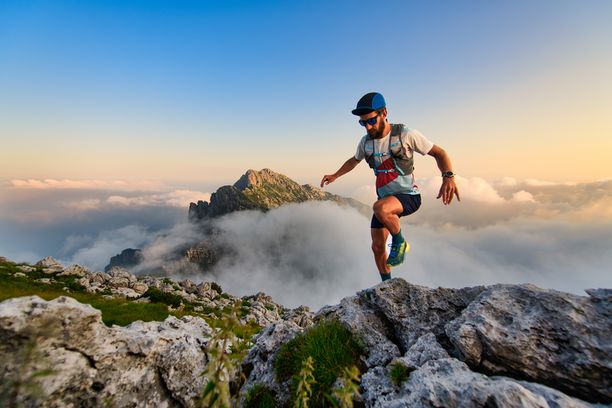
94% of researchers rate our articles as excellent or good
Learn more about the work of our research integrity team to safeguard the quality of each article we publish.
Find out more
REVIEW article
Front. Oncol., 19 December 2023
Sec. Molecular and Cellular Oncology
Volume 13 - 2023 | https://doi.org/10.3389/fonc.2023.1292046
This article is part of the Research TopicEvolutionary Biology of Tumor Initiation and ProgressionView all 12 articles
Breast cancer is the most common malignant neoplasm in women. Despite progress to date, 700,000 women worldwide died of this disease in 2020. Apparently, the prognostic markers currently used in the clinic are not sufficient to determine the most appropriate treatment. For this reason, great efforts have been made in recent years to identify new molecular biomarkers that will allow more precise and personalized therapeutic decisions in both primary and recurrent breast cancers. These molecular biomarkers include genetic and post-transcriptional alterations, changes in protein expression, as well as metabolic, immunological or microbial changes identified by multiple omics technologies (e.g., genomics, epigenomics, transcriptomics, proteomics, glycomics, metabolomics, lipidomics, immunomics and microbiomics). This review summarizes studies based on omics analysis that have identified new biomarkers for diagnosis, patient stratification, differentiation between stages of tumor development (initiation, progression, and metastasis/recurrence), and their relevance for treatment selection. Furthermore, this review highlights the importance of clinical trials based on multiomics studies and the need to advance in this direction in order to establish personalized therapies and prolong disease-free survival of these patients in the future.
According to GLOBOCAN 2020, female breast cancer (BC) is the most common type of cancer. In 2020, female BC accounted for nearly 700,000 cancer deaths and 2.3 million new cases worldwide (1). Breast cancer is highly heterogeneous, and its treatment classically depends on its clinical status (early stage, locally advanced or metastatic), histological characteristics and biomarker profile (2). Histologically, breast cancers can be classified as sarcomas or carcinomas depending on whether they originate from connective tissue or epithelial cells. In turn, carcinomas are classified as carcinomas in situ if they have not invaded other tissues, or as invasive carcinomas if they have invaded adjacent tissues or other organs of the body. Both in situ and invasive carcinomas are found in the lobules and ducts (lobular carcinoma in situ, LCIS; ductal carcinoma in situ, DCIS; invasive lobular carcinoma, ILC; and invasive ductal carcinoma, IDC) (2). The presence or absence of estrogen receptor (ER), progesterone receptor (PR), and human epidermal growth factor receptor 2 (HER2) molecularly determines breast cancer subtypes (3). Estrogen receptor positive (ER+) tumors require estrogen to subsist and grow. PR expression, in turn, is estrogen dependent. Therefore, ER+ and/or PR+ tumors are amenable to endocrine therapies targeting estrogen biosynthesis or estrogen receptors (4, 5). Likewise, HER2 amplification or overexpression determines the use of HER2-targeted therapy (6). Breast cancer can be classified into luminal A, luminal B, HER2-enriched and triple-negative breast cancer (TNBC), also known as basal-like. The luminal A subtype is characterized as ER+ and/or PR+ but HER2−, the luminal B subtype is ER+ and/or PR+/HER2+, the HER2-enriched subtype is characterized by overexpression of HER2 and ER−/PR−, and TNBC is negative for ER, PR and HER2 expression (2, 3, 7, 8). In addition, six TNBC subtypes have been identified: basal-like 1 (BL1), basal-like 2 (BL2), mesenchymal (M), mesenchymal stem-like (MSL), immunomodulatory (IM), and luminal androgen receptor (LAR) (9). In principle, the stratification of patients allows the establishment of treatments adapted to each subtype of breast cancer (2, 10) (Figure 1).
Figure 1 Schematic of the workflow performed in the review. Once the commonly accepted classifications of breast cancer have been described, the biomarkers identified by different omics techniques are described.
Despite efforts, 700,000 women died from breast cancer in 2020 (1). Apparently, prognostic markers currently used in the clinic, such as ER and HER2 status, histologic subtype, size, lymph node status and Nottingham grade, as well as current predictive tests such as germline BRCA status, tumor PIK3CA mutation status and programmed death-ligand 1 (PDL-1) status, are not sufficient to determine the most appropriate treatment (11). Relapses are the main obstacle faced by clinicians and are mainly due to the development of resistance to the drugs administered. However, there are still insufficient data to determine whether resistance develops after exposure to the drug or whether resistant cells are present from the onset of the disease, preceding antineoplastic treatments (12). This has prompted the search for biomarkers related to different characteristics of breast cancers, such as genetic alterations, epigenetic reprogramming, tumor-promoting inflammation and immune evasion, dysregulation of cell metabolism, or changes in the microbiota, among others (13, 14). Over the past 50 years, efforts have been made to identify genetic and post-transcriptional alterations, changes in protein expression, and more recently, metabolic changes and even immunological or microbial changes. These changes can be detected at the single molecule or pathway level and can serve as markers for diagnosis and/or discovery of personalized therapies. The detection of these modifications is made possible by technologies known as “omics”, such as genomics, transcriptomics, proteomics or metabolomics, or more specific ones such as epigenomics, glycomics or lipidomics, among others. The identification of new biomarkers might allow more precise, and personalized therapeutic decisions in both primary and recurrent cancers (Figure 1).
Genomics collectively characterizes and quantifies all the genes of an organism. Genomic analysis includes techniques such as microarrays, gene expression profiling, serial analysis of gene expression (SAGE), comparative genomic hybridization (CGH), array-based CGH (array-CGH), whole genome amplification (WGA), and high-throughput sequencing or next-generation sequencing (NGS). Different genomic studies have focused on the search for markers with predictive or prognostic value, for patient stratification and for determining the appropriate therapy (15, 16). Transcriptomics studies the set of all RNA transcripts of an organism. The most commonly used techniques to study the transcriptome are microarrays and RNA sequencing (RNA-seq) using high-throughput sequencing or NGS. Transcriptomic analysis allows the measurement of differential gene expression, often presented as hierarchical clustering (17, 18). Proteomics detects and quantifies the presence of proteins produced or modified by an organism. Proteomic analysis is performed by using separation techniques (gas chromatography, liquid chromatography, ultra-high performance liquid chromatography, capillary electrophoresis) combined with mass spectrometry (MS, resulting in GC-MS, LC-MS, UPLC-MS, CE-MS, respectively), nuclear magnetic resonance spectroscopy (NMR), reverse phase protein arrays (RPPA), and sequential windowed acquisition of all theoretical fragment ion mass spectra (SWATH-MS) (19). Glycomics provides insight into the biological significance of N-glycosylation of plasma proteins in cancer (20). Metabolomics approaches based on NMR, LC-MS, GC-MS and desorption electrospray ionization mass spectrometry imaging (DESI-MSI) enable the linking of genotype and phenotype thanks to the knowledge generated by dynamic metabolism (21, 22). Epigenomics (study of gene modifications through the aggregation of chemical compounds, with no changes in the DNA sequence), lipidomics (study of lipids in the biological system), and microbiomics (study of microorganisms present in the human body) are branches that use some of the mentioned above.
Due to the complexity that governs carcinogenesis and tumor progression, recent years have seen efforts to integrate data from different omics into a computational approach that allows for more complex reconstruction of biochemical connections (23). This review aims to summarize the existing knowledge on the advances made thanks to omics studies in human breast cancer. In particular, we focus on 1) the discovery of markers that can be used for diagnosis, 2) molecular and/or signaling pathway alterations in onset, progression, and metastasis, and 3) resistance to therapies and attempts to establish personalized treatments (Figure 1).
A cancer biomarker, found in tissues or body fluids, is used to detect the presence of cancer (differences between tumor and healthy samples or between tumor subtypes) and provides information on prognosis/prediction, cancer progression, and cancer medicine/treatment guidance, among others (24). In this review, we provide an overview of cancer-specific molecules and/or pathways that have been identified as biomarkers in breast cancer using different omics technologies.
One of the first uses of omics has been to identify biomarkers that differentiate tumor tissue from healthy tissue, as well as to identify biomarkers specific to different subtypes of breast cancer. In addition, the last decade has seen efforts to develop minimally invasive techniques to predict breast cancer subtypes, such as identifying biomarkers in human plasma, saliva, and urine.
The presence, copy number alterations, mutations, or amplifications of various genes are markers of breast cancer in tissue. Table 1 summarizes the most commonly mutated genes (27, 44). Copy number alterations have been observed in PIK3CA, ERBB2, TP53, MAP2K4, MLL3, CDKN2A, PTEN, and RB1 (44). Approximately 35-40% of primary breast cancers harbor mutations in TP53 and PIK3CA, as well as amplification of ERBB2, FGFR1, and CCND (~15%), and mutations in MUC16, AHNAK2, SYNE1, KMT2C, AKT1, and GATA3 genes (10%) (25, 45). In 2012, the Cancer Genome Atlas Network (CGAN) identified novel significantly mutated genes (SMGs), including TBX3, RUNX1, CBFB, AFF2, PIK3R1, PTPN22, PTPRD, NF1, SF3B1, and CCND3 (26).
A proteomic analysis has identified canonical up- and downregulated pathways in breast cancer. Pathways upregulated in breast cancer include glycolysis; metabolic pathways such as pyruvate fatty acid, arginine and proline, and valine, leucine and isoleucine catabolism; protein ubiquitination; RhoA, PI3K/AKT, ILK, 14-3-3-mediated, RAN, aryl hydrocarbon receptor, integrin, clathrin-mediated endocytosis, IGF-1, VEGF, EIF2, actin cytoskeleton, ERK5, GABA receptor, and HER-2 signaling pathways; NRF2-mediated response to oxidative stress; Rho-mediated regulation of actin-based motility; and LPS/IL-1-mediated inhibition of RXR function. Downregulated pathways include the citrate cycle, acute phase response signaling, P53 signaling, primary immunodeficiency signaling, urea cycle and amino group metabolism, Cdc42 signaling, glyoxylate and dicarboxylate metabolism, and autoimmune thyroid disease signaling (46).
A fucosylated triantennary glycan containing three α2-3 sialic acids (also called H6N5F1L3) and a non-fucosylated triantennary glycan containing a combination of α2-3 and α2-6 sialic acids (H6N5L2E1) are found at lower levels in breast cancer patient samples (28), although other studies have reported conflicting data for H6N5F1L3 (47). In addition, a fucosylated tetraantennary glycan containing a combination of α2-3 and α2-6 sialic acids (H7N6F1L1E3) is significantly elevated in breast cancer patients (28). Similarly, elevated levels of other trisialylated triantennary fucosylated glycans (termed H6N5F1S3, consisting of H6N5F1E3, H6N5F1L3, H6N5F1L2E1, H6N5F1L2E1, H6N5F1L1E2, and H6N5F1E3) have been observed (28, 30). Glycomic analysis have identified seven glycosylated proteins with O-linked β-D-N-acetylglucosamine (O-GlcNAc), an important post-translational modification involving reversible and highly dynamic covalent binding of β-N-GlcNAc to Ser/Thr residues in proteins (48), such as vimentin, keratin 7, enolase 2, pyruvate kinase isozyme M 2 (PKM2), protein disulfide isomerase (PDI) A6, TP, and voltage-dependent anion selective channel protein in breast cancer (31) (Table 1).
Eight pathways have been implicated in breast cancer: protein digestion and absorption, central carbon metabolism in cancer, neuroactive ligand receptor interaction, ABC transporters, mineral uptake, inositol phosphate metabolism, glutathione metabolism, and cysteine and methionine metabolism (49). A combinatorial study of metabolomic and proteomic profiles in human plasma samples has identified metabolic signatures for breast cancer diagnosis. The most abundant metabolites in breast cancer patients include mainly primary and secondary compounds of bile acid metabolism, compounds of fructose, mannose, galactose, tyrosine and glycerolipid metabolism. Critical metabolic pathways in breast cancer include alanine, aspartate and glutamate pathways, glutamine and glutamate metabolic pathways, and arginine biosynthesis pathways. The specific metabolites are listed in Table 1. In addition, thanks to the combined study with the proteome, aspartate aminotransferase (GOT1), L-lactate dehydrogenase B-chain (LDHB), glutathione synthetase (GSS) and glutathione peroxidase 3 (GPX3) have been found to be closely involved in these metabolic pathways (34).
On the other hand, metabolomic analysis of polyamines in saliva before and after surgical treatment has shown a decrease in N1-Ac-SPD and an increase in N8-Ac-SPD in patients after surgical treatment. Thus, the ratio (N8-Ac-SPD)/(N1-Ac-SPD+N8-Ac-SPD) could be an index of health status after surgical treatment (32) (Table 1). A metabolomic study of urine and breast tissue samples has identified dysregulation of lactate, valine, aspartate and glutamine pathways in breast cancer. In addition, five metabolites (acetone, 3-hexanone, 4-heptanone, 2-methyl-5-(methylthio)-furan, and acetate) allow correlation between urine and tissue samples (33). Inositol triphosphate receptor (IP3R) type 2 and 3 expression is increased in breast tumor tissue compared to adjacent healthy tissue. Increased lipoproteins, increased levels of metabolites such as lactate, lysine and alanine, and decreased levels of pyruvate and glucose in the serum of patients with high IP3R expression compared to healthy individuals (35).
The combination of metabolic profiling with tissue protein expression increases the accuracy in characterizing breast cancer patients (35). Lipidomic analyses have shown that the levels of sphingosine-1-phosphate (S1P), ceramides, and other sphingolipids are significantly higher in breast tumors than in normal breast tissue (36). In addition, sphingomyelin phosphodiesterase (SMase), which converts sphingomyelin to ceramide phosphate, is downregulated in 60% of breast tumors (37). An increase in fatty acids such as palmitate-containing phosphatidylcholines (PC) has also been found, especially in ER− and grade 3 tumors compared to healthy breast tissue. Phospholipids may have diagnostic potential as they have been associated with cancer progression and patient survival (38). When plasma samples from breast cancer patients and healthy controls were compared, significantly lower levels of lysophosphatidylcholines (LysoPC) and higher levels of sphingomyelins have been observed in plasma samples from cancer patients. In addition, three metabolites (LysoPC a C16:0, PC ae C42:5 and PC aa C34:2) differentiate breast cancer patients from healthy controls (39) (Table 1).
Lastly, the bacteria found on the skin have direct access to the breast ducts through the nipple, so the breast tissue contains a wide variety of bacteria, such as Staphylococcus epidermidis and Micrococcus luteus (50, 51). Different species of bacteria perform different functions. For example, Lactobacillus triggers protective mechanisms that include immune activation, competitive inhibition of pathogenic strains, and synthesis of signaling intermediates. In contrast, E. coli and Staphylococcus induce DNA damage (40). Changes in the microbiota of breast and intestinal tissues have been associated with the development of breast cancer (50). Several studies even describe correlations between dysbiosis of the tissue microbiome and the development of breast cancer. When characterizing the microbiome of tumor tissue and adjacent non-tumor tissue from different patients, a higher abundance of taxa belonging to the phylum Actinobacteria was observed in the non-tumor samples. In contrast, Firmicutes and Alpha-Proteobacteria were significantly overrepresented in tumor tissue (52). Healthy individuals show a significantly higher abundance of Lactobacillus, Thermoanaerobacterium thermosaccharolyticum, Candidatus Aquiluna sp., IMCC13023, Anoxybacillus, Leuconostoc, Lactococcus, Geobacillus, Methylobacterium, Turicella otitidis (40), Prevotella, Lactococcus, Streptococcus, Corynebacterium and Micrococcus (41). In cancer patients, there is an abundance of Thermus scotoductus, E. coli, Bacillus cereus, Shewanella, Corynbacterium (40), Bacillus, Staphylococcus, Enterobacteriaceae, Comamondaceae, Bacteroidetes (41), Mycobacterium fortuitum, and Mycobacterium phlei (42). The breast tissue microbiome of women with enrichment in lower abundance taxa, including the genera Fusobacterium, Atopobium, Gluconacetobacter, Hydrogenophaga and Lactobacillus, compared to that of women with benign breast disease (43) (Table 1).
Genomic alterations have been found in the different molecular subtypes of primary breast cancer. In general, approximately 5-10% of breast cancers are hereditary, mostly due to pathogenic variants or mutations in the BRCA1 and BRCA2 genes (53). Mutations in BRCA1 are associated with ER− and PR− tumors (54, 55), while mutations in BRCA2 are associated with ER+ and PR+ tumors (56). SMGs are more diverse and recurrent in both luminal subtypes, particularly in luminal A (26), and the heat shock protein (HSP) family has been specifically associated with different cancer types (18). In the luminal subtype A, the most common SMG is PIK3CA (45%), followed by MAP3K1, GATA3, TP53, CDH1, and MAP2K4 (26). Copy number gains of CCND1, FGF3, and FGFR1 have also been identified (25). The heat shock protein (HSP) genes DNAJB4, DNAJC18, HSPA12A, HSPA12B, HSPB2, HSPB6, HSPB7, CRYAB, and SACS are downregulated, whereas DNAJC5B, DNAJB13, DNAJC1, DNAJC22, HSPB1, HSPA6, and DNAJC12 are upregulated (18). The luminal subtype B is characterized by TP53 and PIK3CA SMGs (29% each) (26), as well as increased copy number of CCND1, FGF3 and FGFR1 (25); downregulation of HSP genes such as DNAJB4, DNAJC18, HSPA12A, HSPA12B, HSPB2, HSPB6, HSPB7, CRYAB and SACS and upregulation of DNAJC5B, DNAJB13, DNAJC1, DNAJC22, HSPB1, HSPA6, CCT5, CCT3, HSPE1, DNAJC9, HSPD1, DNAJC12, DNAJA4, HSPH1, CCT2, and DNAJA3 (18). The HER2-enriched subtype presents with HER2/ERBB2 amplification (80%), high frequency of mutations in TP53 (72%) and PIK3CA (39%) (26). PTEN and INPP4B have also been identified as genes of interest. Deletions in PPP2R2A, MTAP, and MAP2K4 genes have been reported (45). Among the HSP genes, CRYAB, SACS, DNAJB4, DNAJC18, HSPA12A, HSPA12B, HSPB2, HSPB6, HSPB7, HSPB8, DNAJC5G and BBS12 are downregulated, while the upregulated genes are DNAJC5B, DNAJB13, DNAJC1, DNAJC22, HSPB1, CCT5, CCT3, HSPE1, DNAJC9, HSPD1, DNAJA4, HSPH1, HYOU1, DNAJB11, CCT6A and DNAJB3 (18). Finally, mutations in TP53 are observed in 80% of TNBC cases, followed by alterations in PIK3R1 and NF1 (26). INPP4B is another gene of interest in TNBC, and deletions in PPP2R2A, MTAP and MAP2K4 genes have also been reported (45). Among the downregulated HSP genes in TNBC, we found DNAJB4, DNAJC18, HSPA12A, HSPA12B, HSPB2, HSPB6, HSPB7, HSPB8, DNAJC12 and DNAJC27. As for the upregulated genes, DNAJC5B, HSPA6, CCT5, CCT3, HSPE1, DNAJC9, HSPD1, HYOU1, DNAJB11, CCT6A, HSPA5, HSPA14, CRYAA, DNAJC2 and DNAJC6 were found (18) (Table 2).
MicroRNAs (miRNAs) are small non-coding RNAs that modulate gene expression to regulate various cellular processes, including those involved in breast cancer (66, 67). miR-206 is highly expressed in ER− tumors and also targets the ERα receptor, as do miR-221 and miR-222 (57). In addition, DCIS and LCIS are characterized by the upregulated expression of several miRNAs, which are listed in Table 2 (57).
Through an integrated study combining genomics and epigenomics, pathways unique to TNBC and non-TNBC were identified. The most significant pathways for TNBC are retinal biosynthesis, BAG2, LXR/RXR, EIF2, and P2Y purinergic receptor signaling pathways, whereas in non-TNBC they are UVB-induced MAPK, PCP, endothelial apelin, endoplasmic reticulum stress, and host viral egress mechanisms (68). Based on the genomic and transcriptomic landscape, Xie et al. have established a new classification of immune subtypes of ER+/PR−/HER2− breast cancer, termed clusters 1 to 5. Cluster 1 is characterized by an activated but suppressive immune microenvironment, immune infiltration, increased stromal content, and an elevated TGF-β response signature. Cluster 2 has an inactivated immune phenotype. Cluster 3 has an activated immune phenotype enriched in innate, adaptive, and immunosuppressive cells, as well as interferon (IFN)-γ response, inflammation, macrophage upregulation, and cytolytic signatures. Cluster 4 is characterized by an immunologically inactive phenotype and low infiltration of the microenvironment. Finally, cluster 5 lacks immunologic properties but presents a phenotype associated with hormonal responses (69) (Table 2).
Several studies have investigated the classification of breast cancers using proteomic technologies. By analyzing differential protein expression in tissues, they identified the expression of proteins that characterize luminal subtypes, HER2-enriched breast cancers, and TNBC. In addition, proteomic profiling of these three breast cancer types revealed functional differences. Cytoskeletal remodeling as well as alterations in the cell adhesion process are found in all BC types. Luminal tumors are characterized by increased “energy metabolism” as indicated by an elevated expression of key proteins in gluconeogenesis, electron transport chain and ATP synthase complex. “Immune response” is altered in both luminal A and luminal B subtypes, while “cell cycle regulation” is important in luminal B tumors. On the other hand, luminal tumors show decreased expression of proteins related to metabolic pathways, including glycolysis, serine synthesis, and glutamine consumption. The HER2-enriched subtype is characterized by decreased “amino acid and energy metabolism”, reduced “cellular community” and increased “glycan biosynthesis and metabolism”. Finally, the TNBC subtype is characterized by increased “replication and repair”, “cell growth and death” and “translation” pathways. Other relevant processes are “immune response” and “blood coagulation”. The most relevant proteins involved in these pathways are listed in Table 2 (58, 59, 70). Besides that, the expression of the protein enhancer of zeste homolog 2 (EZH2) is elevated in premalignant atypical ductal hyperplasia (ADH) and even higher in DCIS compared to normal epithelium (15).
Luminal tumors have been classified into 3 proteomic clusters. Luminal cluster-1 is enriched for RNA processing and splicing processes but depleted for immune-related proteins including the ones involved in antigen processing and presentation, and type I and type II IFN signaling. Luminal cluster-2 is enriched for stromal proteins and extracellular matrix (ECM) components. Luminal cluster-3 has high expression of proteins for DNA replication, cell cycle, response to DNA damage, and immune response, while depleted for ECM components, blood coagulation, epithelial cell differentiation, and response to estrogen and steroid hormones compared to luminal clusters-1 and -2. In addition, significantly higher expression of Ki67 is found in luminal cluster-3 compared to luminal cluster-1 and -2 (71). On the other hand, 4 TNBC subgroups have been identified according to 4 proteomic clusters. TNBC cluster-1 has the most favorable survival and is characterized by immune response, antigen processing and presentation, and IFN type I and II signaling processes. TNBC cluster-2 has intermediate survival and is enriched for ECM components, coagulation, and humoral immune response processes. TNBC cluster-3 has intermediate survival and is enriched for lipid metabolism, catabolism, and oxidation-reduction processes. TNBC cluster-4 exhibits the poorest survival and is enriched for DNA replication and cell cycle proteins (71).
A comprehensive genomic, transcriptomic and proteomic analysis of patients with ILC has identified mutations in cadherin-1 (CDH1) and the phosphatidylinositol 3- kinase (PI3K) pathway as the most common molecular alterations in ILC. In addition, two major subtypes of ILC have been identified: an immune-related (IR) subtype and a hormone-related (HR) subtype: The IR subtype is characterized by upregulation of PD-L1 mRNA, PD-1 and CTLA-4, increased sensitivity to DNA damaging agents, and upregulation of lymphoid signaling molecules at the mRNA level (TGFBR2, IL11RA, TNFRSF17, CCL15, CCL14, CCR2, CD27, XCL2, IFNAR2, and CD40LG). In addition, the IR subtype shows upregulated genes in the cytokine-cytokine receptor interaction pathway, suggesting alterations in the composition or functional activity of immune cells within these tumors. Interestingly, the negative regulators of the immune response PDCD1 (PD-1), CD274 (PD-L1) and CTLA4 are expressed at higher mRNA levels in the IR subtype. The related HR subtype is associated with epithelial-to-mesenchymal transition (EMT). Moreover, the HR subtype shows higher levels of estrogen receptors (ESR1) and progesterone receptors (PGR) and upregulation of cell cycle genes and estrogen receptor (ER) target genes (60) (Table 2).
Differential metabolites have been identified when comparing HER2+ with HER2− patients, as well as when comparing ER+ with ER− patients. Plasma samples from HER2+ patients are characterized by increased aerobic glycolysis, gluconeogenesis and fatty acid biosynthesis and decreased Krebs cycle. Specifically, HER2+ is characterized by overexpression of carnitine, LysoPC (20:4), proline, valine, and 2-octenedioic acid. Strong metabolic differences correlate with hormone receptor status. Plasma samples from ER+ patients reflect increased alanine, aspartate, and glutamate metabolism, decreased glycerolipid catabolism and increased purine metabolism. ER+ is characterized by overexpression of glycochenodeoxycholic acid and decreased expression of alanine, LysoPC (16:1), valine, and 2-octenedioic acid. Many glycolytic and glycogenolytic intermediates, components of the glutathione (GSH) pathway, the oncometabolite 2-hydroxyglutarate (2-HG), and the immunomodulatory tryptophan metabolite kynurenine are elevated in ER− compared to ER+ cancers (72, 73). Using deep learning techniques, metabolites, and pathways have been identified that can discriminate between ER+ and ER− patient samples. Among the identified metabolites, five have been proposed as breast cancer biomarkers: β-alanine, xanthine, isoleucine, glutamate, and taurine (49). Studies performed on plasma samples from breast cancer patients have allowed the identification of specific metabolomic profiles for each cellular subtype: L-tryptophan, LysoPC(14:0), glycoursodeoxycholic acid, lysophosphoethanolamine (LysoPE)(18:2) in luminal A; LysoPE(18:2), LysoPE(18:1(11Z/9Z)), LysoPC(20: 3), biliverdin, LysoPE(16:0) in luminal B; LysoPE(18:1(11Z)/9Z), LysoPC(0:0/16:0), biliverdin, L-tryptophan, LysoPE(18:2) in HER2+; and L-tryptophan, LysoPC(16:0/0:0), LysoPE(18:1(11Z)/9Z) in TNBC (62) Table 2. When plasma samples from patients with mutated BRCA1 and non-mutated BRCA1 were compared, the levels of adenine, N6-methyladenosine, and 1-methylguanine were found to be significantly lower in patients with BRCA1 mutations (74).
The lipidomic profiles of invasive breast cancer (IBC), DCIS and benign surrounding tissue (BAT) have been investigated. IBC is characterized by the presence of polyunsaturated fatty acids, deprotonated glycerophospholipids and sphingolipids. IBC can be distinguished from BAT by the presence of highly saturated lipids and antioxidant molecules. DCIS differs from IBC by lipids associated with cell signaling and apoptosis (61). Lipidomics have allowed the identification of glycerol-3-phosphate acyltransferase (GPAM), an enzyme involved in triacylglycerol and phospholipid biosynthesis, which shows increased cytoplasmic expression in HR-associated breast cancer and improved OS (63) (Table 2).
Finally, Benarjee et al. have identified a local microbial signature associated with each type of breast tumor. Actinomyces, Bartonella, Brevundimonas, Coxiella, Mobiluncus, Mycobacterium, Rickettsia, and Sphingomonas are common in all types of breast cancer. In the luminal A subtype, Arcanobacterium, Bifidobacterium, Cardiobacterium, Citrobacter, and Escherichia are detected. Bordetella, Campylobacter, Chlamydia, Chlamydophila, Legionella, and Pasteurella are associated with the luminal B subtype. The HER2-enriched subtype is characterized by the presence of Streptococcus, whereas Aerococcus, Arcobacter, Geobacillus, Orientia, and Rothia are associated to with TNBC (65) (Table 2).
The various omics technologies have allowed the identification of prognostic biomarkers in both solid and liquid samples. Gene expression analysis have identified genes associated with good and poor prognosis in breast cancer (18). In addition, using data from breast cancer databases (TCGA-BRCA and CMI-MBC), a 45-gene optimal prognostic gene signature has been constructed from genes regulated by tumor-associated macrophages (TAMs). All these genes are listed in Table 3 (75).
Not only genomic but also epigenomic differences have been found. By applying a powerful integrative network algorithm to paired DNA methylation and RNA-Seq data from ER+ breast cancer and adjacent healthy tissue, it has been shown that increased levels of DNA methylation and alterations in mRNA expression can predict poor prognosis. In particular, epigenetic silencing of WNT signaling antagonists and bone morphogenetic proteins (BMPs) has been observed in both luminal subtypes, but predominantly in luminal B breast cancer (76). Davalos et al. identified hypermethylation of RASSF1A and PITX2 associated with poor prognosis in early stage of breast cancer (77).
Various proteomic analyses have validated proteins related to apoptosis, cell cycle arrest, cell adhesion, cytokeratins, cell metabolism and lipid binding as prognostic biomarkers of breast cancer OS (78, 79). Elevated expression of serine hydroxymethyltransferase 2 (SHMT2) correlates with poor OS and relapse-free survival (RFS), the amino acid transporter ASCT2 (SLC1A5) correlates with poor RFS (80), endoplasmin (HSP90B1) has been associated with distant metastasis and worse OS, and decorin (DCN) has been associated with lymph node metastasis, increased number of positive lymph nodes and worse OS; and (81). Finally, increased levels of E-cadherin and β-catenin correlate with poor survival in invasive breast cancer but not in lobular carcinoma (82) (Table 3).
An analysis of breast cancer transcriptomic and proteomic data from the Clinical Proteomic Tumor Analysis Consortium (CPTAC) resource has identified 2 candidates associated with survival: Fms-related receptor tyrosine kinase 1 (FLT1) in TNBC, Fas-associated death domain (FADD) protein with the luminal type, while 8 candidates: Fructose-bisphosphate aldolase A (ALDOA), C-X-C motif chemokine (CXCL)16, fibroblast growth factor receptor 1 (FGFR1), 1-phosphatidylinositol 4,5-bisphosphate phosphodiesterase beta-3 (PLCB3), serine/threonine protein phosphatase 2A 55 kDa regulatory subunit B alpha isoform (PPP2R2A), and replication protein A1 (RPA1) are clearly associated with poor survival in the HER2-enriched type (83). Of note, protein glycosylation correlates with increased tumor burden and poor prognosis in breast cancer (84) (Table 3).
Early-stage breast cancer lesions are so small that there may be insufficient material for analysis and it is difficult to obtain accurate data.
The onset of breast cancer is characterized by abnormal paracrine and autocrine signaling, as genes that are highly expressed in healthy breast epithelium are lost in carcinomas, including genes encoding cytokines such as LIF, IL-6, and HIN-1, also known as SCGB3A1, and chemokines such as IL-8, GROα, GROβ, and MIP3α, also known as CCL20 (85). In addition, genes silenced by hypermethylation have been identified as responsible for mammary carcinogenesis, including TWIST, RASSF1A, CCND2, HIN1, BRCA1, APC, GSTP1, BIN1, BMP6, ESR2, CDKN2A, CDKN1A, TIMP3 and CST6, as well as the WNT-negative regulators WIF1 and DKK3 (77). In addition, two methylated modifications (H3K9 me2 and me3) of the DNA packaging protein histone H3 decrease during cancer transformation, and the demethylase KDM3A/JMJD1A gradually increases (86). On the other hand, methylated genes such as ITIH5, DKK3, RASSF1A, SFN, CDKN2A, MLH1, HOXD13 and PCDHGB7 have been proposed as potential markers for early detection of breast cancer. Hypermethylation of RASSF1A, CCDN2, HIN1 and APC correlates mainly with HR+ breast cancer, whereas hypermethylation of CDH1 and CDH13 is more frequent in TNBC patients (77). Furthermore, the expression of olfactomedin-4 (OLFM4) is higher in non-invasive breast tumors than in invasive breast cancer. Therefore, OLFM4 may also be a biomarker for early breast cancer (87) (Figure 2A).
Figure 2 Biomarkers of breast cancer at different time points of tumor development detected by omics. Summary of markers observed in breast cancer onset (A), progression (B) and metastasis/relapse (C) detected by genomic, transcriptomic, proteomic, metabolomics, lipidomic and/or microbiomic. 87 immune gene signature includes LAG3, RELB, CCL2, IFNG, MSH6, ZC3HAV1, CD68, ORM1, LYZ, USP14, SLA2, HERC5, LAMP3, NONO, BATF, FCER1G, CCR5, REL, DTX3L, HMGB2, C2, CLEC4E, CLEC4D, CLEC7A, IL12A, CXCL10, CXCL11, RASGRP1, HAVCR2, ICOS, ATRIP, TRIM25, RNF166, CCR8, CSF1, NFAM1, TUBB4B, LYAR, CLEC12A, IL27, PIK3CG, XRCC6, PARP9, DNAJC5, MPEG1, TIFA, TLR1, CD47, EXO1, NCF2, SLAMF7, CTSS, GBP5, GBP4, GBP1, CREG1, RNF19B, RC3H2, RAB14, SYK, ACTR2, KCNAB2, OPTN, DDX58, IL2RA, JAK2, CLEC6A, LYST, CCL25, CCL8, HLADRA, RAB27A, PTK2B, PDCD1LG2, IFI30, TLR6, DSN1, HLA-DOB, CXCR6, TNF, IL10, SERPINA1, GSDMD, TRAF3, IL12RB1, CCL5 and LIG4.
Alterations in circulating metabolites have been identified in premenopausal women that may predict the development of breast cancer. In fact, ten metabolites listed in Figure 2A have been associated with breast cancer risk (88). Metabolomic signatures of the taurine and hypotaurine pathways and the alanine, aspartate and glutamate pathways obtained from the plasma of breast cancer patients are critical for early diagnosis (89). In addition, six metabolites and eight metabolic pathways have been identified in blood samples that can be used in the early diagnosis of breast cancer. Of the six metabolites, ethyl (R)-3-hydroxyhexanoate, caprylic acid, and hypoxanthine are noteworthy. Of the eight metabolic pathways identified, fatty acid and aminoacyl-tRNA biosynthesis and inositol phosphate metabolism are the pathways most implicated in the early diagnosis of BC (90). Alterations in lipid metabolism favor processes such as growth, proliferation, and motility of cancer cells, favoring tumor progression (91). The detection of lipids in human plasma samples has led to the identification of diagnostic biomarkers that reflect the early stage of TNBC (ES-TNBC). Diacylglycerol (DG) 34:2 is significantly downregulated in the TNBC subtype. Furthermore, a panel of 5 lipids (DG 34:2, PC 40:3, PC 39:8, PC 34:0 and PC 38:9) can differentiate TNBC from non-TNBC and ES-TNBC from ES-non-TNBC. Finally, in TNBC, ceramides are upregulated, whereas DG and LysoPC are downregulated and PC fluctuates (92) (Figure 2A).
Genomic evidence suggests that IDC is a consequence of DCIS progression. Genes and/or signaling pathways are altered during tumor progression. The most significant changes occur during the transition from normal tissue to carcinoma in situ. In addition to the loss of LIF, IL-6, HIN-1, IL-8, GROα, GROβ and MIP3α in carcinomas, glutamine synthase (GS) and desmoplakin (DSP) are the only two genes specifically upregulated in DCIS, while the metabolic enzymes 3-phosphoglycerate dehydrogenase and glyceraldehyde dehydrogenase and mitochondrial NADH: Ubiquinone dehydrogenase and NADH dehydrogenase 1α, have been observed in invasive carcinomas (85). Videlicet, cancer cells have important metabolic alterations (Figure 2B).
Downregulated genes in DCIS include TM4SF1, NFKB1A, PBEF, RASD1, TNFRSF10B, TNFAIP, CLU, NSEP1, LITAF/PIG7, BZW1, and CCNL1, as well as genes encoding several cytokines and chemokines such as ILF2, IL13RA1, LIF, CLC, CCL2, and CXCL1. Some transcripts are frequently overexpressed in the DCIS such as PKD1-like, STARD10, EPS8L2, and KIAA0545. Some of these genes are associated with nuclear factor kappa-light-chain-enhancer of activated B cells (NFκB) and tumor necrosis factor (TNF) pathways, resulting in impaired apoptosis and sustained proliferation of breast cancer cells (93). In IDC, upregulated genes can be grouped into genes related to cell cycle, extracellular matrix or secreted proteins, cell adhesion and motility, and signal transduction. Several underexpressed genes have also been detected in IDC, such as TM4SF1, TRAF4, PPARBP, AKR1A1, RSRP1, MAP1LC3A and RBBP6 (93). As for ILCs, they are characterized by CDH1 alterations, as well as dysregulation in the PI3K/Akt pathway due to mutations in PIK3CA, PTEN alterations, and mutations in AKT1 (94) (Figure 2B).
Transcriptomic analysis of tumor cells and their corresponding adjacent microenvironment cells [belonging to the Molecular Taxonomy of Breast Cancer International Consortium “METABRIC” cohort (95)] have shown that CDH1 mutation may deregulate immune cells in the tumor microenvironment (96). Expression of genes encoding the α and β subunits of the integrins ITGA4, ITGB2, ITGAX, ITGB7, ITGAM, ITGAL and ITGA8 correlates positively with the presence of immune cell infiltrates in the tumor, with markers of T cell activation and antigen presentation, and with immunosurveillance gene signatures. Expression of these integrins indicates a favorable prognosis in TNBC and HER2-enriched breast cancers. In contrast, expression of IBSP, ITGB3BP, ITGB6, ITGB1 and ITGAV predict a poor outcome (97) (Figure 2B).
Epigenetic modifications also contribute to breast cancer progression. For example, DNA methylation causes transcriptional silencing of tumor suppressor genes such as RASSF1A, RARB, SFN and TGM2 (98). Genome-wide methylation analysis revealed that CpG sites were hypermethylated and hypomethylated after CRY2 silencing. These data suggest that the absence of CRY2 causes epigenetic dysregulation of genes leading to breast cancer progression (99). The differences in miRNA expression profile are greater in IDC than in ILC compared to their respective carcinomas in situ. Thus, IDC is characterized by upregulation of let-7d, miR-210 and miR-221 and downregulation of miR-10b, miR-126, miR-143, miR-218, and miR-335-5p. In contrast, ILC is characterized by upregulation of miR-9, miR-375, miR-182 and miR-183 (57) (Figure 2B).
Proteomic analysis of plasma samples from hereditary BC patients carrying a mutation in the BRCA1 gene has shown that gelsolin, whose loss negatively correlates with tumor progression, is downregulated in these samples. In addition, its levels are associated with BRCA1 mutational status (100) (Figure 2B). Furthermore, O-GlcNAcylation is increased in primary malignant breast tumors, and this increase is associated with increased expression of O-GlcNAc transferase in grades II and III breast tumors (31).
Metabolomic and transcriptomic data integration studies have enabled the identification of genes, pathways, and metabolites as a part of a cancer prediction model and a better understanding of cancer progression. For example, adenosine monophosphate deaminase 1 (AMPD1) and ribonucleotide reductase regulatory subunit M2 (RRM2), which are involved in purine metabolism, have been associated with survival in breast cancer patients. Therefore, dysregulation of the purine metabolism pathway may influence breast cancer progression (101). Lipidomic analysis has identified two biomarkers capable of differentiating benign from malignant breast tumors: phosphatidylinositol (PI) (16:0/16:1) and PI (18:0/20:4). In addition, PI (16:0/18:1), phosphatidylglycerol (36:3) and glucosylceramide (d18:1/15:1) have been identified as potential biomarkers for assessing the degree of malignancy of breast tumors (102) (Figure 2B).
Microbiota dysbiosis contributes to breast cancer progression through its effects on skin and breast tissue. The presence of Listeria fleischmannii in breast tumor tissue is associated with epithelial-mesenchymal transition (EMT), whereas Haemophilus influenza correlates with tumor growth, cell cycle progression, E2F signaling, and mitotic spindle assembly (51). Alterations in the gut microbiome alter the production of bacterial metabolites that may influence tumor progression in breast cancer. Uric acid, glycolic acid, d-mannitol, 2,3-butanediol and trans-ferulic acid exert cytostatic effects, while 3-hydroxyphenylacetic acid, 4-hydroxybenzoic acid and vanillic acid stimulate breast cancer proliferation in vitro. In addition, 3-hydroxyphenylacetic acid, 4-hydroxybenzoic acid, 2,3-butanediol and hydrocinnamic acid inhibit EMT, and 2,3-butanediol has both cytostatic and anti-EMT properties (103). Gut microbiota may affect tumor progression by influencing the cancer-immunity dialogue. Gut bacteria elicit a complex and coordinated set of innate and adaptive immune responses to maintain tissue homeostasis. Consequently, when the microbiota-host balance is disrupted and dysbiosis occurs, increased production of inflammatory mediators is observed, which is associated with cancer progression. Low diversity in the gut microbiome is associated with decreased lymphocyte level and increased number of neutrophils, as well as decreased survival in breast cancer patients (51) (Figure 2B).
Between 7% and 11% of early breast cancers recur locally within 5 years after treatment, and 20% to 30% of primary breast cancers develop distant metastases. According to a study based on Surveillance, Epidemiology, and End Results Program (SEER) data for 2010-2013, the most common sites of breast cancer metastasis are bone (30-60%), lung (21-32%), liver (15-32%), and brain (4-10%) (104). Different molecular subtypes of breast cancer are associated with organ-specific metastases. Thus, luminal subtypes A and B metastasize primarily to the bone, the HER2-enriched subtype to the brain and liver, and TNBC to the lung (105). Genomic analyses have shown that metastases retain the same molecular subtype and prognostic signature as their primary tumors. These data suggest that metastatic potential is already determined in the primary tumor. The poor prognostic signature consists of genes that regulate cell cycle, invasion, metastasis, and angiogenesis (106, 107). Common alterations in TP53 (51%) and PIK3CA (49%), as well as mutations/deletions in NF1 (15%), mutations in PTEN (10%), and mutations/deletions in ARID1A (15%) have been identified in metastatic breast cancer tumors (108). Bone metastases of breast cancer are characterized by increased expression of the TFF1, TFF3, AGR2, NAT1 and CR1P1 genes, as well as the chemokine receptors CXCR4 and C-C chemokine receptor type 7 (CCR7), and upregulation of the zinc finger protein SNAI1 (SNAI1) (45, 109), which is involved in the induction of EMT (110). In breast to lung cancer metastasis, a number of genes such as MMP1, MMP2, CXCL1, PTGS2, ID1, VCAM1, EREG, SPARC, and IL13RA2 have been identified in breast to lung cancer metastasis that promote and are clinically correlated; as well as the mitogen-activated protein kinases (MAPK), NFκB and vascular endothelial growth factor (VEGF) signaling pathways (111) (45). Mutations in the ESR1, AKT1, ERBB2, and FGFR4 genes have been observed in metastatic breast tumors in the liver (45, 112, 113). Transcriptome analysis revealed that the TNF-α pathway is upregulated in lung metastases compared to liver metastases (114). ATAD2, DERL1 and NEK2A have been shown to be overexpressed (115–117), whereas ATM, CRYAB and HSPB2 genes are often suppressed and/or underexpressed in breast cancer metastases to the brain (45, 118, 119), (Figure 2C). The AURORA study, consisting of genomic and transcriptomic profiling in matched primary tumors and early metastases, has described the key role of somatic mutations GATA1 and MEN1 in metastasis. In addition, the enrichment of ESR1, PTEN, and PIK3CA in metastases have been determined, as well as CDH1 and RB1 mutations, MDM4 and MYC amplifications, and ARID1A deletions (120). Of note, TP53 mutations and MYC amplification are associated with shorter time to relapse (25). Eight common genes have been found to have significant effects on TNBC survival (ELOB, SLC39A7, TIMM13, BANF1, NDUFS1, NDUFB7, TRAPPC5, and MVD). Finally, a signature of 87 immune genes has been established that is highly predictive of pathologic complete response (pCR), which in turn correlates with improved OS and distant metastasis-free survival (DMFS) (121). These 87 immune genes are shown in Figure 2C.
The immune system is involved in the development of cancer, from tumor initiation to metastasis (122). Patients with stage 3 and 4 breast cancer have a higher percentage of immunosuppressive cells (granulocytic myeloid-derived suppressor cells (MDSCs), CD14+CD16+ intermediate monocytes, and CD127−CD25highFoxP3+ Treg cells). Inflammation-related genes are differentially expressed in TNBC. In fact, low expression of CD163 and CXCR4 together with high expression of thrombospondin 1 (THBS1) correlates with an increased risk of relapse and poor survival in TNBC (123). The proinflammatory cytokines serum amyloid A (SAA) and IL-18 are elevated in the serum of patients with recurrent breast cancer. Therefore, SAA and IL-18 may be prognostic markers for breast cancer recurrence (124) (Figure 2C).
miRNAs are also involved in cancer migration and metastasis. miR-21, miR-10b, miR-373 and miR-520c promote metastasis, whereas miR-126, miR-335, miR-31, miR-146a, and miR-497 suppress metastasis. miR-9 is associated with local recurrence and ER+ tumors, whereas miR-10 is involved in cell proliferation, migration, and invasion (57). On the other hand, Cyclin D2, RAR-beta, Twist, RASSF1A and HIN-1 genes show increased methylation in bone, brain and lung metastases compared to primary breast cancer, with HIN-1 and RAR-beta methylation significantly higher in each group (125). Hypermethylation and downregulation of genes involved in breast cancer progression or EMT, such as LYN, MMP7, KLK10 and WNT6, are associated with a significantly lower risk of metastatic relapse (126). Furthermore, H3K4 acetylation has been correlated with breast cancer progression, estrogen responsiveness, and the oncogenic EMT pathway. Therefore, H3K4 is a potential biomarker for tumor progression leading to aggressive metastatic phenotypes (127) (Figure 2C).
Other biomarkers have been identified using other omics techniques. As mentioned above, E-cadherin is considered a good prognostic marker in non-invasive breast cancer, and loss of E-cadherin protein is one of the main features of EMT (82). Furthermore, a metabolomic study has identified 9-cis-retinoic acid as a critical metabolite in breast cancer progression, as it significantly decreased during breast cancer progression to metastasis. This suggests that 9-cis-retinoic acid inhibits tumor progression to metastasis, probably by attenuating cell invasion and migration (128). Combining lipidomic techniques with transcriptomic analysis, PI (18:0/20:3) accumulation has been found to be associated with an increased incidence of lymph node metastasis and activation of the PD-1-related immune checkpoint pathway (129).
Finally, alterations in the microbiota have also been observed to influence breast cancer metastasis. The presence of Bacteroides fragilis, a gut-colonizing bacterium, can induce epithelial hyperplasia to promote tumor growth and metastasis via the β-catenin–Notch1 axis (130). In addition, Fusobacterium nucleatum promotes tumor progression and metastasis (131) (Figure 2C).
Molecular changes that occur during cancer treatment determine the response to different therapies and guide the optimal choice of treatments to reduce local recurrence and distant metastasis, thereby increasing disease-free survival. The various omics are key to identifying these molecular changes. In fact, several clinical trials are based on one or more omics technologies.
Classically, aromatase inhibitors are recommended for patients with ER+ metastatic breast cancer because they suppress estrogen production. Trastuzumab and lapatinib are administered to patients with HER2-enriched breast tumors, as trastuzumab is a humanized monoclonal antibody against the extracellular domain of HER2, and lapatinib is a tyrosine kinase inhibitor that blocks both HER2 and EGFR activation. However, in light of the omics findings, more refined treatments may be considered according to genomic alterations. For example, both luminal subtypes frequently harbor mutations in PIK3CA, so inhibitors of this kinase may be a therapeutic target; MYC amplification suggests the use of platinum analogs and taxanes; and, patients with BRCA1/2 mutations may benefit from poly ADP ribose polymerase (PARP) inhibitors and/or platinum compounds (44). The RAS/RAF/MEK/ERK signaling pathway is altered in tumors that overexpress EGFR and HER2. Since this kinase cascade is critical for survival and apoptosis, alterations in this pathway could affect sensitivity/resistance to anticancer therapies. Mutations in KRAS, HRAS, NRAS, BRAF and NF1 have been observed in all breast cancer subtypes, although their frequency does not exceed 4%. However, copy number alterations (CNAs) of KRAS and BRAF have been observed in TNBC. Therefore, the use of inhibitors of these kinases in combination with other therapies may delay or induce resistance to treatment (132). ESR1 methylation is considered a good predictor of survival in tamoxifen-treated patients, whereas ARHI methylation predicts survival in non-tamoxifen-treated patients. BRCA1 hypermethylation, in turn, sensitizes TNBC patients to DNA-damaging chemotherapeutic agents such as cisplatin and PARP inhibitors (77). The prevalence of the PI3K/AKT/mTOR signaling axis has been observed in TNBC. The c-Kit protein, a receptor tyrosine kinase involved in the initiation of this cascade, is overexpressed in 20-25% of TNBC, hence, the tyrosine kinase inhibitors (TKIs) such as imatinib or sunitinib are used in the treatment of these cancers (133) (Table 4).
The endoplasmic reticulum protein KIAA1199, which is involved in tumor growth and invasiveness, is significantly overexpressed in breast tumor samples. Therefore, KIAA1199 is considered a new target for biomarker development and a novel therapeutic target for breast cancer (134, 135). Similarly, cofilin-1 (CFL-1), interleukin-32 (IL-32), proliferating cell nuclear antigen (PCNA), syntenin-1 (SDCBP), and riboforin-2 (RPN-2) have been identified as potential target antigens for HLA-A2+ TNBC immunotherapy (136), or the proto-oncogene RET and kallikrein (KLK)8 as antigens associated with breast cancer in general (137).
Metabolic and lipidomic profiling of TNBC samples combined with transcriptomic and genomic data have identified a number of metabolites as potential therapeutic targets for different transcriptomic subtypes of TNBC. The LAR subtype is characterized by an enrichment of ceramides and fatty acids. Therefore, sphingosine-1-phosphate (S1P), an intermediate of the ceramide pathway, may be a promising drug for the treatment of LAR tumors. In contrast, the basal-like immunosuppressed transcriptomic subtype (BLIS) is characterized by increased metabolites related to oxidation reaction and glycosyl transfer and the lowest level of metabolic dysregulation. In this case, N-acetyl-aspartyl-glutamate has been identified as a key tumor-promoting metabolite and a potential therapeutic target for high-risk BLIS tumors (138). Furthermore, high levels of sphingomyelins are associated with improved DFS in patients with TNBC. Therefore, sphingomyelins and enzymes involved in sphingolipid metabolism could be considered as prognostic markers and potential therapeutic targets, respectively (139). Omics studies can also identify specific pharmacological pathways of resistance and sensitivity of tumor cells to different therapies. For example, a combination of TP53 deficiency and silencing of BRCA1, BRCA2, or BRCA1/2-associated genes results in cisplatin sensitivity (140). PI3KCA mutations alone or in combination with PTEN appear to predict worse outcome after trastuzumab monotherapy or in combination with chemotherapy (141). BRCA1/2-deficient cells are sensitive to PARP1 inhibitors. In addition, silencing of kinases such as cyclin-dependent kinase 5 (CDK5), mitogen-activated protein kinase 12 (MAPK12), polo-like kinase 3 (PLK3), polynucleotide phosphatase/bifunctional kinase (PNKP), serine/threonine kinase (STK)22c, and STK36 strongly sensitize to PARP inhibitors (142). Cyclin-dependent kinase (CDK)10 has been identified as a determinant of resistance to endocrine therapies such as tamoxifen, as low CDK10 levels lead to early on tamoxifen treatment (143) (Figure 3A). The classic treatment for HR+ breast cancer is endocrine therapy. However, approximately 30% of patients develop resistance to endocrine therapy. A transcriptomic/proteomic study has identified the set of candidate genes CEACAM1, KRT19, TMEM81, TMEM119, ESRRA, ERBB3, SRC, AKT1S1, SGEF, SCG5, ALOX12B, CKB, BID, XRCC1, NSL1, and CHEK2 that are able to discriminate progression/resistance (PD) from complete response (CR) and correlate significantly with survival (144). In addition, 298 differentially expressed genes were identified between drug-sensitive (DS) and drug-resistant (DR) breast cancer patients prior to neoadjuvant treatment. Among them, the peptidyl-prolyl cis-trans isomerase FKBP4 (FKBP4) and the protein S100-A9 (S100A9) could be putative predictive markers to distinguish the DR group from the DS group of breast cancer patients (145).
Figure 3 Biomarkers of breast cancer defining treatment outcome detected by omics. Biomarkers identified by different omics can determine drug sensitivity/resistance (A) or type of response to treatment (B). NATC, neoadjuvant targeted therapy; pCR, pathological complete response; pPR, pathological partial response; pSD, pathological stable disease. “up arrow” means increase.
Metabolic studies have identified biomarkers that predict response to treatment. For example, glycohyocholic and glucodeoxycholic acids can stratify TNBC patients according to response to neoadjuvant chemotherapy and OS (146). On the other hand, when comparing patients with large primary breast cancer who had received neoadjuvant chemotherapy plus bevacizumab with those who had received chemotherapy alone, higher levels of leucine, acetoacetate and trihydroxybutyrate and lower levels of formate were observed 12 weeks after treatment (147). Furthermore, baseline immunometabolic assessment in combination with ER status could predict the response to neoadjuvant targeted chemotherapy (NATC) based on patient trastuzumab-paclitaxel combination and disease relapse in HER2+ patients. HER2+/ER+ patients have higher levels of T-cell stimulating factors, but also higher levels of cytokines that might be responsible for T-cell suppression. The combination of metabolic data with IL-2 and IL-10 cytokine levels has been shown to be prognostic for relapse (148). In addition, serum metabolites such as leucine, formate, valine, and proline, along with hormone receptor status, have been shown to be discriminators of NATC response. For example, formate, proline, valine, HR+, and HER2− are directly associated with NATC resistance. In contrast, leucine, HR− and HER2+ are directly related to NATC sensitivity. In addition, glyoxylate and dicarboxylate metabolism have been implicated in NATC resistance (149) (Figure 3A).
Pathologic complete response (pCR) and residual disease have been correlated with the genome, transcriptome, and tumor immune microenvironment in patients with early and locally advanced breast cancer undergoing neoadjuvant therapy (150). The pCR is associated with overexpression of driver genes such as CDKN2A, EGFR, CCNE1, and MYC and underexpression of CCND1, ZNF703, and ESR1, as well as increased immune activation characterized by enrichment of innate and adaptive immune cells. However, tumors with residual disease, particularly HER2− tumors, showed enrichment of EMT and attenuated immune response due to the enrichment of inhibitory CD56dim natural killer cells and regulatory T cells, leading to therapy resistance (150). In transcriptomic profiling, HSP90AA1, EEF1A1, APP and HSPA4 were associated with recurrence in breast cancer patients with pCR due to neoadjuvant chemotherapy. TP53, EGFR, CTNNB1, ERBB2 and HSPB1 may play an important role in the survival of pCR patients. Patients with tumors expressing high levels of HSP90AA1, HSPA4, S100A8, and TP53 and low levels of EEF1A1, EGFR and CTNNB1 showed significantly worse overall survival (OS) (151) (Figure 3B). A set of genes including AKT1S1, NSL1, ESRRA, TMEM81, CKB, SGEF, KRT19, SCG5, CEACAM1, ALOX12B, IDB, SRC, CHEK2, ERBB3 and XRCC1 have been identified as critical targets of both selective estrogen receptor modulators (SERMs)/selective estrogen receptor downregulators (SERDs) and aromatase inhibitors (AIs) of endocrine resistance (144).
A systemic metabolic study showed that patients with large primary breast cancers undergoing neoadjuvant chemotherapy with poor response have higher citrate levels and lower histidine levels (147). In addition, TNBC patients with pCR had elevated levels of circulating τ-methylhistidine, phenylalanine, π-methylhistidine, lactic acid, glucose, alanine, glutamic acid, citric acid, dimethylamine and phosphocholine, whereas patients with or pathological stable disease (pSD) had elevated levels of valine, 2-aminobutanoic acid, propionic acid, ethanol, proline, asparagine, and N,N-dimethylglycine. Finally, TNBC patients with pathologic partial response (pPR) had high levels of 2-hydroxyisovaleric acid, acetoacetate, trimethylamine, creatine, myo-inositol and ornithine, but low levels of five metabolites, namely isoleucine, phenylalanine, threonine, dimethylamine and glycerophosphocholine (Figure 3B). Therefore, we can infer that alterations in the pathways of glycine, serine, and threonine metabolism; valine, leucine, and isoleucine biosynthesis; and alanine, aspartate, and glutamate metabolism could be used as potential models to predict whether a patient with TNBC is suitable to receive neoadjuvant chemotherapy (152).
The relevance of all these biomarkers lies in their translational potential to identify specific treatments for breast cancer. Several clinical trials are ongoing. A Phase I program conducted at the University of Texas MD Anderson Cancer Center demonstrated that patients who received alteration-matched therapy had a higher objective response rate (ORR), PFS, and OS compared to unmatched therapy (153).
One of the most important clinical trials based on genomic studies is the “Microarray In Node Negative Disease may Avoid ChemoTherapy” (MINDACT). The MINDACT clinical trial aims to demonstrate the clinical relevance of the 70-gene prognostic signature (or MammaPrint™) determined by van’t Veer (106) and to compare it with the traditional clinicopathologic prognostic indicators for the assignment of adjuvant chemotherapy in patients with node-negative breast cancer (154). As a result, MammaPrint™ was found to be effective as traditional tools in identifying high-risk patients, but more accurate in identifying low-risk patients who could avoid adjuvant chemotherapy (155, 156). This gene signature outperforms all traditional clinical prognostic factors and clearly discriminates patients with an excellent prognosis from those at high risk of recurrence (157, 158). Phase III results showed that approximately 46% of women with clinically high-risk breast cancer are unlikely to need chemotherapy (159). Women with high clinical risk and low genomic risk younger than 50 years had excellent DMFS when treated with endocrine therapy alone (160). In addition, patients with ER+, HER2− and stage I lymph node-negative tumors ≤2 cm treated with endocrine therapy had significantly fewer breast cancer events (161). On the other hand, no improvement in outcomes was observed with the use of docetaxel-capecitabine compared with anthracycline-based chemotherapy (162). Last year (2022), the Austrian Group Medical Tumor Therapy prospective registry confirmed that the addition of MammaPrint™ to the routine treatment of early luminal breast cancer yields clinically useful results (163).
Concurrent with the MINDACT clinical trial in Europe, the Trial Assigning Individualized Treatment Options Rx (TAILORx) was conducted in North America with the goal of reducing chemotherapy overtreatment by integrating molecular diagnostic testing into the clinical decision-making process. TAILORx is based on a 21-gene based assay (Oncotype DX™) that calculates a recurrence score (RS) and reserves chemotherapy for patients with a low RS (164). Women with a high RS who were treated with adjuvant chemotherapy regimens containing taxanes and/or anthracyclines plus endocrine therapy had an estimated 5-year freedom rate from distant breast cancer recurrence of 93% (165). In patients with ER+, HER2−, lymph node-negative and intermediate RS, adjuvant endocrine and chemoendocrine therapy had similar efficacy, although chemotherapy had some benefit in some women aged 50 years or younger (166). Among women with intermediate RS, Hispanic ethnicity and Asian race were associated with better outcomes. However, Black race was associated with worse clinical outcomes and did not benefit from adjuvant chemotherapy (167).
Other studies are the aforementioned AURORA US Metastatasis project and the TransNEO study. The AURORA US Metastasis project conducted a multiomic study including genomics, epigenomics and transcriptomics in primary tumors and their corresponding metastatic breast cancers (168). In metastatic TNBC, significantly lower expression of MHC class I genes (HLA-A, HLA-B, and HLA-C), DNA methylation of HLA-A, and small focal HLA-A were observed, which were associated with lower immunity and worse OS. Tumors with DNA-methylated HLA-A could be targeted for DNA demethylating drugs in combination with immune checkpoint inhibitors (ICI) (168, 169). The TransNEO molecular profiling study of patients with early and locally advanced breast cancer undergoing neoadjuvant therapy. Genomic, transcriptomic, and tumor immune microenvironment data were combined with clinical and digital pathology data to perform machine learning to create a predictive pCR model. This model is robust and could guide treatment selection in future clinical trials, including in the context of adjuvant therapy (150).
Currently, treatment selection in breast cancer patients is based on broad clinicopathologic features that fail to accurately classify patients into risk groups, hence resulting into overtreatment of vast segments of patients. Data suggest that ORR, DFS and OS can be improved by the use of tailored therapies. Improved outcomes depend on the identification of new biomarkers that allow for the stratification of patients and eligibility for new therapies. Besides, the identification of biomarkers that predict treatment efficacy will minimize side effects or cumulative toxicity in patients unlikely to benefit from such treatments. Clinical trials, which thus far have mostly been based on genomic, transcriptomic and/or proteomic studies, have been effective in assigning treatment. It is our understanding that multiomics studies, including other omics techniques such as metabolomics, immunomics or microbiomics, are an important step towards precision medicine and hence refine the assignment of the best possible treatment for each patient. Special attention should be paid to the statistical methods used in the analysis of multiomic data to avoid spurious correlations. In fact, correlation coefficients should not be used to explain a process, such as cancer progression, in which multiple variables are involved. In these cases, the use of regression or multivariate analysis techniques will be more appropriate. In addition, validation cohorts will be needed to confirm the reproducibility, robustness, and validity of the results. It is important that validation cohorts have a pre-calculated sample size using statistical power tests and that minimum assay quality criteria have been established. In conclusion, we anticipate that clinical trials based on high-dimensional multiomics data interpreted by artificial intelligence will guide each patient to an optimized and personalized treatment, that will avoid overtreatment, minimize side effects, and improve both DFS and OS.
LA: Conceptualization, Investigation, Writing – original draft, Writing – review & editing. DB: Validation, Writing – original draft, Writing – review & editing. MD: Validation, Writing – original draft, Writing – review & editing. MI: Validation, Writing – original draft, Writing – review & editing. GK: Validation, Writing – original draft, Writing – review & editing. RP: Validation, Writing – original draft, Writing – review & editing. LS: Conceptualization, Funding acquisition, Investigation, Resources, Supervision, Writing – original draft, Writing – review & editing.
The author(s) declare financial support was received for the research, authorship, and/or publication of this article. LS is supported by the Spanish Agencia Estatal de Investigación, AEI (grant number PID2021-126426OB-I00), the Strategic Program from the Institute of Biomedicine and Molecular Genetics (IBGM) of Valladolid (CCVC8485) and the Internationalization Project of the Unit of Excellence IBGM of Valladolid from the Junta de Castilla y León (CL-EI-2021 IBGM), the Institut National du Cancer (INCa, PLBIO2022-093), as well as the “Beatriz Galindo” Program from the Spanish Ministry of Universities. GK is supported by the Ligue contre le Cancer (équipe labellisée); Agence National de la Recherche (ANR) – Projets blancs; AMMICa US23/CNRS UMS3655; Association pour la recherche sur le cancer (ARC); Cancéropôle Ile-de-France; European Research Council Advanced Investigator Grand “ICD-Cancer”, Fondation pour la Recherche Médicale (FRM); a donation by Elior; Equipex Onco-Pheno-Screen; European Joint Programme on Rare Diseases (EJPRD); European Research Council (ICD-Cancer), European Union Horizon 2020 Projects Oncobiome and Crimson; Fondation Carrefour; Institut National du Cancer (INCa); Institut Universitaire de France; LabEx Immuno-Oncology (ANR-18-IDEX-0001); a Cancer Research ASPIRE Award from the Mark Foundation; the RHU Immunolife; Seerave Foundation; SIRIC Stratified Oncology Cell DNA Repair and Tumor Immune Elimination (SOCRATE); and SIRIC Cancer Research and Personalized Medicine (CARPEM). This study contributes to the IdEx Université de Paris ANR-18-IDEX-0001. LA-F holds a predoctoral fellowship from the Asociación Española Contra el Cáncer (AECC). DB and RPR are supported by the University of Valladolid.
GK has been holding research contracts with Daiichi Sankyo, Eleor, Kaleido, Lytix Pharma, PharmaMar, Osasuna Therapeutics, Samsara Therapeutics, Sanofi, Tollys, and Vascage. GK is on the Board of Directors of the Bristol Myers Squibb Foundation France. GK is a scientific co-founder of everImmune, Osasuna Therapeutics, Samsara Therapeutics and Therafast Bio. GK is in the scientific advisory boards of Hevolution, Institut Servier and Longevity Vision Funds. GK is the inventor of patents covering therapeutic targeting of aging, cancer, cystic fibrosis, and metabolic disorders. GK’wife, Laurence Zitvogel, has held research contracts with Glaxo Smyth Kline, Incyte, Lytix, Kaleido, Innovate Pharma, Daiichi Sankyo, Pilege, Merus, Transgene, 9 m, Tusk and Roche, was on the on the Board of Directors of Transgene, is a cofounder of everImmune, and holds patents covering the treatment of cancer and the therapeutic manipulation of the microbiota. GK’s brother, Romano Kroemer, was an employee of Sanofi and now consults for Boehringer-Ingelheim. The funders had no role in the design of the study, in the writing of the manuscript, or in the decision to publish the results.
The remaining authors declare that the research was conducted in the absence of any commercial or financial relationships that could be constructed as a potential conflict of interest.
The author(s) declared that they were an editorial board member of Frontiers, at the time of submission. This had no impact on the peer review process and the final decision.
All claims expressed in this article are solely those of the authors and do not necessarily represent those of their affiliated organizations, or those of the publisher, the editors and the reviewers. Any product that may be evaluated in this article, or claim that may be made by its manufacturer, is not guaranteed or endorsed by the publisher.
1. Sung H, Ferlay J, Siegel RL, Laversanne M, Soerjomataram I, Jemal A, et al. Global cancer statistics 2020: GLOBOCAN estimates of incidence and mortality worldwide for 36 cancers in 185 countries. CA Cancer J Clin (2021) 71(3):209–49. doi: 10.3322/caac.21660
2. Zubair M, Wang S, Ali N. Advanced approaches to breast cancer classification and diagnosis. Front Pharmacol (2020) 11:632079. doi: 10.3389/fphar.2020.632079
3. Parise CA, Bauer KR, Brown MM, Caggiano V. Breast cancer subtypes as defined by the estrogen receptor (Er), progesterone receptor (Pr), and the human epidermal growth factor receptor 2 (Her2) among women with invasive breast cancer in California, 1999-2004. Breast J (2009) 15(6):593–602. doi: 10.1111/j.1524-4741.2009.00822.x
4. Patel HK, Bihani T. Selective estrogen receptor modulators (Serms) and selective estrogen receptor degraders (Serds) in cancer treatment. Pharmacol Ther (2018) 186:1–24. doi: 10.1016/j.pharmthera.2017.12.012
5. Li Z, Wei H, Li S, Wu P, Mao X. The role of progesterone receptors in breast cancer. Drug Des Devel Ther (2022) 16:305–14. doi: 10.2147/DDDT.S336643
6. Schlam I, Swain SM. Her2-positive breast cancer and tyrosine kinase inhibitors: the time is now. NPJ Breast Cancer (2021) 7(1):56. doi: 10.1038/s41523-021-00265-1
7. Perou CM, Sorlie T, Eisen MB, van de Rijn M, Jeffrey SS, Rees CA, et al. Molecular portraits of human breast tumours. Nature (2000) 406(6797):747–52. doi: 10.1038/35021093
8. Sorlie T, Perou CM, Tibshirani R, Aas T, Geisler S, Johnsen H, et al. Gene expression patterns of breast carcinomas distinguish tumor subclasses with clinical implications. Proc Natl Acad Sci USA (2001) 98(19):10869–74. doi: 10.1073/pnas.191367098
9. Lehmann BD, Bauer JA, Chen X, Sanders ME, Chakravarthy AB, Shyr Y, et al. Identification of human triple-negative breast cancer subtypes and preclinical models for selection of targeted therapies. J Clin Invest (2011) 121(7):2750–67. doi: 10.1172/JCI45014
10. Lehmann BD, Pietenpol JA. Identification and use of biomarkers in treatment strategies for triple-negative breast cancer subtypes. J Pathol (2014) 232(2):142–50. doi: 10.1002/path.4280
11. Allison KH. Prognostic and predictive parameters in breast pathology: A pathologist's primer. Mod Pathol (2021) 34(Suppl 1):94–106. doi: 10.1038/s41379-020-00704-7
12. Mitola G, Falvo P, Bertolini F. New insight to overcome tumor resistance: an overview from cellular to clinical therapies. Life (Basel) (2021) 11(11). doi: 10.3390/life11111131
13. Hanahan D, Weinberg RA. Hallmarks of cancer: the next generation. Cell (2011) 144(5):646–74. doi: 10.1016/j.cell.2011.02.013
14. Hanahan D. Hallmarks of cancer: new dimensions. Cancer Discov (2022) 12(1):31–46. doi: 10.1158/2159-8290.CD-21-1059
15. Rennstam K, Hedenfalk I. High-throughput genomic technology in research and clinical management of breast cancer. Molecular signatures of progression from benign epithelium to metastatic breast cancer. Breast Cancer Res (2006) 8(4):213. doi: 10.1186/bcr1528
16. Sato F, Saji S, Toi M. Genomic tumor evolution of breast cancer. Breast Cancer (2016) 23(1):4–11. doi: 10.1007/s12282-015-0617-8
17. Lowe R, Shirley N, Bleackley M, Dolan S, Shafee T. Transcriptomics technologies. PLoS Comput Biol (2017) 13(5):e1005457. doi: 10.1371/journal.pcbi.1005457
18. Zoppino FCM, Guerrero-Gimenez ME, Castro GN, Ciocca DR. Comprehensive transcriptomic analysis of heat shock proteins in the molecular subtypes of human breast cancer. BMC Cancer (2018) 18(1):700. doi: 10.1186/s12885-018-4621-1
19. Perez de Souza L, Alseekh S, Scossa F, Fernie AR. Ultra-high-performance liquid chromatography high-resolution mass spectrometry variants for metabolomics research. Nat Methods (2021) 18(7):733–46. doi: 10.1038/s41592-021-01116-4
20. Adamczyk B, Tharmalingam T, Rudd PM. Glycans as cancer biomarkers. Biochim Biophys Acta (2012) 1820(9):1347–53. doi: 10.1016/j.bbagen.2011.12.001
21. Claudino WM, Quattrone A, Biganzoli L, Pestrin M, Bertini I, Di Leo A. Metabolomics: available results, current research projects in breast cancer, and future applications. J Clin Oncol (2007) 25(19):2840–6. doi: 10.1200/JCO.2006.09.7550
22. Silva C, Perestrelo R, Silva P, Tomas H, Camara JS. Breast cancer metabolomics: from analytical platforms to multivariate data analysis. A Review Metabolites (2019) 9(5). doi: 10.3390/metabo9050102
23. Yugi K, Kubota H, Hatano A, Kuroda S. Trans-omics: how to reconstruct biochemical networks across multiple 'Omic' Layers. Trends Biotechnol (2016) 34(4):276–90. doi: 10.1016/j.tibtech.2015.12.013
24. Sarhadi VK, Armengol G. Molecular biomarkers in cancer. Biomolecules (2022) 12(8). doi: 10.3390/biom12081021
25. Fumagalli C, Ranghiero A, Gandini S, Corso F, Taormina S, De Camilli E, et al. Inter-tumor genomic heterogeneity of breast cancers: comprehensive genomic profile of primary early breast cancers and relapses. Breast Cancer Res (2020) 22(1):107. doi: 10.1186/s13058-020-01345-z
26. Cancer Genome Atlas N. Comprehensive molecular portraits of human breast tumours. Nature (2012) 490(7418):61–70. doi: 10.1038/nature11412
27. Rajendran BK, Deng CX. Characterization of potential driver mutations involved in human breast cancer by computational approaches. Oncotarget (2017) 8(30):50252–72. doi: 10.18632/oncotarget.17225
28. Vreeker GCM, Vangangelt KMH, Bladergroen MR, Nicolardi S, Mesker WE, Wuhrer M, et al. Serum N-glycan profiles differ for various breast cancer subtypes. Glycoconj J (2021) 38(3):387–95. doi: 10.1007/s10719-021-10001-3
29. Abd Hamid UM, Royle L, Saldova R, Radcliffe CM, Harvey DJ, Storr SJ, et al. A strategy to reveal potential glycan markers from serum glycoproteins associated with breast cancer progression. Glycobiology (2008) 18(12):1105–18. doi: 10.1093/glycob/cwn095
30. Alley WR Jr., Madera M, Mechref Y, Novotny MV. Chip-based reversed-phase liquid chromatography-mass spectrometry of permethylated N-linked glycans: A potential methodology for cancer-biomarker discovery. Anal Chem (2010) 82(12):5095–106. doi: 10.1021/ac100131e
31. Champattanachai V, Netsirisawan P, Chaiyawat P, Phueaouan T, Charoenwattanasatien R, Chokchaichamnankit D, et al. Proteomic analysis and abrogated expression of O-glcnacylated proteins associated with primary breast cancer. Proteomics (2013) 13(14):2088–99. doi: 10.1002/pmic.201200126
32. Takayama T, Tsutsui H, Shimizu I, Toyama T, Yoshimoto N, Endo Y, et al. Diagnostic approach to breast cancer patients based on target metabolomics in saliva by liquid chromatography with tandem mass spectrometry. Clin Chim Acta (2016) 452:18–26. doi: 10.1016/j.cca.2015.10.032
33. Silva CL, Perestrelo R, Capelinha F, Tomas H, Camara JS. An integrative approach based on Gc-Qms and Nmr metabolomics data as a comprehensive strategy to search potential breast cancer biomarkers. Metabolomics (2021) 17(8):72. doi: 10.1007/s11306-021-01823-1
34. An R, Yu H, Wang Y, Lu J, Gao Y, Xie X, et al. Integrative analysis of plasma metabolomics and proteomics reveals the metabolic landscape of breast cancer. Cancer Metab (2022) 10(1):13. doi: 10.1186/s40170-022-00289-6
35. Singh A, Sharma RK, Chagtoo M, Agarwal G, George N, Sinha N, et al. 1h nmr metabolomics reveals association of high expression of inositol 1, 4, 5 trisphosphate receptor and metabolites in breast cancer patients. PLoS One (2017) 12(1):e0169330. doi: 10.1371/journal.pone.0169330
36. Nagahashi M, Tsuchida J, Moro K, Hasegawa M, Tatsuda K, Woelfel IA, et al. High levels of sphingolipids in human breast cancer. J Surg Res (2016) 204(2):435–44. doi: 10.1016/j.jss.2016.05.022
37. Peetla C, Vijayaraghavalu S, Labhasetwar V. Biophysics of cell membrane lipids in cancer drug resistance: implications for drug transport and drug delivery with nanoparticles. Adv Drug Deliv Rev (2013) 65(13-14):1686–98. doi: 10.1016/j.addr.2013.09.004
38. Hilvo M, Denkert C, Lehtinen L, Muller B, Brockmoller S, Seppanen-Laakso T, et al. Novel theranostic opportunities offered by characterization of altered membrane lipid metabolism in breast cancer progression. Cancer Res (2011) 71(9):3236–45. doi: 10.1158/0008-5472.CAN-10-3894
39. Qiu Y, Zhou B, Su M, Baxter S, Zheng X, Zhao X, et al. Mass spectrometry-based quantitative metabolomics revealed a distinct lipid profile in breast cancer patients. Int J Mol Sci (2013) 14(4):8047–61. doi: 10.3390/ijms14048047
40. Parida S, Sharma D. The power of small changes: comprehensive analyses of microbial dysbiosis in breast cancer. Biochim Biophys Acta Rev Cancer (2019) 1871(2):392–405. doi: 10.1016/j.bbcan.2019.04.001
41. Urbaniak C, Gloor GB, Brackstone M, Scott L, Tangney M, Reid G. The microbiota of breast tissue and its association with breast cancer. Appl Environ Microbiol (2016) 82(16):5039–48. doi: 10.1128/AEM.01235-16
42. Thompson KJ, Ingle JN, Tang X, Chia N, Jeraldo PR, Walther-Antonio MR, et al. A comprehensive analysis of breast cancer microbiota and host gene expression. PLoS One (2017) 12(11):e0188873. doi: 10.1371/journal.pone.0188873
43. Hieken TJ, Chen J, Hoskin TL, Walther-Antonio M, Johnson S, Ramaker S, et al. The microbiome of aseptically collected human breast tissue in benign and Malignant disease. Sci Rep (2016) 6:30751. doi: 10.1038/srep30751
44. Low SK, Zembutsu H, Nakamura Y. Breast cancer: the translation of big genomic data to cancer precision medicine. Cancer Sci (2018) 109(3):497–506. doi: 10.1111/cas.13463
45. Bennett C, Carroll C, Wright C, Awad B, Park JM, Farmer M, et al. Breast cancer genomics: primary and most common metastases. Cancers (Basel) (2022) 14(13). doi: 10.3390/cancers14133046
46. Fu-Jun L, Shao-Hua J, Xiao-Fang S. Differential proteomic analysis of pathway biomarkers in human breast cancer by integrated bioinformatics. Oncol Lett (2012) 4(5):1097–103. doi: 10.3892/ol.2012.881
47. Saldova R, Haakensen VD, Rodland E, Walsh I, Stockmann H, Engebraaten O, et al. Serum N-glycome alterations in breast cancer during multimodal treatment and follow-up. Mol Oncol (2017) 11(10):1361–79. doi: 10.1002/1878-0261.12105
48. Torres CR, Hart GW. Topography and polypeptide distribution of terminal N-acetylglucosamine residues on the surfaces of intact lymphocytes. Evidence for O-linked glcnac. J Biol Chem (1984) 259(5):3308–17. doi: 10.1016/S0021-9258(17)43295-9
49. Alakwaa FM, Chaudhary K, Garmire LX. Deep learning accurately predicts estrogen receptor status in breast cancer metabolomics data. J Proteome Res (2018) 17(1):337–47. doi: 10.1021/acs.jproteome.7b00595
50. Urbaniak C, Cummins J, Brackstone M, Macklaim JM, Gloor GB, Baban CK, et al. Microbiota of human breast tissue. Appl Environ Microbiol (2014) 80(10):3007–14. doi: 10.1128/AEM.00242-14
51. Bernardo G, Le Noci V, Di Modica M, Montanari E, Triulzi T, Pupa SM, et al. The emerging role of the microbiota in breast cancer progression. Cells (2023) 12(15). doi: 10.3390/cells12151945
52. Esposito MV, Fosso B, Nunziato M, Casaburi G, D'Argenio V, Calabrese A, et al. Microbiome composition indicate dysbiosis and lower richness in tumor breast tissues compared to healthy adjacent paired tissue, within the same women. BMC Cancer (2022) 22(1):30. doi: 10.1186/s12885-021-09074-y
53. Liu L, Hao X, Song Z, Zhi X, Zhang S, Zhang J. Correlation between family history and characteristics of breast cancer. Sci Rep (2021) 11(1):6360. doi: 10.1038/s41598-021-85899-8
55. Lakhani SR, Van De Vijver MJ, Jacquemier J, Anderson TJ, Osin PP, McGuffog L, et al. The pathology of familial breast cancer: predictive value of immunohistochemical markers estrogen receptor, progesterone receptor, Her-2, and P53 in patients with mutations in Brca1 and Brca2. J Clin Oncol (2002) 20(9):2310–8. doi: 10.1200/JCO.2002.09.023
56. Verhoog LC, Brekelmans CT, Seynaeve C, Dahmen G, van Geel AN, Bartels CC, et al. Survival in hereditary breast cancer associated with germline mutations of Brca2. J Clin Oncol (1999) 17(11):3396–402. doi: 10.1200/JCO.1999.17.11.3396
57. Samantarrai D, Dash S, Chhetri B, Mallick B. Genomic and epigenomic cross-talks in the regulatory landscape of Mirnas in breast cancer. Mol Cancer Res (2013) 11(4):315–28. doi: 10.1158/1541-7786.MCR-12-0649
58. Lam SW, Jimenez CR, Boven E. Breast cancer classification by proteomic technologies: current state of knowledge. Cancer Treat Rev (2014) 40(1):129–38. doi: 10.1016/j.ctrv.2013.06.006
59. Tyanova S, Albrechtsen R, Kronqvist P, Cox J, Mann M, Geiger T. Proteomic maps of breast cancer subtypes. Nat Commun (2016) 7:10259. doi: 10.1038/ncomms10259
60. Michaut M, Chin SF, Majewski I, Severson TM, Bismeijer T, de Koning L, et al. Integration of genomic, transcriptomic and proteomic data identifies two biologically distinct subtypes of invasive lobular breast cancer. Sci Rep (2016) 6:18517. doi: 10.1038/srep18517
61. Santoro AL, Drummond RD, Silva IT, Ferreira SS, Juliano L, Vendramini PH, et al. In situ desi-msi lipidomic profiles of breast cancer molecular subtypes and precursor lesions. Cancer Res (2020) 80(6):1246–57. doi: 10.1158/0008-5472.CAN-18-3574
62. Diaz-Beltran L, Gonzalez-Olmedo C, Luque-Caro N, Diaz C, Martin-Blazquez A, Fernandez-Navarro M, et al. Human plasma metabolomics for biomarker discovery: targeting the molecular subtypes in breast cancer. Cancers (Basel) (2021) 13(1). doi: 10.3390/cancers13010147
63. Brockmoller SF, Bucher E, Muller BM, Budczies J, Hilvo M, Griffin JL, et al. Integration of metabolomics and expression of glycerol-3-phosphate acyltransferase (Gpam) in breast cancer-link to patient survival, hormone receptor status, and metabolic profiling. J Proteome Res (2012) 11(2):850–60. doi: 10.1021/pr200685r
64. Shin J, Kim G, Lee JW, Lee JE, Kim YS, Yu JH, et al. Identification of ganglioside Gm2 activator playing a role in cancer cell migration through proteomic analysis of breast cancer secretomes. Cancer Sci (2016) 107(6):828–35. doi: 10.1111/cas.12935
65. Banerjee S, Tian T, Wei Z, Shih N, Feldman MD, Peck KN, et al. Distinct microbial signatures associated with different breast cancer types. Front Microbiol (2018) 9:951. doi: 10.3389/fmicb.2018.00951
66. Negrini M, Calin GA. Breast cancer metastasis: A microrna story. Breast Cancer Res (2008) 10(2):203. doi: 10.1186/bcr1867
67. Ivey KN, Srivastava D. Micrornas as regulators of differentiation and cell fate decisions. Cell Stem Cell (2010) 7(1):36–41. doi: 10.1016/j.stem.2010.06.012
68. Wu J, Mamidi TKK, Zhang L, Hicks C. Unraveling the genomic-epigenomic interaction landscape in triple negative and non-triple negative breast cancer. Cancers (Basel) (2020) 12(6). doi: 10.3390/cancers12061559
69. Xie P, An R, Yu S, He J, Zhang H. A novel immune subtype classification of er-positive, Pr-negative and Her2-negative breast cancer based on the genomic and transcriptomic landscape. J Transl Med (2021) 19(1):398. doi: 10.1186/s12967-021-03076-x
70. Panis C, Pizzatti L, Herrera AC, Correa S, Binato R, Abdelhay E. Label-free proteomic analysis of breast cancer molecular subtypes. J Proteome Res (2014) 13(11):4752–72. doi: 10.1021/pr500676x
71. Asleh K, Negri GL, Spencer Miko SE, Colborne S, Hughes CS, Wang XQ, et al. Proteomic analysis of archival breast cancer clinical specimens identifies biological subtypes with distinct survival outcomes. Nat Commun (2022) 13(1):896. doi: 10.1038/s41467-022-28524-0
72. Fan Y, Zhou X, Xia TS, Chen Z, Li J, Liu Q, et al. Human plasma metabolomics for identifying differential metabolites and predicting molecular subtypes of breast cancer. Oncotarget (2016) 7(9):9925–38. doi: 10.18632/oncotarget.7155
73. Tang X, Lin CC, Spasojevic I, Iversen ES, Chi JT, Marks JR. A joint analysis of metabolomics and genetics of breast cancer. Breast Cancer Res (2014) 16(4):415. doi: 10.1186/s13058-014-0415-9
74. Roig B, Rodriguez-Balada M, Samino S, Lam EW, Guaita-Esteruelas S, Gomes AR, et al. Metabolomics reveals novel blood plasma biomarkers associated to the brca1-mutated phenotype of human breast cancer. Sci Rep (2017) 7(1):17831. doi: 10.1038/s41598-017-17897-8
75. Long M, Wang J, Yang M. Transcriptomic profiling of breast cancer cells induced by tumor-associated macrophages generates a robust prognostic gene signature. Cancers (Basel) (2022) 14(21). doi: 10.3390/cancers14215364
76. Gao Y, Jones A, Fasching PA, Ruebner M, Beckmann MW, Widschwendter M, et al. The integrative epigenomic-transcriptomic landscape of Er positive breast cancer. Clin Epigenet (2015) 7:126. doi: 10.1186/s13148-015-0159-0
77. Davalos V, Martinez-Cardus A, Esteller M. The epigenomic revolution in breast cancer: from single-gene to genome-wide next-generation approaches. Am J Pathol (2017) 187(10):2163–74. doi: 10.1016/j.ajpath.2017.07.002
78. Osz A, Lanczky A, Gyorffy B. Survival analysis in breast cancer using proteomic data from four independent datasets. Sci Rep (2021) 11(1):16787. doi: 10.1038/s41598-021-96340-5
79. Geiger T, Madden SF, Gallagher WM, Cox J, Mann M. Proteomic portrait of human breast cancer progression identifies novel prognostic markers. Cancer Res (2012) 72(9):2428–39. doi: 10.1158/0008-5472.CAN-11-3711
80. Bernhardt S, Bayerlova M, Vetter M, Wachter A, Mitra D, Hanf V, et al. Proteomic profiling of breast cancer metabolism identifies Shmt2 and Asct2 as prognostic factors. Breast Cancer Res (2017) 19(1):112. doi: 10.1186/s13058-017-0905-7
81. Cawthorn TR, Moreno JC, Dharsee M, Tran-Thanh D, Ackloo S, Zhu PH, et al. Proteomic analyses reveal high expression of decorin and endoplasmin (Hsp90b1) are associated with breast cancer metastasis and decreased survival. PLoS One (2012) 7(2):e30992. doi: 10.1371/journal.pone.0030992
82. Borcherding N, Cole K, Kluz P, Jorgensen M, Kolb R, Bellizzi A, et al. Re-evaluating E-cadherin and Beta-catenin: A pan-cancer proteomic approach with an emphasis on breast cancer. Am J Pathol (2018) 188(8):1910–20. doi: 10.1016/j.ajpath.2018.05.003
83. Hari PS, Balakrishnan L, Kotyada C, Everad John A, Tiwary S, Shah N, et al. Proteogenomic analysis of breast cancer transcriptomic and proteomic data, using de novo transcript assembly: genome-wide identification of novel peptides and clinical implications. Mol Cell Proteomics (2022) 21(4):100220. doi: 10.1016/j.mcpro.2022.100220
84. Kirmiz C, Li B, An HJ, Clowers BH, Chew HK, Lam KS, et al. A serum glycomics approach to breast cancer biomarkers. Mol Cell Proteomics (2007) 6(1):43–55. doi: 10.1074/mcp.M600171-MCP200
85. Porter DA, Krop IE, Nasser S, Sgroi D, Kaelin CM, Marks JR, et al. A sage (Serial analysis of gene expression) view of breast tumor progression. Cancer Res (2001) 61(15):5697–702.
86. Zhao QY, Lei PJ, Zhang X, Zheng JY, Wang HY, Zhao J, et al. Global histone modification profiling reveals the epigenomic dynamics during Malignant transformation in a four-stage breast cancer model. Clin Epigenet (2016) 8:34. doi: 10.1186/s13148-016-0201-x
87. Valo I, Raro P, Boissard A, Maarouf A, Jezequel P, Verriele V, et al. Olfm4 expression in ductal carcinoma in situ and in invasive breast cancer cohorts by a swath-based proteomic approach. Proteomics (2019) 19(21-22):e1800446. doi: 10.1002/pmic.201800446
88. Jobard E, Dossus L, Baglietto L, Fornili M, Lecuyer L, Mancini FR, et al. Investigation of circulating metabolites associated with breast cancer risk by untargeted metabolomics: A case-control study nested within the French E3n cohort. Br J Cancer (2021) 124(10):1734–43. doi: 10.1038/s41416-021-01304-1
89. Huang S, Chong N, Lewis NE, Jia W, Xie G, Garmire LX. Novel personalized pathway-based metabolomics models reveal key metabolic pathways for breast cancer diagnosis. Genome Med (2016) 8(1):34. doi: 10.1186/s13073-016-0289-9
90. Wei Y, Jasbi P, Shi X, Turner C, Hrovat J, Liu L, et al. Early breast cancer detection using untargeted and targeted metabolomics. J Proteome Res (2021) 20(6):3124–33. doi: 10.1021/acs.jproteome.1c00019
91. Santos CR, Schulze A. Lipid metabolism in cancer. FEBS J (2012) 279(15):2610–23. doi: 10.1111/j.1742-4658.2012.08644.x
92. Eghlimi R, Shi X, Hrovat J, Xi B, Gu H. Triple negative breast cancer detection using Lc-Ms/Ms lipidomic profiling. J Proteome Res (2020) 19(6):2367–78. doi: 10.1021/acs.jproteome.0c00038
93. Abba MC, Drake JA, Hawkins KA, Hu Y, Sun H, Notcovich C, et al. Transcriptomic changes in human breast cancer progression as determined by serial analysis of gene expression. Breast Cancer Res (2004) 6(5):R499–513. doi: 10.1186/bcr899
94. Desmedt C, Zoppoli G, Sotiriou C, Salgado R. Transcriptomic and genomic features of invasive lobular breast cancer. Semin Cancer Biol (2017) 44:98–105. doi: 10.1016/j.semcancer.2017.03.007
95. Curtis C, Shah SP, Chin SF, Turashvili G, Rueda OM, Dunning MJ, et al. The genomic and transcriptomic architecture of 2,000 breast tumours reveals novel subgroups. Nature (2012) 486(7403):346–52. doi: 10.1038/nature10983
96. Fox NS, Haider S, Harris AL, Boutros PC. Landscape of transcriptomic interactions between breast cancer and its microenvironment. Nat Commun (2019) 10(1):3116. doi: 10.1038/s41467-019-10929-z
97. Rojas K, Baliu-Pique M, Manzano A, Saiz-Ladera C, Garcia-Barberan V, Cimas FJ, et al. In silico transcriptomic mapping of integrins and immune activation in basal-like and Her2+ Breast cancer. Cell Oncol (Dordr) (2021) 44(3):569–80. doi: 10.1007/s13402-020-00583-9
98. Orlando FA, Brown KD. Unraveling breast cancer heterogeneity through transcriptomic and epigenomic analysis. Ann Surg Oncol (2009) 16(8):2270–9. doi: 10.1245/s10434-009-0500-y
99. Mao Y, Fu A, Hoffman AE, Jacobs DI, Jin M, Chen K, et al. The circadian gene Cry2 is associated with breast cancer aggressiveness possibly via epigenomic modifications. Tumour Biol (2015) 36(5):3533–9. doi: 10.1007/s13277-014-2989-3
100. Scumaci D, Tamme L, Fiumara CV, Pappaianni G, Concolino A, Leone E, et al. Plasma proteomic profiling in hereditary breast cancer reveals a brca1-specific signature: diagnostic and functional implications. PloS One (2015) 10(6):e0129762. doi: 10.1371/journal.pone.0129762
101. Luo X, Yu H, Song Y, Sun T. Integration of metabolomic and transcriptomic data reveals metabolic pathway alteration in breast cancer and impact of related signature on survival. J Cell Physiol (2019) 234(8):13021–31. doi: 10.1002/jcp.27973
102. Yang L, Cui X, Zhang N, Li M, Bai Y, Han X, et al. Comprehensive lipid profiling of plasma in patients with benign breast tumor and breast cancer reveals novel biomarkers. Anal Bioanal Chem (2015) 407(17):5065–77. doi: 10.1007/s00216-015-8484-x
103. Ujlaki G, Kovacs T, Vida A, Kokai E, Rauch B, Schwarcz S, et al. Identification of bacterial metabolites modulating breast cancer cell proliferation and epithelial-mesenchymal transition. Molecules (2023) 28(15). doi: 10.3390/molecules28155898
104. Wu Q, Li J, Zhu S, Wu J, Chen C, Liu Q, et al. Breast cancer subtypes predict the preferential site of distant metastases: A seer based study. Oncotarget (2017) 8(17):27990–6. doi: 10.18632/oncotarget.15856
105. Kennecke H, Yerushalmi R, Woods R, Cheang MC, Voduc D, Speers CH, et al. Metastatic behavior of breast cancer subtypes. J Clin Oncol (2010) 28(20):3271–7. doi: 10.1200/JCO.2009.25.9820
106. van 't Veer LJ, Dai H, van de Vijver MJ, He YD, Hart AA, Mao M, et al. Gene expression profiling predicts clinical outcome of breast cancer. Nature (2002) 415(6871):530–6. doi: 10.1038/415530a
107. Weigelt B, Hu Z, He X, Livasy C, Carey LA, Ewend MG, et al. Molecular portraits and 70-gene prognosis signature are preserved throughout the metastatic process of breast cancer. Cancer Res (2005) 65(20):9155–8. doi: 10.1158/0008-5472.CAN-05-2553
108. Akcakanat A, Zheng X, Cruz Pico CX, Kim TB, Chen K, Korkut A, et al. Genomic, transcriptomic, and proteomic profiling of metastatic breast cancer. Clin Cancer Res (2021) 27(11):3243–52. doi: 10.1158/1078-0432.CCR-20-4048
109. Smid M, Wang Y, Klijn JG, Sieuwerts AM, Zhang Y, Atkins D, et al. Genes associated with breast cancer metastatic to bone. J Clin Oncol (2006) 24(15):2261–7. doi: 10.1200/JCO.2005.03.8802
110. Kaufhold S, Bonavida B. Central role of snail1 in the regulation of Emt and resistance in cancer: A target for therapeutic intervention. J Exp Clin Cancer Res (2014) 33(1):62. doi: 10.1186/s13046-014-0062-0
111. Minn AJ, Gupta GP, Siegel PM, Bos PD, Shu W, Giri DD, et al. Genes that mediate breast cancer metastasis to lung. Nature (2005) 436(7050):518–24. doi: 10.1038/nature03799
112. Chen X, Zheng Z, Chen L, Zheng H. Mapk, Nfkappab, and Vegf signaling pathways regulate breast cancer liver metastasis. Oncotarget (2017) 8(60):101452–60. doi: 10.18632/oncotarget.20843
113. Tian C, Liu S, Wang Y, Song X. Prognosis and genomic landscape of liver metastasis in patients with breast cancer. Front Oncol (2021) 11:588136. doi: 10.3389/fonc.2021.588136
114. Berthelet J, Wimmer VC, Whitfield HJ, Serrano A, Boudier T, Mangiola S, et al. The site of breast cancer metastases dictates their clonal composition and reversible transcriptomic profile. Sci Adv (2021) 7(28). doi: 10.1126/sciadv.abf4408
115. Ciro M, Prosperini E, Quarto M, Grazini U, Walfridsson J, McBlane F, et al. Atad2 is a novel cofactor for Myc, overexpressed and amplified in aggressive tumors. Cancer Res (2009) 69(21):8491–8. doi: 10.1158/0008-5472.CAN-09-2131
116. Wang J, Hua H, Ran Y, Zhang H, Liu W, Yang Z, et al. Derlin-1 is overexpressed in human breast carcinoma and protects cancer cells from endoplasmic reticulum stress-induced apoptosis. Breast Cancer Res (2008) 10(1):R7. doi: 10.1186/bcr1849
117. Wang S, Li W, Liu N, Zhang F, Liu H, Liu F, et al. Nek2a contributes to tumorigenic growth and possibly functions as potential therapeutic target for human breast cancer. J Cell Biochem (2012) 113(6):1904–14. doi: 10.1002/jcb.24059
118. Baysal BE, Willett-Brozick JE, Taschner PE, Dauwerse JG, Devilee P, Devlin B. A high-resolution integrated map spanning the sdhd gene at 11q23: A 1.1-Mb Bac Contig, a partial transcript map and 15 new repeat polymorphisms in a tumour-suppressor region. Eur J Hum Genet (2001) 9(2):121–9. doi: 10.1038/sj.ejhg.5200585
119. Smith J, Tho LM, Xu N, Gillespie DA. The Atm-Chk2 and Atr-Chk1 pathways in DNA damage signaling and cancer. Adv Cancer Res (2010) 108:73–112. doi: 10.1016/B978-0-12-380888-2.00003-0
120. Aftimos P, Oliveira M, Irrthum A, Fumagalli D, Sotiriou C, Gal-Yam EN, et al. Genomic and transcriptomic analyses of breast cancer primaries and matched metastases in Aurora, the breast international group (Big) molecular screening initiative. Cancer Discov (2021) 11(11):2796–811. doi: 10.1158/2159-8290.CD-20-1647
121. Vishnubalaji R, Abdel-Razeq H, Gehani S, Albagha OME, Alajez NM. Identification of a gene panel predictive of triple-negative breast cancer response to neoadjuvant chemotherapy employing transcriptomic and functional validation. Int J Mol Sci (2022) 23(18). doi: 10.3390/ijms231810901
122. Gonzalez H, Hagerling C, Werb Z. Roles of the immune system in cancer: from tumor initiation to metastatic progression. Genes Dev (2018) 32(19-20):1267–84. doi: 10.1101/gad.314617.118
123. Foulds GA, Vadakekolathu J, Abdel-Fatah TMA, Nagarajan D, Reeder S, Johnson C, et al. Immune-phenotyping and transcriptomic profiling of peripheral blood mononuclear cells from patients with breast cancer: identification of a 3 gene signature which predicts relapse of triple negative breast cancer. Front Immunol (2018) 9:2028. doi: 10.3389/fimmu.2018.02028
124. Bera A, Russ E, Manoharan MS, Eidelman O, Eklund M, Hueman M, et al. Proteomic analysis of inflammatory biomarkers associated with breast cancer recurrence. Mil Med (2020) 185(Suppl 1):669–75. doi: 10.1093/milmed/usz254
125. Mehrotra J, Vali M, McVeigh M, Kominsky SL, Fackler MJ, Lahti-Domenici J, et al. Very high frequency of hypermethylated genes in breast cancer metastasis to the bone, brain, and lung. Clin Cancer Res (2004) 10(9):3104–9. doi: 10.1158/1078-0432.ccr-03-0118
126. Fang F, Turcan S, Rimner A, Kaufman A, Giri D, Morris LG, et al. Breast cancer methylomes establish an epigenomic foundation for metastasis. Sci Transl Med (2011) 3(75):75ra25. doi: 10.1126/scitranslmed.3001875
127. Messier TL, Gordon JA, Boyd JR, Tye CE, Browne G, Stein JL, et al. Histone H3 lysine 4 acetylation and methylation dynamics define breast cancer subtypes. Oncotarget (2016) 7(5):5094–109. doi: 10.18632/oncotarget.6922
128. Wu J, Yang R, Zhang L, Li Y, Liu B, Kang H, et al. Metabolomics research on potential role for 9-cis-retinoic acid in breast cancer progression. Cancer Sci (2018) 109(7):2315–26. doi: 10.1111/cas.13629
129. Kawashima M, Tokiwa M, Nishimura T, Kawata Y, Sugimoto M, Kataoka TR, et al. High-resolution imaging mass spectrometry combined with transcriptomic analysis identified a link between fatty acid composition of phosphatidylinositols and the immune checkpoint pathway at the primary tumour site of breast cancer. Br J Cancer (2020) 122(2):245–57. doi: 10.1038/s41416-019-0662-8
130. Parida S, Wu S, Siddharth S, Wang G, Muniraj N, Nagalingam A, et al. A procarcinogenic colon microbe promotes breast tumorigenesis and metastatic progression and concomitantly activates notch and Beta-catenin axes. Cancer Discov (2021) 11(5):1138–57. doi: 10.1158/2159-8290.CD-20-0537
131. Parhi L, Alon-Maimon T, Sol A, Nejman D, Shhadeh A, Fainsod-Levi T, et al. Breast cancer colonization by fusobacterium nucleatum accelerates tumor growth and metastatic progression. Nat Commun (2020) 11(1):3259. doi: 10.1038/s41467-020-16967-2
132. Rocca A, Braga L, Volpe MC, Maiocchi S, Generali D. The predictive and prognostic role of Ras-Raf-Mek-Erk pathway alterations in breast cancer: revision of the literature and comparison with the analysis of cancer genomic datasets. Cancers (Basel) (2022) 14(21). doi: 10.3390/cancers14215306
133. Gromova I, Espinoza JA, Grauslund M, Santoni-Rugiu E, Moller Talman ML, van Oostrum J, et al. Functional proteomic profiling of triple-negative breast cancer. Cells (2021) 10(10). doi: 10.3390/cells10102768
134. Evensen NA, Kuscu C, Nguyen HL, Zarrabi K, Dufour A, Kadam P, et al. Unraveling the role of Kiaa1199, a novel endoplasmic reticulum protein, in cancer cell migration. J Natl Cancer Inst (2013) 105(18):1402–16. doi: 10.1093/jnci/djt224
135. Jami MS, Hou J, Liu M, Varney ML, Hassan H, Dong J, et al. Functional proteomic analysis reveals the involvement of Kiaa1199 in breast cancer growth, motility and invasiveness. BMC Cancer (2014) 14:194. doi: 10.1186/1471-2407-14-194
136. Ternette N, Olde Nordkamp MJM, Muller J, Anderson AP, Nicastri A, Hill AVS, et al. Immunopeptidomic profiling of Hla-A2-positive triple negative breast cancer identifies potential immunotherapy target antigens. Proteomics (2018) 18(12):e1700465. doi: 10.1002/pmic.201700465
137. Yi X, Liao Y, Wen B, Li K, Dou Y, Savage SR, et al. Caatlas: an immunopeptidome atlas of human cancer. iScience (2021) 24(10):103107. doi: 10.1016/j.isci.2021.103107
138. Xiao Y, Ma D, Yang YS, Yang F, Ding JH, Gong Y, et al. Comprehensive metabolomics expands precision medicine for triple-negative breast cancer. Cell Res (2022) 32(5):477–90. doi: 10.1038/s41422-022-00614-0
139. Purwaha P, Gu F, Piyarathna DWB, Rajendiran T, Ravindran A, Omilian AR, et al. Unbiased lipidomic profiling of triple-negative breast cancer tissues reveals the association of sphingomyelin levels with patient disease-free survival. Metabolites (2018) 8(3). doi: 10.3390/metabo8030041
140. Bartz SR, Zhang Z, Burchard J, Imakura M, Martin M, Palmieri A, et al. Small interfering Rna screens reveal enhanced cisplatin cytotoxicity in tumor cells having both Brca network and Tp53 disruptions. Mol Cell Biol (2006) 26(24):9377–86. doi: 10.1128/MCB.01229-06
141. Berns K, Horlings HM, Hennessy BT, Madiredjo M, Hijmans EM, Beelen K, et al. A functional genetic approach identifies the pi3k pathway as a major determinant of trastuzumab resistance in breast cancer. Cancer Cell (2007) 12(4):395–402. doi: 10.1016/j.ccr.2007.08.030
142. Turner NC, Lord CJ, Iorns E, Brough R, Swift S, Elliott R, et al. A synthetic lethal sirna screen identifying genes mediating sensitivity to a parp inhibitor. EMBO J (2008) 27(9):1368–77. doi: 10.1038/emboj.2008.61
143. Iorns E, Turner NC, Elliott R, Syed N, Garrone O, Gasco M, et al. Identification of Cdk10 as an important determinant of resistance to endocrine therapy for breast cancer. Cancer Cell (2008) 13(2):91–104. doi: 10.1016/j.ccr.2008.01.001
144. Li CL, Moi SH, Lin HS, Hou MF, Chen FM, Shih SL, et al. Comprehensive transcriptomic and proteomic analyses identify a candidate gene set in cross-resistance for endocrine therapy in breast cancer. Int J Mol Sci (2022) 23(18). doi: 10.3390/ijms231810539
145. Yang WS, Moon HG, Kim HS, Choi EJ, Yu MH, Noh DY, et al. Proteomic approach reveals Fkbp4 and S100a9 as potential prediction markers of therapeutic response to neoadjuvant chemotherapy in patients with breast cancer. J Proteome Res (2012) 11(2):1078–88. doi: 10.1021/pr2008187
146. Diaz C, Gonzalez-Olmedo C, Diaz-Beltran L, Camacho J, Mena Garcia P, Martin-Blazquez A, et al. Predicting dynamic response to neoadjuvant chemotherapy in breast cancer: A novel metabolomics approach. Mol Oncol (2022) 16(14):2658–71. doi: 10.1002/1878-0261.13216
147. Debik J, Euceda LR, Lundgren S, Gythfeldt HVL, Garred O, Borgen E, et al. Assessing treatment response and prognosis by serum and tissue metabolomics in breast cancer patients. J Proteome Res (2019) 18(10):3649–60. doi: 10.1021/acs.jproteome.9b00316
148. Vignoli A, Muraro E, Miolo G, Tenori L, Turano P, Di Gregorio E, et al. Effect of estrogen receptor status on circulatory immune and metabolomics profiles of Her2-positive breast cancer patients enrolled for neoadjuvant targeted chemotherapy. Cancers (Basel) (2020) 12(2). doi: 10.3390/cancers12020314
149. Cardoso MR, Silva AAR, Talarico MCR, Sanches PHG, Sforca ML, Rocco SA, et al. Metabolomics by nmr combined with machine learning to predict neoadjuvant chemotherapy response for breast cancer. Cancers (Basel) (2022) 14(20). doi: 10.3390/cancers14205055
150. Sammut SJ, Crispin-Ortuzar M, Chin SF, Provenzano E, Bardwell HA, Ma W, et al. Multi-omic machine learning predictor of breast cancer therapy response. Nature (2022) 601(7894):623–9. doi: 10.1038/s41586-021-04278-5
151. Takeshita T, Yan L, Peng X, Kimbung S, Hatschek T, Hedenfalk IA, et al. Transcriptomic and functional pathway features were associated with survival after pathological complete response to neoadjuvant chemotherapy in breast cancer. Am J Cancer Res (2020) 10(8):2555–69.
152. He X, Gu J, Zou D, Yang H, Zhang Y, Ding Y, et al. Nmr-based metabolomics analysis predicts response to neoadjuvant chemotherapy for triple-negative breast cancer. Front Mol Biosci (2021) 8:708052. doi: 10.3389/fmolb.2021.708052
153. Tsimberidou AM, Wen S, Hong DS, Wheler JJ, Falchook GS, Fu S, et al. Personalized medicine for patients with advanced cancer in the phase I program at Md Anderson: validation and landmark analyses. Clin Cancer Res (2014) 20(18):4827–36. doi: 10.1158/1078-0432.CCR-14-0603
154. Bogaerts J, Cardoso F, Buyse M, Braga S, Loi S, Harrison JA, et al. Gene signature evaluation as a prognostic tool: challenges in the design of the mindact trial. Nat Clin Pract Oncol (2006) 3(10):540–51. doi: 10.1038/ncponc0591
155. Mook S, Van't Veer LJ, Rutgers EJ, Piccart-Gebhart MJ, Cardoso F. Individualization of therapy using mammaprint: from development to the mindact trial. Cancer Genomics Proteomics (2007) 4(3):147–55.
156. Cardoso F, Van't Veer L, Rutgers E, Loi S, Mook S, Piccart-Gebhart MJ. Clinical application of the 70-gene profile: the Mindact trial. J Clin Oncol (2008) 26(5):729–35. doi: 10.1200/JCO.2007.14.3222
157. van de Vijver MJ, He YD, van't Veer LJ, Dai H, Hart AA, Voskuil DW, et al. A gene-expression signature as a predictor of survival in breast cancer. N Engl J Med (2002) 347(25):1999–2009. doi: 10.1056/NEJMoa021967
158. Rutgers E, Piccart-Gebhart MJ, Bogaerts J, Delaloge S, Veer LV, Rubio IT, et al. The Eortc 10041/Big 03-04 Mindact trial is feasible: results of the pilot phase. Eur J Cancer (2011) 47(18):2742–9. doi: 10.1016/j.ejca.2011.09.016
159. Cardoso F, van't Veer LJ, Bogaerts J, Slaets L, Viale G, Delaloge S, et al. 70-gene signature as an aid to treatment decisions in early-stage breast cancer. N Engl J Med (2016) 375(8):717–29. doi: 10.1056/NEJMoa1602253
160. Piccart M, van 't Veer LJ, Poncet C, Lopes Cardozo JMN, Delaloge S, Pierga JY, et al. 70-gene signature as an aid for treatment decisions in early breast cancer: updated results of the phase 3 randomised mindact trial with an exploratory analysis by age. Lancet Oncol (2021) 22(4):476–88. doi: 10.1016/S1470-2045(21)00007-3
161. Lopes Cardozo JMN, Byng D, Drukker CA, Schmidt MK, Binuya MA, van 't Veer LJ, et al. Outcome without any adjuvant systemic treatment in stage I Er+/Her2- breast cancer patients included in the mindact trial. Ann Oncol (2022) 33(3):310–20. doi: 10.1016/j.annonc.2021.11.014
162. Delaloge S, Piccart M, Rutgers E, Litiere S, van 't Veer LJ, van den Berkmortel F, et al. Standard anthracycline based versus docetaxel-capecitabine in early high clinical and/or genomic risk breast cancer in the Eortc 10041/Big 3-04 Mindact phase iii trial. J Clin Oncol (2020) 38(11):1186–97. doi: 10.1200/JCO.19.01371
163. Westphal T, Gampenrieder SP, Rinnerthaler G, Balic M, Posch F, Dandachi N, et al. Transferring mindact to daily routine: implementation of the 70-gene signature in luminal early breast cancer - results from a prospective registry of the Austrian group medical tumor therapy (Agmt). Breast Care (Basel) (2022) 17(1):1–9. doi: 10.1159/000512467
164. Sparano JA. Tailorx: trial assigning individualized options for treatment (Rx). Clin Breast Cancer (2006) 7(4):347–50. doi: 10.3816/CBC.2006.n.051
165. Sparano JA, Gray RJ, Makower DF, Albain KS, Saphner TJ, Badve SS, et al. Clinical outcomes in early breast cancer with a high 21-gene recurrence score of 26 to 100 assigned to adjuvant chemotherapy plus endocrine therapy: A secondary analysis of the tailorx randomized clinical trial. JAMA Oncol (2020) 6(3):367–74. doi: 10.1001/jamaoncol.2019.4794
166. Sparano JA, Gray RJ, Makower DF, Pritchard KI, Albain KS, Hayes DF, et al. Adjuvant chemotherapy guided by a 21-gene expression assay in breast cancer. N Engl J Med (2018) 379(2):111–21. doi: 10.1056/NEJMoa1804710
167. Albain KS, Gray RJ, Makower DF, Faghih A, Hayes DF, Geyer CE, et al. Race, ethnicity, and clinical outcomes in hormone receptor-positive, Her2-negative, node-negative breast cancer in the randomized Tailorx trial. J Natl Cancer Inst (2021) 113(4):390–9. doi: 10.1093/jnci/djaa148
168. Garcia-Recio S, Hinoue T, Wheeler GL, Kelly BJ, Garrido-Castro AC, Pascual T, et al. Multiomics in primary and metastatic breast tumors from the Aurora us network finds microenvironment and epigenetic drivers of metastasis. Nat Cancer (2023) 4(1):128–47. doi: 10.1038/s43018-022-00491-x
Keywords: breast cancer, early-stage, cancer progression, metastasis, omics, biomarkers
Citation: Alvarez-Frutos L, Barriuso D, Duran M, Infante M, Kroemer G, Palacios-Ramirez R and Senovilla L (2023) Multiomics insights on the onset, progression, and metastatic evolution of breast cancer. Front. Oncol. 13:1292046. doi: 10.3389/fonc.2023.1292046
Received: 10 September 2023; Accepted: 23 November 2023;
Published: 19 December 2023.
Edited by:
Yusuke Suenaga, Chiba Cancer Center, JapanReviewed by:
Barani Kumar Rajendran, Yale University, United StatesCopyright © 2023 Alvarez-Frutos, Barriuso, Duran, Infante, Kroemer, Palacios-Ramirez and Senovilla. This is an open-access article distributed under the terms of the Creative Commons Attribution License (CC BY). The use, distribution or reproduction in other forums is permitted, provided the original author(s) and the copyright owner(s) are credited and that the original publication in this journal is cited, in accordance with accepted academic practice. No use, distribution or reproduction is permitted which does not comply with these terms.
*Correspondence: Laura Senovilla, bGF1cmFzZW5vdmlsbGFAaG90bWFpbC5jb20=
Disclaimer: All claims expressed in this article are solely those of the authors and do not necessarily represent those of their affiliated organizations, or those of the publisher, the editors and the reviewers. Any product that may be evaluated in this article or claim that may be made by its manufacturer is not guaranteed or endorsed by the publisher.
Research integrity at Frontiers
Learn more about the work of our research integrity team to safeguard the quality of each article we publish.