proposed “i-Net” achieved 99.18% accuracy on the SN-AM dataset, surpassing ResNet-50 (84.5%) and VGG-19 (93.5%). The model’s generalization was tested, highlighting its potential for clinical decision support systems. Despite limitations due to computational constraints and a smaller dataset, the proposed “i-Net” model outperformed established models, showing promise for clinical use.
Furthermore, Kavitha et al. introduce a groundbreaking methodology for the diagnosis and classification of bone marrow cancers, with a particular focus on ALL and MM. The proposed model employs optimized deep CNNs utilizing a novel CAT (Cat Swarm Optimization) algorithm for hyperparameter tuning (41). The process involves three essential phases: data preparation, data augmentation, and classification. The data preparation phase involves capturing microscopic images from bone marrow aspirate slides, which are stained using the Jenner-Giemsa method. These raw images are then pre-processed to create a dataset that is utilized for both training and testing purposes. Data augmentation techniques are employed to alleviate overfitting concerns and augment the model’s ability to generalize. The architecture of the CNN incorporates convolutional layers for feature extraction, pooling layers for dimension reduction, and fully connected layers for accurate classification. The introduction of the CAT algorithm further enhances the model’s overall performance by drawing inspiration from the behaviours of cats, combining seeking and tracing modes to effectively optimize the network’s parameters. The evaluation of the proposed approach is conducted using the SN-AM dataset. The results showcase remarkable achievements, with an outstanding accuracy of 99.6% attained in accurately predicting ALL. This performance surpasses that of pre-trained deep learning models, such as AlexNets, VGG-16 Nets, and U-Nets. The proposed model’s superiority is further substantiated through comprehensive comparisons with other machine learning methodologies, including support vector machines, random forest, and naïve bayes.
Lastly, the study conducted by Kumar et al. introduces a robust mechanism for classifying B-ALL and MM using CNNs. The study leverages deep learning techniques to automate the classification process, eliminating errors associated with manual assessment. The model is trained on cell images, undergoing preprocessing and feature extraction. It employs a dense convolutional neural network (DCNN) framework for classification, depicted in Figure 3, and achieved an impressive overall accuracy of 97.2%. Notably, the model demonstrates exceptional precision, sensitivity, specificity, and F1 score, with a precision of 100%, sensitivity of 93.97%, specificity of 95.19%, and an F1 score of 96.89%. The CNN architecture comprises convolution, max-pooling, and fully connected layers. Data augmentation techniques enhance generalization, while feature selection relies on the Chi-square test. Training utilizes an Adam optimizer with a sigmoid cross-entropy loss function and a learning rate of 0.01. Comparisons with machine learning methods and transferred learning models like VGG-16 were conducted. Random Forests achieved an accuracy of 96.83% on the dataset. However, the proposed CNN model significantly outperforms these approaches, boasting higher precision, sensitivity, specificity, and F1 score. Its capacity to extract features directly from images, coupled with adaptability across datasets, underscores its advantages. Although acknowledging limitations stemming from dataset size, the study underscores the potential of the proposed model as a reliable tool for diagnosing bone marrow blood cancers.
This subsection focuses on another set of studies that address the diagnosis of ALL through the analysis of retrospectively collected bone marrow samples from hospital patients. These studies emphasize the importance of accurate and efficient diagnosis for different leukemia subtypes.
The article by Duggal et al. presents an innovative convergence of deep learning techniques and stain deconvolution in the domain of medical image analysis. While CNNs have proven successful in medical imaging, the authors highlight a crucial limitation: CNNs primarily function in the RGB color space, potentially missing the nuanced tissue-stain interactions crucial for precise diagnostics. To address this, the study introduces the stain deconvolution layer (SD-Layer). Positioned at the forefront of CNN architectures, this layer operates in the optical density (OD) color space. Beer-Lambert’s law is employed to convert RGB microscopic images into the OD space, revealing pixel stain quantities that hold key diagnostic information. The SD-Layer operates on two fronts: converting RGB to OD space and using backpropagation to derive optimal stain basis vectors for diverse cell types. OD images are then deconvolved with these vectors, providing tissue-specific stain absorption quantities as input for downstream CNN layers. The study focuses on differentiating malignant WBCs from normal ones in cancer detection, particularly ALL. Texture-CNN and CNN (AlexNet) are evaluated using the SD-Layer in two modes: frozen (fixed stain vectors) and trainable (refined vectors). Impressively, the SD-Layer, initialized with stain basis vectors from SVD of the reference image, notably enhances classification accuracy for both architectures. This enhancement is attributed not to model capacity but to the biologically meaningful image representation the SD-Layer offers. With a well-structured dataset of around 9000 cell nuclei, balanced between normal and malignant cells and stained with Jenner-Giemsa, the study’s robustness is underscored. Rigorous training and augmentation techniques yield high performances on 5-fold cross-validation accuracy in distinguishing malignant from normal WBCs. The Texture-CNN achieves 93.20% accuracy and 93.08% F1 score with an additional SD-Layer, while CNN (AlexNet) achieves 88.5% accuracy and 88.32% F1 score with an additional SD-Layer. SD-Layer bridges RGB limitations, leveraging the OD space to capture crucial diagnostic insights. As medical imaging evolves, this study paves the way for harnessing stain quantities to enhance classification accuracy and diagnostic efficacy across diverse scenarios.
Moreover, Rehman et al., proposes a computer-aided system that combines image processing and deep learning to improve ALL diagnosis accuracy, depicted in Figure 4. The study focuses on classifying ALL into its subtypes and distinguishing reactive bone marrow (normal) using stained bone marrow images. The authors collect a dataset of bone marrow images from patients with ALL and normal cases. The images are captured using a digital microscope and processed to segment the regions of interest. A novel segmentation technique based on thresholding is introduced, followed by the application of CNNs for classification. The dataset is split into training and testing sets to train the CNN model. The researchers utilize the AlexNet architecture with transfer learning to fine-tune the model to the new data. To assess the effectiveness of their approach, the authors perform experiments and compare the results with other classification methods such as naïve Bayesian, K-nearest neighbor, and support vector machine. The proposed method achieves an impressive accuracy of 97.78% on the test dataset. The classification accuracy is plotted against the number of iterations, demonstrating that higher accuracy can be achieved with more epochs and a lower learning rate. The training time is also noted, with the proposed architecture taking approximately 163.63 seconds for 20 epochs. The authors highlight the significance of their work, as it provides an automated solution for accurate ALL diagnosis and classification. By employing DL techniques, the proposed system improves the accuracy of classification, which could significantly assist hematologists and pathologists in their diagnostic processes. Despite the promising results, this study does have limitations. The dataset size might impact the generalizability of the model, and external validation on larger datasets is necessary.
Lastly, Huang et al.’s study addresses leukemia classification and diagnosis through bone marrow cell morphology, employing CNNs alongside transfer learning. Traditional manual microscopy for leukemia diagnosis is subjective and error-prone, motivating an automated, precise approach. Their proposed method utilizes CNNs for identifying AML, ALL, and chronic myelocytic leukemia (CML). The researchers obtained microscopy images from healthy subjects and leukemia patients, implementing preprocessing techniques like perfect reflection and adaptive filtering to enhance quality and reduce background noise. They employed three CNN architectures for classification models on both raw and preprocessed datasets: Inception-V3, ResNet50, and DenseNet121. Transfer learning was leveraged to optimize model performance by extracting features or fine-tuning pre-trained models. In line with internal validation practices, the dataset is divided into a training set (991 samples) and a prediction set (331 samples) using a 3:1 ratio. The training set is utilized to train the models, while the prediction set serves as unseen data for testing the model’s generalization capability. DenseNet121 excelled among the CNN architectures, consistently achieving superior performance. Transfer learning notably expedited model convergence, significantly boosting accuracy. The study’s outcomes indicate that the DenseNet121 model on the preprocessed dataset garnered the highest accuracy at 74.8%. After transfer learning, its accuracy surged to 95.3%, a notable 20.5% improvement. The model exhibited accuracy rates of 90% for normal samples, 99% for ALL, 97% for CML, and 95% for AML, demonstrating efficacy in classifying various leukemia types. Nonetheless, the model faced challenges distinguishing immature granulocytes and lymphocytes, affecting AML classification accuracy. Its adaptability to rare leukemia types remains to be explored. Huang et al.’s study contributes a rapid, accurate, and objective method for leukemia diagnosis by merging CNNs with transfer learning. The combination overcomes the limitations of manual methods, catering to efficient, precise medical imaging despite small sample sizes. Though it confronts challenges, like distinguishing specific cell types, the study offers a promising path towards enhancing leukemia diagnosis and classification.
In this section, we explore studies that utilize ensemble techniques and hybrid approaches, combining multiple models to enhance diagnosis accuracy. The subsequent subsections present the findings from these studies and provide insights into their contributions to the field.
This subsection examines the work by Ordaz-Gutierrez et al., which introduces an algorithm for diagnosing ALL using a combination of robust fuzzy logic and radial basis function neural networks (RBFNN). The primary aim of this research is to develop a reliable method for diagnosing ALL, particularly in developing countries like Mexico, where laboratory resources and equipment might be limited. The algorithm leverages bone marrow aspirates to extract specific features related to the disease. The process begins with acquiring microscopic cell images, which are then converted to grayscale to eliminate unnecessary color information and reduce processing time. Histogram equalization enhances image contrast. The segmentation stage involves utilizing the Sobel edge detection and mathematical morphology algorithms to isolate cells from the images. Suitable mathematical expressions are utilized to analyze cell size, circularity, and nuclei-to-cytoplasm ratio, crucial for ALL diagnosis. The heart of the method lies in applying fuzzy logic, chosen for incorporating human knowledge and mathematical modeling. The algorithm determines if a cell has ALL based on computed features. Fuzzy membership values combine using algebraic expressions to generate a diagnosis variable that classifies cells. To enhance the algorithm, a radial basis function (RBF) neural network is introduced, improving classification accuracy. Trained on a dataset, the algorithm achieves high sensitivity (98.00%) and specificity (91.00%). The results of the proposed method are promising, outperforming comparative methods, showing superiority in detection rates. The potential for real-time diagnosis is highlighted due to efficient feature extraction and RBF’s computational speed.
Here, we delve into the article by Yang et al. which presents a deep learning-based approach for diagnosing leukemias using bone marrow aspirates. The study collected 2033 microscopic images of bone marrow samples, encompassing images for 6 disease types and 1 healthy control, from two Chinese medical websites. These images were divided into training, validation, and test datasets. To address variations in staining styles, a novel method called “stain domain augmentation” was introduced using the MultiPathGAN model. This technique normalized stain styles and expanded the dataset. A lightweight hybrid model named MobileViTv2, combining strengths of CNNs and vision transformers (ViTs), was developed for disease classification. MobileViTv2 achieved an average accuracy of 94.28% on the test set, with the highest accuracy values (98%, 96%, and 96%) obtained for MM, ALL, and lymphoma, respectively. Patient-level prediction accuracy averaged 96.72%. The model outperformed both CNNs and ViTs despite using only 9.8 million parameters. Furthermore, MobileViTv2 was compared to other deep learning models, demonstrating its superiority. The model’s effectiveness was also externally validated on public datasets (ALL-IDB1 and SN-AM), achieving high accuracy values of 99.75% and 99.72%, respectively. This indicates its robust generalization ability. While the model shows promise, there are some limitations. The dataset size is relatively small, and efforts to collect more images from various scanning devices could enhance its performance. Additionally, the model’s application is primarily focused on diagnosing broad disease categories, not specific subtypes. Future research could explore finer disease subtype classification and optimization of the model.
This subsection explores Zhou et al.’s study, which develops a deep learning-based system for leukemia diagnosis and evaluates it using real clinical scenarios. The subsection discusses the unique aspects of the system, its effectiveness in diagnosing different types of leukemia, and its practical utility in clinical settings. The researchers collected 1,732 bone marrow images, containing 27,184 cells, from children with leukemia in a dataset named “AI-cell platform”. This dataset was used to train a CNN architecture for the differential count of WBCs. Unlike prior approaches that preprocess images, this study used raw images without pre-processing. The developed system mimicked the process of hematologists by detecting and excluding uncountable and crushed cells, classifying remaining cells, and making diagnoses utilizing different configurations of ResNet and ResNeXt architectures for WBC detection and classification, as depicted in Figure 5. The ensemble of CNNs, comprising ResNeXt101_32x8d, ResNeXt50_32x4d, and ResNet50, emerged as the top-performing configuration. On internal validation using Train-Test Split, the ensemble model demonstrated very high performances for classifying WBCs (82.93% accuracy, 86.07% precision, and 82.02% F1 score). On external validation using real-world clinical samples of bone marrow, the system showed notable performance in diagnosing ALL (89% accuracy, 86% sensitivity, and 95% specificity). The validation results reveal significant insights into the ensemble’s performances and underscore its robustness and its potential for effectively diagnosing leukemia subtypes. Additionally, it accurately detected bone marrow metastasis of lymphoma and neuroblastoma (average accuracy of 82.93%). The system’s development was unique in using raw clinical images and replicating the hematologists’ workflow. The CNN differentiated crush cells, commonly excluded during manual counts, and demonstrated high accuracy across diverse WBC types. Furthermore, the system achieved successful ALL diagnosis in clinical practice, providing evidence of its practical utility. Comparison with existing studies revealed the uniqueness of this research in its broader variety of cell types, achieving high accuracy across complex clinical scenarios. Prior studies often focused on single-cell classification or employed pre-processed images, hindering real-world applications. While this study excelled in leukemia diagnosis, the limited dataset size for certain cell types posed challenges. However, the study’s innovative use of ensemble models mitigated this issue, enhancing overall accuracy.
Recent advancements in medical image analysis have yielded remarkable progress in the automated detection and classification of acute leukemia, a critical hematological malignancy. Deep learning techniques have emerged as pivotal tools, demonstrating the potential to revolutionize diagnostic accuracy and efficiency. Key studies have explored acute leukemia detection and classification intricacies, addressing essential elements such as datasets, validation methodologies, and performance metrics.
The existing literature on AI-based acute lymphoblastic leukemia (ALL) classification, as discussed in systematic reviews by Das et al. (42) and Mustaqim et al. (43), reveals notable limitations that our review seeks to address. While these reviews have explored recent advancements in AI-based ALL classification, they primarily emphasize studies and datasets focused on peripheral blood samples. Although peripheral blood samples provide valuable insights, the gold standard for leukemia diagnosis has long been bone marrow samples, given their ability to offer a more comprehensive understanding of the disease’s characteristics. Bone marrow samples are particularly crucial for accurately distinguishing different leukemia subtypes. By including studies that utilize bone marrow samples in the context of AI-based ALL classification, our review fills this crucial gap in the literature, contributing to a more holistic understanding of advancements in leukemia diagnosis and emphasizing the significance of bone marrow analysis in achieving accurate and reliable results. Additionally, Alsalem et al.’s (44) comprehensive review on automated acute leukemia detection and classification complexities, while valuable, predominantly focuses on studies applying artificial intelligence to peripheral blood smear (PBS) samples (45–48). Similarly, Deshpande et al. (49) adopt an AI-centric approach, enhancing diagnostic accuracy through microscopic blood cell analysis. While these approaches contribute significantly to the field, they underscore the need for a more inclusive examination of bone marrow samples, as we address in our review. Furthermore, the literature showcases the effectiveness of deep learning models in distinguishing acute leukemia subtypes, such as the work by Anilkumar et al. (50) on automated B cell and T cell acute lymphoblastic leukemia differentiation using blood smear samples and Boldú et al.’s (51) introduction of ALNet for effective diagnosis of acute leukemia lineages using peripheral blood cell images. Moreover, Ouyang et al.’s (52) proposal of a convolutional neural network-based acute promyelocytic leukemia diagnosis highlights the versatility of deep learning across various subtypes. Laosai & Chamnongthai’s innovative approach using CD markers of blood cells for automated acute leukemia classification (15) adds another dimension to the literature. Notably, the significance of well-annotated datasets, such as ALL-IDB, SN-AM, C-NMC, and SDCT-AuxNet, in standardizing algorithm evaluation is acknowledged in the literature (53, 54). Finally, the literature emphasizes the importance of integrating real-world clinical scenarios and transfer learning to improve model robustness (55, 56), aspects that our review aims to further elucidate and emphasize in the context of bone marrow samples.
Several studies have been dedicated to the development of specialized CNN architectures, aiming to enhance the accuracy of classification. Devi et al. introduced the CLR-CXG model, synergizing convolutional leaky rectified linear units (ReLU) with CatBoost and XGBoost boosting algorithms for cancer cell classification. This integration showcased promising results, underlining the potential of hybrid models. The “i-Net” model by Ikechukwu et al. effectively combined pre-trained deep learning networks, segmentation techniques, and data augmentation to achieve exceptional accuracy in segmenting and classifying acute lymphoblastic leukemia (ALL). Kavitha et al. contributed an optimized deep CNN architecture for diagnosing bone marrow cancers, leveraging the Cat Swarm optimization (CAT) algorithm for hyperparameter tuning. By focusing on precise segmentation and feature extraction using deep CNNs, this study demonstrated the power of specialized designs in achieving robust classification. Additionally, Kumar et al. emphasized the automatic detection of white blood cell cancers, specifically ALL and MM using CNNs. Their study highlighted the capacity of CNNs to discern pertinent features within images, effectively enhancing classification accuracy.
Hybrid and ensemble methodologies also emerged as valuable avenues for leukemia diagnosis. Ordaz-Gutierrez et al. introduced a practical hybrid approach, uniting the RBFNN with the fuzzy logic algorithm for ALL diagnosis. This study’s emphasis on resource-constrained settings underscores the importance of accessible and effective models. Yang et al. ventured into hybrid modeling by integrating CNNs with ViTs to diagnose hematologic malignancies through bone marrow images. By incorporating stain domain augmentation and hybrid modeling, this study showcased the potential of blending diverse deep learning techniques. Zhou et al. devised a deep learning-based system for leukemia diagnosis, employing an ensemble of multiple CNN models. The exceptional performance observed in classifying different white blood cell types and accurately diagnosing ALL in clinical scenarios exemplified the potential of ensemble techniques for practical medical applications.
The discussed studies offer a promising path for improving ALL diagnosis and classification through specialized CNN designs, hybrid models, and ensemble techniques. However, recognizing associated limitations is essential. One of the key limitations of the reviewed studies is the relatively small dataset sizes. While these studies demonstrate the potential of DL in ALL diagnosis, the limited data may raise concerns about the generalizability of the results. It is noteworthy that only two out of the ten reviewed studies employed external validation. This is a significant limitation, as relying solely on internal validation can lead to inflated performance metrics. To address these limitations, further efforts are required. This includes exploring larger datasets, refining segmentation techniques, and assessing clinical feasibility. It is imperative to develop a comprehensive evaluation framework that incorporates external validation and real-world clinical testing to enhance the robustness and generalizability of AI models for ALL diagnosis and classification. Moreover, considering the complexity of leukemia diagnosis, incorporating more complex samples, such as those with 10-15% blast in normal marrow, is vital for a thorough assessment of deep learning’s potential in distinguishing normal and malignant blasts.
Future research endeavors should consider the incorporation of molecular and genomic data into the analysis pipeline. Combining these data sources with image-based analyses can potentially provide a more holistic and accurate assessment of ALL cases. Furthermore, the development of AI models that can effectively integrate and interpret both image and molecular/genomic data represents an exciting avenue for future research in the field. By confronting limitations and pursuing the identified research agenda, the field can move into a new era of accurate, efficient, and accessible methods for diagnosing and classifying leukemia using bone marrow images. This advancement holds the potential to revolutionize clinical practices, enabling timely interventions and personalized treatment strategies. Moreover, establishing standardized protocols for external validation and benchmarking across different datasets and institutions will be instrumental in establishing the reliability and generalizability of deep learning models. Ultimately, as these future directions unfold, they will contribute to the ongoing refinement and deployment of AI-powered tools in the realm of ALL diagnosis and classification, improving patient outcomes and advancing the field of medical image analysis.
In conclusion, this review highlights the potential of deep learning models in enhancing acute lymphoblastic leukaemia diagnosis and classification. The proposed methodologies could revolutionize leukaemia diagnostics, providing accurate tools for early detection and treatment. Specialized CNN architectures, hybrid models, and ensemble techniques demonstrate the adaptability of deep learning in medical image analysis. However, limitations like small datasets and lack of external validation must be acknowledged. The reported high model performance metrics might be overestimated without robust validation. Future research should focus on refining and validating models, utilizing larger datasets, and conducting clinical feasibility studies. Collaborative efforts could integrate AI tools for precise leukaemia diagnosis, advancing patient care and reshaping medical imaging diagnostics.
BE: Conceptualization, Methodology, Project administration, Writing – original draft, Writing – review & editing. ME: Methodology, Writing – original draft, Writing – review & editing. RME: Methodology, Writing – original draft, Writing – review & editing. AE: Methodology, Writing – original draft, Writing – review & editing. AB: Validation, Writing – original draft, Writing – review & editing. OM: Validation, Writing – original draft, Writing – review & editing. RAE: Validation, Writing – original draft, Writing – review & editing. MS: Validation, Writing – original draft, Writing – review & editing. FK: Writing – original draft, Writing – review & editing. AA: Writing – original draft, Writing – review & editing. DM: Writing – original draft, Writing – review & editing. MY: Conceptualization, Project administration, Supervision, Writing – original draft, Writing – review & editing.
The author(s) declare financial support was received for the research, authorship, and/or publication of this article. The open access publication of this article was made possible due to a generous fund from QU Health, Qatar University.
The authors declare that the research was conducted in the absence of any commercial or financial relationships that could be construed as a potential conflict of interest.
The author(s) declared that they were an editorial board member of Frontiers, at the time of submission. This had no impact on the peer review process and the final decision.
All claims expressed in this article are solely those of the authors and do not necessarily represent those of their affiliated organizations, or those of the publisher, the editors and the reviewers. Any product that may be evaluated in this article, or claim that may be made by its manufacturer, is not guaranteed or endorsed by the publisher.
1. Onciu M. Acute lymphoblastic leukemia. Hematol Oncol Clin North Am (2009) 23(4):655–74. doi: 10.1016/j.hoc.2009.04.009
2. Jabbour E, O'Brien S, Konopleva M, Kantarjian H. New insights into the pathophysiology and therapy of adult acute lymphoblastic leukemia. Cancer (2015) 121(15):2517–28. doi: 10.1002/cncr.29383
3. Duffield AS, Mullighan CG, Borowitz MJ. International consensus classification of acute lymphoblastic leukemia/lymphoma. Virchows Arch (2023) 482(1):11–26. doi: 10.1007/s00428-022-03448-8
4. Ward E, DeSantis C, Robbins A, Kohler B, Jemal A. Childhood and adolescent cancer statistics, 2014. CA Cancer J Clin (2014) 64(2):83–103. doi: 10.3322/caac.21219
5. Bhojwani D, Yang JJ, Pui CH. Biology of childhood acute lymphoblastic leukemia. Pediatr Clin North Am (2015) 62(1):47–60. doi: 10.1016/j.pcl.2014.09.004
6. Larson RA. Managing cns disease in adults with acute lymphoblastic leukemia. Leuk Lymphoma (2018) 59(1):3–13. doi: 10.1080/10428194.2017.1326597
7. Jemal A, Ward EM, Johnson CJ, Cronin KA, Ma J, Ryerson B, et al. Annual report to the nation on the status of cancer, 1975-2014, featuring survival. J Natl Cancer Inst (2017) 109(9). doi: 10.1093/jnci/djx030
8. Terwilliger T, Abdul-Hay M. Acute lymphoblastic leukemia: A comprehensive review and 2017 update. Blood Cancer J (2017) 7(6):e577. doi: 10.1038/bcj.2017.53
9. Shah A, John BM, Sondhi V. Acute lymphoblastic leukemia with treatment–naive fanconi anemia. Indian Pediatr (2013) 50(5):508–10.
10. German J. Bloom's syndrome. Xx. The first 100 cancers. Cancer Genet Cytogenet (1997) 93(1):100–6. doi: 10.1016/s0165-4608(96)00336-6
11. Bielorai B, Fisher T, Waldman D, Lerenthal Y, Nissenkorn A, Tohami T, et al. Acute lymphoblastic leukemia in early childhood as the presenting sign of ataxia-telangiectasia variant. Pediatr Hematol Oncol (2013) 30(6):574–82. doi: 10.3109/08880018.2013.777949
12. Lai R, Hirsch-Ginsberg CF, Bueso-Ramos C. Pathologic diagnosis of acute lymphocytic leukemia. Hematol Oncol Clin North Am (2000) 14(6):1209–35. doi: 10.1016/s0889-8588(05)70183-0
13. Chiaretti S, Zini G, Bassan R. Diagnosis and subclassification of acute lymphoblastic leukemia. Mediterr J Hematol Infect Dis (2014) 6(1):e2014073. doi: 10.4084/MJHID.2014.073
14. Bene MC, Castoldi G, Knapp W, Ludwig WD, Matutes E, Orfao A, et al. Proposals for the immunological classification of acute leukemias. European group for the immunological characterization of leukemias (Egil). Leukemia (1995) 9(10):1783–6.
15. Dese K, Raj H, Ayana G, Yemane T, Adissu W, Krishnamoorthy J, et al. Accurate machine-learning-based classification of leukemia from blood smear images. Clin Lymphoma Myeloma Leuk (2021) 21(11):e903–e14. doi: 10.1016/j.clml.2021.06.025
16. Thomas X, Le QH, Danaila C, Lheritier V, Ffrench M. Bone marrow biopsy in adult acute lymphoblastic leukemia: morphological characteristics and contribution to the study of prognostic factors. Leuk Res (2002) 26(10):909–18. doi: 10.1016/s0145-2126(02)00034-6
17. Elsabagh AA, Elhadary M, Elsayed B, Elshoeibi AM, Ferih K, Kaddoura R, et al. Artificial intelligence in sickle disease. Blood Rev (2023) 61:101102. doi: 10.1016/j.blre.2023.101102
18. Ferih K, Elsayed B, Elshoeibi AM, Elsabagh AA, Elhadary M, Soliman A, et al. Applications of artificial intelligence in thalassemia: A comprehensive review. Diagnostics (Basel) (2023) 13(9):1551. doi: 10.3390/diagnostics13091551
19. Elhadary M, Elsabagh AA, Ferih K, Elsayed B, Elshoeibi AM, Kaddoura R, et al. Applications of machine learning in chronic myeloid leukemia. Diagnostics (Basel) (2023) 13(7):1330. doi: 10.3390/diagnostics13071330
20. Elsayed B, Elshoeibi AM, Elhadary M, Ferih K, Elsabagh AA, Rahhal A, et al. Applications of artificial intelligence in philadelphia-negative myeloproliferative neoplasms. Diagnostics (Basel) (2023) 13(6):1123. doi: 10.3390/diagnostics13061123
21. Elshoeibi AM, Ferih K, Elsabagh AA, Elsayed B, Elhadary M, Marashi M, et al. Applications of artificial intelligence in thrombocytopenia. Diagnostics (Basel) (2023) 13(6):1060. doi: 10.3390/diagnostics13061060
22. Alzubaidi L, Zhang J, Humaidi AJ, Al-Dujaili A, Duan Y, Al-Shamma O, et al. Review of deep learning: concepts, cnn architectures, challenges, applications, future directions. J Big Data (2021) 8(1):53. doi: 10.1186/s40537-021-00444-8
23. Chan HP, Samala RK, Hadjiiski LM, Zhou C. Deep learning in medical image analysis. Adv Exp Med Biol (2020) 1213:3–21. doi: 10.1007/978-3-030-33128-3_1
24. Litjens G, Kooi T, Bejnordi BE, Setio AAA, Ciompi F, Ghafoorian M, et al. A survey on deep learning in medical image analysis. Med Image Anal (2017) 42:60–88. doi: 10.1016/j.media.2017.07.005
25. Das PK, Meher S, Panda R, Abraham A. An efficient blood-cell segmentation for the detection of hematological disorders. IEEE Trans Cybern (2022) 52(10):10615–26. doi: 10.1109/TCYB.2021.3062152
26. Raina R, Gondhi NK, Chaahat, Singh D, Kaur M, Lee H-N. A systematic review on acute leukemia detection using deep learning techniques. Arch Comput Methods Eng (2023) 30(1):251–70. doi: 10.1007/s11831-022-09796-7
27. Salah HT, Muhsen IN, Salama ME, Owaidah T, Hashmi SK. Machine learning applications in the diagnosis of leukemia: current trends and future directions. Int J Lab Hematol (2019) 41(6):717–25. doi: 10.1111/ijlh.13089
28. Clark JM, Sanders S, Carter M, Honeyman D, Cleo G, Auld Y, et al. Improving the translation of search strategies using the polyglot search translator: A randomized controlled trial. J Med Libr Assoc (2020) 108(2):195–207. doi: 10.5195/jmla.2020.834
29. Ouzzani M, Hammady H, Fedorowicz Z, Elmagarmid A. Rayyan-a web and mobile app for systematic reviews. Syst Rev (2016) 5(1):210. doi: 10.1186/s13643-016-0384-4
30. Devi TG, Patil N, Rai S, Sarah CP. Segmentation and classification of white blood cancer cells from bone marrow microscopic images using duplet-convolutional neural network design. Multimedia Tools Appl (2023) 82(23):1–23. doi: 10.1007/s11042-023-14899-9
31. Duggal R, Gupta A, Gupta R, Mallick P. (2017). Sd-layer: stain deconvolutional layer for cnns in medical microscopic imaging, In: Medical image computing and computer assisted intervention − MICCAI 2017. (Cham, Switzerland: Springer) 10435:435–43. doi: 10.1007/978-3-319-66179-7_50
32. Huang F, Guang P, Li F, Liu X, Zhang W, Huang W. Aml, all, and cml classification and diagnosis based on bone marrow cell morphology combined with convolutional neural network: A stard compliant diagnosis research. Med (Baltimore) (2020) 99(45):e23154. doi: 10.1097/md.0000000000023154
33. Ikechukwu AV, Murali S. I-net: A deep cnn model for white blood cancer segmentation and classification. Int J Advanced Technol Eng Explor (2022) 9(95):17. doi: 10.19101/IJATEE.2021.875564
34. Kavitha R, Viswanathan N. Cat-inspired deep convolutional neural network for bone marrow cancer cells detection. Intelligent Automation Soft Computing (2022) 33(2):16. doi: 10.32604/iasc.2022.022816
35. Kumar D, Jain N, Khurana A, Mittal S, Satapathy SC, Senkerik R, et al. Automatic detection of white blood cancer from bone marrow microscopic images using convolutional neural networks. IEEE Access (2020) 8:142521–31. doi: 10.1109/access.2020.3012292
36. Ordaz-Gutierrez S, Gallegos-Funes FJ, Rosales-Silva AJ, Carvajal-Gamez BE, Mujica-Vargas D. Diagnosis of acute lymphoblastic leukaemia using fuzzy logic and neural networks. Imaging Sci J (2013) 61(1):8. doi: 10.1179/1743131X11Y.0000000057
37. Rehman A, Abbas N, Saba T, Rahman SIU, Mehmood Z, Kolivand H. Classification of acute lymphoblastic leukemia using deep learning. Microsc Res Tech (2018) 81(11):1310–7. doi: 10.1002/jemt.23139
38. Yang G, Qin Z, Mu J, Mao H, Han M. Efficient diagnosis of hematologic Malignancies using bone marrow microscopic images: A method based on multipathgan and mobilevitv2. Comput Methods Programs BioMed (2023) 237:107583. doi: 10.1016/j.cmpb.2023.107583
39. Zhou M, Wu KF, Yu LS, Xu MD, Yang JJ, Shen Q, et al. Development and evaluation of a leukemia diagnosis system using deep learning in real clinical scenarios. Front Pediatr (2021) 9:693676. doi: 10.3389/fped.2021.693676
40. Clark K, Vendt B, Smith K, Freymann J, Kirby J, Koppel P, et al. The cancer imaging archive (Tcia): maintaining and operating a public information repository. J Digit Imaging (2013) 26(6):1045–57. doi: 10.1007/s10278-013-9622-7
41. Chu S-C, Tsai P-w, Pan J-S. Cat swarm optimization, in: PRICAI 2006: trends in artificial intelligence. Berlin, Heidelberg: Springer (2006). p. 854–8.
42. Das PK DVA, Meher S, Panda R, Abraham A. A systematic review on recent advancements in deep and machine learning based detection and classification of acute lymphoblastic leukemia. IEEE Access (2022) 10:81741–63. doi: 10.1109/ACCESS.2022.3196037
43. Mustaqim T, Fatichah C, Suciati N. Deep learning for the detection of acute lymphoblastic leukemia subtypes on microscopic images: A systematic literature review. IEEE Access (2023) 11:16108–27. doi: 10.1109/ACCESS.2023.3245128
44. Alsalem MA, Zaidan AA, Zaidan BB, Hashim M, Madhloom HT, Azeez ND, et al. A review of the automated detection and classification of acute leukaemia: coherent taxonomy, datasets, validation and performance measurements, motivation, open challenges and recommendations. Comput Methods Programs BioMed (2018) 158:93–112. doi: 10.1016/j.cmpb.2018.02.005
45. Abunadi I, Senan EM. Multi-method diagnosis of blood microscopic sample for early detection of acute lymphoblastic leukemia based on deep learning and hybrid techniques. Sensors (Basel) (2022) 22(4):1629. doi: 10.3390/s22041629
46. Sampathila N, Chadaga K, Goswami N, Chadaga RP, Pandya M, Prabhu S, et al. Customized deep learning classifier for detection of acute lymphoblastic leukemia using blood smear images. Healthcare (Basel) (2022) 10(10):1812. doi: 10.3390/healthcare10101812
47. Atteia G, Alhussan AA, Samee NA. Bo-allcnn: bayesian-based optimized cnn for acute lymphoblastic leukemia detection in microscopic blood smear images. Sensors (Basel) (2022) 22(15):5520. doi: 10.3390/s22155520
48. Rastogi P, Khanna K, Singh V. Leufeatx: deep learning-based feature extractor for the diagnosis of acute leukemia from microscopic images of peripheral blood smear. Comput Biol Med (2022) 142:105236. doi: 10.1016/j.compbiomed.2022.105236
49. Deshpande NM, Gite S, Aluvalu R. A review of microscopic analysis of blood cells for disease detection with ai perspective. PeerJ Comput Sci (2021) 7:e460. doi: 10.7717/peerj-cs.460
50. Anilkumar KK, Manoj VJ, Sagi TM. Automated detection of leukemia by pretrained deep neural networks and transfer learning: A comparison. Med Eng Phys (2021) 98:8–19. doi: 10.1016/j.medengphy.2021.10.006
51. Boldu L, Merino A, Acevedo A, Molina A, Rodellar J. A deep learning model (Alnet) for the diagnosis of acute leukaemia lineage using peripheral blood cell images. Comput Methods Programs BioMed (2021) 202:105999. doi: 10.1016/j.cmpb.2021.105999
52. Ouyang N, Wang W, Ma L, Wang Y, Chen Q, Yang S, et al. Diagnosing acute promyelocytic leukemia by using convolutional neural network. Clin Chim Acta (2021) 512:1–6. doi: 10.1016/j.cca.2020.10.039
53. Gupta R, Gehlot S, Gupta A. C-nmc: B-lineage acute lymphoblastic leukaemia: A blood cancer dataset. Med Eng Phys (2022) 103:103793. doi: 10.1016/j.medengphy.2022.103793
54. Gehlot S, Gupta A, Gupta R. Sdct-auxnet(Theta): dct augmented stain deconvolutional cnn with auxiliary classifier for cancer diagnosis. Med Image Anal (2020) 61:101661. doi: 10.1016/j.media.2020.101661
55. Shafique S, Tehsin S. Acute lymphoblastic leukemia detection and classification of its subtypes using pretrained deep convolutional neural networks. Technol Cancer Res Treat (2018) 17:1533033818802789. doi: 10.1177/1533033818802789
Keywords: acute lymphoblastic leukemia, bone marrow images, medical image analysis, deep learning, convolutional neural networks, diagnosis, classification
Citation: Elsayed B, Elhadary M, Elshoeibi RM, Elshoeibi AM, Badr A, Metwally O, ElSherif RA, Salem ME, Khadadah F, Alshurafa A, Mudawi D and Yassin M (2023) Deep learning enhances acute lymphoblastic leukemia diagnosis and classification using bone marrow images. Front. Oncol. 13:1330977. doi: 10.3389/fonc.2023.1330977
Received: 31 October 2023; Accepted: 27 November 2023;
Published: 06 December 2023.
Edited by:
Felix Seyfried, Ulm University Medical Center, GermanyReviewed by:
Mohammad Alibakhshikenari, Universidad Carlos III de Madrid de Madrid, SpainCopyright © 2023 Elsayed, Elhadary, Elshoeibi, Elshoeibi, Badr, Metwally, ElSherif, Salem, Khadadah, Alshurafa, Mudawi and Yassin. This is an open-access article distributed under the terms of the Creative Commons Attribution License (CC BY). The use, distribution or reproduction in other forums is permitted, provided the original author(s) and the copyright owner(s) are credited and that the original publication in this journal is cited, in accordance with accepted academic practice. No use, distribution or reproduction is permitted which does not comply with these terms.
*Correspondence: Basel Elsayed, YmUxOTA1MjMxQHF1LmVkdS5xYQ==; Mohamed Yassin, eWFzc2lubW9oYUBnbWFpbC5jb20=
Disclaimer: All claims expressed in this article are solely those of the authors and do not necessarily represent those of their affiliated organizations, or those of the publisher, the editors and the reviewers. Any product that may be evaluated in this article or claim that may be made by its manufacturer is not guaranteed or endorsed by the publisher.
Research integrity at Frontiers
Learn more about the work of our research integrity team to safeguard the quality of each article we publish.