- 1School of Pharmacy, Qingdao University, Qingdao, China
- 2Research and Development Department, NanoPeptide (Qingdao) Biotechnology Ltd., Qingdao, China
- 3Department of Gastrointestinal Surgery, The Affiliated Hospital of Qingdao University, Qingdao, Shandong, China
- 4Department of Chemical Engineering and Waterloo Institute for Nanotechnology, University of Waterloo, Waterloo, ON, Canada
Lung cancer is a malignancy with high incidence and mortality rates globally, and it has a 5-year survival rate of only 10%–20%. The significant heterogeneity in clinical presentation, histological features, multi-omics findings, and drug sensitivity among different lung cancer patients necessitate the development of personalized treatment strategies. The current precision medicine for lung cancer, primarily based on pathological and genomic multi-omics testing, fails to meet the needs of patients with clinically refractory lung cancer. Lung cancer organoids (LCOs) are derived from tumor cells within tumor tissues and are generated through three-dimensional tissue culture, enabling them to faithfully recapitulate in vivo tumor characteristics and heterogeneity. The establishment of a series of LCOs biobanks offers promising platforms for efficient screening and identification of novel targets for anti-tumor drug discovery. Moreover, LCOs provide supplementary decision-making factors to enhance the current precision medicine for lung cancer, thereby addressing the limitations associated with pathology-guided approaches in managing refractory lung cancer. This article presents a comprehensive review on the construction methods and potential applications of LCOs in both preclinical and clinical research. It highlights the significance of LCOs in biomarker exploration, drug resistance investigation, target identification, clinical precision drug screening, as well as microfluidic technology-based high-throughput drug screening strategies. Additionally, it discusses the current limitations and future prospects of this field.
1 Introduction
Lung cancer is the foremost malignancy in terms of morbidity and mortality worldwide, with a 5-year survival rate of only 10%–20% (1, 2). The disease is characterized by a high degree of heterogeneity in its histology, genomic landscape, and response to therapeutic interventions. According to histopathological classification, lung cancer is primarily categorized into non-small cell lung cancer (NSCLC) and small cell lung cancer (SCLC) (3, 4). Among all lung cancers, NSCLC accounts for approximately 85-88%, while SCLC accounts for the remaining 12-15%. Morever, there exists a diversity of gene mutations among different patients with lung cancer, and the key driver genes of lung cancer also exhibit significant variation (4). The National Comprehensive Cancer Network guidelines recommend testing for a panel of key driver genes in NSCLC, including EGFR, ALK and ROS1 (5). Furthermore, the genetic mutations of KRAS and TP53 are pivotal in the pathogenesis of NSCLC. Due to the heterogeneity of tumors, there exist significant variations in patients’ response to specific chemotherapy. Nevertheless, the selection of chemotherapy regimens for lung cancer patients is still largely based on clinical experience, resulting in suboptimal treatment outcomes (6, 7). Currently, an increasing number of targeted drugs are available for lung cancer (8–10). However, the efficacy of these therapies is limited by the absence of reliable biomarkers to predict drug response and the secondary resistance during treatment (11). The current precision medicine strategy, which is based on pathology, gene, and other multi-omics detection results, falls short in effectively addressing the challenging issue of lung cancer. Therefore, it is imperative to explore novel technical approaches or research models to enhance the existing precision medicine strategy.
Conventional approaches to lung cancer research entail the utilization of immortalized lung cancer cell lines, which possess appropriate tumor characteristics can be cultured on a large scale and are amenable to sophisticated analytical techniques, thereby making a significant contribution to cancer research. However, immortalized cell lines may accumulate genetic alterations during prolonged culture, rendering them an inaccurate representation of the original tumor’s genetic properties (12). Monolayer cultured cells display a growth phenotype far removed from that observed in tumors and have limited physiological relevance to human tumors (13). The patient-derived tumor xenografts (PDXs) involve the transplantation of small fragments of surgically excised tumors from cancer patients into highly immunodeficient mice (14). The PDXs often maintain the cellular and histopathological architecture of the original tumor, exhibiting a genomic and gene-expression profile that is remarkably similar to that of the parent patient’s tumor (15–18). However, the application of PDX models is constrained by low success rates, time-consuming procedures, high costs, ethical concerns and species-specific (14, 19). A pressing need exists for an in vitro model that accurately preserves the biological characteristics of the original tumor, thereby augmenting the efficacy of lung cancer treatment. The focus of personalized medicine research has shifted towards emerging technologies, such as tumor organoids - three-dimensional structural models composed of multiple cell types in vitro that can simulate the structure and function of tumors within a patient’s body (20, 21). The generation of organoids represents one of the most cutting-edge advancements in model development, and they have been successfully derived from a diverse range of tumors (22–25). Lung cancer organoids (LCOs) are capable of faithfully recapitulating in vivo tumor characteristics and heterogeneity (26, 27). Furthermore, the response of LCOs to therapeutic drugs is closely correlated with the clinical data of the patients involved, thereby providing supplementary decision-making factors to enhance the current precision medicine system for lung cancer. Compared to PDXs, organoids exhibit a higher success rate in culture, long-term expansion and passage, lower cost and shorter time consumption, making them more suitable for high-throughput drug screening platforms and gene editing technology (28–30). However, LCOs still face several challenges, including low purity (31, 32), lack of specific tumor microenvironment and vascular system (28), as well as issues with standardization and reproducibility of culture, which hinder its broader adoption and implementation in preclinical and clinical research.
In this review, we provide a comprehensive overview of the general approach employed in the construction of LCOs and their potential applications in both preclinical and clinical research. This article will commence with the construction of LCOs, and concentrate on the crucial aspects, current challenges, and potential solutions in the process of constructing LCOs. It elucidates its significance in biomarker exploration, drug resistance research, target identification and drug screening, as well as high-throughput drug screening strategy based on microfluidic technology. Finally, we will address the limitations and future prospects of current organoid applications in lung cancer.
2 Methodology for construction of LCOs
With the advancement of 3D culture technology, numerous laboratories have established biobanks of LCOs (33–35). Ebisudani et al. collected lung cancer samples from various sources, including tumor tissue, sputum, and circulating tumor cells, and established a biobank of 43 patient-derived LCOs that accurately recapitulated the histological and molecular characteristics of the original tumors (33). However, the culture success rate of the 37 samples derived from circulating tumor cells was only 8% (3/37). Similarly, only five out of the 25 sputum samples were successfully cultured (5/25). The presence of a limited number of tumor cells in these samples leads to a low success rate in constructing LCOs. In a study conducted by Kim et al., they successfully established 83 non-small cell LCOs with a success rate of 83.0% (83/100), including specimens from malignant pleural effusion, brain metastasis, bone metastasis and primary tumors (34). Wang et al. established a biobank of 160 LCOs mainly derived from malignant serous effusions obtained from 107 patients with various subtypes of lung cancer, including adenocarcinoma, squamous cell carcinoma, small cell lung cancer, adenosquamous carcinoma, sarcomatoid carcinoma (35). The overall success rate for constructing these organoids was 75.7%. And they proposed that lung adenocarcinoma (LUAD) and samples from malignant serous cavity fluid were more favorable for successful culture. The investigation of the relationship between organoid construction and pathology necessitates further studies with larger samples sizes. Data from different research groups often indicated no significant association between organoid construction success rate and pathology. Shi et al. reported success rates of 84.2% (n=16) for LUAD tissue and 93.3% (n=14) for lung squamous cell carcinoma (LUSC) tissue (22). Hu et al. Reported success rates of 77.5% (n=55), 78.3% (n=18) and 100% (n=4) for LUAD tissue, LUSC tissue, and SCLC tissue, respectively (36). Additionally, Wang et al., proposed that insufficient cells was one of the most common factors (35). Similarly, Sachs et al. showed a significant difference in the success rate of organoid culture between surgically resected tissues and biopsy samples. Biopsy samples-derived NSCLC organoids had a modest success rate of only 28% (5/18), whereas surgically resected tissues-derived NSCLC organoids exhibited a high success rate of up to 88% (14/16) (37). Nevertheless, it should be noted that organoids obtained from biopsy samples tend to be more pure compared to those derived from surgical tissues. The methods used to construct the LCOs will be described in detail below.
Successful organoid construction requires three key elements: appropriate cell origin to ensure initial cell activity and sufficient cell numbers, scaffolds to support the 3D spatial structure of the organoids, and culture media that promote both proliferation and differentiation of the organoids. Patient-derived organoids represent the most crucial category of LCOs. These are generated from tumor tissues or cells obtained from the patient’s body, including surgically resected tumor tissues, biopsy specimens, circulating tumor cells, malignant effusions and sputum-derived tumor cells (33). The success rate of LCOs is contingent upon the evaluation of several factors of specimens, including the number of tumor cells in the initial sample, cell viability, degree of tissue necrosis, and pathological type of tumor tissue. The application of scaffolds is a crucial component in facilitating the growth of organoids in 3D mode. LCOs were primarily embedded in Matrigel, which is a material rich in extracellular matrix proteins secreted by Engelbreth-Holm-Swarm mouse sarcoma cells (38). Matrigel not only supports the spatial structure of organoids but also facilitates their formation and differentiation. Although Matrigel currently used in organoid culture is versatile and affordable, its composition is extremely complex with over 1800 unique proteins identified by proteomics analysis alone (39, 40). This complexity can pose challenges in identifying signals necessary for proper organoid structure and function. The variability of Matrigel between batches is significant. The potential immunogenicity of Matrigel, which is derived from mouse cells, has hindered certain studies on human tumor organoids in immunology (41). Currently, synthetic hydrogels are receiving increased attention due to their mechanical properties, functionality and controllable erosion rate. Replacements of Matrigel with synthetic hydrogels are increasingly gaining popularity for the culture of organoids (42, 43). The culture medium serves as a crucial determinant for the successful cultivation of organoids. In terms of lung cancer, the commonly utilized organoid culture medium is composed of two main components: the basal medium and the additive factors. The basal medium consisted of Advanced DMEM/F12 supplemented with HEPES, B27, N2, antibiotics for microbial contamination control, L-glutamine as a nitrogen source, N-acetylcysteine acting as antioxidants and free radical scavengers, and nicotinamide involved in cellular metabolism capacity. The additive factors, including growth factors, pathway inhibitors, and activators, are comprehensively summarized in Table 1. Different medium formulations need to be selected for different culture purposes. A study have indicated that cancer organoids cultured in different media may exhibit varying sensitivities to the same drug (46). Therefore, it is crucial to consider experimental culture conditions when correlating functional analysis of LCOs with clinical outcomes.
As in vitro stand-ins for patients, patient-derived LCOs need to maintain important properties of patient tumor tissue, including molecular subtype, histological and phenotypic consistency (22, 33–35, 44). Multiple validations have demonstrated that LCOs can accurately replicate the histological subtypes of lung tumor tissues in vivo. Ebisudani et al. have established biobanks encompassing subtypes of lung cancer, including adenocarcinoma, squamous cell carcinoma, small cell lung cancer and large cell neuroendocrine carcinoma organoids (33). Moreover, long-term cultured non-small cell LCOs are capable of maintaining the histological characteristics of their parental tumors. For instance, lung adenocarcinoma organoids can preserve a diverse range of histological subtypes including acinar, lepidic, solid, papillary and mixed types (22). LCOs exhibit genetic mutations, copy number alterations, and aneuploidy patterns that are comparable to those observed in clinical specimens, while largely maintaining the key molecular properties of their parental tumors (33). In tumor tissue, there exist not only aberrantly proliferating neoplastic cells but also no-tumor cells, encompassing immune cells, cancer-associated fibroblasts(CAFs), vascular endothelial cells and other non-neoplastic cells that can be targeted for antitumor therapy (47–51). Dijkstra et al. developed a method capable of co-culturing lung cancer organoids with immune cells, enabling the generation of tumor-reactive T cells by co-culturing non-small cell lung cancer organoids with PBMCs (41). The success rates for generating tumor-reactive CD8+ T cell populations ranged from 33 to 50%. Activated CD8+ T cells exhibited efficient killing of tumor organoids without the normal tissue organoids. This system provides a valuable tool for investigating the mechanisms sensitivity or resistance to immunotherapy and holds promise for producing patient-specific T cell products for adoptive T cell transfer therapy. The lung cancer organoids were co-cultured with peripheral blood monocytes by Takahashi et al., enabling the in vitro evaluation of PD-1 targeted monoclonal antibodies nivolumab and pembrolizumab, both being immune checkpoint inhibitors (52). Another approach developed by Neal et al. involves the utilization of an air-liquid interface (ALI) co-culture system for cultivating non-small cell lung cancer organoids (53). This method enables the preservation of endogenous immune and non-immune interstitial components associated with tumor tissue during organoid construction. A human in vitro immunotherapy model was established through uniform culture of tumor epithelium and autologous tumor-reactive tumor-infiltrating cells. Tumor-infiltrating lymphocytes of human and mouse tumor organoids demonstrated functional activation, expansion, and cytotoxic responses to PD-1/PD-L1 checkpoint blockade as evaluated through a 7-day rapid assessment. However, a limitation of this technique is that tumor-infiltrating lymphocytes cannot be maintained in the culture medium for more than 60 days. The formation of tumor blood vessels creates a malignant tumor microenvironment within the body, providing nourishment to tumors and promoting both tumor progression and drug resistance (54, 55). Inhibiting angiogenesis is a crucial strategy in the treatment of tumors. Seitlinger et al. presented a methodology for the vascularization of lung cancer organoids, wherein human lung fibroblasts were incorporated into NSCLC patient-derived tumor cells to generate more intricate tumor organoids that mimic spatial organization (56). Subsequently, these tumor organoids were vascularized using primary human endothelial and connected to pre-vascularized fibrin hydrogel, thereby simulating the authentic vascular network within the tumor and its microenvironment. This approach holds promise for integration with microfluidic chips in order to evaluate drug efficacy. Nashimoto et al. present a lung cancer organoid chip integrated with a perfusable vascular network, which, when combined with an electrochemical sensing platform, enables the evaluation of oxygen metabolism changes in LCOs before and after drug administration (57). Furthermore, 3D bioprinting is a crucial technique in the field of vascularization strategy. Choi et al. presented an advanced model for vascularized LCOs, which consists of LCOs, lung fibroblasts, and a perfusable vascular network created through 3D biopanning (58). This model allowed for the evaluation of drug responsiveness in a vascularized LCOs. CAFs play a crucial role in various biological processes of cancer, including cancer initiation, progression, drug resistance, and distant metastasis (47). Sen et al. established an organoid model of SCLC with fibroblasts, which validated the paracrine effects of fibroblasts in promoting faster and stronger regeneration of SCLC cells (59). This model provides a valuable platform for targeted drug screening to identify novel therapeutic strategies for SCLC. A 3D co-culture system incorporating extracellular matrix and CAFs can effectively recapitulate the progression of lung squamous cell carcinoma, providing a valuable tool for investigating the dynamic interplay between tumor cells and stromal components (60). Utilizing this model, it was demonstrated that CAFs are capable of inhibiting SOX2 function while promoting the proliferation of patient-derived non-small cell lung cancer (NSCLC) cells. 3D co-culture models that incorporate patient-derived organoids and CAFs hold great promise as a means of capturing the heterogeneity and complexity of primary tumors, making them valuable tools for investigating more effective treatment regimens within the tumor microenvironment.
Tumor organoids for clinical precision medicine require the reliable establishment of pure tumor organoids to obtain more accurate drug screening and genetic testing data. Currently, surgically resected lung specimens are the primary source of LCOs, but these specimens contain a diverse range of cells including not only tumor cells but also a significant number of normal lung epithelial and interstitial cells. With the aforementioned culture methods and conditions, airway epithelial cells of normalcy can be derived from lung cancer tissue and subsequently undergo excessive proliferation. Dijkstra et al. identified 70 organoids from NSCLC samples using a genetic testing method, and determined that only 17% of the cultures were pure non-small cell LCOs while 80% showed normal airway overgrowth (32). Furthermore, distinguishing between these two types of organoids is not possible through simple histomorphological methods, rendering manual removal of normal morphology organoids an unsuitable method for purifying LCOs. In order to inhibit the growth of normal airway and alveolar organoids, various media formulations have been developed based on the differential reliance on growth factors between normal and cancerous cells. For instance, since normal airway and alveolar organoids are unable to proliferate in the presence of ERBB inhibitor, this condition hinders ERBB signaling by eliminating EGF, insulin growth factor-1, fibroblast growth factor-2 while introducing a pan-ERBB inhibitor to prevent formation of normal airway organoids (33). Alternatively, based on the high frequency of TP53 mutations in lung cancer, the addition of Nutlin-3 (an MDM-2 inhibitor) to the culture medium effectively suppressed normal organoids and enriched TP53-mutant LCOs (33, 61). However, this approach also inhibited the formation and growth of TP53 wild-type LCOs, resulting in a partial loss of heterogeneity. It is noteworthy that Hu et al. have reported a mechanical treatment method, which involves gentle grinding followed by filtration through a 100 µm filter using a syringe and collection of tumor fragments ranging from 40 to 100 µm with the aid of a 40 µm filter (36). Late culture using growth factor-deficient medium for LCOs, which lack essential factors such as FGF7, FGF10, Rspondin-1 and Noggin, resulted in a significant increase of tumor cell proportion from 49 ± 15% in tumor tissue to 78 ± 17% in all no-passaged LCOs. This approach has been demonstrated to facilitate rapid formation of large LCOs within 24 h while minimizing contamination by mixed cells. The purity of organoids is significantly affected by the type of sample. Organoids derived from malignant ascites are preferred due to their mainly tumor cell composition, which results in more purer tumor organoids and makes them excellent candidates for drug sensitivity testing (33–35). Both tissue and malignant ascites-derived LCOs effectively reflect the pathological and molecular characteristics of primary tumors, providing a reliable foundation for subsequent drug sensitivity testing (35). While some progress has been made in purifying LCOs, further exploration is necessary to develop an easy-to-use purification method with higher purity that fully preserves the heterogeneity of the original tumor.
The process of normal cell transformation into tumor cells necessitates a sequence of genetic mutations, including the activation of oncogenes or the inactivation of tumor suppressor genes (62). Naranjo et al. generated LCOs harboring specific mutations by introducing alterations in key genes, such as KRAS, BRAF, and ALK, into AT2 cells-the initiating cell type of mouse lung adenocarcinoma (63). LCOs can also be derived from various pluripotent stem cells, such as human embryonic stem cells, lung epithelial progenitor cells, induced pluripotent stem cells (64–66). The utilization of gene-edited LCOs, presents an opportunity to investigate the initial stages of lung cancer and how genetic damage triggers carcinogenesis (66). These non-tumor cell-derived models offer a valuable research tool for exploring the relationship between gene mutations and cancer initiation, as well as the biological behavior of tumors.
3 LCOs for preclinical research
Lung cancer is a disease characterized by heterogeneity, which results in diverse responses to drugs among patients. Genetic detection methods can assist in selecting targeted therapy for lung cancer patients; however, the complexity of gene mutations and the lack of reliable biomarkers contribute to primary or secondary drug resistance, leading to suboptimal overall patient benefit rates (67). The LCOs represent an in vitro culture model that can faithfully recapitulate the characteristics of tumors. Some research groups have established LCO-based biobanks, which provide a valuable tool for identifying biomarkers (33), discovering therapeutic targets (68) and investigating drug resistance (69) (Figure 1).
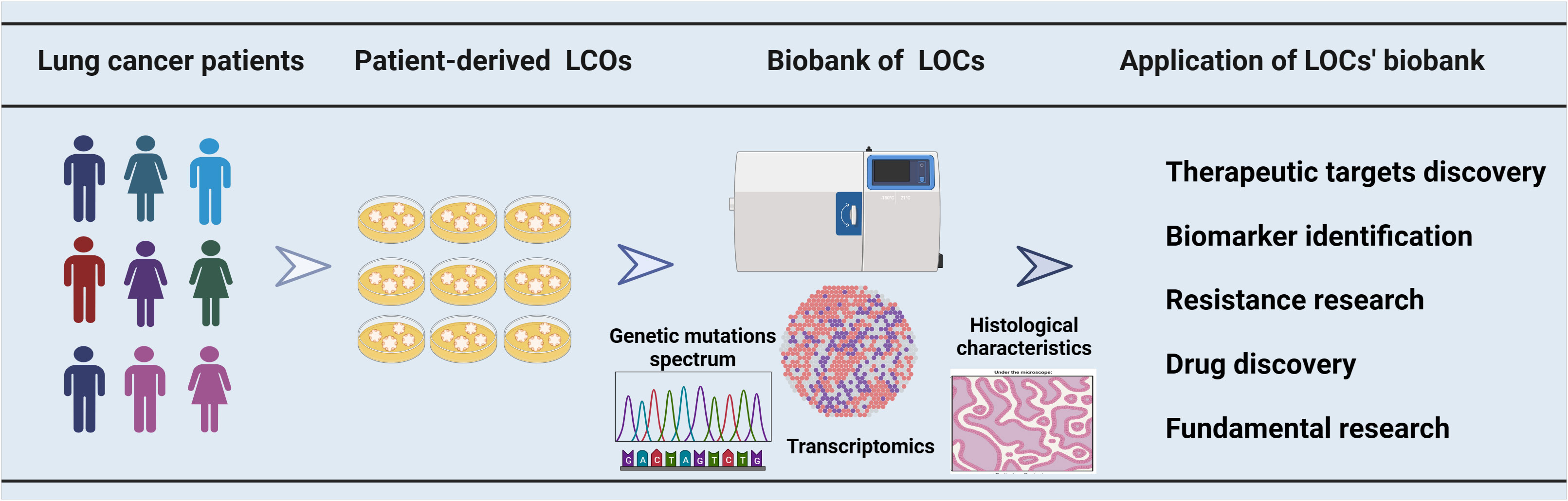
Figure 1 The utilization of a biobank containing organoids derived from lung cancer patients in cancer research. A biobank comprising multiple samples was established using LCOs derived from patients with lung cancer. The LCOs biobanks for lung cancer were characterized through gene sequencing, transcriptome analysis, and histological examination. These biobanks have a broad range of applications, encompassing therapeutic target discovery, biomarker identification, drug resistance research, drug development and some fundamental research.
3.1 LCOs for identifying biomarkers
Predictive biomarkers commonly evaluated in cancer therapy include specific protein expression levels, somatic DNA alterations in a single gene, genome-wide patterns of somatic DNA alterations, and populations of non-tumor cells that shape the tumor microenvironment (70). Biomarker-matched therapies have demonstrated significant survival benefits in lung cancer patient (71, 72). However, despite the development of biomarkers in cell and animal models, less than 1% of cancer biomarkers published are ultimately translated into clinical practice (71, 73). LCOs have the ability to replicate the genetic characteristics and protein expression of individual patients, making them a promising tool for predicting treatment outcomes through biomarker analysis. The identification of new biomarkers based on the relationship between niche factor-dependent phenotypes and genotypes in LCOs represents an innovative approach. A chemically defined serum-free medium was utilized to investigate the impact of growth factors on LCOs proliferation. Through gene sequencing techniques, Ebisudani et al. discovered that loss of NKX2-1 resulted in Wnt-3A and R-spondin dependence in lung adenocarcinoma organoids (33). Further investigation revealed that the porcupine inhibitor (C59) targeting Wnt signaling pathway could specifically target NKX2-1 deficient LCOs and effectively inhibit their proliferation. The Wnt signaling pathway is a promising drug target for lung cancer, and the efficacy of Wnt inhibitors (porcupine inhibitors) is currently being validated in multiple phase I clinical trials. However, drug resistance has been observed in some patients. Therefore, from a clinical perspective, NKX2-1 expression identified by LCOs has the potential to serve as a practical biomarker for predicting response to Wnt-targeted therapy in lung cancer. LCOs can be combined with genetic data to identify novel biomarkers. The high frequency of KRAS mutation in lung cancer is often associated with increased invasiveness, poor prognosis, and drug resistance (74–76). Tyc et al. employed a genetic assay to identify KDS30, a marker comprising 30 gene expression signatures in KRAS mutation-dependent tumors (77). The combination therapy of neratinib (an EGFR/ERBB2 inhibitor) and cobiotinib (a MEK inhibitor) exhibited synergistic anti-tumor proliferation exclusively in organoids derived from patients with high KDS30 mt KRAS rather than low ones. Organoids, as in vitro models of patients, possess inherent advantages when combined with cutting-edge biotechnology to obtain valuable predictive biomarkers (78). Utilizing CyTOF, a single-cell detection technique, Taverna et al. stratified cells based on the expression levels of AXK and JAK and employed LCOs to evaluate the efficacy of AXL and JAK inhibitors (45). LCOs with moderate to high levels of AXL and JAK proteins exhibited heightened sensitivity to TP-0903 (an AXL inhibitor) and ruxolitinib (a JAK inhibitor), whereas those with low expression failed to respond. Analysis of The Cancer Genome Atlas patient samples revealed that chromosome 3p24, which encompasses RBMS3, is frequently lost in NSCLC and correlates with poor prognosis. CRISPR/Cas9-mediated RBMS3 knockdown promotes the growth of BRAFV600E-driven lung organoids (79). Silencing of RBMS3 confers resistance to dabrafenib/trametinib but sensitivity to porcupine inhibition in BRAFV600E-mutant lung tumors, suggesting that RBMS3 silencing and BRAFV600E mutation may serve as predictive biomarkers for drug response. LCOs, as a preclinical tumor model with similar molecular characteristics to tumor samples while minimizing the confounding effects of miscellaneous cells on test results, can be combined with high-throughput RNA sequencing (RNA-Seq) to identify IRAK1BP1 as a novel disease predictor in lung adenocarcinoma (80). The loss of IRAK1BP1 is associated with poor prognosis in patients with lung adenocarcinoma. Biomarker-based stratification of patients enables identification of individuals with specific characteristics that would benefit from treatment, thereby enhancing overall therapeutic efficacy. Patient-derived LCOs generated from malignant pleural effusions were utilized to assess drug sensitivity and distinguish the impact of MET dysregulation on first-line therapy in EGFR-mutated patients (81). Patients were stratified into two groups: EGFR+/METamp- (n = 22) and EGFR+/METamp+ (n = 18). The clinical outcomes of untreated patients with advanced non-small cell lung cancer in the EGFR+/METamp+ group were compared to those in the EGFR+/METamp- group. Dual targeted therapy was found to be more effective than tyrosine kinase inhibitor (TKI) monotherapy for patients in the EGFR+/METamp + group. In the realm of lung cancer research, a plethora of biomarkers have been identified and deemed efficacious; however, their clinical validation remains elusive (71). FGFR1 amplification is not a reliable biomarker for FGFR inhibitors in treating lung squamous cell carcinoma due to its limited efficacy with only 7% to 11% of patients exhibiting durable responses in clinical trials (82, 83). Shi et al. proposed the utilization of LCOs characterized by FGFR1 amplification for evaluating the efficacy of combination therapy regimens (22). The co-administration of FGFR inhibitor and MEK inhibitor (trametinib) exhibited a potent synergistic effect, targeting pFGFR, pAkt, and pErk, thereby effectively inhibiting tumor organoid proliferation. Amplification of FGFR1 as a biomarker supports the use of combined FGFR and MEK inhibitors in lung squamous cell carcinoma.
3.2 LCOs for discovering targets
The identification of novel therapeutic targets is a crucial foundation for the development of new drugs. However, conventional lung cancer cell lines utilized in research often present challenges in identifying valuable therapeutic targets due to limited cellular diversity, loss of spatial organization and tumor microenvironment, as well as gradual loss of tumor specificity during prolonged culture and alterations in gene expression profiles (84). LCOs posses inherent advantages in the identification of novel therapeutic targets due to their ability to faithfully replicate crucial characteristics of lung tumors. Ma et al. utilized non-small cell LCOs to identify CDK1, CCNB2, and CDC25A as pivotal oncogenes in lung adenocarcinoma but not in lung squamous cell carcinoma (68). Subsequent knockdown experiments targeting CDK1 and CCNB2 using both adenocarcinoma cell lines and LCOs demonstrated their inhibitory effects on the proliferation of lung adenocarcinoma. Knockdown of CDC25A did not impede the proliferation of lung adenocarcinoma cell lines, but effectively suppressed the growth of lung adenocarcinoma organoids. These findings are likely attributed to differential gene expression between 2D and 3D cultures. CDK1, CCNB2, and CDC25 may serve as promising therapeutic targets and potential biomarkers. Fascin, a pro-metastatic actin bundling protein upregulated in all metastatic cancers, promotes tumor growth and metastasis by increasing glycolysis in lung cancer. Lin et al. reported that pharmacological inhibitors of Fascin can effectively inhibit YAP1-PFKFB3 signaling and glycolysis in LCOs, thereby inhibiting tumor growth and metastasis (85). These findings suggest a promising therapeutic target for lung cancer.
3.3 LCOs for studying drug resistance
Many cancer patients initially respond well to drug treatment, but eventually develop resistance through complex mechanisms such as drug efflux, DNA damage repair, inhibition of cell death, and DNA mutations (86–89). In order to further advance the development of new generation anti-tumor drugs, an in vitro drug-resistant tumor model is crucial for preclinical drug evaluation. Tumor organoids also offer significant advantages in addressing lung cancer drug resistance due to their ability to accurately replicate the epigenetics, genetic profiling, and histopathology of tumors in vivo. Banda et al. introduced erlotinib, an EGFR inhibitor commonly used in lung cancer treatment, into organoids culture and observed a significant enrichment of at least one known therapy-resistant mutation (BRAFV600E, KRASG12D, KRASG12V, and PIK3CAH1047R) associated with erlotinib after prolonged culture (69). They established an erlotinib resistance model for lung adenocarcinoma organoids that can be utilized to simulate tumors with various mutation subsets. Organoids can serve as tools for investigating drug resistance mechanisms and developing novel therapeutic strategies. Han et al. utilized patient-derived non-small cell LCOs to explore drug resistance and found that elevated expression of tumor CD73 in patients with EGFR mutation contributes to the immunologically quiescent microenvironment of EGFR-mutant NSCLC, leading to immune checkpoint therapy resistance (90). Yan et al. demonstrated the crucial role of DCLK1 in maintaining tumor cell stemness properties, as well as its high expression in EGFR-TKI-resistant LCOs (91). Furthermore, they found that DCLK1 inhibitors can reverse this secondary resistance to TKI. Therefore, for lung adenocarcinoma patients with EGFR-TKI (gefitinib, erlotinib) resistance, DCLK1 inhibitors may serve as a promising alternative treatment option. Combination therapy or multidrug therapy is a promising approach for treating lung cancer and eradicating mutant subpopulations that cause drug resistance. Tumor organoids can provide a more accurate representation of drug resistance occurrence in vivo and facilitate the development of new drug combinations that effectively prevent tumor growth and overcome drug resistance. Glutathione S-transferase pi (GSTP1) is a phase II detoxification enzyme that is highly expressed in lung cancer and mediates chemotherapy resistance (92). The combination of ezatiostat, a specific GSTP1 inhibitor, and crizotinib, an ALK inhibitor, can regulate the activity of lung cancer stem cells. This combined treatment has demonstrated significant inhibitory effects on the proliferation of TKI-resistant lung adenocarcinoma organoids. The development of sensitizing agents is a crucial strategy in combating drug resistance. Manoalide, a natural inhibitor of PLA2, has been identified as a potential EGFR-TKI sensitizer for KRAS-mutated and osimertinib-resistant lung cancer organoid by inhibiting the KRAS-ERK signaling pathway (93). Cisplatin, a first-line chemotherapeutic agent for lung cancer treatment, often leads to drug resistance in patients (94, 95). Li et al. discovered that halofuginone, a natural compound, can sensitize cisplatin-resistant LCOs by inducing G0/G1 phase arrest and promoting apoptosis through PI3K/AKT and MAPK signaling pathway inhibition (96). This finding may improve the prognosis of cisplatin-resistant lung cancer patients.
4 The application of LCOs in drug screening and precision medicine
Chemotherapy and radiotherapy are established treatment modalities that serve as the standard of care for a variety of cancers. However, many antitumor therapies are associated with toxicity and non-response (97). Tumor organoids can be utilized to identify the direct impact of antineoplastic drugs on cancer cells, thereby distinguishing effective from ineffective treatments (Figure 2). In a real-world study, Wang et al. established a biobank of living LCOs derived from malignant ascites of patients with lung cancer, and demonstrated its efficacy in predicting patient response (35). The sensitivity of organoids to osimertinib, chemotherapy, dual targeted therapy and other targeted therapy was 86.7%(13/15), 83.3%(10/12), 100%(10/10) and 70.6%(12/17), respectively. The overall sensitivity and specificity of the test were 84.0% (95%CI, 63.08%-94.75%) and 82.8% (95%CI, 63.51%-93.47%), respectively, with an accuracy rate of 83.3%. LCOs have the potential to prevent unnecessary treatment for patients who are unlikely to benefit from it. Further prospective clinical trials are required to investigate the feasibility of organoid-guided therapy for lung cancer patients. The subsequent systematic review focuses on the utilization of LCOs as in vitro models to assess drug sensitivity, encompassing chemotherapeutic agents and targeted drugs against common lung cancer biomarkers such as EFGR, ALK, and KRAS.
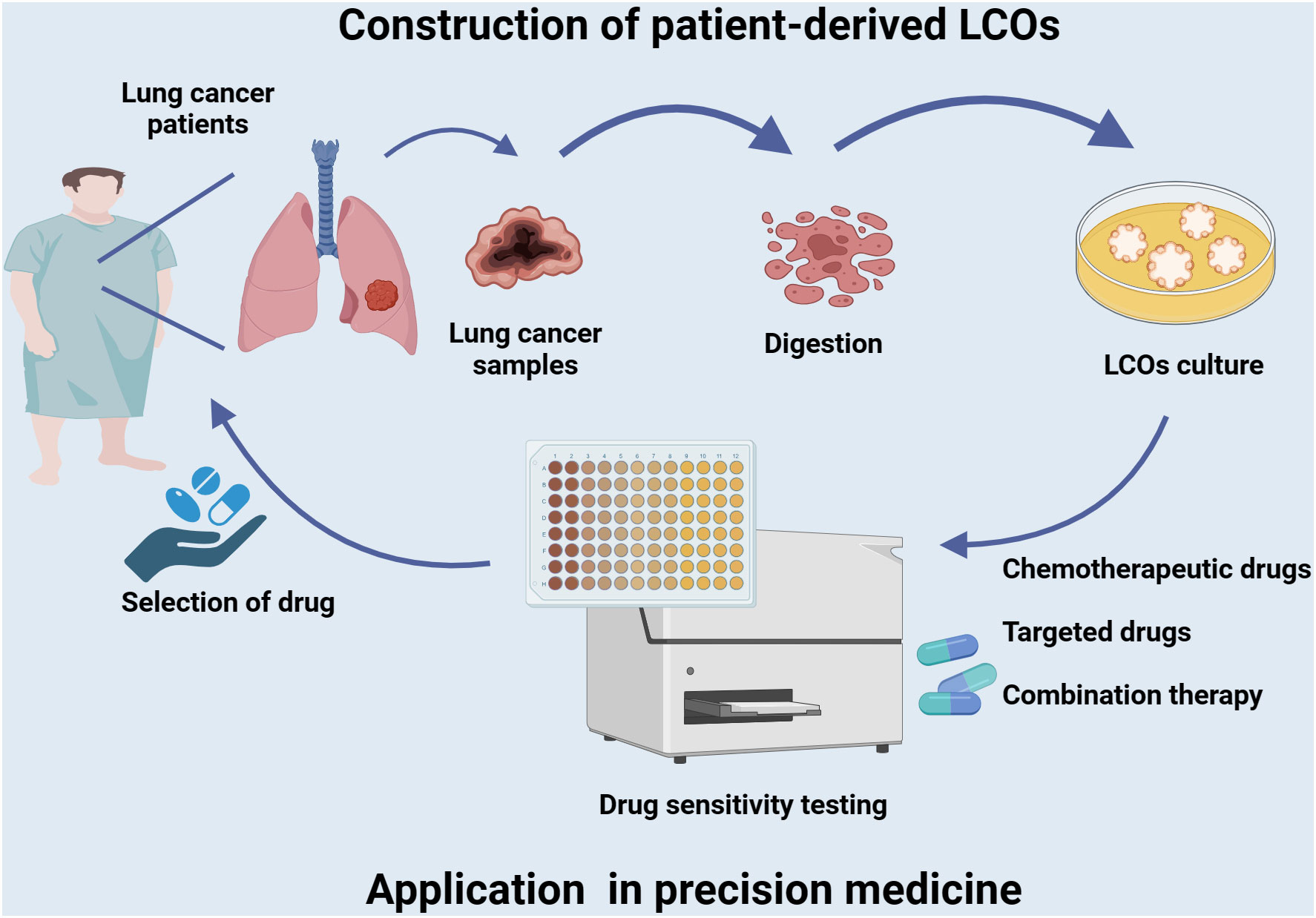
Figure 2 Establishment of LCOs and its application in precision medicine. Tumor tissues of lung cancer patients are collected to generate LCOs. The LCOs can be utilized directly for drug screening of a variety of commonly used first-line and second-line drugs, including chemotherapy agents, targeted therapies, or combination regimens. Corresponding sensitive drugs are selected to develop a personalized treatment plan based on the patient’s characteristics and facilitate precise treatment.
EGFR is a tyrosine kinase receptor that triggers the activation of multiple downstream pathways, including the RAS/MAPK, PI3K/AKT, and JAK/STAT pathways (98–100). It plays a pivotal role in regulating cellular processes such as proliferation, survival, adhesion, migration, and differentiation. Overexpression of EGFR and dysregulation of its signaling pathways have been observed in various types of cancer, particularly NSCLC (101, 102). Wang et al. utilized LCOs derived from malignant ascites of patients to predict the clinical response to osimertinib, an EGFR mutation-targeting agent (35). Based on both the clinical treatment outcomes and drug sensitivity test results of corresponding patient-derived LCOs, it was observed that the IC50 value for progressive disease (PD) group was significantly higher than that for partial response (PR) group. The confirmation of the correlation between tumor volume reduction and IC50 value suggests that this approach may serve as a predictive tool for the efficacy of targeted agents in treating EGFR mutations-associated lung cancers. There exist eight prevalent EGFR mutations, encompassing DeletionExon19, DeletionExon21, G719X mutation, L861Q mutation, L858R mutation, S768I mutation, T790M mutation and Insertion-Exon20 mutation (103). Bie et al. reported that organoids could also serve as a valuable tool for assessing the sensitivity of rare EGFR double mutations to EGFR-TKIs (104). A case study involved that patient-derived non-small cell lung cancer organoids containing the rare EGFR 19Del/L643V double mutation type organoid, which was found to be responsive to osimertinib and gefitinib but resistant to erlotinib and icotinib, highlighting the importance of personalized treatment for patients harboring rare EGFR double mutations. Kim et al. showed that two LCOs with same EGFR p.L858R mutant exhibited varying degrees of sensitivity to the c-Met inhibitor crizotinib (44). It is noteworthy that certain organoids may still manifest sensitivity to EGFR-TKI, despite the absence of EGFR mutations (103). In a female Asian NSCLC patient, common EGFR mutations were not detected in either primary tumor tissue or biopsy-derived PDO. The genetic test report did not indicate sensitivity to EGFR TKIs, such as gefitinib and erlotinib. However, the patient’s PDO drug sensitivity test results revealed that she was sensitive to gefitinib. Following treatment with gefitinib, the patient’s prognosis significantly improved. This underscores the importance of functional drug testing using LCO and studying resistance mechanisms through organoids research in order to better predict clinical response to drugs.
NSCLC is the most prevalent tumor associated with ALK gene fusion. Approximately 5% of NSCLC patients exhibit positive results for ALK fusion, with EML4 being the primary fusion partner (105, 106). The patient was initially diagnosed with EML4-ALK fusion (35). Following resistance to second-generation ALK-TKIs, ceritinib and SAF-189s, alectinib was administered as third-line targeted therapy. However, organoid drug susceptibility testing revealed a lack of sensitivity to alectinib, ultimately leading to the development of intracranial metastasis and disease progression. The clinical efficacy of targeted therapy in ALK gene fusion lung cancer patients was validated, and the drug susceptibility detection results from all organoids were consistent with the clinical response (100%, 5/5). Despite a small sample size, this study highlights the potential for tumor organoids to serve as in vitro surrogates for patients.
RAS mutations are frequently observed in gastrointestinal and lung malignancies, with KRAS mutations being the most prevalent subtype (107). KRAS mutations account for approximately 78% of all RAS mutations detected in NSCLC (108, 109). KRAS mutant tumor cells have been shown to exhibit greater sensitivity to MEK inhibitors compared to KRAS wild-type cell lines (110). This finding is consistent with preclinical studies conducted on KRAS mutant LCOs, which demonstrated that LCOs carrying the KRAS mutation were more responsive to trametinib, a MEK inhibitor, than their wild-type counterparts. Specifically, the IC50 value for KRAS mutant organoids was lower than wild-type counterparts (22). The MEK inhibitor selumetinib yielded similar results. Although not yet validated in clinical treatment outcomes, these findings support the use of organoids as a promising tool for preclinical drug screening.
The occurrence of resistance to individual anticancer agents is prevalent among patients with advanced lung cancer (111, 112). Due to the intricate mechanisms underlying drug resistance, it is challenging to satisfy clinical demands through monotherapy. The utilization of LCOs holds significant potential in guiding combination therapy efficacy prediction in vitro. Wang et al. reported that the patient’s tumor exhibited molecular characteristics of EGFR L858R mutation and MET copy number gain, and despite treatment with osimertinib monotherapy, disease progression persisted (35). In this patient-derived LCOs model, the IC50 value for osimertinib in combination with itself was lower than that observed for either agent alone. The patient exhibited a PR to a treatment regimen consisting of osimertinib and vorolitinib. These cases suggest that LCOs have the potential to predict effective combination therapies.
Monoclonal antibody blockade is the most direct targeted therapy for EGFR which overexpressed in 85% of NSCLC (102, 113). Cetuximab’s ability to inhibit EGFR signaling and lead to growth inhibition is due to the high expression of EGFR and EGF-dependent growth of LCOs (106). HER2 can activate the same key signaling pathways as EGFR, making it an ideal target for anticancer drugs. The sensitivity of three LCOs to monoclonal antibody drugs targeting tumor cells, including trastuzumab, pertuzumab, and trastuzumab emtansine, was assessed using a 384-well plate high-throughput screening system (52). Trastuzumab did not exhibit any inhibitory effect on the tested organoids; however, trastuzumab emtansine, an antibody-drug conjugate (ADC) targeting microtubules, demonstrated potent cytotoxicity against each LCOs. The degree of inhibition correlated with the level of HER2 protein expression. The LCOs models represent a valuable tool for screening antibody-based therapeutics and the drug sensitivity profiling supports the clinical potential of ADCs as efficacious anti-tumor agents.
The efficacy of chemotherapy drugs commonly used in the treatment of lung cancer is generally limited, and they also carry significant risks of adverse effects (6, 114). Therefore, it is crucial to determine appropriate medication strategies for individual patients and identify those who are most likely to benefit from such treatments. Wang et al. reported on the treatment of lung cancer patients with a combination therapy involving both etoposide and cisplatin, referred to as EP therapy (35). Despite variations in disease subtypes, including lung adenocarcinoma and small cell lung cancer, drug sensitivity testing conducted on LCOs demonstrated consistent sensitivity independent of disease pathology, accurately predicting clinical response to the EP regimen. The in vitro detection of LCOs may serve as a reliable indicator for the clinical response of patients to chemotherapy agents.
5 LCOs integrated on a microfluidic chip
Due to the limited number of viable tumor cells obtained from patient samples and the use of conventional cell culture techniques operating in microupscaling volumes, drug testing using organoids may take weeks or even months before providing results to patients (115, 116). Moreover, in comparison to the standard cell culture system, tumor organoids suffer from several drawbacks including high cost, low throughput, complex operation and poor repeatability, which ultimately reduces the reliability and accessibility of tumor organoids as a tool for predicting clinical responses. To surmount these technical challenges, an approach to address these issues is to establish an organoid drug susceptibility testing platform utilizing microfluidic technology (117, 118). Jung et al. employed soft lithography technology to produce a cost-effective, one-step 3D microfluidic platform. The device featuring 29 wells was infused with Matrigel and seeded with patient-derived LCOs for drug sensitivity testing of cisplatin and etoposide via flow medium culture (119). Organoids exhibiting faithful recapitulation of tumor characteristics and uniform size distribution were found to yield reliable and accurate drug responses. To meet the patient’s time constraints and shorten testing duration, Hu and colleagues have developed an integrated superhydrophobic microwell array chip (InSMARchip) as a replacement for conventional 96-well culture plates in drug susceptibility testing of LCOs at the nanoliter scale (36). 3-day-old no-passaged organoids were seeded into InSMARchip and subjected to a three-day drug susceptibility test. A set of drug tests recommended by clinical practice guidelines can be completed within a week without requiring prolonged amplification time. Furthermore, analysis of patient samples has demonstrated robust associations between reported drug responses and genetic mutations as well as clinical outcomes. The research group subsequently developed a vitrification-based freezing method for the in situ cryopreservation of LCOs (120). The tumor organoids are frozen on smart chips and can be stored in a liquid nitrogen tank for a long time. After thawing, drug sensitivity testing can be resumed with minimal damage to LCOs induced by freezing and thawing. The cryopreserved chip is now ready for subsequent high-throughput drug screening applications, providing convenience in the use of LCOs.
6 Current challenges and perspectives
Although LCOs hold great promise as a drug screening tool, potential biomarker library, and model for drug evaluation, this advanced model is not without limitations.
1. Cells within the tumor microenvironment, including CAFs, adipocytes, endothelial cells, and immune cells, play a pivotal role in the initiation and progression of cancer (121–123). These cells associated with cancer regulate various aspects of cancer cell behavior such as proliferation, migration, invasion and apoptosis through direct cell-to-cell interactions as well as secretion of soluble factors, extracellular matrix components and small metabolites (47, 124, 125). Therefore, to better replicate cancer progression and drug response in vivo, it is imperative to incorporate these components into an in vitro model. However, the current LCOs culture oversimplify the interaction between extracellular matrix and cancer cells. Most LCO models solely consist of malignant cells, with fibroblasts and immune cells gradually disappearing during prolonged cultivation (126). Although growth factors and supplements can partially replicate the function of stromal cells, the lack of multicellular components in the tumor microenvironment remains a significant limitation of current LCO models. As such, they cannot be used to evaluate PD-1/PD-L1 immunotherapy or vascular-targeted drug treatments. With the advancement of co-culture, microfluidic and 3D printing technologies, numerous studies have been conducted on the interaction between tumor organoids and stromal cells to facilitate the identification of more targets and biomarkers for lung cancer treatment, as well as a superior drug evaluation platform. Despite many attempts by researchers to address this issue, enhancing mimicry in the tumor microenvironment remains a challenge.
2. LCOs serve as surrogate models for in vitro drug testing, enabling the prediction of patient-specific drug sensitivity and facilitating precision medicine. Due to variations in tumor tissue or cell sources, differences in culture media components across laboratories, variability in drug sensitivity detection methods for organoids, and diverse evaluation indicators of drug sensitivity (including IC50 based on cell activity detection reagents and area changes based on staining and imaging) (23, 35, 127), the complex operational steps, the organoid drug susceptibility testing results is suboptimal in reproducibility and accuracy. Organoid-based drug susceptibility testing requires more standardized culture and detection methods to gain greater clinical recognition. Liquid handling robots and automated high-throughput culture and analysis systems are among the new technologies that can optimize the utilization of tumor organoids (36, 128, 129). Furthermore, combining whole-exome sequencing, copy number assessment, and RNA sequencing can standardize the characterization of tumor organoids to ensure reproducibility and clinical efficacy consistency.
3. Organoids require integration with advanced biotechnologies to optimize their functionality (130). By combining organoids with single-cell technology, it is possible to determine whether tumor organoids can accurately represent the heterogeneity of lung cancer and gain insight into lung cancer development through organoid models (16, 45). Additionally, CRISPR/CAS9 gene-editing technology can be employed in conjunction with LCOs (79, 131, 132). This efficient system for editing organoids’ genes can be utilized to investigate the molecular mechanisms underlying lung cancer development, rapidly characterize genes related to cancer in vivo, replicate the entire process of tumor progression and metastasis, and explore its mechanism. Additionally, organoids can be combined with high-content imaging techniques to investigate drug mechanisms of action in a more sophisticated manner (133, 134). Organoids can be integrated with a genetic testing-based big data platform to identify biomarkers for drug efficacy and address the challenge of chemotherapy sensitivity that cannot be resolved by conventional genetic testing methods (77, 80). The incorporation of artificial intelligence analysis into organoid models enables accurate evaluation of drug effectiveness and facilitates new drug development (135, 136). The integration of organoid, microfluidic and 3D printing technologies enables the rapid establishment of a high-throughput organoid platform for drug screening and personalized medicine in cancer patients within one week (36, 128, 129, 137). Organoids hold immense potential for application in conjunction with state-of-the-art biomedical technologies, thereby enhancing their efficacy in research.
7 Conclusion
Organoid culture has already made a significant impact on the study of lung cancer. With its wider application, it has surpassed the limitations of previous clinical and laboratory studies and demonstrated extensive potential for use. By providing an easily manipulable model that allows for direct comparison of genotypes and phenotypes in a short period of time, organoid culture has opened up various experimental techniques that were previously unattainable. The utilization of LCOs enhances our fundamental comprehension of the initiation and progression, biology, and pathology of lung cancer, and is anticipated to be extensively applied in biomedical fields ranging from disease modeling to drug screening and personalized medicine. Nevertheless, despite its remarkable utility as a model system, the challenges confronting organoids cannot be disregarded. The resolution of these inquiries necessitates a multidisciplinary approach, requiring close collaboration between biologists, clinicians, and bioengineers to further investigate the numerous scientific questions surrounding LCOs. We firmly believe that organoids offer unique advantages in comprehending the onset, progression, and treatment of lung cancer. This will significantly advance both basic research and clinical treatment of cancer while greatly enhancing human health.
Author contributions
YL: Writing – original draft, Writing – review & editing. YZ: Conceptualization, Methodology, Writing – review & editing. PC: Conceptualization, Formal Analysis, Resources, Writing – review & editing.
Funding
The author(s) declare that no financial support was received for the research, authorship, and/or publication of this article. This work was supported by the Postdoctoral Project of Qingdao (QDBSH20220202207).
Acknowledgments
Thanks to the related institutions for their help.
Conflict of interest
The authors declare that the research was conducted in the absence of any commercial or financial relationships that could be construed as a potential conflict of interest.
Publisher’s note
All claims expressed in this article are solely those of the authors and do not necessarily represent those of their affiliated organizations, or those of the publisher, the editors and the reviewers. Any product that may be evaluated in this article, or claim that may be made by its manufacturer, is not guaranteed or endorsed by the publisher.
References
1. Sung H, Ferlay J, Siegel RL, Laversanne M, Soerjomataram I, Jemal A, et al. Global cancer statistics 2020: globocan estimates of incidence and mortality worldwide for 36 cancers in 185 countries. CA Cancer J Clin (2021) 71:209–49. doi: 10.3322/caac.21660
2. Torre LA, Siegel RL, Jemal A. Lung cancer statistics. Adv Exp Med Biol (2016) 893:1–19. doi: 10.1007/978-3-319-24223-1_1
3. de Sousa V, Carvalho L. Heterogeneity in lung cancer. Pathobiology. (2018) 85:96–107. doi: 10.1159/000487440
4. Rodriguez-Canales J, Parra-Cuentas E, Wistuba II. Diagnosis and molecular classification of lung cancer. Cancer Treat Res (2016) 170:25–46. doi: 10.1007/978-3-319-40389-2_2
5. Wadowska K, Bil-Lula I, Trembecki L, Sliwinska-Mosson M. Genetic markers in lung cancer diagnosis: a review. Int J Mol Sci (2020) 21:4569. doi: 10.3390/ijms21134569
6. Nagasaka M, Gadgeel SM. Role of chemotherapy and targeted therapy in early-stage non-small cell lung cancer. Expert Rev Anticancer Ther (2018) 18:63–70. doi: 10.1080/14737140.2018.1409624
7. Baxevanos P, Mountzios G. Novel chemotherapy regimens for advanced lung cancer: have we reached a plateau? Ann Transl Med (2018) 6:139. doi: 10.21037/atm.2018.04.04
8. Hirsch FR, Scagliotti GV, Mulshine JL, Kwon R, Curran WJ, Wu YL, et al. Lung cancer: current therapies and new targeted treatments. Lancet. (2017) 389:299–311. doi: 10.1016/S0140-6736(16)30958-8
9. Alexander M, Kim SY, Cheng H. Update 2020: management of non-small cell lung cancer. Lung. (2020) 198:897–907. doi: 10.1007/s00408-020-00407-5
10. Naylor EC, Desani JK, Chung PK. Targeted therapy and immunotherapy for lung cancer. Surg Oncol Clin N Am (2016) 25:601–9. doi: 10.1016/j.soc.2016.02.011
11. Lim ZF, Ma PC. Emerging insights of tumor heterogeneity and drug resistance mechanisms in lung cancer targeted therapy. J Hematol Oncol (2019) 12:134. doi: 10.1186/s13045-019-0818-2
12. Ben-David U, Siranosian B, Ha G, Tang H, Oren Y, Hinohara K, et al. Genetic and transcriptional evolution alters cancer cell line drug response. Nature. (2018) 560:325–30. doi: 10.1038/s41586-018-0409-3
13. Han K, Pierce SE, Li A, Spees K, Anderson GR, Seoane JA, et al. Crispr screens in cancer spheroids identify 3d growth-specific vulnerabilities. Nature. (2020) 580:136–41. doi: 10.1038/s41586-020-2099-x
14. Yoshida GJ. Applications of patient-derived tumor xenograft models and tumor organoids. J Hematol Oncol (2020) 13:4. doi: 10.1186/s13045-019-0829-z
15. Tentler JJ, Tan AC, Weekes CD, Jimeno A, Leong S, Pitts TM, et al. Patient-derived tumour xenografts as models for oncology drug development. Nat Rev Clin Oncol (2012) 9:338–50. doi: 10.1038/nrclinonc.2012.61
16. Lawson DA, Bhakta NR, Kessenbrock K, Prummel KD, Yu Y, Takai K, et al. Single-cell analysis reveals a stem-cell program in human metastatic breast cancer cells. Nature. (2015) 526:131–5. doi: 10.1038/nature15260
17. Reyal F, Guyader C, Decraene C, Lucchesi C, Auger N, Assayag F, et al. Molecular profiling of patient-derived breast cancer xenografts. Breast Cancer Res (2012) 14:R11. doi: 10.1186/bcr3095
18. Zhao X, Liu Z, Yu L, Zhang Y, Baxter P, Voicu H, et al. Global gene expression profiling confirms the molecular fidelity of primary tumor-based orthotopic xenograft mouse models of medulloblastoma. Neuro Oncol (2012) 14:574–83. doi: 10.1093/neuonc/nos061
19. Hidalgo M, Amant F, Biankin AV, Budinska E, Byrne AT, Caldas C, et al. Patient-derived xenograft models: an emerging platform for translational cancer research. Cancer Discovery (2014) 4:998–1013. doi: 10.1158/2159-8290.CD-14-0001
20. Li M, Izpisua BJ. Organoids - preclinical models of human disease. N Engl J Med (2019) 380:569–79. doi: 10.1056/NEJMra1806175
21. Lancaster MA, Knoblich JA. Organogenesis in a dish: modeling development and disease using organoid technologies. Science. (2014) 345:1247125. doi: 10.1126/science.1247125
22. Shi R, Radulovich N, Ng C, Liu N, Notsuda H, Cabanero M, et al. Organoid cultures as preclinical models of non-small cell lung cancer. Clin Cancer Res (2020) 26:1162–74. doi: 10.1158/1078-0432.CCR-19-1376
23. van de Wetering M, Francies HE, Francis JM, Bounova G, Iorio F, Pronk A, et al. Prospective derivation of a living organoid biobank of colorectal cancer patients. Cell. (2015) 161:933–45. doi: 10.1016/j.cell.2015.03.053
24. Xiao Y, Ma D, Yang YS, Yang F, Ding JH, Gong Y, et al. Comprehensive metabolomics expands precision medicine for triple-negative breast cancer. Cell Res (2022) 32:477–90. doi: 10.1038/s41422-022-00614-0
25. Yan H, Siu HC, Law S, Ho SL, Yue S, Tsui WY, et al. A comprehensive human gastric cancer organoid biobank captures tumor subtype heterogeneity and enables therapeutic screening. Cell Stem Cell (2018) 23:882–97. doi: 10.1016/j.stem.2018.09.016
26. Tuveson D, Clevers H. Cancer modeling meets human organoid technology. Science. (2019) 364:952–5. doi: 10.1126/science.aaw6985
27. Wensink GE, Elias SG, Mullenders J, Koopman M, Boj SF, Kranenburg OW, et al. Patient-derived organoids as a predictive biomarker for treatment response in cancer patients. NPJ Precis Oncol (2021) 5:30. doi: 10.1038/s41698-021-00168-1
28. Drost J, Clevers H. Organoids in cancer research. Nat Rev Cancer. (2018) 18:407–18. doi: 10.1038/s41568-018-0007-6
29. Weeber F, Ooft SN, Dijkstra KK, Voest EE. Tumor organoids as a pre-clinical cancer model for drug discovery. Cell Chem Biol (2017) 24:1092–100. doi: 10.1016/j.chembiol.2017.06.012
30. Zhan T, Rindtorff N, Betge J, Ebert MP, Boutros M. Crispr/cas9 for cancer research and therapy. Semin Cancer Biol (2019) 55:106–19. doi: 10.1016/j.semcancer.2018.04.001
31. Lee D, Kim Y, Chung C. Scientific validation and clinical application of lung cancer organoids. Cells-Basel. (2021) 10:3012. doi: 10.3390/cells10113012
32. Dijkstra KK, Monkhorst K, Schipper LJ, Hartemink KJ, Smit EF, Kaing S, et al. Challenges in establishing pure lung cancer organoids limit their utility for personalized medicine. Cell Rep (2020) 31:107588. doi: 10.1016/j.celrep.2020.107588
33. EbiSudani T, Hamamoto J, Togasaki K, Mitsuishi A, Sugihara K, Shinozaki T, et al. Genotype-phenotype mapping of a patient-derived lung cancer organoid biobank identifies nkx2-1-defined wnt dependency in lung adenocarcinoma. Cell Rep (2023) 42:112212. doi: 10.1016/j.celrep.2023.112212
34. Kim SY, Kim SM, Lim S, Lee JY, Choi SJ, Yang SD, et al. Modeling clinical responses to targeted therapies by patient-derived organoids of advanced lung adenocarcinoma. Clin Cancer Res (2021) 27:4397–409. doi: 10.1158/1078-0432.CCR-20-5026
35. Wang HM, Zhang CY, Peng KC, Chen ZX, Su JW, Li YF, et al. Using patient-derived organoids to predict locally advanced or metastatic lung cancer tumor response: a real-world study. Cell Rep Med (2023) 4:100911. doi: 10.1016/j.xcrm.2022.100911
36. Hu Y, Sui X, Song F, Li Y, Li K, Chen Z, et al. Lung cancer organoids analyzed on microwell arrays predict drug responses of patients within a week. Nat Commun (2021) 12:2581. doi: 10.1038/s41467-021-22676-1
37. Sachs N, Papaspyropoulos A, Zomer-van OD, Heo I, Bottinger L, Klay D, et al. Long-term expanding human airway organoids for disease modeling. EMBO J (2019) 38:e100300. doi: 10.15252/embj.2018100300
38. Kleinman HK, Martin GR. Matrigel: basement membrane matrix with biological activity. Semin Cancer Biol (2005) 15:378–86. doi: 10.1016/j.semcancer.2005.05.004
39. Kozlowski MT, Crook CJ, Ku HT. Towards organoid culture without matrigel. Commun Biol (2021) 4:1387. doi: 10.1038/s42003-021-02910-8
40. Hughes CS, Postovit LM, Lajoie GA. Matrigel: a complex protein mixture required for optimal growth of cell culture. Proteomics. (2010) 10:1886–90. doi: 10.1002/pmic.200900758
41. Dijkstra KK, Cattaneo CM, Weeber F, Chalabi M, van de Haar J, Fanchi LF, et al. Generation of tumor-reactive t cells by co-culture of peripheral blood lymphocytes and tumor organoids. Cell. (2018) 174:1586–98. doi: 10.1016/j.cell.2018.07.009
42. Ma P, Chen Y, Lai X, Zheng J, Ye E, Loh XJ, et al. The translational application of hydrogel for organoid technology: challenges and future perspectives. Macromol Biosci (2021) 21:e2100191. doi: 10.1002/mabi.202100191
43. Luo X, Fong E, Zhu C, Lin Q, Xiong M, Li A, et al. Hydrogel-based colorectal cancer organoid co-culture models. Acta Biomater. (2021) 132:461–72. doi: 10.1016/j.actbio.2020.12.037
44. Kim M, Mun H, Sung CO, Cho EJ, Jeon HJ, Chun SM, et al. Patient-derived lung cancer organoids as in vitro cancer models for therapeutic screening. Nat Commun (2019) 10:3991. doi: 10.1038/s41467-019-11867-6
45. Taverna JA, Hung CN, DeArmond DT, Chen M, Lin CL, Osmulski PA, et al. Single-cell proteomic profiling identifies combined axl and jak1 inhibition as a novel therapeutic strategy for lung cancer. Cancer Res (2020) 80:1551–63. doi: 10.1158/0008-5472.CAN-19-3183
46. Senkowski W, Gall-Mas L, Falco MM, Li Y, Lavikka K, Kriegbaum MC, et al. A platform for efficient establishment and drug-response profiling of high-grade serous ovarian cancer organoids. Dev Cell (2023) 58:1106–21. doi: 10.1016/j.devcel.2023.04.012
47. de Visser KE, Joyce JA. The evolving tumor microenvironment: from cancer initiation to metastatic outgrowth. Cancer Cell (2023) 41:374–403. doi: 10.1016/j.ccell.2023.02.016
48. Roma-Rodrigues C, Mendes R, Baptista PV, Fernandes AR. Targeting tumor microenvironment for cancer therapy. Int J Mol Sci (2019) 20:61–68. doi: 10.3390/ijms20040840
49. Wu T, Dai Y. Tumor microenvironment and therapeutic response. Cancer Lett (2017) 387:61–8. doi: 10.1016/j.canlet.2016.01.043
50. Xiao Y, Yu D. Tumor microenvironment as a therapeutic target in cancer. Pharmacol Ther (2021) 221:107753. doi: 10.1016/j.pharmthera.2020.107753
51. Mantovani A, Allavena P, Marchesi F, Garlanda C. Macrophages as tools and targets in cancer therapy. Nat Rev Drug Discovery (2022) 21:799–820. doi: 10.1038/s41573-022-00520-5
52. Takahashi N, Hoshi H, Higa A, Hiyama G, Tamura H, Ogawa M, et al. An in vitro system for evaluating molecular targeted drugs using lung patient-derived tumor organoids. Cells-Basel. (2019) 8:481. doi: 10.3390/cells8050481
53. Neal JT, Li X, Zhu J, Giangarra V, Grzeskowiak CL, Ju J, et al. Organoid modeling of the tumor immune microenvironment. Cell. (2018) 175:1972–88. doi: 10.1016/j.cell.2018.11.021
54. Patel SA, Nilsson MB, Le X, Cascone T, Jain RK, Heymach JV. Molecular mechanisms and future implications of vegf/vegfr in cancer therapy. Clin Cancer Res (2023) 29:30–9. doi: 10.1158/1078-0432.CCR-22-1366
55. Terrassoux L, Claux H, Bacari S, Meignan S, Furlan A. A bloody conspiracy- blood vessels and immune cells in the tumor microenvironment. Cancers (Basel). (2022) 14:4581. doi: 10.3390/cancers14194581
56. Seitlinger J, Nounsi A, Idoux-Gillet Y, Santos PE, Le H, Grandgirard E, et al. Vascularization of patient-derived tumoroid from non-small-cell lung cancer and its microenvironment. Biomedicines. (2022) 10:1103. doi: 10.3390/biomedicines10051103
57. Nashimoto Y, Mukomoto R, Imaizumi T, Terai T, Shishido S, Ino K, et al. Electrochemical sensing of oxygen metabolism for a three-dimensional cultured model with biomimetic vascular flow. Biosens Bioelectron. (2022) 219:114808. doi: 10.1016/j.bios.2022.114808
58. Choi YM, Lee H, Ann M, Song M, Rheey J, Jang J. 3d bioprinted vascularized lung cancer organoid models with underlying disease capable of more precise drug evaluation. Biofabrication. (2023) 15. doi: 10.1088/1758-5090/acd95f
59. Sen C, Koloff C, Kundu S, Wilkinson DC, Yang J, Shia DW, et al. Small cell lung cancer co-culture organoids provide insights into cancer cell survival after chemotherapy. Biorxiv (2023) 522668. doi: 10.1101/2023.01.03.522668
60. Chen S, Giannakou A, Wyman S, Gruzas J, Golas J, Zhong W, et al. Cancer-associated fibroblasts suppress sox2-induced dysplasia in a lung squamous cancer coculture. Proc Natl Acad Sci U S A. (2018) 115:E11671–80. doi: 10.1073/pnas.1803718115
61. Yokota E, Iwai M, Yukawa T, Yoshida M, Naomoto Y, Haisa M, et al. Clinical application of a lung cancer organoid (tumoroid) culture system. NPJ Precis Oncol (2021) 5:29. doi: 10.1038/s41698-021-00166-3
62. Lee EY, Muller WJ. Oncogenes and tumor suppressor genes. Cold Spring Harb Perspect Biol (2010) 2:a3236. doi: 10.1101/cshperspect.a003236
63. Naranjo S, Cabana CM, LaFave LM, Romero R, Shanahan SL, Bhutkar A, et al. Modeling diverse genetic subtypes of lung adenocarcinoma with a next-generation alveolar type 2 organoid platform. Genes Dev (2022) 36:936–49. doi: 10.1101/gad.349659.122
64. Miura A, Yamada D, Nakamura M, Tomida S, Shimizu D, Jiang Y, et al. Oncogenic potential of human pluripotent stem cell-derived lung organoids with her2 overexpression. Int J Cancer. (2021) 149:1593–604. doi: 10.1002/ijc.33713
65. Dost A, Moye AL, Vedaie M, Tran LM, Fung E, Heinze D, et al. Organoids model transcriptional hallmarks of oncogenic kras activation in lung epithelial progenitor cells. Cell Stem Cell (2020) 27:663–78. doi: 10.1016/j.stem.2020.07.022
66. Feng J, Li L, Tan Z, Zhao S, Zhang X, Wang J, et al. Lung adeno-squamous carcinoma modeling using oncogenic kras activation in human embryonic stem cell-derived alveolar organoids. Genes Dis (2023) 10:33–6. doi: 10.1016/j.gendis.2022.03.015
67. Tan AC, Tan D. Targeted therapies for lung cancer patients with oncogenic driver molecular alterations. J Clin Oncol (2022) 40:611–25. doi: 10.1200/JCO.21.01626
68. Ma X, Yang S, Jiang H, Wang Y, Xiang Z. Transcriptomic analysis of tumor tissues and organoids reveals the crucial genes regulating the proliferation of lung adenocarcinoma. J Transl Med (2021) 19:368. doi: 10.1186/s12967-021-03043-6
69. Banda M, McKim KL, Myers MB, Inoue M, Parsons BL. Outgrowth of erlotinib-resistant subpopulations recapitulated in patient-derived lung tumor spheroids and organoids. PloS One (2020) 15:e238862. doi: 10.1371/journal.pone.0238862
70. Villalobos P, Wistuba II. Lung cancer biomarkers. Hematol Oncol Clin North Am (2017) 31:13–29. doi: 10.1016/j.hoc.2016.08.006
71. Nadler E, Vasudevan A, Wang Y, Ogale S. Real-world patterns of biomarker testing and targeted therapy in de novo metastatic non-small cell lung cancer patients in the us oncology network. Cancer Treat Res Commun (2022) 31:100522. doi: 10.1016/j.ctarc.2022.100522
72. Malone ER, Oliva M, Sabatini P, Stockley TL, Siu LL. Molecular profiling for precision cancer therapies. Genome Med (2020) 12:8. doi: 10.1186/s13073-019-0703-1
73. Kern SE. Why your new cancer biomarker may never work: recurrent patterns and remarkable diversity in biomarker failures. Cancer Res (2012) 72:6097–101. doi: 10.1158/0008-5472.CAN-12-3232
74. Chetty R, Govender D. Gene of the month: kras. J Clin Pathol (2013) 66:548–50. doi: 10.1136/jclinpath-2013-201663
75. Pao W, Wang TY, Riely GJ, Miller VA, Pan Q, Ladanyi M, et al. Kras mutations and primary resistance of lung adenocarcinomas to gefitinib or erlotinib. PloS Med (2005) 2:e17. doi: 10.1371/journal.pmed.0020017
76. Slebos RJ, Kibbelaar RE, Dalesio O, Kooistra A, Stam J, Meijer CJ, et al. K-ras oncogene activation as a prognostic marker in adenocarcinoma of the lung. N Engl J Med (1990) 323:561–5. doi: 10.1056/NEJM199008303230902
77. Tyc KM, Kazi A, Ranjan A, Wang R, Sebti SM. Novel mutant kras addiction signature predicts response to the combination of erbb and mek inhibitors in lung and pancreatic cancers. Iscience. (2023) 26:106082. doi: 10.1016/j.isci.2023.106082
78. Luo L, Ma Y, Zheng Y, Su J, Huang G. Application progress of organoids in colorectal cancer. Front Cell Dev Biol (2022) 10:815067. doi: 10.3389/fcell.2022.815067
79. Vaishnavi A, Juan J, Jacob M, Stehn C, Gardner EE, Scherzer MT, et al. Transposon mutagenesis reveals rbms3 silencing as a promoter of Malignant progression of brafv600e-driven lung tumorigenesis. Cancer Res (2022) 82:4261–73. doi: 10.1158/0008-5472.CAN-21-3214
80. Guo L, Zhou W, Xu Z, Cao X, Wan S, Zhang YY, et al. Identification of irak1bp1 as a candidate prognostic factor in lung adenocarcinoma. Front Oncol (2023) 13:1132811. doi: 10.3389/fonc.2023.1132811
81. Peng KC, Su JW, Xie Z, Wang HM, Fang MM, Li WF, et al. Clinical outcomes of egfr+/metamp+ vs. Egfr+/metamp- untreated patients advanced non-small Cell Lung cancer. Thorac Cancer. (2022) 13:1619–30. doi: 10.1111/1759-7714.14429
82. Chae YK, Ranganath K, Hammerman PS, Vaklavas C, Mohindra N, Kalyan A, et al. Inhibition of the fibroblast growth factor receptor (fgfr) pathway: the current landscape and barriers to clinical application. Oncotarget. (2017) 8:16052–74. doi: 10.18632/oncotarget.14109
83. Nogova L, Sequist LV, Perez GJ, Andre F, Delord JP, Hidalgo M, et al. Evaluation of bgj398, a fibroblast growth factor receptor 1-3 kinase inhibitor, in patients with advanced solid tumors harboring genetic alterations in fibroblast growth factor receptors: results of a global phase i, dose-escalation and dose-expansion study. J Clin Oncol (2017) 35:157–65. doi: 10.1200/JCO.2016.67.2048
84. Xu H, Lyu X, Yi M, Zhao W, Song Y, Wu K. Organoid technology and applications in cancer research. J Hematol Oncol (2018) 11:116. doi: 10.1186/s13045-018-0662-9
85. Lin S, Li Y, Wang D, Huang C, Marino D, Bollt O, et al. Fascin promotes lung cancer growth and metastasis by enhancing glycolysis and pfkfb3 expression. Cancer Lett (2021) 518:230–42. doi: 10.1016/j.canlet.2021.07.025
86. Lackner MR, Wilson TR, Settleman J. Mechanisms of acquired resistance to targeted cancer therapies. Future Oncol (2012) 8:999–1014. doi: 10.2217/fon.12.86
87. Ye C, Deng Y, Sun W, Yuan Y. Editorial: recent advances of drug resistance research in colorectal cancer therapy. Front Oncol (2022) 12:1059223. doi: 10.3389/fonc.2022.1059223
88. Zhang JT, Liu Y. Use of comparative proteomics to identify potential resistance mechanisms in cancer treatment. Cancer Treat Rev (2007) 33:741–56. doi: 10.1016/j.ctrv.2007.07.018
89. Moiseenko F, Volkov N, Bogdanov A, Dubina M, Moiseyenko V. Resistance mechanisms to drug therapy in breast cancer and other solid tumors: an opinion. F1000Res. (2017) 6:288. doi: 10.12688/f1000research.10992.1
90. Han Y, Lee T, He Y, Raman R, Irizarry A, Martin ML, et al. The regulation of cd73 in non-small cell lung cancer. Eur J Cancer. (2022) 170:91–102. doi: 10.1016/j.ejca.2022.04.025
91. Yan R, Fan X, Xiao Z, Liu H, Huang X, Liu J, et al. Inhibition of dclk1 sensitizes resistant lung adenocarcinomas to egfr-tki through suppression of wnt/beta-catenin activity and cancer stemness. Cancer Lett (2022) 531:83–97. doi: 10.1016/j.canlet.2022.01.030
92. Wang SQ, Chen JJ, Jiang Y, Lei ZN, Ruan YC, Pan Y, et al. Targeting gstp1 as therapeutic strategy against lung adenocarcinoma stemness and resistance to tyrosine kinase inhibitors. Adv Sci (Weinh). (2023) 10:e2205262. doi: 10.1002/advs.202205262
93. Ni Y, Liu J, Zeng L, Yang Y, Liu L, Yao M, et al. Natural product manoalide promotes egfr-tki sensitivity of lung cancer cells by kras-erk pathway and mitochondrial ca(2+) overload-induced ferroptosis. Front Pharmacol (2022) 13:1109822. doi: 10.3389/fphar.2022.1109822
94. Galluzzi L, Senovilla L, Vitale I, Michels J, Martins I, Kepp O, et al. Molecular mechanisms of cisplatin resistance. Oncogene. (2012) 31:1869–83. doi: 10.1038/onc.2011.384
95. O’Grady S, Finn SP, Cuffe S, Richard DJ, O’Byrne KJ, Barr MP. The role of dna repair pathways in cisplatin resistant lung cancer. Cancer Treat Rev (2014) 40:1161–70. doi: 10.1016/j.ctrv.2014.10.003
96. Li H, Zhang Y, Lan X, Yu J, Yang C, Sun Z, et al. Halofuginone sensitizes lung cancer organoids to cisplatin via suppressing pi3k/akt and mapk signaling pathways. Front Cell Dev Biol (2021) 9:773048. doi: 10.3389/fcell.2021.773048
97. Weingart SN, Zhang L, Sweeney M, Hassett M. Chemotherapy medication errors. Lancet Oncol (2018) 19:e191–9. doi: 10.1016/S1470-2045(18)30094-9
98. Singh D, Attri BK, Gill RK, Bariwal J. Review on egfr inhibitors: critical updates. Mini Rev Med Chem (2016) 16:1134–66. doi: 10.2174/1389557516666160321114917
99. Roskoski RJ. Small molecule inhibitors targeting the egfr/erbb family of protein-tyrosine kinases in human cancers. Pharmacol Res (2019) 139:395–411. doi: 10.1016/j.phrs.2018.11.014
100. Hoshi H, Hiyama G, Ishikawa K, Inageda K, Fujimoto J, Wakamatsu A, et al. Construction of a novel cell-based assay for the evaluation of anti-egfr drug efficacy against egfr mutation. Oncol Rep (2017) 37:66–76. doi: 10.3892/or.2016.5227
101. Tian X, Gu T, Lee MH, Dong Z. Challenge and countermeasures for egfr targeted therapy in non-small cell lung cancer. Biochim Biophys Acta Rev Cancer. (2022) 1877:188645. doi: 10.1016/j.bbcan.2021.188645
102. Da CSG, Shepherd FA, Tsao MS. Egfr mutations and lung cancer. Annu Rev Pathol (2011) 6:49–69. doi: 10.1146/annurev-pathol-011110-130206
103. Pan Y, Cui H, Song Y. Organoid drug screening report for a non-small cell lung cancer patient with egfr gene mutation negativity: a case report and review of the literature. Front Oncol (2023) 13:1109274. doi: 10.3389/fonc.2023.1109274
104. Bie Y, Wang J, Xiong L, Wang D, Liao J, Zhang Y, et al. Lung adenocarcinoma organoids harboring egfr 19del and l643v double mutations respond to osimertinib and gefitinib: a case report. Med (Baltimore). (2021) 100:e24793. doi: 10.1097/MD.0000000000024793
105. Lei Y, Lei Y, Shi X, Wang J. Eml4-alk fusion gene in non-small cell lung cancer. Oncol Lett (2022) 24:277. doi: 10.3892/ol.2022.13397
106. Du X, Shao Y, Qin HF, Tai YH, Gao HJ. Alk-rearrangement in non-small-cell lung cancer (nsclc). Thorac Cancer. (2018) 9:423–30. doi: 10.1111/1759-7714.12613
107. Moore AR, Rosenberg SC, McCormick F, Malek S. Ras-targeted therapies: is the undruggable drugged? Nat Rev Drug Discovery (2020) 19:533–52. doi: 10.1038/s41573-020-0068-6
108. Veluswamy R, Mack PC, Houldsworth J, Elkhouly E, Hirsch FR. Kras g12c-mutant non-small cell lung cancer: biology, developmental therapeutics, and molecular testing. J Mol Diagn. (2021) 23:507–20. doi: 10.1016/j.jmoldx.2021.02.002
109. Chapman AM, Sun KY, Ruestow P, Cowan DM, Madl AK. Lung cancer mutation profile of egfr, alk, and kras: meta-analysis and comparison of never and ever smokers. Lung Cancer. (2016) 102:122–34. doi: 10.1016/j.lungcan.2016.10.010
110. Han J, Liu Y, Yang S, Wu X, Li H, Wang Q. Mek inhibitors for the treatment of non-small cell lung cancer. J Hematol Oncol (2021) 14:1. doi: 10.1186/s13045-020-01025-7
111. Wu SG, Shih JY. Management of acquired resistance to egfr tki-targeted therapy in advanced non-small cell lung cancer. Mol Cancer. (2018) 17:38. doi: 10.1186/s12943-018-0777-1
112. Passaro A, Janne PA, Mok T, Peters S. Overcoming therapy resistance in egfr-mutant lung cancer. Nat Cancer. (2021) 2:377–91. doi: 10.1038/s43018-021-00195-8
113. Panahi Y, Mohammadzadeh AH, Behnam B, Orafai HM, Jamialahmadi T. Sahebkar A. A Rev monoclonal antibody-based treatments non-small Cell Lung cancer. Adv Exp Med Biol (2021) 1286:49–64. doi: 10.1007/978-3-030-55035-6_3
114. Pirker R. Chemotherapy remains a cornerstone in the treatment of nonsmall cell lung cancer. Curr Opin Oncol (2020) 32:63–7. doi: 10.1097/CCO.0000000000000592
115. Driehuis E, Kretzschmar K, Clevers H. Establishment of patient-derived cancer organoids for drug-screening applications. Nat Protoc (2020) 15:3380–409. doi: 10.1038/s41596-020-0379-4
116. Bose S, Clevers H, Shen X. Promises and challenges of organoid-guided precision medicine. Med. (2021) 2:1011–26. doi: 10.1016/j.medj.2021.08.005
117. Zarrintaj P, Saeb MR, Stadler FJ, Yazdi MK, Nezhad MN, Mohebbi S, et al. Human organs-on-chips: a review of the state-of-the-art, current prospects, and future challenges. Adv Biol (Weinh). (2022) 6:e2000526. doi: 10.1002/adbi.202000526
118. Sontheimer-Phelps A, Hassell BA, Ingber DE. Modelling cancer in microfluidic human organs-on-chips. Nat Rev Cancer. (2019) 19:65–81. doi: 10.1038/s41568-018-0104-6
119. Jung DJ, Shin TH, Kim M, Sung CO, Jang SJ, Jeong GS. A one-stop microfluidic-based lung cancer organoid culture platform for testing drug sensitivity. Lab Chip. (2019) 19:2854–65. doi: 10.1039/c9lc00496c
120. Liu Q, Zhao T, Wang X, Chen Z, Hu Y, Chen X. In situ vitrification of lung cancer organoids on a microwell array. Micromachines (Basel). (2021) 12:624. doi: 10.3390/mi12060624
121. Goto H, Nishioka Y. Fibrocytes: a novel stromal cells to regulate resistance to anti-angiogenic therapy and cancer progression. Int J Mol Sci (2017) 19:98. doi: 10.3390/ijms19010098
122. Lambrechts D, Wauters E, Boeckx B, Aibar S, Nittner D, Burton O, et al. Phenotype molding of stromal cells in the lung tumor microenvironment. Nat Med (2018) 24:1277–89. doi: 10.1038/s41591-018-0096-5
123. Xu R, Zhou X, Wang S, Trinkle C. Tumor organoid models in precision medicine and investigating cancer-stromal interactions. Pharmacol Ther (2021) 218:107668. doi: 10.1016/j.pharmthera.2020.107668
124. Guo S, Deng CX. Effect of stromal cells in tumor microenvironment on metastasis initiation. Int J Biol Sci (2018) 14:2083–93. doi: 10.7150/ijbs.25720
125. Alkasalias T, Flaberg E, Kashuba V, Alexeyenko A, Pavlova T, Savchenko A, et al. Inhibition of tumor cell proliferation and motility by fibroblasts is both contact and soluble factor dependent. Proc Natl Acad Sci U S A. (2014) 111:17188–93. doi: 10.1073/pnas.1419554111
126. Clinton J, McWilliams-Koeppen P. Initiation, expansion, and cryopreservation of human primary tissue-derived normal and diseased organoids in embedded three-dimensional culture. Curr Protoc Cell Biol (2019) 82:e66. doi: 10.1002/cpcb.66
127. Yao Y, Xu X, Yang L, Zhu J, Wan J, Shen L, et al. Patient-derived organoids predict chemoradiation responses of locally advanced rectal cancer. Cell Stem Cell (2020) 26:17–26. doi: 10.1016/j.stem.2019.10.010
128. Schuster B, Junkin M, Kashaf SS, Romero-Calvo I, Kirby K, Matthews J, et al. Automated microfluidic platform for dynamic and combinatorial drug screening of tumor organoids. Nat Commun (2020) 11:5271. doi: 10.1038/s41467-020-19058-4
129. Zhang YS, Aleman J, Shin SR, Kilic T, Kim D, Mousavi SS, et al. Multisensor-integrated organs-on-chips platform for automated and continual in situ monitoring of organoid behaviors. Proc Natl Acad Sci U S A. (2017) 114:E2293–302. doi: 10.1073/pnas.1612906114
130. Trivedi P, Liu R, Bi H, Xu C, Rosenholm JM, Akerfelt M. 3d modeling of epithelial tumors-the synergy between materials engineering, 3d bioprinting, high-content imaging, and nanotechnology. Int J Mol Sci (2021) 22:6225. doi: 10.3390/ijms22126225
131. Ringel T, Frey N, Ringnalda F, Janjuha S, Cherkaoui S, Butz S, et al. Genome-scale crispr screening in human intestinal organoids identifies drivers of tgf-beta resistance. Cell Stem Cell (2020) 26:431–40. doi: 10.1016/j.stem.2020.02.007
132. Artegiani B, Hendriks D, Beumer J, Kok R, Zheng X, Joore I, et al. Fast and efficient generation of knock-in human organoids using homology-independent crispr-cas9 precision genome editing. Nat Cell Biol (2020) 22:321–31. doi: 10.1038/s41556-020-0472-5
133. Brandenberg N, Hoehnel S, Kuttler F, Homicsko K, Ceroni C, Ringel T, et al. High-throughput automated organoid culture via stem-cell aggregation in microcavity arrays. Nat BioMed Eng. (2020) 4:863–74. doi: 10.1038/s41551-020-0565-2
134. Beghin A, Grenci G, Sahni G, Guo S, Rajendiran H, Delaire T, et al. Automated high-speed 3d imaging of organoid cultures with multi-scale phenotypic quantification. Nat Methods (2022) 19:881–92. doi: 10.1038/s41592-022-01508-0
135. Abdul L, Rajasekar S, Lin D, Venkatasubramania RS, Sotra A, Feng Y, et al. Deep-lumen assay - human lung epithelial spheroid classification from brightfield images using deep learning. Lab Chip. (2020) 20:4623–31. doi: 10.1039/d0lc01010c
136. Matthews JM, Schuster B, Kashaf SS, Liu P, Ben-Yishay R, Ishay-Ronen D, et al. Organoid: a versatile deep learning platform for tracking and analysis of single-organoid dynamics. PloS Comput Biol (2022) 18:e1010584. doi: 10.1371/journal.pcbi.1010584
Keywords: lung cancer organoids, biobanks, drug screening, precision medicine, biomarker exploration
Citation: Liu Y, Zhou Y and Chen P (2023) Lung cancer organoids: models for preclinical research and precision medicine. Front. Oncol. 13:1293441. doi: 10.3389/fonc.2023.1293441
Received: 13 September 2023; Accepted: 27 September 2023;
Published: 24 October 2023.
Edited by:
Shoumin Zhu, University of Miami Health System, United StatesReviewed by:
Fang Li, University of Miami, United StatesLei Chen, University of Miami Health System, United States
Copyright © 2023 Liu, Zhou and Chen. This is an open-access article distributed under the terms of the Creative Commons Attribution License (CC BY). The use, distribution or reproduction in other forums is permitted, provided the original author(s) and the copyright owner(s) are credited and that the original publication in this journal is cited, in accordance with accepted academic practice. No use, distribution or reproduction is permitted which does not comply with these terms.
*Correspondence: Yanbing Zhou, emhvdXlhbmJpbmdAcWR1aG9zcGl0YWwuY24=; Pu Chen, cDRjaGVuQHV3YXRlcmxvby5jYQ==