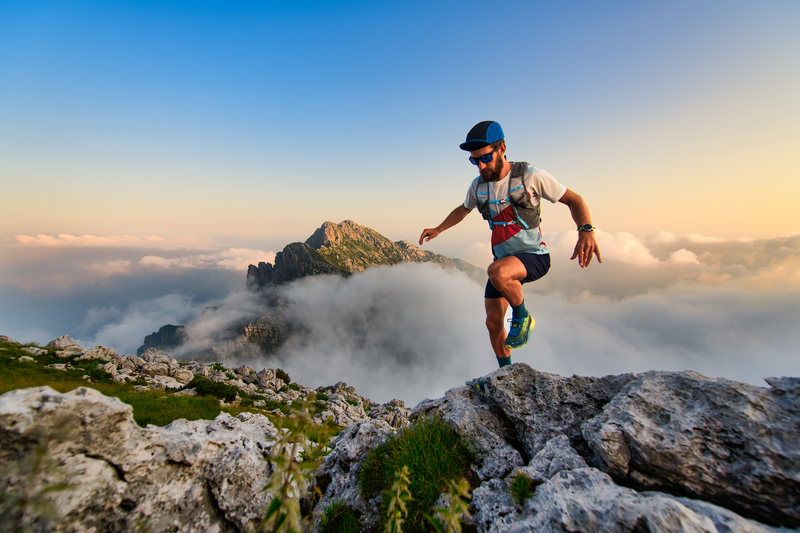
95% of researchers rate our articles as excellent or good
Learn more about the work of our research integrity team to safeguard the quality of each article we publish.
Find out more
EDITORIAL article
Front. Oncol. , 14 November 2023
Sec. Hematologic Malignancies
Volume 13 - 2023 | https://doi.org/10.3389/fonc.2023.1330407
This article is part of the Research Topic Digital Health and Real-World Evidence in Onco-Hematological Patients View all 7 articles
Editorial on the Research Topic
Digital health and real-world evidence in onco-hematological patients
Digital health, as defined by the World Health Organization (WHO), encompasses electronic health (e-health) and, in general, the use of advanced computer sciences, including big data and artificial intelligence (AI). In this context, digital health enhances our ability to study and treat onco-hematological patients in real-world (RW) settings by collecting and sharing RW data from electronic records. The ultimate goal is to support clinical and translational research. Furthermore, digital health RW studies can compile data from numerous cases, providing added value when analyzing rare hematological malignancies.
Digital health solutions address several unmet needs. These include providing easy access to care, reassuring patients, increasing adherence to clinical protocols, enhancing treatment efficacy, reducing hospitalizations, and optimizing healthcare resources. Additionally, AI can support the remote monitoring of cancer patients, assisting healthcare providers with timely interventions. Notably, current challenges in the field of digital health studies involve issues such as data sharing, interoperability, the promotion of open community data standards, and adherence to international guidelines.
Our Research Topic underscores the significance of RW digital health efforts in studying and managing onco-hematological disorders. For instance, Morabito et al. used deep learning and explainable AI to perform gene selection, predicting the need and timing of therapy in chronic lymphocytic leukemia (CLL). Mangone et al. analyzed the incidence, mortality, and survival rates of hematological malignancies in northern Italian patients with data updated through 2020. Mu et al. proposed new machine learning (ML) models by integrating next-generation sequencing and tumor cell size, improving subtype classification of mature B-cell neoplasms (MBN). Wang et al. developed and validated the platelet-to-albumin ratio as a clinical predictor for diffuse large B-cell lymphomas (DLBCL). Bartolini et al. validated the use of Italian administrative healthcare data to describe the RW utilization of infusive antineoplastic drugs. Finally, Zhuang et al. conducted a RW, multi-centered study in China, revealing that clinicians prefer to prescribe lenalidomide or bortezomib as maintenance therapy in non-transplant newly diagnosed multiple myeloma (MM) patients with high-risk cytogenetic abnormalities, as they are superior to thalidomide.
Despite the immense potential of digital RW data for monitoring and evaluating healthcare patterns, including diagnosis, therapy, and daily clinical patient assistance, certain methodological issues continue to be the subject of debate and are discussed here.
First of all, in the six collected articles, data access and sharing primarily occur upon request. Nowadays, we generate vast volumes of data to fuel data-driven scientific discovery. Unfortunately, not all data can be openly shared due to privacy concerns, ownership issues, technical challenges, and trust considerations, hindering research progress and data utility. In medical research, data sharing plays a crucial role in promoting discovery, innovation, transparency, reproducibility, and trust in scientific findings. The COVID-19 pandemic has further accelerated the demand for data sharing, with increasing calls for the rapid dissemination, evaluation, integration, and analysis of new medical research results (1).
Secondly, some digital health projects fail to address clinical needs relevant to clinicians, patients, or public health decision makers. In many cases, they appear to be technical efforts focused on developing statistical or mathematical techniques without effectively solving clinical problems or addressing essential questions. In contrast, papers included in this Research Topic address specific needs. For example, Morabito et al. contributed to the management and treatment of CLL patients. Mangone et al. provided essential epidemiological information on onco-hematological disorders in northern Italy. Mu et al. enhanced the classification of MBN. Wang et al. proposed a clinical predictor for DLBCL. Bartolini et al. validated Italian administrative healthcare data, which is valuable for the public health system. Zhuang et al. contributed to the management of MM patients in a RW Chinese setting.
Thirdly, it’s important to note that while statistical analysis methods like Cox models remain widely used, ML techniques are gradually gaining traction. Some studies in this Research Topic (Mu et al.; Morabito et al.) have utilized ML methods such as Random Forest, K-Nearest Neighbors, Naive Bayes, and Neural Networks. Nevertheless, the widespread adoption of these advanced techniques in this field appears to be in its early stages.
Fourthly, validation is a crucial step in the development of both statistical and ML models as it ensures their ability to generalize to unseen data. Internal validation methods, such as cross-validation, provide an initial assessment of model performance, while external validation, which tests the model on entirely new data, offers a more robust measure of its generalizability. Despite their importance, these validation techniques were not extensively utilized in the studies within this Research Topic. Mu et al. stands out for performing both internal and external validation for the ML techniques, and Wang et al. applied them in their developed Cox models. This may be attributed to a lack of awareness or insufficient data.
After our comprehensive evaluation of the papers’ quality, we considered the issue of translating results into clinical practice. Within our Research Topic, we have identified certain studies that highlight the potential for this translation (Mangone et al.; Mu et al.), while other studies have begun the process of validating their procedures in RW healthcare systems (Bartolini et al.). However, some studies are still in the process of validation (Morabito et al.; Wang et al.; Zhuang et al.).
While progress has been made in applying ML techniques and addressing relevant clinical needs, there is still room for improvement in several areas. These include model validation and adherence to best practice guidelines, such as TRIPOD(-AI) and PROBAST(-AI) (2) for data-driven model reporting, as well as SPIRIT(-AI) and CONSORT(-AI) (3) for designing protocols for clinical studies. These improvements are essential to better address the gaps identified in this Research Topic.
Furthermore, a significant effort should be made to emphasize unmet clinical needs, taking a global perspective and, above all, incorporating aspects related to the patient’s quality of life.
CV: Conceptualization, Methodology, Supervision, Writing – original draft, Writing – review & editing. LL-P: Conceptualization, Methodology, Supervision, Writing – original draft, Writing – review & editing. MC-U: Supervision, Writing – review & editing. GF: Conceptualization, Supervision, Writing – review & editing.
The author(s) declare that no financial support was received for the research, authorship, and/or publication of this article.
The authors declare that the research was conducted in the absence of any commercial or financial relationships that could be construed as a potential conflict of interest.
The author(s) declared that they were an editorial board member of Frontiers, at the time of submission. This had no impact on the peer review process and the final decision.
All claims expressed in this article are solely those of the authors and do not necessarily represent those of their affiliated organizations, or those of the publisher, the editors and the reviewers. Any product that may be evaluated in this article, or claim that may be made by its manufacturer, is not guaranteed or endorsed by the publisher.
1. Flanagin A, Curfman G, Bibbins-Domingo K. Data sharing and the growth of medical knowledge. JAMA (2022) 328(24):2398–9. doi: 10.1001/jama.2022.22837
2. Gary SC. Protocol for development of a reporting guideline (TRIPOD-AI) and risk of bias tool (PROBAST-AI) for diagnostic and prognostic prediction model studies based on artificial intelligence. BMJ Open (2021) 11:e048008. doi: 10.1136/bmjopen-2020-048008
Keywords: clinical practice, digital health, hematological malignancies, real-world evidence, representativeness-generalizations
Citation: Vener C, Lopez-Perez L, Cabrera-Umpiérrez MF and Fico G (2023) Editorial: Digital health and real-world evidence in onco-hematological patients. Front. Oncol. 13:1330407. doi: 10.3389/fonc.2023.1330407
Received: 30 October 2023; Accepted: 03 November 2023;
Published: 14 November 2023.
Edited and Reviewed by:
Alessandro Isidori, AORMN Hospital, ItalyCopyright © 2023 Vener, Lopez-Perez, Cabrera-Umpiérrez and Fico. This is an open-access article distributed under the terms of the Creative Commons Attribution License (CC BY). The use, distribution or reproduction in other forums is permitted, provided the original author(s) and the copyright owner(s) are credited and that the original publication in this journal is cited, in accordance with accepted academic practice. No use, distribution or reproduction is permitted which does not comply with these terms.
*Correspondence: Claudia Vener, Y2xhdWRpYS52ZW5lckBpc3RpdHV0b3R1bW9yaS5taS5pdA==
†These authors have contributed equally to this work
Disclaimer: All claims expressed in this article are solely those of the authors and do not necessarily represent those of their affiliated organizations, or those of the publisher, the editors and the reviewers. Any product that may be evaluated in this article or claim that may be made by its manufacturer is not guaranteed or endorsed by the publisher.
Research integrity at Frontiers
Learn more about the work of our research integrity team to safeguard the quality of each article we publish.