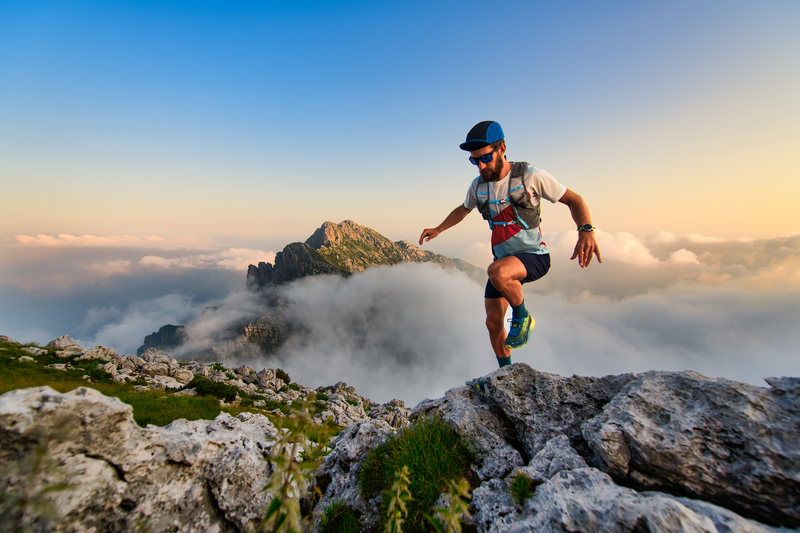
95% of researchers rate our articles as excellent or good
Learn more about the work of our research integrity team to safeguard the quality of each article we publish.
Find out more
SYSTEMATIC REVIEW article
Front. Oncol. , 20 December 2023
Sec. Gynecological Oncology
Volume 13 - 2023 | https://doi.org/10.3389/fonc.2023.1289050
Background: The early identification of lymph node metastasis status in endometrial cancer (EC) is a serious challenge in clinical practice. Some investigators have introduced machine learning into the early identification of lymph node metastasis in EC patients. However, the predictive value of machine learning is controversial due to the diversity of models and modeling variables. To this end, we carried out this systematic review and meta-analysis to systematically discuss the value of machine learning for the early identification of lymph node metastasis in EC patients.
Methods: A systematic search was conducted in Pubmed, Cochrane, Embase, and Web of Science until March 12, 2023. PROBAST was used to assess the risk of bias in the included studies. In the process of meta-analysis, subgroup analysis was performed according to modeling variables (clinical features, radiomic features, and radiomic features combined with clinical features) and different types of models in various variables.
Results: This systematic review included 50 primary studies with a total of 103,752 EC patients, 12,579 of whom had positive lymph node metastasis. Meta-analysis showed that among the machine learning models constructed by the three categories of modeling variables, the best model was constructed by combining radiomic features with clinical features, with a pooled c-index of 0.907 (95%CI: 0.886-0.928) in the training set and 0.823 (95%CI: 0.757-0.890) in the validation set, and good sensitivity and specificity. The c-index of the machine learning model constructed based on clinical features alone was not inferior to that based on radiomic features only. In addition, logistic regression was found to be the main modeling method and has ideal predictive performance with different categories of modeling variables.
Conclusion: Although the model based on radiomic features combined with clinical features has the best predictive efficiency, there is no recognized specification for the application of radiomics at present. In addition, the logistic regression constructed by clinical features shows good sensitivity and specificity. In this context, large-sample studies covering different races are warranted to develop predictive nomograms based on clinical features, which can be widely applied in clinical practice.
Systematic review registration: https://www.crd.york.ac.uk/PROSPERO, identifier CRD42023420774.
Endometrial cancer (EC) is the most common gynecological cancer in high-income countries. In 2020, 417,367 women were diagnosed with EC worldwide. Compared with low-income and middle-income countries, EC is more common in high-income regions. The regions with the highest EC diagnosis are North America and Western Europe, and the incidence rate of EC seems to be increasing rapidly (1, 2). EC is a serious threat to women’s lives. As of 2018, the incidence and mortality of women with EC in Europe were 19.2-20.2/100,000 and 2.0-3.7/100,000 (3, 4), respectively.
Surgery is the main treatment for patients with localized EC. However, whether lymphadenectomy is necessary during surgery is controversial, and whether para-aortic lymphadenectomy should be added to pelvic lymphadenectomy has been disputed (2, 5). Previously, all patients were advised to undergo complete standard lymphadenectomy (i.e., dissection and evaluation of pelvic and para-aortic lymph nodes), but this was associated with more side effects (6). Therefore, the effective preoperative assessment of lymph node metastasis is of profound significance in clinical practice. Unfortunately, there is a lack of efficient preoperative assessment methods. The Mayo criteria are widely applied in clinical practice for predicting the risk of lymph node metastasis in EC (7). However, its true prediction accuracy still needs to be further improved.
With the gradual improvement of statistical theory, researchers have gradually applied machine learning methods (especially supervised machine learning methods) into clinical practice, mainly for the diagnosis of disease status (8, 9), the prediction of disease occurrence (10, 11), or the prediction of prognosis (12, 13). In some fields, the accuracy of machine learning in screening or diagnosing diseases is not inferior to human clinical practice (14, 15). In this context, some investigators have tried to apply machine learning methods to the identification of preoperative lymph node metastasis in EC. However, machine learning includes diversified mathematical modeling methods (such as logistic regression, random forest, support vector machine, and artificial neural network), and machine learning models also involve a wide range of modeling variables (such as radiomic features, clinical features, and pathological imaging). As modeling methods and modeling variables are diversified, there is a lack of comprehensive and systematic understanding of the preoperative diagnostic value of machine learning for lymph node metastasis status in EC patients (16). Therefore, this systematic review and meta-analysis was conducted to explore the predictive value of machine learning for lymph node metastasis in EC patients. Also, we comprehensively summarized the effective predictive variables and compared the predictive values of clinical and radiomic features for lymph node metastasis in EC patients.
This study followed the Preferred Reporting Items for Systematic Reviews and Meta-analyses (PRISMA 2020) and was prospectively registered on PROSPERO (ID: CRD42023420774).
(1) Study subjects were patients diagnosed with EC;
(2) Study types were case-control study, cohort study, nested case-control study, and case-cohort study;
(3) Studies with a complete machine learning model for the prediction of lymph node metastasis status;
(4) Studies constructing a corresponding machine learning model but lacking external validation or independent validation set;
(5) Studies on different types of machine learning constructed from the same dataset;
(6) Studies reported in English.
(1) Study types were meta-analysis, review, guidelines, expert opinions, etc.;
(2) Studies with risk factors analyzed only but no complete risk model constructed;
(3) Studies lacking outcome indicators (Roc, c-statistic, c-index, sensitivity, specificity, accuracy, recovery, precision, confusion matrix, diagnostic fourfold table, F1 score, calibration curve) for the prediction accuracy of the risk model;
(4) Studies to validate maturity scale;
(5) Studies with the accuracy predicted by single factor.
A systematic search was conducted in Pubmed, Embase, Web of Science, and Cochrane until March 12, 2023, using subject terms and free terms. No restrictions were imposed on publication regions. The complete search strategy is shown in Table S1.
The retrieved studies were imported into Endnote X9 to automatically and manually remove duplicate publications. Then, the titles or abstracts were checked to obtain primary studies that were initially eligible. Finally, the full texts of the remaining studies were read to include primary studies that were eligible for this systematic overview.
Before data extraction, a standardized data extraction form was developed. Extracted data encompassed title, first author, publication year, study type (case-control, retrospective/prospective cohort study, nested cohort study, case-cohort study), patient source (single-center, multi-center, registry database), FIGO stage for tumor, number of cases with lymph node metastasis (training set, validation set), total number of cases, generation method of validation set (internal validation: random sampling, k-fold cross-validation, leave-one-out; external validation: prospective, multi-center; over-fitting method: k- fold cross-validation, bootstrap), missing value handling method, variable screening/feature selection method, types of mathematical models, and modeling variables.
Two investigators (RZL and YJY) independently screened the literature, extracted data, and cross-checked the data. In case of disagreement, a third investigator (LYQ) participated in discussions and decisions.
PROBAST was used to assess the risk of bias in the included studies, including several questions in four different domains: participants, predictive variables, results, and statistical analysis, which reflected the overall risk of bias and overall application (17). The four domains contained 2, 3, 6, and 9 questions in specificity respectively, each of which had three answers (yes/probably yes, no/probably no, and no available information). A domain was categorized as having a high risk of bias if at least one specific question in the domain indicated “no/probably no”. A domain was categorized as having a low risk of bias if all specific questions in the domain indicated “yes/probably yes”. A domain was categorized as having an unclear risk of bias if all specific questions in the domain indicated “no/probably no” with at least one “no available information”. The PROBAST was used to assess the machine learning models in the included literature.
Two investigators (RZL and YJY) independently assessed and cross-checked the risk of bias. In case of disagreement, a third investigator (LYQ) participated in discussions and decisions.
The outcome indicator in this systematic review was the c-index, which reflected the overall accuracy of the model. However, the c-index cannot reflect the accuracy of the model in predicting lymph node metastasis, especially when there is a serious imbalance in the number of lymph node metastasis and non-metastasis samples. Even if a high c-index is presented, it may be caused by the high accuracy of the model in predicting negative events (lymph node non-metastasis). Therefore, outcome indicators of this systematic review also included the sensitivity and specificity of machine learning models in predicting lymph node metastasis. In addition, we also summarized the modeling variables. As the machine learning models constructed clinically is mainly logistic regression, in order to try to construct the logistic regression risk equation for lymph node metastasis, outcome indicators also included the odds ratio (OR) of each modeling variable for constructing logistic regression.
The c-index, its standard error (SE), and 95% confidence interval (95%CI) should be provided for meta-analysis of c-index. However, since many included studies lacked the SE and 95%CI of c-index, the SE of c-index was estimated with reference to the study conducted by Debray TP et al. (18). This study also performed meta-analysis on sensitivity and specificity, for which a diagnostic fourfold table was required in the included studies. However, the included studies only provided outcome indicators such as sensitivity, specificity, precision and accuracy, so we developed the diagnostic fourfold table by combining the number of cases with lymph node metastasis and the total number of cases. In addition, some original studies only provided the receiver operating characteristic curve (Roc) of the machine learning model. In this case, we extracted the sensitivity and specificity on the Roc curve by using Origen2021, selected the sensitivity and specificity by using the best Youden’s index, and then developed the diagnostic fourfold table by combining the number of cases and the total number of cases. Moreover, the included studies converted continuous variables into categorical variables or remained them in the original continuous state when summarizing the OR values of modeling variables in Logistic regression, so we conducted meta-analysis of continuous variables.
In meta-analyses of c-index and OR values of modeling variables, a random-effects model was used when heterogeneity index I2≥50%, and a fixed-effects model was used when I2<50%. The meta-analysis of sensitivity and specificity was performed using a bivariate mixed-effects model.
In addition, the modeling variables consisted of clinical features, radiomic features, and radiomic + clinical features, and there were also diversified machine learning models. Therefore, subgroup analyses were conducted according to modeling variables and model types. This meta-analysis was performed in R4.2.0 (R development Core Team, Vienna, http://www.R-project.org), with a P value less than 0.05 indicating statistical significance.
A total of 3,033 studies were retrieved, including 782 duplicate studies marked by Endnote. Endnote can only mark the literature with a completely consistent title and author’s writing style. However, a large number of duplicate studies had slight differences in these aspects, making it difficult to mark them automatically. Therefore, there were 356 studies that were manually identified duplicates. Then, after reading the titles or abstracts of the remaining literature, 62 primary studies were initially eligible, and their full texts were downloaded. After reading the full texts, 50 studies were finally included in this systematic review (19–68). The literature screening process is shown in Figure 1.
The 50 included studies covered a total of 103,752 EC patients, of whom 12,579 EC patients had positive lymph node metastasis. The included studies were published from 2013 to 2023, mainly from 2016 to 2023. Study types included case-control study and cohort study. Among them, there were only 10 cohort studies (28, 35, 39, 49, 51–53, 62–64), 11 multicenter studies (26, 30, 33, 45, 46, 60–64, 66), and 1 study (40) from the SEER database, and the remaining studies were single-center studies. The median number of cases is 342 (IQR: 200~661), and the median number of cases in training sets is 300 (IQR: 154~533). Only 29 studies (19–21, 23, 25, 26, 29–33, 36–38, 40, 43, 45, 47–50, 52, 54, 55, 58, 60–63) had independent validation sets. Among them, 11 studies (21, 30, 31, 33, 36, 45, 55, 61–63, 65) adopted the method of external validation, and the remaining studies carried out internal validation by random sampling. Nine studies (20–22, 31, 32, 35, 45, 52) did not clearly describe the variable screening method and mainly adopted single-factor and multi-factor logistic regression.
14 studies (22, 23, 25, 26, 32, 34, 36, 37, 45, 47–50, 52, 55) had modeling variables from radiomic features and included a total of 27 models, which were divided into the following categories of diversified mathematical models: logistic regression (n=16), ridge regression (n=2), J48 (Decision tree (n=1)), random forest (n=1), support vector machine (n=2), XGBoost (n=1), artificial neural network (n=2), Hoeffding tree (n=1), and ResNet-18 (n=1). The remaining studies had modeling variables from clinical features and included a total of 41 models, which were divided into the following categories of diversified mathematical models: logistic regression (n=38), ridge regression (n=1), random forest (n=1), and support vector machine (n=1). The basic information is detailed in Table 1.
The original studies included 39 case-control studies, based on which the machine learning model constructed was rated as high risk of bias for Populations by PROBAST. Also, it was unclear whether the assessment of predictive factors was carried out under the condition of known lymph node metastasis status in a large number of single-center case-control studies, based on which the machine learning model constructed was rated as high risk of bias for Prediction factors by PROBAST. In contrast, it was clear that the assessment of Results was carried out under the condition of confirmed lymph node metastasis status by biopsy in a large number of single-center case-control studies, based on which the machine learning model constructed was rated as high risk of bias for very few Results. In addition, according to Statistic analysis, the number of cases in the training set needed to meet EVP≥20. An independent validation set was required, and the number of cases in the validation set should be>100, leading to a main high risk of bias. The results of the risk of bias assessment are provided in Figure 2.
Eight datasets from the included studies were used to validate the accuracy of Meyo criteria for predicting lymph node metastasis in EC patients. The results of meta-analysis showed that the c-index in the training set was 0.690 (95%CI: 0.640-0.740), the sensitivity was 0.81 (95%CI: 0.66-0.90), and the specificity was 0.59 (95%CI: 0.38-0.77). The detailed results are shown in Tables 2, 3.
Table 2 Meta-analysis results of c-index for predicting lymph node metastasis in EC patients using machine learning.
Table 3 Meta-analysis results of sensitivity and specificity of machine learning in predicting lymph node metastasis in EC patients.
In the included studies, there were a total of 41 machine learning models constructed based on clinical features alone. The training set contained 36 machine learning models with a pooled c-index of 0.824 (95%CI: 0.806-0.843), and the validation set contained 19 machine learning models with a pooled c-index of 0.793 (95%CI: 0.756-0.829). The pooled sensitivity and specificity in the training set were 0.81 (95%CI: 0.77-0.84) and 0.75(95%CI: 0.71-0.79), and 0.75 (95%CI: 0.67-0.82) and 0.78 (95%CI: 0.74-0.82) in the validation set respectively. The detailed results are shown in Tables 2, 3.
In the included studies, there were a total of 16 machine learning models constructed based on radiomic features alone. The pooled c-index was 0.798 (95%CI: 0.758-0.837) in the training set and 0.810 (95%CI: 0.770-0.850) in the validation set. The pooled sensitivity and specificity in the training set were 0.82 (95%CI: 0.79-0.85) and 0.83 (95%CI: 0.79-0.87), and 0.77 (95%CI: 0.64-0.87) and 0.84 (95%CI: 0.74-0.91) in the validation set, respectively. The detailed results are shown in Tables 2, 3.
In the included studies, there were a total of 11 machine learning models constructed based on radiomic features combined with clinical features. The pooled c-index was 0.907 (95%CI: 0.886-0.928) in the training set and 0.823 (95%CI: 0.757-0.890) in the validation set. The pooled sensitivity and specificity in the training set were 0.88 (95%CI: 0.84-0.92) and 0.83 (95%CI: 0.79-0.87), and 0.77 (95%CI: 0.64-0.87) and 0.84 (95%CI: 0.74-0.91) in the validation set, respectively. The detailed results are shown in Tables 2, 3.
Subgroup analyses were performed by the type of machine learning models constructed based on clinical features, radiomic features, and radiomic features combined with clinical features. The models for different modeling variables were mainly logistic regression, and most of the studies also constructed the visual Nomograms. The results of meta-analysis showed that logistic regression had a good predictive value not inferior to that of other machine learning models for the same modeling variable.
In the logistic regression constructed based on clinical features alone, the pooled c-index, sensitivity and specificity were 0.828 (95%CI: 0.809~0.846), 0.81 (95%CI: 0.78-0.84) and 0.75 (95% CI: 0.71-0.79) in the training set, and 0.806 (95%CI: 0.758-0.853), 0.81 (95%CI: 0.78-0.84) and 0.75 (95%CI: 0.71-0.79) in the validation set, respectively, as shown in Figures S1 and S2.
In the logistic regression constructed based on radiomic features alone, the pooled c-index, sensitivity and specificity were 0.850 (95%CI: 0.815-0.884), 0.83 (95%CI: 0.75-0.88) and 0.79 (95%CI: 0.76-0.82) in the training set, and 0.818 (95%CI: 0.775-0.861), 0.77 (95%CI: 0.60-0.88) and 0.86 (95%CI: 0.75-0.93) in the validation set, respectively, as shown in Figure S3 and S4.
In the logistic regression constructed based on radiomic features combined with clinical features, the pooled c-index, sensitivity and specificity in the training set were 0.905 (95%CI: 0.885-0.925), 0.90 (95%CI: 0.84-0.94) and 0.80 (95%CI: 0.72-0.86), respectively, and those in the validation set were 0.842 (95%CI: 0.793-0.891), 0.90 (95%CI: 0.84-0.94) and 0.80 (95%CI: 0.72-0.86), respectively, as shown in Figure S5, S6.
Among the machine learning models constructed by the same type of modeling variables, logistic regression was not inferior to other models in the predictive value. We summarized the modeling variables included in logistic regression, and the results of meta-analysis showed that Grade, Histological type, Myometrial invasion, Cervical stromal invasion, LVSI, CA125, CA153, CA199, Ki67, P53, Tumor size, ER, Enlarged lymph nodes, Mitosis and SII were effective predictive variables (P<0.05) of lymph node metastasis status in EC, as shown in Table 4 and Figures S7-S12.
Table 4 Meta-analysis results of the OR of modeling variables used to construct a Logistic regression model for predicting lymph node metastasis in EC.
Preoperative identification of the status of lymph node metastases in EC patients is of profound clinical significance. For EC patients, some postoperative complications can seriously affect the quality of life of surviving patients. Among them, lymphoedema is one of the adverse complications that we need to pay attention to (69). Lymphadenectomy increases the risk of lymphoedema (70). Although the technique of sentinel lymph node (SLN) biopsy is used to infer the surgical staging of EC (71), however, researchers are still actively exploring some artificial intelligence-based lymph node metastasis detection tools.
This study showed that the modeling variables for predicting lymph node metastasis status in EC patients included clinical features, radiomic features, and radiomic combined with clinical features. Among all types of modeling methods, logistic regression was mostly used to construct a nomogram, and it seems to have a c-index not inferior to that of other models in the training set and validation set. In addition, the c-index of the machine learning model constructed based on clinical features alone was not inferior to that of the machine learning model constructed based on radiomic features alone. In terms of the nomogram based on logistic regression, the c-index of the nomogram based on clinical features alone was close to that of the nomogram based on radiomic features alone. The machine learning method with the best predictive value was the one constructed based on radiomic features combined with clinical features, which was also applied to the nomogram.
Previous clinical research explored the accuracy of preoperative detection for lymph node metastasis in EC patients by using CT, MRI, PET/CT, ultrasound and other imaging approaches, mainly MRI and PET/CT. Bollineni VR et al. (72) systematically reviewed 13 original studies and reported that the sensitivity and specificity of 18F-FDG PET/CT in preoperative detection of lymph node metastasis in EC patients were 0.72 (95% CI: 0.55 ~ 0.98) and 0.92 (95% CI: 0.84 ~ 0.97), respectively. A recent study showed that the sensitivity of 18F-FDG PET and PET/CT in preoperative detection of lymph node metastasis in EC patients was 0.68 (95% CI: 0.63 ~ 0.73) and 0.96 (95% CI: 0.96 ~ 0.97), respectively (73). Qiu et al. (74) systematically reviewed 14 studies and found that the sensitivity and specificity of MRI for preoperative prediction of pelvic or/and para-aortic lymph node metastasis in EC patients were 0.59 (95%CI: 0.48 ~ 0.69) and 0.95 (95%CI: 0.93 ~ 0.96), while those of MRI for preoperative prediction of pelvic lymph node metastasis were 0.65 (95%CI: 0.51 ~ 0.77) and 0.95 (95%CI: 0.93 ~ 0.96). A systematic review by Luomaranta A et al. (75) on the preoperative detection of EC patients by MRI showed similar sensitivity and specificity to that reported by Qiu et al. The detection rate of lymph node metastasis in EC patients by ultrasound seems to be unsatisfactory (76). Thus, the preoperative detection of lymph node metastasis in EC patients by imaging approaches had a good specificity, but a seriously insufficient sensitivity. Our study showed that the machine learning method had a better sensitivity (> 0.8), and the machine learning model constructed based on clinical features had a higher sensitivity but a lower specificity to some extent.
In addition, this study showed that the Meyo criteria currently used in clinical practice had a high sensitivity, but its specificity was worrying. However, this finding was based on a small number of studies, and the identification value of Meyo criteria for lymph node metastasis in EC patients requires further verification.
Among diversified machine learning models, some had better prediction performance, such as convolutional neural network, support vector machine, and XGBoost (77, 78), but it seemed that the most popular machine learning model in clinical practice was still logistic regression. This is mainly because the nomogram can be constructed based on logistic regression. Nomogram features a simple application method and good performance in the visualization of results, which is very important for predicting lymph node metastasis in tumors, such as Briganti nomogram for prostate cancer (79). As shown in this study, logistic regression seemed to have a relatively good predictive value. Therefore, follow-up studies can try to develop more general nomograms for predicting lymph node metastasis in EC patients.
Modeling variables are of critical importance for improving the accuracy of machine learning. However, only a few studies summarized the evidence in this regard. The systematic review by Reijnen C et al. (80) showed that CA-125 and thrombocytosis were associated with the risk of lymph node metastasis in EC patients, and the systematic review by Fu et al. (81) reported that tumor diameter was also related to lymph node metastasis. Therefore, the lack of comprehensive independent predictors for lymph node metastasis in EC patients has posed a challenge to the early identification of lymph node metastasis status in EC patients. In this study, we summarized the modeling variables included in machine learning. Since the risk model constructed based on clinical features alone also had good sensitivity (>0.8), risk equations or predictive nomograms for preoperative prediction of lymph node metastasis in EC patients can be constructed based on this study.
The FIGO 2023 staging system (82) classifies lymph node metastases to micrometastasis and macrometastasis, in which IIIC1 was metastasis to the pelvic lymph nodes (IIIC1i: micrometastasis, IIIC1ii: macrometastasis), IIIC2 was metastasis to para-aortic lymph nodes up to the renal vessels, with or without metastasis to the pelvic lymph nodes (IIIC2i: micrometastasis, IIIC2ii: macrometastasis). SLN biopsy is an appropriate alternative to systematic lymphadenectomy, and ultrastaging provides more sensitive and accurate identification of lymphatic disease than standard lymph node dissection. SLN biopsy may also be considered for low/low intermediate-risk patients to rule out occult lymph node metastases and to identify disease that is truly confined to the uterus. Therefore, the ESGO-ESTRO-ESP guidelines allow a SLN approach for all EC patients, which is recognized by FIGO. Although, the value of machine learning for the identification of lymph node metastatic status in EC patients was systematically described in our study, the detection of lymph node metastatic site and extent is also necessary. Future studies could explore the identification of metastatic status for SLN.
The strengths of this study lie in that it was the first systematic review on the preoperative diagnostic value of machine learning for lymph node metastasis in EC patients, and it summarized the existing main modeling variables (clinical features, radiomic features), so as to provide guidance and references for the development of clinical risk tools in the future. However, there are still some limitations in this study. Firstly, most of the included studies focused on logistic regression, with less exploration on other models, making it difficult to summarize their applied value. Secondly, in the included studies, the validation method of the models was mainly internal validation with random sampling, which likely restricted the promotion of the model to other fields. Especially for models based on radiomic features, it poses a serious challenge, since the radiomic features are seriously affected by the experience of radiologists, and the configuration of radiation devices. Thirdly, the included studies were mainly case-control studies, some of which had a small sample size, raising a concern about the stability of the model. Fourthly, The Cancer Genome Atlas (TCGA) classifies EC into four distinct molecular categories: POLE ultramutated (POLEmut), high microsatellite instability (MSI-H) or mismatch repair defective (MSI-H or MMRd), copy number low or no specific molecular profiling (CNL or NSMP), and copy number high or p53 abnormal (CNH or p53abn). However, the original studies included did not strictly differentiate between molecular subtypes (POLEmut, MMRd, NSMP, and p53bn), which resulted in our systematic review failing to provide corresponding evidence. Finally, we only included studies that constructed machine learning for detecting lymph node metastasis and aggregated interpretable clinical features and associations with lymph node metastasis. However, we did not include studies that only analyzed risk factors. Thus, the pooled results may have missed a small number of other clinical features.
The machine learning model is feasible for preoperative prediction of the lymph node metastasis status of EC patients, and the visual nomogram of logistic regression constructed based on clinical features has favorable sensitivity and specificity. In addition, models based on radiomic features combined with clinical features have a better predictive value. Large-sample studies covering different races are warranted to develop predictive nomograms based on clinical features, which can be widely applied in clinical practice. In view of the excellent predictive performance of machine learning models constructed based on radiomic features combined with clinical features, we also look forward to accelerating the development and application of radiomic features and proposing standardized criteria for their application, so as to develop intelligent diagnosis of complex disease status and intelligent prediction of disease prognosis based on radiomic features.
The original contributions presented in the study are included in the article/Supplementary Material. Further inquiries can be directed to the corresponding authors.
ZR: Conceptualization, Data curation, Formal analysis, Methodology, Resources, Writing – original draft, Writing – review & editing. BC: Conceptualization, Investigation, Supervision, Validation, Writing – original draft. CH: Formal analysis, Investigation, Supervision, Validation, Writing – original draft. JYY: Data curation, Formal analysis, Investigation, Resources, Writing – original draft. JD: Software, Supervision, Validation, Visualization, Writing – original draft. YC: Formal analysis, Investigation, Validation, Visualization, Writing – original draft. JLY: Data curation, Formal analysis, Investigation, Validation, Writing – original draft. YL: Formal analysis, Methodology, Software, Visualization, Writing – review & editing.
The author(s) declare that no financial support was received for the research, authorship, and/or publication of this article.
The authors declare that the research was conducted in the absence of any commercial or financial relationships that could be construed as a potential conflict of interest.
All claims expressed in this article are solely those of the authors and do not necessarily represent those of their affiliated organizations, or those of the publisher, the editors and the reviewers. Any product that may be evaluated in this article, or claim that may be made by its manufacturer, is not guaranteed or endorsed by the publisher.
The Supplementary Material for this article can be found online at: https://www.frontiersin.org/articles/10.3389/fonc.2023.1289050/full#supplementary-material
1. Shokunbi MT, Vinters HV, Kaufmann JC. Fusiform intracranial aneurysms. Clinicopathologic features. Surg Neurol (1988) 29(4):263–70. doi: 10.1016/0090-3019(88)90157-7
2. Makker V, MacKay H, Ray-Coquard I, Levine DA, Westin SN, Aoki D, et al. Endometrial cancer. Nat Rev Dis Primers (2021) 7(1):88. doi: 10.1038/s41572-021-00324-8
3. Bray F, Ferlay J, Soerjomataram I, Siegel RL, Torre LA, Jemal A. Erratum: Global cancer statistics 2018: GLOBOCAN estimates of incidence and mortality worldwide for 36 cancers in 185 countries. CA: Cancer J Clin (2020) 70(4):313. doi: 10.3322/caac.21609
4. Ferlay J, Colombet M, Soerjomataram I, Mathers C, Parkin DM, Piñeros M, et al. Estimating the global cancer incidence and mortality in 2018: GLOBOCAN sources and methods. Int J Cancer (2019) 144(8):1941–53. doi: 10.1002/ijc.31937
5. Koh WJ, Abu-Rustum NR, Bean S, Bradley K, Campos SM, Cho KR, et al. Uterine neoplasms, version 1.2018, NCCN clinical practice guidelines in oncology. J Natl Compr Cancer Network JNCCN (2018) 16(2):170–99. doi: 10.6004/jnccn.2018.0006
6. Abu-Rustum N, Yashar C, Arend R, Barber E, Bradley K, Brooks R, et al. Uterine neoplasms, version 1.2023, NCCN clinical practice guidelines in Oncology. J Natl Compr Cancer Network JNCCN (2023) 21(2):181–209. doi: 10.6004/jnccn.2023.0006
7. Mariani A, Webb MJ, Keeney GL, Haddock MG, Calori G, Podratz KC. Low-risk corpus cancer: is lymphadenectomy or radiotherapy necessary? Am J Obstet Gynecol (2000) 182(6):1506–19. doi: 10.1067/mob.2000.107335
8. Cho SJ, Sunwoo L, Baik SH, Bae YJ, Choi BS, Kim JH. Brain metastasis detection using machine learning: a systematic review and meta-analysis. Neuro-Oncology (2021) 23(2):214–25. doi: 10.1093/neuonc/noaa232
9. Wu JH, Liu TYA, Hsu WT, Ho JH, Lee CC. Performance and limitation of machine learning algorithms for diabetic retinopathy screening: meta-analysis. J Med Internet Res (2021) 23(7):e23863. doi: 10.2196/23863
10. Fleuren LM, Klausch TLT, Zwager CL, Schoonmade LJ, Guo T, Roggeveen LF, et al. Machine learning for the prediction of sepsis: a systematic review and meta-analysis of diagnostic test accuracy. Intensive Care Med (2020) 46(3):383–400. doi: 10.1007/s00134-019-05872-y
11. Aimo A, Pelliccia F, Panichella G, Vergaro G, Barison A, Passino C, et al. Indications of beta-adrenoceptor blockers in Takotsubo syndrome and theoretical reasons to prefer agents with vasodilating activity. Int J Cardiol (2021) 333:45–50. doi: 10.1016/j.ijcard.2021.02.074
12. Tang R, Luo R, Tang S, Song H, Chen X. Machine learning in predicting antimicrobial resistance: a systematic review and meta-analysis. Int J antimicrobial Agents (2022) 60(5-6):106684. doi: 10.1016/j.ijantimicag.2022.106684
13. Bellou V, Belbasis L, Konstantinidis AK, Tzoulaki I, Evangelou E. Prognostic models for outcome prediction in patients with chronic obstructive pulmonary disease: systematic review and critical appraisal. BMJ (Clinical Res ed) (2019) 367:l5358. doi: 10.1136/bmj.l5358
14. Hickman SE, Woitek R, Le EPV, Im YR, Mouritsen Luxhøj C, Aviles-Rivero AI, et al. Machine learning for workflow applications in screening mammography: systematic review and meta-analysis. Radiology (2022) 302(1):88–104. doi: 10.1148/radiol.2021210391
15. Liu X, Faes L, Kale AU, Wagner SK, Fu DJ, Bruynseels A, et al. A comparison of deep learning performance against health-care professionals in detecting diseases from medical imaging: a systematic review and meta-analysis. Lancet Digital Health (2019) 1(6):e271–e97. doi: 10.1016/S2589-7500(19)30123-2
16. Akazawa M, Hashimoto K. Artificial intelligence in gynecologic cancers: Current status and future challenges - A systematic review. Artif Intell Med (2021) 120:102164. doi: 10.1016/j.artmed.2021.102164
17. Wolff RF, Moons KGM, Riley RD, Whiting PF, Westwood M, Collins GS, et al. PROBAST: A tool to assess the risk of bias and applicability of prediction model studies. Ann Internal Med (2019) 170(1):51–8. doi: 10.7326/M18-1376
18. Debray TP, Damen JA, Riley RD, Snell K, Reitsma JB, Hooft L, et al. A framework for meta-analysis of prediction model studies with binary and time-to-event outcomes. Stat Methods Med Res (2019) 28(9):2768–86. doi: 10.1177/0962280218785504
19. Huang Y, Jiang P, Kong W, Tu Y, Li N, Wang J, et al. Comprehensive assessment of ERα, PR, ki67, P53 to predict the risk of lymph node metastasis in low-risk endometrial cancer. J Invest Surg (2023) 36(1):2152508. doi: 10.1080/08941939.2022.2152508
20. Feng M, Zhao Y, Chen J, Zhao T, Mei J, Fan Y, et al. A deep learning model for lymph node metastasis prediction based on digital histopathological images of primary endometrial cancer. Quant Imaging Med Surg (2023) 13(3):1899–913. doi: 10.21037/qims-22-220
21. Vinklerová P, Ovesná P, Hausnerová J, Pijnenborg JMA, Lucas PJF, Reijnen C, et al. External validation study of endometrial cancer preoperative risk stratification model (ENDORISK). Front Oncol (2022) 12:939226. doi: 10.3389/fonc.2022.939226
22. Soydal Ç, Varlı B, Araz M, Bakırarar B, Taşkın S, Ortaç UF. Radiomics analysis of uterine tumors in 18F-fluorodeoxyglucose positron emission tomography for prediction of lymph node metastases in endometrial carcinoma. Turkish J Med Sci (2022) 52(3):762–9. doi: 10.55730/1300-0144.5371
23. Otani S, Himoto Y, Nishio M, Fujimoto K, Moribata Y, Yakami M, et al. Radiomic machine learning for pretreatment assessment of prognostic risk factors for endometrial cancer and its effects on radiologists' decisions of deep myometrial invasion. Magn Reson Imaging (2022) 85:161–7. doi: 10.1016/j.mri.2021.10.024
24. Lu W, Chen X, Ni J, Li Z, Su T, Li S, et al. A model to identify candidates for lymph node dissection among patients with high-risk endometrial endometrioid carcinoma according to mayo criteria. Front Oncol (2022) 12:895834. doi: 10.3389/fonc.2022.895834
25. Liu XF, Yan BC, Li Y, Ma FH, Qiang JW. Radiomics nomogram in assisting lymphadenectomy decisions by predicting lymph node metastasis in early-stage endometrial cancer. Front Oncol (2022) 12:894918. doi: 10.3389/fonc.2022.894918
26. Liu XF, Yan BC, Li Y, Ma FH, Qiang JW. Radiomics feature as a preoperative predictive of lymphovascular invasion in early-stage endometrial cancer: A multicenter study. Front Oncol (2022) 12:966529. doi: 10.3389/fonc.2022.966529
27. Liro M, Śniadecki M, Wycinka E, Wojtylak S, Brzeziński M, Jastrzębska J, et al. Incorporation of tumor-free distance and other alternative ultrasound biomarkers into a myometrial invasion-based model better predicts lymph node metastasis in endometrial cancer: evidence and future prospects. Diagnostics (Basel Switzerland) (2022) 12(11):2604. doi: 10.3390/diagnostics12112604
28. Hsiao YY, Fu HC, Wu CH, Lan J, Ou YC, Tsai CC, et al. Quantitative measurement of progesterone receptor immunohistochemical expression to predict lymph node metastasis in endometrial cancer. Diagnostics (Basel Switzerland) (2022) 12(4):790. doi: 10.3390/diagnostics12040790
29. Guo X, Lin C, Zhao J, Tang M. Development of a novel predictive model for lymph node metastasis in patients with endometrial endometrioid carcinoma. BMC Cancer (2022) 22(1):1333. doi: 10.1186/s12885-022-10437-2
30. Guani B, Gaillard T, Teo-Fortin LA, Balaya V, Feki A, Paoletti X, et al. Estimation risk of lymph nodal invasion in patients with early-stage cervical cancer: Cervical cancer application. Front Oncol (2022) 12:935628. doi: 10.3389/fonc.2022.935628
31. Grube M, Reijnen C, Lucas PJF, Kommoss F, Kommoss FKF, Brucker SY, et al. Improved preoperative risk stratification in endometrial carcinoma patients: external validation of the ENDORISK Bayesian network model in a large population-based case series. J Cancer Res Clin Oncol (2022) 149(7):3361–9. doi: 10.21203/rs.3.rs-1690261/v1
32. Bo J, Jia H, Zhang Y, Fu B, Jiang X, Chen Y, et al. Preoperative prediction value of pelvic lymph node metastasis of endometrial cancer: combining of ADC value and radiomics features of the primary lesion and clinical parameters. J Oncol (2022) 2022:3335048. doi: 10.1155/2022/3335048
33. Asami Y, Hiranuma K, Takayanagi D, Matsuda M, Shimada Y, Kato MK, et al. Predictive model for the preoperative assessment and prognostic modeling of lymph node metastasis in endometrial cancer. Sci Rep (2022) 12(1):19004. doi: 10.1038/s41598-022-23252-3
34. Zhang K, Zhang Y, Fang X, Fang M, Shi B, Dong J, et al. Nomograms of combining apparent diffusion coefficient value and radiomics for preoperative risk evaluation in endometrial carcinoma. Front Oncol (2021) 11:705456. doi: 10.3389/fonc.2021.705456
35. Yang LY, Siow TY, Lin YC, Wu RC, Lu HY, Chiang HJ, et al. Computer-aided segmentation and machine learning of integrated clinical and diffusion-weighted imaging parameters for predicting lymph node metastasis in endometrial cancer. Cancers (2021) 13(6):1406. doi: 10.3390/cancers13061406
36. Yan BC, Li Y, Ma FH, Zhang GF, Feng F, Sun MH, et al. Radiologists with MRI-based radiomics aids to predict the pelvic lymph node metastasis in endometrial cancer: a multicenter study. Eur Radiol (2021) 31(1):411–22. doi: 10.1007/s00330-020-07099-8
37. Xu Y, Zhao R. A prediction model of endometrial cancer lesion metastasis under region of interest target detection algorithm. Sci Program (2021). doi: 10.1155/2021/9928842
38. Wang Z, Zhang S, Ma Y, Li W, Tian J, Liu T. A nomogram prediction model for lymph node metastasis in endometrial cancer patients. BMC Cancer (2021) 21(1):748. doi: 10.1186/s12885-021-08466-4
39. Liro M, Śniadecki M, Wycinka E, Wojtylak S, Brzeziński M, Stańczak A, et al. Ultrasound measurement of tumor-free distance from the serosal surface as the alternative to measuring the depth of myometrial invasion in predicting lymph node metastases in endometrial cancer. Diagnostics (Basel Switzerland) (2021) 11(8):1472. doi: 10.3390/diagnostics11081472
40. Li X, Cheng Y, Dong Y, Zhou J, Wang Z, Li X, et al. Development and validation of predictive model for lymph node metastasis in endometrial cancer: a SEER analysis. Ann Trans Med (2021) 9(7):538. doi: 10.21037/atm-20-5034
41. Lei H, Xu S, Mao X, Chen X, Chen Y, Sun X, et al. Systemic immune-inflammatory index as a predictor of lymph node metastasis in endometrial cancer. J Inflammation Res (2021) 14:7131–42. doi: 10.2147/JIR.S345790
42. Jiang P, Yuan R. Analysis of factors related to lymph node metastasis in early-stage type 1 endometrial cancer: verifying the clinical value of positive threshold of the immunohistochemical parameter ki67. Cancer Manage Res (2021) 13:6319–28. doi: 10.2147/CMAR.S316211
43. Jiang P, Huang Y, Tu Y, Li N, Kong W, Di F, et al. Combining clinicopathological parameters and molecular indicators to predict lymph node metastasis in endometrioid type endometrial adenocarcinoma. Front Oncol (2021) 11:682925. doi: 10.3389/fonc.2021.682925
44. Zhang Y, Zhao W, Chen Z, Zhao X, Ren P, Zhu M. Establishment and evaluation of a risk-scoring system for lymph node metastasis in early-stage endometrial carcinoma: Achieving preoperative risk stratification. J Obstet Gynaecol Res (2020) 46(11):2305–13. doi: 10.1111/jog.14422
45. Reijnen C, Gogou E, Visser NCM, Engerud H, Ramjith J, van der Putten LJM, et al. Preoperative risk stratification in endometrial cancer (ENDORISK) by a Bayesian network model: A development and validation study. PloS Med (2020) 17(5):e1003111. doi: 10.1371/journal.pmed.1003111
46. Eriksson LSE, Epstein E, Testa AC, Fischerova D, Valentin L, Sladkevicius P, et al. Ultrasound-based risk model for preoperative prediction of lymph-node metastases in women with endometrial cancer: model-development study. Ultrasound Obstet Gynecol (2020) 56(3):443–52. doi: 10.1002/uog.21950
47. Crivellaro C, Landoni C, Elisei F, Buda A, Bonacina M, Grassi T, et al. Combining positron emission tomography/computed tomography, radiomics, and sentinel lymph node mapping for nodal staging of endometrial cancer patients. Int J Gynecol Cancer (2020) 30(3):378–82. doi: 10.1136/ijgc-2019-000945
48. Chen J, He B, Dong D, Liu P, Duan H, Li W, et al. Noninvasive CT radiomic model for preoperative prediction of lymph node metastasis in early cervical carcinoma. Br J Radiol (2020) 93(1108):20190558. doi: 10.1259/bjr.20190558
49. Berg HF, Ju Z, Myrvold M, Fasmer KE, Halle MK, Hoivik EA, et al. Development of prediction models for lymph node metastasis in endometrioid endometrial carcinoma. Br J Cancer (2020) 122(7):1014–22. doi: 10.1038/s41416-020-0745-6
50. Xu X, Li H, Wang S, Fang M, Zhong L, Fan W, et al. Multiplanar MRI-based predictive model for preoperative assessment of lymph node metastasis in endometrial cancer. Front Oncol (2019) 9:1007. doi: 10.3389/fonc.2019.01007
51. Meydanli MM, Aslan K, Oz M, Muftuoglu KH, Yalcin I, Engin-Ustun Y. A novel multivariable prediction model for lymphatic dissemination in endometrioid endometrial cancer: The lymph node Metastasis Risk Index. Eur J Obstet Gynecol Reprod Biol (2019) 240:310–5. doi: 10.1016/j.ejogrb.2019.07.015
52. Kan Y, Dong D, Zhang Y, Jiang W, Zhao N, Han L, et al. Radiomic signature as a predictive factor for lymph node metastasis in early-stage cervical cancer. J Magn Reson Imaging JMRI (2019) 49(1):304–10. doi: 10.1002/jmri.26209
53. Günakan E, Atan S, Haberal AN, Küçükyıldız İA, Gökçe E, Ayhan A. A novel prediction method for lymph node involvement in endometrial cancer: machine learning. Int J Gynecol Cancer (2019) 29(2):320–4. doi: 10.1136/ijgc-2018-000033
54. Dong Y, Cheng Y, Tian W, Zhang H, Wang Z, Li X, et al. An externally validated nomogram for predicting lymph node metastasis of presumed stage I and II endometrial cancer. Front Oncol (2019) 9:1218. doi: 10.3389/fonc.2019.01218
55. De Bernardi E, Buda A, Guerra L, Vicini D, Elisei F, Landoni C, et al. Radiomics of the primary tumour as a tool to improve (18)F-FDG-PET sensitivity in detecting nodal metastases in endometrial cancer. EJNMMI Res (2018) 8(1):86. doi: 10.1186/s13550-018-0441-1
56. Teixeira AM, Ribeiro R, Schmeler KM, Herzog TJ, Nicolau SM, Marques RM. A preoperative and intraoperative scoring system to predict nodal metastasis in endometrial cancer. Int J Gynaecol Obstet (2017) 137(1):78–85. doi: 10.1002/ijgo.12103
57. Taşkın S, Şükür YE, Varlı B, Koyuncu K, Seval MM, Ateş C, et al. Nomogram with potential clinical use to predict lymph node metastasis in endometrial cancer patients diagnosed incidentally by postoperative pathological assessment. Arch Gynecol Obstet (2017) 296(4):803–9. doi: 10.1007/s00404-017-4477-7
58. Yang B, Shan B, Xue X, Wang H, Shan W, Ning C, et al. Predicting lymph node metastasis in endometrial cancer using serum CA125 combined with immunohistochemical markers PR and ki67, and a comparison with other prediction models. PloS One (2016) 11(5):e0155145. doi: 10.1371/journal.pone.0155145
59. Pollom EL, Conklin CM, von Eyben R, Folkins AK, Kidd EA. Nomogram to predict risk of lymph node metastases in patients with endometrioid endometrial cancer. Int J Gynecol Pathol (2016) 35(5):395–401. doi: 10.1097/PGP.0000000000000246
60. Koskas M, Fournier M, Vanderstraeten A, Walker F, Timmerman D, Vergote I, et al. Evaluation of models to predict lymph node metastasis in endometrial cancer: A multicentre study. Eur J Cancer (Oxford Engl 1990) (2016) 61:52–60. doi: 10.1016/j.ejca.2016.03.079
61. Koskas M, Luton D, Graesslin O, Barranger E, Clavel-Chapelon F, Haddad B, et al. Direct comparison of logistic regression and recursive partitioning to predict lymph node metastasis in endometrial cancer. Int J Gynecol Cancer (2015) 25(6):1037–43. doi: 10.1097/IGC.0000000000000451
62. Bendifallah S, Canlorbe G, Raimond E, Hudry D, Coutant C, Graesslin O, et al. External validation of nomograms designed to predict lymphatic dissemination in patients with early-stage endometrioid endometrial cancer: a multicenter study. Am J Obstet Gynecol (2015) 212(1):56.e1–7. doi: 10.1016/j.ajog.2014.06.058
63. Bendifallah S, Canlorbe G, Laas E, Huguet F, Coutant C, Hudry D, et al. A predictive model using histopathologic characteristics of early-stage type 1 endometrial cancer to identify patients at high risk for lymph node metastasis. Ann Surg Oncol (2015) 22(13):4224–32. doi: 10.1245/s10434-015-4548-6
64. Koskas M, Genin AS, Graesslin O, Barranger E, Haddad B, Darai E, et al. Evaluation of a method of predicting lymph node metastasis in endometrial cancer based on five pre-operative characteristics. Eur J Obstet Gynecol Reprod Biol (2014) 172:115–9. doi: 10.1016/j.ejogrb.2013.10.028
65. Kang S, Lee JM, Lee JK, Kim JW, Cho CH, Kim SM, et al. A Web-based nomogram predicting para-aortic nodal metastasis in incompletely staged patients with endometrial cancer: a Korean Multicenter Study. Int J Gynecol Cancer (2014) 24(3):513–9. doi: 10.1097/IGC.0000000000000090
66. Luomaranta A, Leminen A, Loukovaara M. Prediction of lymph node and distant metastasis in patients with endometrial carcinoma: a new model based on demographics, biochemical factors, and tumor histology. Gynecol Oncol (2013) 129(1):28–32. doi: 10.1016/j.ygyno.2013.01.008
67. Koskas M, Chereau E, Ballester M, Dubernard G, Lécuru F, Heitz D, et al. Accuracy of a nomogram for prediction of lymph-node metastasis detected with conventional histopathology and ultrastaging in endometrial cancer. Br J Cancer (2013) 108(6):1267–72. doi: 10.1038/bjc.2013.95
68. Benoit MF, Ward KK. Uterine cancer normogram to predict lymph node metastasis: comparison to the mayo algorithm and an external validation of a model in a north american population. Eur J Gynaecol Oncol (2020) 41(5):681–4. doi: 10.31083/j.ejgo.2020.05.5401
69. Wisner KPA, Ahmad S, Holloway RW. Indications and techniques for robotic pelvic and para-aortic lymphadenectomy with sentinel lymph node mapping in gynecologic oncology. Best Pract Res Clin Obstet Gynaecol (2017) 45:83–93. doi: 10.1016/j.bpobgyn.2017.04.006
70. Lindqvist E, Wedin M, Fredrikson M, Kjølhede P. Lymphedema after treatment for endometrial cancer - A review of prevalence and risk factors. Eur J Obstet Gynecol Reprod Biol (2017) 211:112–21. doi: 10.1016/j.ejogrb.2017.02.021
71. Helgers RJA, Winkens B, Slangen BFM, Werner HMJ. Lymphedema and Post-Operative Complications after Sentinel Lymph Node Biopsy versus Lymphadenectomy in Endometrial Carcinomas-A Systematic Review and Meta-Analysis. J Clin Med (2020) 10(1):120. doi: 10.3390/jcm10010120
72. Bollineni VR, Ytre-Hauge S, Bollineni-Balabay O, Salvesen HB, Haldorsen IS. High diagnostic value of 18F-FDG PET/CT in endometrial cancer: systematic review and meta-analysis of the literature. J Nucl Med (2016) 57(6):879–85. doi: 10.2967/jnumed.115.170597
73. Hu J, Zhang K, Yan Y, Zang Y, Wang Y, Xue F. Diagnostic accuracy of preoperative (18)F-FDG PET or PET/CT in detecting pelvic and para-aortic lymph node metastasis in patients with endometrial cancer: a systematic review and meta-analysis. Arch Gynecol Obstet (2019) 300(3):519–29. doi: 10.1007/s00404-019-05207-8
74. Bi Q, Chen Y, Wu K, Wang J, Zhao Y, Wang B, et al. The diagnostic value of MRI for preoperative staging in patients with endometrial cancer: A meta-analysis. Acad Radiol (2020) 27(7):960–8. doi: 10.1016/j.acra.2019.09.018
75. Luomaranta A, Leminen A, Loukovaara M. Magnetic resonance imaging in the assessment of high-risk features of endometrial carcinoma: a meta-analysis. Int J Gynecol Cancer (2015) 25(5):837–42. doi: 10.1097/IGC.0000000000000194
76. Burg LC, Hengeveld EM, In 't Hout J, Bulten J, Bult P, Zusterzeel PLM. Ultrastaging methods of sentinel lymph nodes in endometrial cancer - a systematic review. Int J Gynecol Cancer (2021) 31(5):744–53. doi: 10.1136/ijgc-2020-001964
77. Mukherjee J, Sharma R, Dutta P, Bhunia B. Artificial intelligence in healthcare: a mastery. Biotechnol Genet Eng Rev (2023), 1–50. doi: 10.1080/02648725.2023.2196476
78. Zhang A, Wu Z, Wu E, Wu M, Snyder MP, Zou J, et al. Leveraging physiology and artificial intelligence to deliver advancements in healthcare. Physiol Rev (2023) 103(4):2423–50. doi: 10.1152/physrev.00033.2022
79. Gandaglia G, Martini A, Ploussard G, Fossati N, Stabile A, De Visschere P, et al. External validation of the 2019 briganti nomogram for the identification of prostate cancer patients who should be considered for an extended pelvic lymph node dissection. Eur Urol (2020) 78(2):138–42. doi: 10.1016/j.eururo.2020.03.023
80. Reijnen C, IntHout J, Massuger L, Strobbe F, Küsters-Vandevelde HVN, Haldorsen IS, et al. Diagnostic accuracy of clinical biomarkers for preoperative prediction of lymph node metastasis in endometrial carcinoma: A systematic review and meta-analysis. Oncologist (2019) 24(9):e880–e90. doi: 10.1634/theoncologist.2019-0117
81. Fu R, Zhang D, Yu X, Zhang H. The association of tumor diameter with lymph node metastasis and recurrence in patients with endometrial cancer: a systematic review and meta-analysis. Trans Cancer Res (2022) 11(11):4159–77. doi: 10.21037/tcr-22-2595
Keywords: endometrial cancer, lymph node metastasis, radiomics, machine learning, systematic review
Citation: Ren Z, Chen B, Hong C, Yuan J, Deng J, Chen Y, Ye J and Li Y (2023) The value of machine learning in preoperative identification of lymph node metastasis status in endometrial cancer: a systematic review and meta-analysis. Front. Oncol. 13:1289050. doi: 10.3389/fonc.2023.1289050
Received: 05 September 2023; Accepted: 06 December 2023;
Published: 20 December 2023.
Edited by:
Alberto Farolfi, Scientific Institute of Romagna for the Study and Treatment of Tumors (IRCCS), ItalyReviewed by:
Filippo Merloni, Instituto Tumori della Romagna IRST IRCCS, ItalyCopyright © 2023 Ren, Chen, Hong, Yuan, Deng, Chen, Ye and Li. This is an open-access article distributed under the terms of the Creative Commons Attribution License (CC BY). The use, distribution or reproduction in other forums is permitted, provided the original author(s) and the copyright owner(s) are credited and that the original publication in this journal is cited, in accordance with accepted academic practice. No use, distribution or reproduction is permitted which does not comply with these terms.
*Correspondence: Yanqin Li, OTA0MTQ3Mzc1QHFxLmNvbQ==
Disclaimer: All claims expressed in this article are solely those of the authors and do not necessarily represent those of their affiliated organizations, or those of the publisher, the editors and the reviewers. Any product that may be evaluated in this article or claim that may be made by its manufacturer is not guaranteed or endorsed by the publisher.
Research integrity at Frontiers
Learn more about the work of our research integrity team to safeguard the quality of each article we publish.