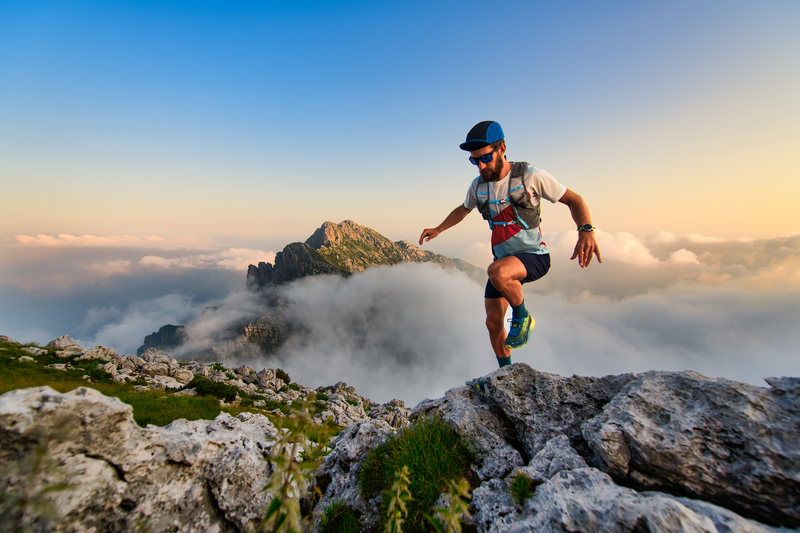
95% of researchers rate our articles as excellent or good
Learn more about the work of our research integrity team to safeguard the quality of each article we publish.
Find out more
REVIEW article
Front. Oncol. , 28 November 2023
Sec. Cancer Metabolism
Volume 13 - 2023 | https://doi.org/10.3389/fonc.2023.1285209
This article is part of the Research Topic Methods and Applications of Tumour Metabolic Imaging in the Preclinical and Clinical Setting View all 5 articles
Cancer metabolism has emerged as a pivotal area of research recently. The ability to visualize and comprehend the metabolic processes of cancer holds immense clinical value, particularly in the diagnosis of malignant tumors and the assessment of treatment responses. Deuterium Metabolic Imaging (DMI), as a robust, simple, and versatile MR spectroscopic imaging tool, demonstrates promise in tumor diagnosis and treatment efficacy assessment. This review explored the latest developments and applications of DMI in oncology across various tumor metabolic axes, with a specific emphasis on its potential for clinical translation. DMI offers invaluable insights into tumor biology, treatment responses, and prognostic outcomes. Notably, DMI can identify early responses to immunotherapy, a prominent area of current research interest. In conclusion, DMI harbors the potential to evolve into a convenient and efficient imaging technique in clinical practice, thereby advancing precision medicine and improving the diagnosis and evaluation of cancer treatments.
Metabolism has long been a topic of interest in cancer research, due to the distinct metabolic traits that tumor cells exhibit compared to their normal counterpart. These metabolic adaptations, encompassing various intracellular and extracellular alterations, play a pivotal role not only in the growth and proliferation of malignant cells but also in shaping gene expression, cellular differentiation, and the tumor microenvironment (1–4). Among these alterations, glucose metabolic reprogramming holds particular significance in tumor cells, leading to increased glucose uptake and consumption (5). This metabolic reprogramming opens up new avenues for malignancy treatment, including the use of glycolytic inhibitors such as 2-deoxy-D-glucose and 3-bromopyruvate (6–8). Consequently, understanding and visualizing aberrant tumor metabolism offers immense potential for non-invasive malignancy diagnosis and treatment assessment.
The advancement of precision medicine in modern oncology has driven the development of biomedical imaging techniques and functional metabolic therapies, enabling comprehensive investigations of tumor biology at the cellular and molecular levels (9–11). Recently, there has been an increasing emphasis on imaging tumor tissue metabolism, leading to dedicated studies in this field (4, 12). Among the various metabolic imaging techniques, such as Positron Emission Computed Tomography (PET), dynamic hyperpolarized 13C Magnetic Resonance Spectroscopy Imaging (MRSI), and Deuterium Metabolic Imaging (DMI), DMI stands out as a versatile and robust approach that provides more flexible dosing and scanning time by using 2H-enriched metabolites with high homology to endogenous molecules (13). Its simplicity, including the use of MR (magnetic resonance) methods, obviates the need for water and lipid signal suppression, making it inherently robust for metabolic imaging (14). Furthermore, the low gyromagnetic ratio of the 2H isotope coupled with the sparse MR spectrum of 2H-containing metabolic molecules renders DMI minimally affected by magnetic field inhomogeneity (14). Additionally, favorable T1 and T2 relaxation times of DMI further facilitate high sensitivity through fast scanning with excellent spectral resolution (15). The availability of a wide range of non-radioactive and biocompatible 2H-enriched substrates targeting various metabolic pathways has further propelled the development of DMI, making it a promising tool in oncology. Hence, this review focuses on recent advancements and applications of DMI in oncology, highlighting its potential for clinical translation, and discussing its future development trends.
Currently, clinical diagnosis and treatment monitoring of cancer heavily rely on PET imaging, primarily using 2-18F-fluoro-2-deoxy-D-glucose (18FDG) as the imaging agent. PET exploits the increased glycolysis in tumor tissue, allowing for the detection of tumor uptake and phosphorylation of FDG (16–19). It plays a crucial role in tumor diagnosis, staging, and assessing treatment response, while also aiding in identifying and monitoring immune-related toxicities (17, 19). However, PET has several limitations: First, PET provides high-resolution maps of glucose uptake but is limited in detecting downstream metabolites; additionally, it may encounter challenges in identifying tumors in organs with high baseline glucose uptake, such as the brain, resulting in decreased contrast in brain tumor imaging (20, 21). Second, 18FDG is a radioactive tracer that limits longitudinal measurements and may prohibit examination in certain patient populations due to safety concerns.
Hyperpolarized 13C MRSI is an emerging magnetic resonance spectroscopy imaging technique that significantly enhances the magnetic resonance signal by utilizing dynamic nuclear polarization (DNP) (22). This technology holds promise for early-stage cancer diagnosis and treatment response monitoring, particularly in prostate cancer, breast cancer, renal clear cell tumors, and central nervous system tumors (23–27). The primary advantage of hyperpolarized 13C MRSI is its ability to detect downstream metabolites of glucose. However, its clinical implementation faces challenges due to additional hardware requirements, lengthy operating time and associated high costs (28).
DMI employs magnetic resonance to detect 2H-labeled molecular probes, and related metabolites as illustrated in Figure 1. By analyzing the downstream metabolites of these probes, DMI can visualize various metabolic pathways and provide molecular insights into tumor tissue metabolism without radiation exposure (30). Incorporating spatial localization coding allows for the analysis and comparison of the concentration of related 2H-labeled metabolites in different tissues (30). Due to the lower chemical shift range, J-coupling constants, and coupling effects of deuterium compared to hydrogen, the complexity of deuterium spectrum is simplified, which facilitates the interpretation and analysis of DMI and enables researchers to focus on specific deuterium labeled metabolites and their metabolic kinetics (14, 29). DMI enables dynamic tracking of the entire process of cell metabolism within tumor cells, including glucose transport, pentose 6-phosphate pathway conversion (PPP), glycolysis, and the Krebs cycle (31). These capabilities hold significant potential for guiding precision treatments (29).
Figure 1 Workflow of a Deuterium Metabolic Imaging (DMI) study: Following the administration of deuterium-labeled glucose, via tail vein injection in mice and oral ingestion in humans, deuterium metabolism magnetic resonance imaging (DMSI) is employed for signal detection. The obtained data is subsequently subjected to comprehensive processing and analysis as shown in (A–F) to facilitate interpretation. Deuterium NMR spectra acquired without localization from (A) rat brain in vivo at 11.7 T before infusion of any 2H-labeled substrate, (B) rat brain after infusion of 2H-glucose in vivo, (C) rat brain after infusion of 2H-glucose postmortem, and (D) rat brain after infusion of 2H-acetate in vivo. (E) 2H NMR spectrum acquired from human brain at 4 T, 60 min after oral administration of 2H-glucose. (F) 2H NMR spectra from rat (top, black) and human (bottom, gray) liver after intravenous and oral administration of 2H-labeled glucose, respectively. (A-F) are cited from De Feyter et al. (29) (non-commercial 4.0 international license, CC BY-NC 4.0).
The critical advantage of DMI lies in its ability to utilize exogenous 2H labeling to capture multiple metabolic steps involved in glucose metabolism, as depicted in Figure 2. Furthermore, DMI facilitates the quantitative determination of biochemical kinetic constants, enhancing its utility in metabolic research. In contrast to 1H-MRSI, DMI does not necessitate the use of additional radio frequency (RF) pulses for the suppression of protonated water, due to the low abundance of natural deuterated water, leading to a low specific absorption rate (SAR) of RF power(14, 29). This will mitigate the rise in temperature during the patient examination process, thereby simplifying and enhancing the safety of DMI’s clinical application (35). Unlike PET, which faces challenges in brain tumor imaging (36), DMI has emerged as a valuable alternative (37). It overcomes the limitations posed by high glucose uptake in both normal brain tissue and malignant tumors, enabling precise imaging of malignancies (38). Although the combination of PET and CT provides a higher spatial resolution advantage, DMI offers a simpler and more convenient imaging method without any radiation exposure, making it safer for longitudinal studies (39). As a complementary technique to hyperpolarized 13C MRSI, DMI allows for the long-term monitoring of metabolic processes without requiring significant modifications to existing clinical magnetic resonance instruments. Additionally, the naturally low abundance of 2H (40) eliminates the need to suppress 2H signals from water and fat during MRSI (30).
Figure 2 Metabolic processes within tumor cells: Glucose ingress into tumor cells via the glucose transporter (GLUT) initiates two vital metabolic pathways, glycolysis and the tricarboxylic acid (TCA) cycle. Within tumor cells, glycolysis predominates as the primary energy source, resulting in the substantial production of lactic acid, which is extruded by the Monocarboxylic Acid Transporter (MCT). Deuterium Metabolic Imaging (DMI) is adept at monitoring the conversion of 2H-pyruvate to 2H-lactate (highlighted by the red arrow) during glycolysis, thereby offering insights into the metabolic flux of glycolysis in tumors (32). Similarly, DMI facilitates the evaluation of tumor cell death by tracking the metabolism of 2H-fumaric acid to 2H-malic acid (indicated by the blue arrow) during the TCA cycling (33, 34).
In this review, we highlight the potential of DMI in studying tumor metabolism, with a specific focus on glucose metabolism pathways. To provide a comprehensive perspective, Table 1 provides an overview of various studies that have explored the application of DMI in the realms of metabolic imaging and treatment evaluation.
Metabolic reprogramming is a hallmark of almost every tumor cell, leading to a preference for utilizing glucose for glycolysis, even in the presence of oxygen (47, 48). The advent of DMI technology now enables the visualization and quantitative analysis of this metabolic reprogramming in tumors, as shown in Figure 2.
Several studies have demonstrated the potential of DMI in investigating the glucose-lactate metabolism pathway in tumors. De Feyter et al. successfully identified metabolic differences between normal brain tissue and tumor tissue using DMI in a rat glioma model, showing a higher level of the lactate/glutamine ratio in tumors (29). They also observed similar metabolic patterns and image contrast in two patients with a high-grade brain tumor after oral intake of 2H-labeled glucose. L. Tan et al. utilized DMI to observe metabolic changes in acute myeloid leukemia cells following cisplatin treatment, revealing higher lactate levels in AML cells compared to normal cells (41). The both studies jointly highlight the potential of DMI in tumor diagnosis from both in vivo and in vitro perspectives. Kreis et al. implemented a dynamic DMI approach to quantify glycolytic metabolic flux in a lymphoma mice model, revealing heterogeneity within tumors (32). Markovic et al. analyzed glucose metabolism in an orthotopic pancreatic cancer mouse model, unveiling differences in lactate clearance kinetics between two distinct pancreatic cancer subtypess (39). Simões et al. developed a dynamic glucoseenhanced deuterium spectroscopy (DGE2H-MRS) to evaluate the glucose metabolic turnover rate in mice with two different pathological types (GL261 and CT2A cells) of glioma by differential glycolysis and mitochondrial oxidation(43). n addition to providing insights into glycolysis metabolism, DMI also offers crucial information regarding another key aspect of tumor metabolism: choline uptake. Veltien et al. utilized DMI to monitor and image 2H-choline and 2H-glucose signals in subcutaneous kidney tumors in mice (42).
Tumor cells majorly obtain energy from anaerobic glycolysis resulting in lactate accumulation within the tumor tissue (12, 49). Elevated lactate levels in tumors are associated with cancer metastasis, progression, recurrence, and poor prognosis (49). Tumor cells not only use lactate as an energy source, but also shuttle it to adjacent cancer cells, stroma, and vascular endothelial cells, inducing metabolic reprogramming (50). Notably, glycolytic enzymes such as hexokinase2 (HK2)2 and pyruvate kinase (PK)M2 have been extensively studied for their correlation with cancer cell motility and invasive capacity (51–55). The integration of macroscopic imaging of tumor metabolism using DMI, in combination with Western blot analysis and other techniques, holds the potential to explore metabolic markers related to tumor metastasis and provide valuable assistance in tumor staging and prognosis.
In the tricarboxylic acid cycle of aerobic metabolism, fumarate is converted to malate catalyzed by the enzyme fumarase (or fumarate hydratase, EC 4.2.1.2) (56). Monitoring the malate signal generated from fumarate metabolism can provide insights into the early response of tumors to treatment and other pathological conditions associated with cell death, such as toxic injury or ischemia (57–61). While hyperpolarized 13C MRSI has demonstrated an increase in malate signal in treated tumor tissues due to tumor cell necrosis, researchers are exploring the potential of DMI as a cost-effective and more straightforward imaging tool for monitoring the fumarate-malate metabolism process in tumors (Figure 2) (56).
Hesse et al. (33) utilized DMI in a 7-Tesla MR scanner to monitor the metabolized 2H-malate signal in lymphoma cells and tumor-bearing mice before and after treatment with etoposide. Their findings suggest that DMI could serve as a noninvasive imaging tool to monitor early tumor response to therapy. Building on this research, they used DMI to track the malate signal in tumor tissue before and after treating tumor-bearing mice with etoposide and compared the effects of oral administration and intravenous administration of deuterated fumaric acid (34). This study discovered that 2H-fumarate, a cell death marker, could be detected with similar sensitivity through different administration methods. These findings provide a promising prospect for the clinical use of DMI in monitoring the early response of tumor patients to oral anti-tumor drugs.
These studies underscore the potential of DMI as a valuable tool for detecting tumor cell death by monitoring the conversion of fumarate to malate. DMI offers advantages over 13C hyperpolarized MRSI in terms of cost-effectiveness and ease of implementation. Consequently, DMI shows promise as a non-invasive imaging technique for monitoring cell death and early response to tumor treatment.
In addition to the Warburg effect, continuous cell division is a hallmark of advanced malignancy, often linked with the reactivation of telomere reverse transcriptase (TERT) (62). A recent study by Batsios et al. (44) has demonstrated that DMI can evaluate TERT expression in vivo, revealing a TERT-FOXO1 axis in cancer metabolic reprogramming. This connection sheds light on the role of TERT in the glycolytic pathway, particularly in the conversion of pyruvate to lactate (Figure 3B). The noninvasive detection of metabolic changes related to TERT expression through DMI holds significant promise. Further studies should aim to validate these findings in clinical settings and explore the clinical utility of DMI, providing new insights into the intricate relationship between TERT expression and cancer metabolism.
Figure 3 Schematic summary of the study of Batsios et al. Batsios et al. (44) and Taglang et al. Taglang et al. (45) (A) The alternative lengthening telomeres (ALT) pathway elevates glycolytic flux via enzyme phosphofructokinase-1(PFK1). This metabolic reprogramming and response to PARPi (polymerase inhibitors) that cause telomeric fusion in ALT cells can be non-invasively monitored via quantification of lactate production from 2H-glucose. (B) Schematic representation elucidating the intricate relationship among telomerase reverse transcriptase (TERT), FOXO1 (a member of the Fox family of transcription factors), nicotinamide phosphoribosyl transferase (NAMPT), glyceraldehyde-3-phosphate dehydrogenase (GAPDH), nicotinamide (NAM), nicotinamide mononucleotide (NMN), nicotinamide adenine dinucleotide (NADH), and pyruvate flux to lactate in TERT-positive (TERT+) cancer cells.
Diagnosing and evaluating the treatment response of astrocytoma can be challenging, especially in distinguishing tumor progression from pseudoprogression (63). Taglang et al. (45) have demonstrated that DMI could serve as a novel imaging technique for studying the alternative lengthening telomeres (ALT) pathway in astrocytoma. This telomerase-independent telomere maintenance mechanism involves metabolic reprogramming that increases glycolytic flux in astrocytoma (Figure 3A). The study utilized DMI to visualize glycolysis flux in astrocytoma mouse models, confirming the relationship between the ALT pathway and glycolytic flux through genetic and pharmacological approaches. These findings highlight the value of DMI in visualizing ALT-related metabolic reprogramming in astrocytoma and its potential for diagnosing and evaluating treatment response. Additionally, DMI exhibits the ability to detect early changes in tumor lactate signal, making it effective in identifying pseudo-progression.
These studies provided valuable insights into the telomere-related aspects of tumors and proposed a promising approach for metabolic imaging in cancer patients applicable in clinical practice. A biological link between tumor telomeres and glycolytic metabolism is established through the TERT or ALT pathway. Gene set enrichment analysis has further revealed enhanced glycolysis in adrenocortical carcinoma with high TERT levels (64). Overall, telomere maintenance is a hallmark of cancer and is often achieved through the reactivation of TERT or ALT pathways, which are associated with the glycolysis (45, 64–66). DMI offers a non-invasive and clinically translatable biomarker for evaluating the telomere-related expression in tumors, providing indirect but valuable imaging insights into the TERT and ALT pathways.
Deuterated 3-O-methylglucose (OMG) has emerged as a promising tracer in DMI, offering an alternative to PET for quantifying glucose uptake (46). Unlike 18FDG used in PET, OMG is a non-metabolizable glucose analog that is transported similarly to D-glucose. Hartmann et al. (46) conducted a study to explore the potential of OMG-DMI as a non-ionizing radiation alternative for tumor imaging. Their results demonstrated the feasibility of using OMG-DMI as a viable method for specific detection and quantification of glucose uptake in tumors.
Recent advancements in tumor immunotherapy have yielded significant clinical breakthroughs for numerous tumor patients (67–69). Infiltrating immune cells (e.g., T cells, B cells, neutrophils, etc.) undergo metabolic changes that can impact tumor progression and antitumor function. These metabolic alterations often involve increased glycolysis and lactate accumulation (70). However, the tumor microenvironment poses challenges to effective anti-tumor immunity due to hypoxia, acidity, and nutrient depletion (71). Studies have highlighted that highly expressed glycolytic genes can hinder the effectiveness of immunotherapy, while targeted glycolytic drugs (e.g., dimethyl fumarate, 2-deoxyglucose, diclofenac, etc.) have been found to enhance immunotherapy efficacy by promoting IFN-γ production in immune cells (72–74). Strategies aimed at inhibiting tumor cell glycolysis are actively under investigation to improve immunosurveillance and control tumor growth. Real-time visualization of tumor glycolysis can play a crucial role in treatment planning and monitoring the response to immunotherapy, thus enhancing its safety and effectiveness. DMI has been demonstrated as a simple and efficient imaging tool capable of real-time monitoring 2H-glucose and 2H-lactate signals to visualize the tumor glycolytic metabolism De Feyter et al. (29); Tan et al. (41). Consequently, DMI is expected to emerge as a non-invasive and promising imaging tool in tumor immunotherapy and targeted therapy.
This review provides an overview of DMI’s applications in various tumor metabolic processes. The primary focus of most studies has been on the metabolic reprogramming of tumors, especially the Warburg effect. These investigations have successfully harnessed DMI for non-invasive imaging of tumor glycolysis metabolism. DMI has also shown potential in detecting the malate signal produced by fumarate metabolism within tumor tissue, enabling the non-invasive evaluation of early tumor response to treatment and cell death. Furthermore, the reactivation of the TERT andor ALT pathways, critical for maintaining tumor telomeres, has been biologically linked to glycolysis. Researchers have utilized DMI for TERT and ALT pathway imaging, allowing non-invasive evaluation of telomere-related expression in tumors. As a result, DMI holds the potential to become a convenient and efficient imaging tool in clinical practice, providing non-invasive evaluation of tumor metabolism.
Recent studies have demonstrated that T cell effector functions and augments immunotherapy were correlated with restricted glycolysis (72–74). This makes DMI a valuable tool for evaluating treatment response after immunotherapy, as it can image and monitor tumor glycolytic metabolism. DMI can aid in pinpointing optimal time windows for immunotherapy and improving the precision of clinical interventions. Additionally, lactate accumulation in tumors is associated with cancer metastasis and prognosis (49, 75). DMI enables non-invasive visualization of the entire metabolic process within tumors, providing valuable insights into tumor prognosis and staging. Recent research has also unveiled a mechanistic connection between tumor glycolysis metabolism and telomere elongation, which can be evaluated using DMI (44, 45). As a macroscopic imaging tool, DMI complements and validates microscopic perspectives, such as proteins, DNA, RNA, etc., offering valuable information on metabolic changes.
Furthermore, DMI can provide complementary perspectives and evidence when used in conjunction with other techniques, such as 13C MRSI (57–61). Labeled malate production in 13C MRSI has demonstrated the in vivo imaging of cell death (57–61). As our understanding of tumor and immune cell metabolism advances in cancer progression and immunotherapy, DMI exhibits great potential in cancer diagnosis and treatment evaluation (76), DMI exhibited the great application prospects in cancer diagnosis and treatment evaluation. While DMI is rapidly advancing in cancer research, there are still several challenges to be addressed for its clinical translation. These challenges encompass the development of reliable methods for quantifying deuterium substance injection, ensuring the safety of DMI probes, and advancing the hardware development of clinical systems. A standardized and comprehensive set of indicators and protocols for DMI can be adopted from relevant diagnostic and evaluation approaches of PET. This standardization would be crucial for advancing further clinical research in cancer using DMI.
In conclusion, DMI emerges as a powerful and promising tool for investigating tumor metabolism. Its unique capabilities allow non-invasive imaging of various metabolic pathways within tumors, encompassing glycolysis, fumarate metabolism, and telomere-related pathways. DMI can provide invaluable insights into tumor biology, treatment response, and prognosis assessment. Moreover, its potential application in immunotherapy holds the promise of evaluating early treatment responses and optimizing therapeutic strategies. However, it is imperative to recognize that further research is essential to address challenges related to quantification, safety, and hardware development. With ongoing advancements, DMI stands poised to evolve into a convenient and efficient imaging tool in clinical practice. Its contributions will play a pivotal role in the advancement of precision medicine and the improvement of patient outcomes across the spectrum of cancer diagnosis and treatment evaluation.
JW: Conceptualization, Investigation, Writing – original draft, Writing – review & editing, Methodology. YG: Conceptualization, Writing – review & editing. HC: Conceptualization, Writing – review & editing. PS: Conceptualization, Methodology, Supervision, Visualization, Writing – review & editing. XZ: Conceptualization, Formal Analysis, Supervision, Visualization, Writing – review & editing. TY: Project administration, Resources, Supervision, Writing – review & editing. LL: Investigation, Visualization, Writing – review & editing. FP: Visualization, Writing – review & editing. LY: Visualization, Writing – review & editing.
The author(s) declare that no financial support was received for the research, authorship, and/or publication of this article.
Authors PS and XZ were employed by the company Philips Healthcare.
The remaining authors declare that the research was conducted in the absence of any commercial or financial relationships that could be construed as a potential conflict of interest.
All claims expressed in this article are solely those of the authors and do not necessarily represent those of their affiliated organizations, or those of the publisher, the editors and the reviewers. Any product that may be evaluated in this article, or claim that may be made by its manufacturer, is not guaranteed or endorsed by the publisher.
1. Teicher BA, Linehan WM, Helman LJ. Targeting cancer metabolism. Clin Cancer Res (2012) 18:5537–45. doi: 10.1158/1078-0432.CCR-12-2587
2. DeBerardinis RJ, Chandel NS. Fundamentals of cancer metabolism. Sci Adv (2016) 2:e1600200. doi: 10.1126/sciadv.1600200
3. Pavlova NN, Thompson CB. The emerging hallmarks of cancer metabolism. Cell Metab (2016) 23:27–47. doi: 10.1016/j.cmet.2015.12.006
4. Vaghari-Tabari M, Ferns GA, Qujeq D, Andevari AN, Sabahi Z, Moein S. Signaling, metabolism, and cancer: An important relationship for therapeutic intervention. J Cell Physiol (2021) 236:5512–32. doi: 10.1002/jcp.30276
5. Li Z, Zhang H. Reprogramming of glucose, fatty acid and amino acid metabolism for cancer progression. Cell Mol Life Sci (2016) 73:377–92. doi: 10.1007/s00018-015-2070-4
6. Lunt SY, Vander Heiden MG. Aerobic glycolysis: meeting the metabolic requirements of cell proliferation. Annu Rev Cell Dev Biol (2011) 27:441–64. doi: 10.1146/annurev-cellbio-092910-154237
7. Abdel-Wahab AF, Mahmoud W, Al-Harizy RM. Targeting glucose metabolism to suppress cancer progression: prospective of anti-glycolytic cancer therapy. Pharmacol Res (2019) 150:104511. doi: 10.1016/j.phrs.2019.104511
8. Xia L, Oyang L, Lin J, Tan S, Han Y, Wu N, et al. The cancer metabolic reprogramming and immune response. Mol Cancer (2021) 20:1–21. doi: 10.1186/s12943-021-01316-8
9. von Schulthess GK, Steinert HC, Hany TF. Integrated pet/ct: current applications and future directions. Radiology (2006) 238:405–22. doi: 10.1148/radiol.2382041977
10. Hundshammer C, Braeuer M, Müller CA, Hansen AE, Schillmaier M, Düwel S, et al. Simultaneous characterization of tumor cellularity and the warburg effect with pet, mri and hyperpolarized 13c-mrsi. Theranostics (2018) 8:4765. doi: 10.7150/thno.25162
11. Hughes DJ, Subesinghe M, Taylor B, Bille A, Spicer J, Papa S, et al. 18f fdg pet/ct and novel molecular imaging for directing immunotherapy in cancer. Radiology (2022) 304:246–64. doi: 10.1148/radiol.212481
12. Eisenhauer EA, Therasse P, Bogaerts J, Schwartz LH, Sargent D, Ford R, et al. New response evaluation criteria in solid tumours: revised recist guideline (version 1.1). Eur J Cancer (2009) 45:228–47. doi: 10.1016/j.ejca.2008.10.026
13. Low JCM, Wright AJ, Hesse F, Cao J, Brindle KM. Metabolic imaging with deuterium labeled substrates. Prog Nucl Magnetic Resonance Spectrosc (2023) 39–51:40. doi: 10.1016/j.pnmrs.2023.02.002
14. De Feyter HM, de Graaf RA. Deuterium metabolic imaging–back to the future. J Magnetic Resonance (2021) 326:106932. doi: 10.1016/j.jmr.2021.106932
15. de Graaf RA, Hendriks AD, Klomp DW, Kumaragamage C, Welting D, Arteaga de Castro CS, et al. On the magnetic field dependence of deuterium metabolic imaging. NMR Biomedicine (2020) 33:e4235. doi: 10.1002/nbm.4235
16. Kostakoglu L, Agress H Jr., Goldsmith SJ. Clinical role of fdg pet in evaluation of cancer patients. Radiographics (2003) 23:315–40. doi: 10.1148/rg.232025705
17. Farwell MD, Pryma DA, Mankoff DA. Pet/ct imaging in cancer: current applications and future directions. Cancer (2014) 120:3433–45. doi: 10.1002/cncr.28860
18. Boellaard R, Delgado-Bolton R, Oyen WJ, Giammarile F, Tatsch K, Eschner W, et al. Fdg pet/ct: Eanm procedure guidelines for tumour imaging: version 2.0. Eur J Nucl Med Mol Imaging (2015) 42:328–54. doi: 10.1007/s00259-014-2961-x
19. McKay MJ, Taubman KL, Foroudi F, Lee ST, Scott AM. Molecular imaging using pet/ct for radiation therapy planning for adult cancers: current status and expanding applications. Int J Radiat Oncol Biol Phys (2018) 102:783–91. doi: 10.1016/j.ijrobp.2018.03.013
20. Kurhanewicz J, Vigneron DB, Brindle K, Chekmenev EY, Comment A, Cunningham CH, et al. Analysis of cancer metabolism by imaging hyperpolarized nuclei: prospects for translation to clinical research. Neoplasia (2011) 13:81–97. doi: 10.1593/neo.101102
21. Sengupta D, Pratx G. Imaging metabolic heterogeneity in cancer. Mol Cancer (2016) 15:1–12. doi: 10.1186/s12943-015-0481-3
22. Kurhanewicz J, Vigneron DB, Ardenkjaer-Larsen JH, Bankson JA, Brindle K, Cunningham CH, et al. Hyperpolarized 13c mri: path to clinical translation in oncology. Neoplasia (2019) 21:1–16. doi: 10.1016/j.neo.2018.09.006
23. Nelson SJ, Kurhanewicz J, Vigneron DB, Larson PE, Harzstark AL, Ferrone M, et al. Metabolic imaging of patients with prostate cancer using hyperpolarized [1-13c] pyruvate. Sci Trans Med (2013) 5:198ra108–198ra108. doi: 10.1126/scitranslmed.3006070
24. Autry AW, Gordon JW, Chen H-Y, LaFontaine M, Bok R, Van Criekinge M, et al. Characterization of serial hyperpolarized 13c metabolic imaging in patients with glioma. NeuroImage: Clin (2020) 27:102323. doi: 10.1016/j.nicl.2020.102323
25. Gallagher FA, Woitek R, McLean MA, Gill AB, Manzano Garcia R, Provenzano E, et al. Imaging breast cancer using hyperpolarized carbon-13 mri. Proc Natl Acad Sci (2020) 117:2092–8. doi: 10.1073/pnas.1913841117
26. Chen J, Patel TR, Pinho MC, Choi C, Harrison CE, Baxter JD, et al. Preoperative imaging of glioblastoma patients using hyperpolarized 13c pyruvate: Potential role in clinical decision making. Neuro-Oncology Adv (2021) 3:vdab092. doi: 10.1093/noajnl/vdab112.033
27. Tang S, Meng MV, Slater JB, Gordon JW, Vigneron DB, Stohr BA, et al. Metabolic imaging with hyperpolarized 13c pyruvate magnetic resonance imaging in patients with renal tumors—initial experience. Cancer (2021) 127:2693–704. doi: 10.1002/cncr.33554
28. Wang ZJ, Ohliger MA, Larson PE, Gordon JW, Bok RA, Slater J, et al. Hyperpolarized 13c mri: state of the art and future directions. Radiology (2019) 291:273–84. doi: 10.1148/radiol.2019182391
29. De Feyter HM, Behar KL, Corbin ZA, Fulbright RK, Brown PB, McIntyre S, et al. Deuterium metabolic imaging (dmi) for mri-based 3d mapping of metabolism in vivo. Sci Adv (2018) 4:eaat7314. doi: 10.1126/sciadv.aat7314
30. Polvoy I, Qin H, Flavell RR, Gordon J, Viswanath P, Sriram R, et al. Deuterium metabolic imaging—rediscovery of a spectroscopic tool. Metabolites (2021) 11:570. doi: 10.3390/metabo11090570
31. Rich LJ, Bagga P, Wilson NE, Schnall MD, Detre JA, Haris M, et al. 1h magnetic resonance spectroscopy of 2h-to-1h exchange quantifies the dynamics of cellular metabolism in vivo. Nat Biomed Eng (2020) 4:335–42. doi: 10.1038/s41551-019-0499-8
32. Kreis F, Wright AJ, Hesse F, Fala M, Hu D-e, Brindle KM. Measuring tumor glycolytic flux in vivo by using fast deuterium mri. Radiology (2020) 294:289–96. doi: 10.1148/radiol.2019191242
33. Hesse F, Somai V, Kreis F, Bulat F, Wright AJ, Brindle KM. Monitoring tumor cell death in murine tumor models using deuterium magnetic resonance spectroscopy and spectroscopic imaging. Proc Natl Acad Sci (2021) 118:e2014631118. doi: 10.1073/pnas.2014631118
34. Hesse F, Wright AJ, Bulat F, Somai V, Kreis F, Brindle KM. Deuterium mrsi of tumor cell death in vivo following oral delivery of 2h-labeled fumarate. Magnetic Resonance Med (2022) 88:2014–20. doi: 10.1002/mrm.29379
35. International Commission on Non-Ionizing Radiation Protection (ICNIRP). Medical magnetic resonance (mr) procedures: protection of patients. Health Phys (2004) 87:197–216. doi: 10.1097/00004032-200408000-00008
36. Niess F, Strasser B, Hingerl L, Niess E, Motyka S, Hangel G, et al. Reproducibility of 3d mrsi for imaging human brain glucose metabolism using direct (2h) and indirect (1h) detection of deuterium labeled compounds at 7t and clinical 3t. NeuroImage (2023) 277:120250. doi: 10.1016/j.neuroimage.2023.120250
37. Corbin ZA. New metabolic imaging tools in neuro-oncology. Curr Opin Neurol (2019) 32:872–7. doi: 10.1097/WCO.0000000000000758
38. Kaggie JD, Khan AS, Matys T, Schulte RF, Locke MJ, Grimmer A, et al. Deuterium metabolic imaging and hyperpolarized 13c-mri of the normal human brain at clinical field strength reveals differential cerebral metabolism. Neuroimage (2022) 257:119284. doi: 10.1016/j.neuroimage.2022.119284
39. Markovic S, Roussel T, Neeman M, Frydman L. Deuterium magnetic resonance imaging and the discrimination of fetoplacental metabolism in normal and l-name-induced preeclamptic mice. Metabolites (2021) 11:376. doi: 10.3390/metabo11060376
40. Schoenheimer R, Rittenberg D. Deuterium as an indicator in the study of intermediary metabolism. Science (1935) 82:156–7. doi: 10.1126/science.82.2120.156
41. Tan JL, Djayakarsana D, Wang H, Chan RW, Bailey C, Lau AZ. Deuterium mrs of early treatment-induced changes in tumour lactate in vitro. NMR Biomedicine (2021) 34:e4599. doi: 10.1002/nbm.4599
42. Veltien A, van Asten J, Ravichandran N, de Graaf RA, De Feyter HM, Oosterwijk E, et al. Simultaneous recording of the uptake and conversion of glucose and choline in tumors by deuterium metabolic imaging. Cancers (2021) 13:4034. doi: 10.3390/cancers13164034
43. Simões RV, Henriques RN, Cardoso BM, Fernandes FF, Carvalho T, Shemesh N. Glucose fluxes in glycolytic and oxidative pathways detected in vivo by deuterium magnetic resonance spectroscopy reflect proliferation in mouse glioblastoma. NeuroImage: Clin (2022) 33:102932. doi: 10.1016/j.nicl.2021.102932
44. Batsios G, Taglang C, Tran M, Stevers N, Barger C, Gillespie AM, et al. Deuterium metabolic imaging reports on tert expression and early response to therapy in cancer. Clin Cancer Res (2022) 28:3526–36. doi: 10.1158/1078-0432.CCR-21-4418
45. Taglang C, Batsios G, Mukherjee J, Tran M, Gillespie AM, Hong D, et al. Deuterium magnetic resonance spectroscopy enables noninvasive metabolic imaging of tumor burden and response to therapy in low-grade gliomas. Neuro-oncology (2022) 24:1101–12. doi: 10.1093/neuonc/noac022
46. Hartmann B, Müller M, Seyler L, Bäuerle T, Wilferth T, Avdievitch N, et al. Feasibility of deuterium magnetic resonance spectroscopy of 3-o-methylglucose at 7 tesla. PloS One (2021) 16:e0252935. doi: 10.1371/journal.pone.0252935
47. Vander Heiden MG, Cantley LC, Thompson CB. Understanding the warburg effect: the metabolic requirements of cell proliferation. science (2009) 324:1029–33. doi: 10.1126/science.1160809
48. Ganapathy-Kanniappan S, Geschwind J-FH. Tumor glycolysis as a target for cancer therapy: progress and prospects. Mol Cancer (2013) 12:1–11. doi: 10.1186/1476-4598-12-152
49. Ippolito L, Morandi A, Giannoni E, Chiarugi P. Lactate: a metabolic driver in the tumour landscape. Trends Biochem Sci (2019) 44:153–66. doi: 10.1016/j.tibs.2018.10.011
50. Elia I, Doglioni G, Fendt S-M. Metabolic hallmarks of metastasis formation. Trends Cell Biol (2018) 28:673–84. doi: 10.1016/j.tcb.2018.04.002
51. Li Y, Lu Z, Liang Z, Ji D, Zhang P, Liu Q, et al. Metastasis-associated in colon cancer-1 is associated with poor prognosis in hepatocellular carcinoma, partly by promoting proliferation through enhanced glucose metabolism. Mol Med Rep (2015) 12:426–34. doi: 10.3892/mmr.2015.3416
52. Sun H, Zhu A, Zhang L, Zhang J, Zhong Z, Wang F. Knockdown of pkm2 suppresses tumor growth and invasion in lung adenocarcinoma. Int J Mol Sci (2015) 16:24574–87. doi: 10.3390/ijms161024574
53. Yu G, Yu W, Jin G, Xu D, Chen Y, Xia T, et al. Pkm2 regulates neural invasion of and predicts poor prognosis for human hilar cholangiocarcinoma. Mol Cancer (2015) 14:1–13. doi: 10.1186/s12943-015-0462-6
54. Edry Botzer L, Maman S, Sagi-Assif O, Meshel T, Nevo I, Yron I, et al. Hexokinase 2 is a determinant of neuroblastoma metastasis. Br J Cancer (2016) 114:759–66. doi: 10.1038/bjc.2016.26
55. Lu W, Cao Y, Zhang Y, Li S, Gao J, Wang X-A, et al. Up-regulation of pkm2 promote Malignancy and related to adverse prognostic risk factor in human gallbladder cancer. Sci Rep (2016) 6:26351. doi: 10.1038/srep26351
56. Gallagher FA, Kettunen MI, Hu D-E, Jensen PR, Zandt Ri, Karlsson M, et al. Production of hyperpolarized [1, 4-13c2] malate from [1, 4-13c2] fumarate is a marker of cell necrosis and treatment response in tumors. Proc Natl Acad Sci (2009) 106:19801–6. doi: 10.1073/pnas.0911447106
57. Bohndiek SE, Kettunen MI, Hu D-e, Witney TH, Kennedy BW, Gallagher FA, et al. Detection of tumor response to a vascular disrupting agent by hyperpolarized 13c magnetic resonance spectroscopy. Mol Cancer Ther (2010) 9:3278–88. doi: 10.1158/1535-7163.MCT-10-0706
58. Witney T, Kettunen M, Hu D, Gallagher F, Bohndiek S, Napolitano R, et al. Detecting treatment response in a model of human breast adenocarcinoma using hyperpolarised [1-13c] pyruvate and [1, 4-13c2] fumarate. Br J Cancer (2010) 103:1400–6. doi: 10.1038/sj.bjc.6605945
59. Clatworthy MR, Kettunen MI, Hu D-E, Mathews RJ, Witney TH, Kennedy BW, et al. Magnetic resonance imaging with hyperpolarized [1, 4-13c2] fumarate allows detection of early renal acute tubular necrosis. Proc Natl Acad Sci (2012) 109:13374–9. doi: 10.1073/pnas.1205539109
60. Mignion L, Dutta P, Martinez GV, Foroutan P, Gillies RJ, Jordan BF. Monitoring chemotherapeutic response by hyperpolarized 13c-fumarate mrs and diffusion mri. Cancer Res (2014) 74:686–94. doi: 10.1158/0008-5472.CAN-13-1914
61. Miller JJ, Lau AZ, Nielsen PM, McMullen-Klein G, Lewis AJ, Jespersen NR, et al. Hyperpolarized [1, 4-13c2] fumarate enables magnetic resonance-based imaging of myocardial necrosis. JACC: Cardiovasc Imaging (2018) 11:1594–606. doi: 10.1016/j.jcmg.2017.09.020
62. Shay JW. Role of telomeres and telomerase in aging and cancer. Cancer Discovery (2016) 6:584–93. doi: 10.1158/2159-8290.CD-16-0062
63. Li Y, Ma Y, Wu Z, Xie R, Zeng F, Cai H, et al. Advanced imaging techniques for differentiating pseudoprogression and tumor recurrence after immunotherapy for glioblastoma. Front Immunol (2021) 12:790674. doi: 10.3389/fimmu.2021.790674
64. Yuan H, Wu Y, Wang J, Qin X, Huang Y, Yan L, et al. Synergistic effects of telomerase reverse transcriptase and regulator of telomere elongation helicase 1 on aggressiveness and outcomes in adrenocortical carcinoma. Biomed Pharmacother (2022) 149:112796. doi: 10.1016/j.biopha.2022.112796
65. Cesare AJ, Reddel RR. Alternative lengthening of telomeres: models, mechanisms and implications. Nat Rev Genet (2010) 11:319–30. doi: 10.1038/nrg2763
66. Dilley RL, Greenberg RA. Alternative telomere maintenance and cancer. Trends Cancer (2015) 1:145–56. doi: 10.1016/j.trecan.2015.07.007
67. Brahmer JR, Tykodi SS, Chow LQ, Hwu W-J, Topalian SL, Hwu P, et al. Safety and activity of anti–pd-l1 antibody in patients with advanced cancer. New Engl J Med (2012) 366:2455–65. doi: 10.1056/NEJMoa1200694
68. Pardoll DM. The blockade of immune checkpoints in cancer immunotherapy. Nat Rev Cancer (2012) 12:252–64. doi: 10.1038/nrc3239
69. Yang Y. Cancer immunotherapy: harnessing the immune system to battle cancer. J Clin Invest (2015) 125:3335–7:6–7. doi: 10.1172/JCI83871
70. Biswas SK. Metabolic reprogramming of immune cells in cancer progression. Immunity (2015) 43:435–49. doi: 10.1016/j.immuni.2015.09.001
71. Wang Z-H, Peng W-B, Zhang P, Yang X-P, Zhou Q. Lactate in the tumour microenvironment: From immune modulation to therapy. EBioMedicine (2021) 73:6–7. doi: 10.1016/j.ebiom.2021.103627
72. Sukumar M, Liu J, Ji Y, Subramanian M, Crompton JG, Yu Z, et al. Inhibiting glycolytic metabolism enhances cd8+ t cell memory and antitumor function. J Clin Invest (2013) 123:4479–88. doi: 10.1172/JCI69589
73. Renner K, Bruss C, Schnell A, Koehl G, Becker HM, Fante M, et al. Restricting glycolysis preserves t cell effector functions and augments checkpoint therapy. Cell Rep (2019) 29:135–50. doi: 10.1016/j.celrep.2019.08.068
74. Lei J, Yang Y, Lu Z, Pan H, Fang J, Jing B, et al. Taming metabolic competition via glycolysis inhibition for safe and potent tumor immunotherapy. Biochem Pharmacol (2022) 202:115153. doi: 10.1016/j.bcp.2022.115153
75. El Hassouni B, Granchi C, Vallés-Martí A, Supadmanaba IGP, Bononi G, Tuccinardi T, et al. The dichotomous role of the glycolytic metabolism pathway in cancer metastasis: interplay with the complex tumor microenvironment and novel therapeutic strategies. In Semin Cancer Biol (2020) 60:238–48. doi: 10.1016/j.semcancer.2019.08.025
Keywords: cancer metabolism, magnetic resonance imaging, molecular imaging, deuterium metabolic imaging (DMI), cancer immunotherapy
Citation: Wan J, Guo Y, Chen H, Sun P, Zhang X, Ye T, Li L, Pan F and Yang L (2023) Application and development of Deuterium Metabolic Imaging in tumor glucose metabolism: visualization of different metabolic pathways. Front. Oncol. 13:1285209. doi: 10.3389/fonc.2023.1285209
Received: 31 August 2023; Accepted: 01 November 2023;
Published: 28 November 2023.
Edited by:
Juan Carlos Gallardo-Pérez, National Institute of Cardiology Ignacio Chavez, MexicoReviewed by:
James Grist, University of Oxford, United KingdomCopyright © 2023 Wan, Guo, Chen, Sun, Zhang, Ye, Li, Pan and Yang. This is an open-access article distributed under the terms of the Creative Commons Attribution License (CC BY). The use, distribution or reproduction in other forums is permitted, provided the original author(s) and the copyright owner(s) are credited and that the original publication in this journal is cited, in accordance with accepted academic practice. No use, distribution or reproduction is permitted which does not comply with these terms.
*Correspondence: Feng Pan, dWhmZW5ncGFuQGh1c3QuZWR1LmNu; Lian Yang, eWFuZ2xpYW5AaHVzdC5lZHUuY24=
Disclaimer: All claims expressed in this article are solely those of the authors and do not necessarily represent those of their affiliated organizations, or those of the publisher, the editors and the reviewers. Any product that may be evaluated in this article or claim that may be made by its manufacturer is not guaranteed or endorsed by the publisher.
Research integrity at Frontiers
Learn more about the work of our research integrity team to safeguard the quality of each article we publish.