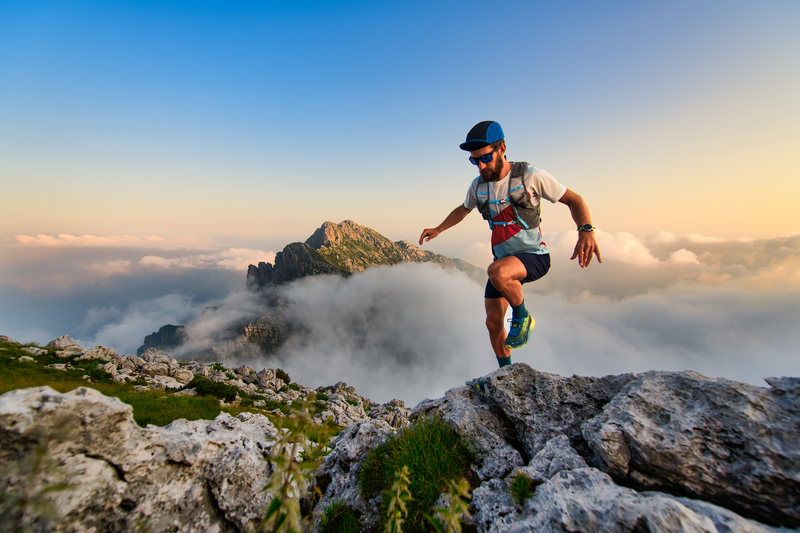
95% of researchers rate our articles as excellent or good
Learn more about the work of our research integrity team to safeguard the quality of each article we publish.
Find out more
ORIGINAL RESEARCH article
Front. Oncol. , 09 February 2024
Sec. Cancer Epidemiology and Prevention
Volume 13 - 2023 | https://doi.org/10.3389/fonc.2023.1275913
This article is part of the Research Topic Mendelian Randomization: the Study of Causal Relationships between Tumors and Underlying Pathogenic Factors View all 15 articles
Background: Previous research has indicated that there may be a link between Crohn’s disease (CD) and breast cancer (BC), but the causality remains unclear. This study aimed to investigate the causal association between CD and BC using Mendelian randomization (MR) analysis.
Methods: The summary data for CD (5,956 cases/14,927 controls) was obtained from the International Inflammatory Bowel Disease Genetics Consortium (IIBDGC). And the summary data for BC (122,977 cases/105,974 controls) was extracted from the Breast Cancer Association Consortium (BCAC). Based on the estrogen receptor status, the cases were classified into two subtypes: estrogen receptor-positive (ER+) BC and estrogen receptor-negative (ER−) BC. We used the inverse variance weighted method as the primary approach for two-sample MR. MR-PRESSO method was used to rule out outliers. Heterogeneity and pleiotropy tests were carried out to improve the accuracy of results. Additionally, multivariable MR was conducted by adjusting for possible confounders to ensure the stability of the results.
Results: The two-sample MR indicated that CD increased the risks of overall (OR: 1.020; 95% CI: 1.010-1.031; p=0.000106), ER+ (OR: 1.019; 95%CI: 1.006-1.034; p=0.006) and ER− BC (OR: 1.019; 95%CI: 1.000-1.037; p=0.046) after removal of outliers by MR-PRESSO. This result was reliable in the sensitivity analysis, including Cochran’s Q and MR-Egger regression. In multivariate MR analyses, after adjusting for smoking and drinking separately or concurrently, the positive association between CD and the risks of overall and ER+ BC remained, but it disappeared in ER− BC. Furthermore, reverse MR analysis suggested that BC did not have a significant impact on CD risk.
Conclusion: Our findings provide evidence for a possible positive association between CD and the risk of BC. However, further studies are needed to fully understand the underlying mechanisms and establish a stronger causal relationship.
Crohn’s disease (CD) is a chronic and progressive inflammatory disease characterized by alternating periods of remission and relapse (1, 2). CD primarily affects the gastrointestinal tract with extraintestinal manifestations and related immune dysregulation (3). Patients with CD are more susceptible to cancer, depression, and infection (4).
Breast cancer (BC) is the most prevalent malignancy among women globally, with 684,996 deaths reported in 2020, representing a substantial threat to their health (5–7). Patients with CD have an increased risk of digestive tract, skin, bladder, and lung cancers (8, 9), but the association between CD and BC remains unclear. Chronic inflammation characterized by sustained immune activation is associated with promoting the occurrence, growth, and progression of BC (10–12). Several researchers have investigated the association between CD and BC. Riegler et al. found first-degree relatives of patients with CD have a higher risk of developing BC (13). Further, a study by Pellino et al. showed that CD was an independent risk factor for BC (OR: 2.76; 95% CI: 1.2-6.2; p=0.017) (14). In contrast, Gong et al. reported no significant association between CD and BC risk (15). Hence, there is controversy regarding the relationship between CD and BC risk. In addition, immunosuppressive medications are the cornerstone of long-term maintenance treatment for CD (16). Due to the decreased immune surveillance, immunosuppression may potentially increase the risk of cancer (17). A retrospective study attributed the development of BC in CD patients to immunosuppressive therapy (18). Thus, the association between CD itself and BC needs to be further investigated. Moreover, assessing the true causal association between CD and BC is challenging due to the interference of common residual confounders and reverse causality in traditional observational studies.
To overcome these challenges and gain a more nuanced understanding of the causality between CD and BC, we turned to Mendelian randomization (MR). MR is a robust statistical method that harnesses genetic variants as instrumental variables (IVs) to explore causal connections between exposure and outcome (19, 20). By capitalizing on the natural random assortment of genetic variants during conception, MR effectively mimics the randomized controlled trial (RCT) setting, thereby mitigating issues like confounding and reverse causation that often plague observational studies (21–23).
In order to assess the potential causal association between CD and BC, we conducted a two-sample MR study. The single nucleotide polymorphisms (SNPs) selected as IVs were required to adhere to three following key premises (24): (1) SNPs must be intensely linked to CD; (2) SNPs must not be linked to confounding factors; and (3) SNPs should not be directly linked to BC (Figure 1).
The summary data for CD (5,956 cases/14,927 controls) was obtained from the International Inflammatory Bowel Disease Genetics Consortium (IIBDGC) (25). And the summary data for BC (122,977 cases/105,974 controls) was extracted from the Breast Cancer Association Consortium (BCAC). Based on the estrogen receptor status, the cases were classified into two subtypes: estrogen receptor-positive (ER+) BC and estrogen receptor-negative (ER−) BC (26). Table 1 presents details of the exposure and outcomes.
First, we screened for SNPs that were strongly associated with exposure at a genome-wide significance level (p < 5×10–8). Second, we implemented a criterion (r2 < 0.001, kb=10000) to select SNPs that were independent of linkage disequilibrium (LD) (27). Third, we excluded SNPs that were not found in the BC dataset and palindromic SNPs that may cause bias. Next, we harmonized the exposure and outcome data, ensuring that the effect of the SNP on the exposure corresponded to the same allele as the effect on the outcome. Subsequently, we evaluated the possibility of weak instrumental bias by calculating F-statistics and excluded SNPs with F-statistics less than 10 (28, 29). The F statistic was calculated as F = beta2/se2 (30, 31). Finally, the MR-PRESSO method was conducted to detect outlier SNPs (32), and after excluding these outlier SNPs, the remaining SNPs were used for subsequent MR analysis. Figure 2 shows the selection flowchart.
Three commonly used MR methods were applied to estimate causal effects: inverse variance weighted (IVW) (24), weighted median (33), and MR-Egger (34). The IVW method is considered to be the most effective method for assessing causality (35); therefore, the results were mainly based on the IVW method. We used odds ratios (ORs) to express the effects of CD on BC risk. If the result of the IVW method is significant (p < 0.05), even if no significant result is obtained by the other methods, it can be considered as a positive result as long as the ORs of the other methods are in the same direction and there is no heterogeneity or pleiotropy (36).
Cochran’s Q test was employed to assess heterogeneity, with p > 0.05 representing the absence of heterogeneity (37). The MR-Egger regression test was applied to detect horizontal pleiotropy, with a zero intercept signifying the absence of pleiotropy (p > 0.05) (38).
Based on the search results on the PhenoScanner website and possible confounders between CD and BC, we performed multivariable MR (MVMR) analyses with the addition of smoking and drinking separately or together to adjust for causal impacts between exposure and outcome (39).
To explore whether BC has any causal effect on CD, we also conducted a reverse MR analysis (i.e., BC as the exposure and CD as the outcome) using SNPs related to BC as IVs.
All analyses were performed in R software (version 4.2.3) using the “TwoSampleMR” (version 0.5.6), “MRPRESSO” (version 1.0), and “MendelianRandomization” (version 0.7.0) packages (40).
Initially, we extracted 53 genome-wide significant (p<5×10-8) SNPs associated with CD. No SNPs were ruled out due to LD. Next, during the extraction of information on IVs and outcome, we excluded rs11564236 due to the lack of corresponding outcome data. Additionally, we excluded one palindromic SNP (rs12692254) while harmonizing the exposure and outcome data. Furthermore, we removed rs7543234 from the analysis of overall BC due to its association with the outcome. Finally, potentially outlier SNPs were excluded using MR-PRESSO. Specifically, rs12194825, rs1873625, rs2188962, and rs3091315 were excluded from the analysis of overall BC; rs12194825, rs1873625, rs2188962, and rs7543234 were excluded from the analysis of ER+ BC, and rs1873625 and rs3091315 were removed from the analysis of ER− BC. The F-statistics of all SNPs were greater than 10. After removing these SNPs, 46 SNPs, 47 SNPs, and 49 SNPs were included in the analysis of overall, ER+, and ER− BC, respectively (Supplementary Sheet).
Using existing SNPs as IV, the results of the IVW method showed that CD was positively associated with the risks of overall (OR: 1.020; 95% CI: 1.010-1.031; p=0.000106), ER+ (OR: 1.019; 95% CI: 1.006-1.034; p=0.006), and ER− (OR: 1.019; 95% CI: 1.000-1.037; p=0.046) BC (Figure 3). The scatterplot depicts the causal estimates obtained from every SNP (Figure 4). Although the weighted median and MR-Egger methods did not obtain significant results (p > 0.05), the direction of the ORs was consistent with the IVW method (OR > 1). Furthermore, Cochran’s Q and MR-Egger regression analyses demonstrated that there was no heterogeneity or horizontal pleiotropy affecting the stability of the results. The same result was also suggested by the symmetry of the funnel plots (Figure 5). Therefore, based on the significant IVW results (p < 0.05), we can conclude that there is a causal effect of CD on BC. The details of the results are presented in Table 2.
Figure 3 Forest plot of the impact of Crohn’s disease on breast cancer risk using IVW method (after removing outliers). Het.p refers to the p-value for heterogeneity; Ple.p refers to the p-value for pleiotropy; OR, odds ratio; CI, confidence interval.
Figure 4 Scatterplots for effects of Crohn’s disease on breast cancer risk (after removing outliers). (A) overall BC; (B) ER+ BC; (C) ER− BC.
Figure 5 Funnel plots for effects of Crohn’s disease on breast cancer risk (after removing outliers). (A) overall BC; (B) ER+ BC; (C) ER− BC.
After adjusting for current tobacco smoking and alcoholic drinks per week separately or together, MVMR analysis revealed that the positive association between CD and the risks of overall and ER+ BC remained, but it disappeared in ER− BC. In addition, no potential horizontal pleiotropy was discovered for the MR-Egger intercept (Table 3). Results of MVMR suggested that the observed effects of CD on overall and ER+ BC were stable and not influenced by potential confounders.
In the reverse study (BC on the risk of CD), no genetic effects of overall BC (OR: 1.082; 95% CI: 0.989-1.183; p=0.085), ER+ BC (OR: 1.039; 95% CI: 0.950-1.136; p=0.405), and ER− BC (OR: 1.033; 95% CI: 0.924-1.156; p=0.567) on the risk of CD were detected (Table 4). In all of the analyses, MR-Egger regression did not show IVs had horizontal pleiotropy. Therefore, genetically predicted BC exerts no impact on the risk of CD.
In this study, we carried out two-sample MR analyses to appraise the causal relationship of CD with overall, ER+, and ER− BC for the first time. The results showed that CD increased the risks of overall, ER+, and ER− BC. We further assessed the robustness of the results by MVMR analysis. However, in MVMR analysis, CD only increased the risks of overall and ER+ BC, but not ER− BC. This suggested a potential impact of smoking and alcohol drinking on the correlation between CD and ER− BC. Additionally, reverse MR analysis revealed that BC did not have a significant impact on CD risk.
However, a recent MR study found no association between CD and BC risk (41). We analyzed possible reasons for the discrepancy. Their study included only 732 cases of CD, whereas our study included 5,956 cases. They used a significance threshold of p<5×10-6 for SNP selection, but re-running MR on their data at p<5×10-8 revealed a positive association of CD on BC risk (p=0.016). Furthermore, we conducted subtype analyses based on estrogen receptor status and performed MVMR to adjust for possible confounding factors.
This MR study provides some insights into the association between CD and BC. Some studies have also revealed an elevated risk of BC in patients with CD (13, 14). The result of a 20-year follow-up study indicated that CD patients have a higher risk of developing BC (42). In addition, a study from Denmark showed BC patients with CD have a more advanced stage and a worse chemotherapy prognosis than patients without CD (43).
Several possible factors may account for the association between CD and BC. Existing studies indicated that CD and BC may share common molecular mechanisms. Recent evidence suggested that there are 53 overlapping differentially expressed genes between the CD and BC, with enrichment analyses showing that both diseases are related to NF-κB signaling pathways and interleukin-17 (IL-17) (44). It has been shown that inflammation is involved in the process of development and progression of malignant tumors (45). T helper 17 (Th17) cells are important inflammatory mediators in CD, and when Th17 cells reach breast tumor tissues, they upregulate a variety of cytokines including IL-17 and tumor necrosis factor-α (TNF-α) (46). IL-17 can upregulate the expression of chemokine CXCL1 in BC cells. This chemokine increases the activation of the AKT/NF-κB signaling pathway to promote BC growth and metastasis (47). Furthermore, previous studies have indicated that TNF-α is involved in epithelial-mesenchymal transition (EMT), thereby promoting tumor metastasis (48). A study conducted on patients with inflammatory BC demonstrated a direct association between TNF-α and the presence of tumor cells expressing EMT markers (49). In addition, there is another potential point of association between CD and BC that lies in the involvement of estrogen and the G protein-coupled estrogen receptor (GPER) (50, 51). GPER has been shown to regulate intestinal function, inflammation, and immune responses, and promote the occurrence and progression of BC (52, 53).
There is growing interest in the role of the microbiome in health and disease. Studies in human subjects have revealed distinct differences in the gut microbiome between patients with CD and healthy control subjects (54). Notably, the gut microbiome also affects the risk of developing BC (55). Dysbiosis of the intestinal flora has been found to have a direct effect on the dissemination of breast tumors (56, 57). The gut microbiome may also be involved in the correlation between CD and the risk of BC, and more relevant research is needed to confirm this in the future.
Research has demonstrated that chronic psychological stress can inhibit the anti-tumor effects of the immune system in CD (58). Intestinal inflammation in CD can activate the hypothalamic–pituitary–adrenal (HPA) axis through the opposite action of the brain-gut axis, thereby inducing anxiety and depression (59, 60). Several studies have shown that patients with BC also experience varying degrees of anxiety and depression (61). Hence, the mechanisms behind the effects of mental and emotional factors on CD and BC need to be further explored. The possible mechanisms for the effect of CD on BC risk are depicted in Figure 6.
The strength of our study is that it explored the causality between CD and BC risk by MR analysis. Compared to previous observational studies that found CD to be associated with BC, MR studies are less susceptible to confounders and reverse causation. Besides, our study utilized a large sample size and SNPs from GWAS, providing sufficient statistical validity to estimate causality. Furthermore, sensitivity analyses enhanced the credibility of our results.
Nevertheless, this study has several limitations. First, the GWAS data for this study included only European populations, which limits the application of our findings to other populations. Hence, future studies are required to verify the applicability of our results to different populations. Second, we cannot stratify the analysis by sex due to the lack of sex-specific GWAS data. Third, the OR of CD on BC risk is relatively small, indicating that the enhanced risk is just modest. Therefore, we don’t recommend that patients with CD be screened for BC more frequently or earlier than the routine screening. Finally, MR also has its limitations. (1) SNPs are generally considered to have lifetime effects, but in specific situations, the effects of SNPs may vary due to an individual’s physiological status, environmental factors, or interactions with other genetic variations. If the genetic variants used in MR analysis change over time, it could potentially affect the validity of the causal estimates. (2) Additional adjustments for smoking and alcohol consumption may lead to collider bias. (3) The MR study can only analyze the causality and cannot explain the mechanism of CD on BC risk. Further research is necessary to investigate the mechanisms behind the link between CD and the risk of BC.
Our findings provide evidence for a potential positive association between CD and the risk of BC. However, further studies are needed to fully understand the underlying mechanisms and establish a stronger causal relationship.
In this study, all GWAS data were extracted from the IEU Open GWAS project (https://gwas.mrcieu.ac.uk/).
CY: Conceptualization, Investigation, Methodology, Writing – original draft. JX: Data curation, Writing – original draft. SX: Data curation, Writing – review & editing. YH: Writing – review & editing. LT: Writing – review & editing. XZ: Writing – review & editing. TY: Formal Analysis, Writing – original draft. WC: Visualization, Writing – review & editing. ZS: Funding acquisition, Supervision, Validation, Writing – review & editing.
The author(s) declare financial support was received for theresearch, authorship, and/or publication of this article. This work was supported by the Wu Jieping Medical Foundation (320.6750.2020-20-17) and the Open Fund for Scientific Research of Jiangxi Cancer Hospital (2021K04).
Thanks to the MRC Integrative Epidemiology Unit (IEU) at the University of Bristol for their invaluable contribution to the development of the IEU open GWAS project. Thank them for deriving relevant GWAS data from IIBDGC and BCAC. We plotted Figure 6 by Figdraw, thanks to Home for Researchers for providing the plotting platform.
The authors declare that the research was conducted in the absence of any commercial or financial relationships that could be construed as a potential conflict of interest.
All claims expressed in this article are solely those of the authors and do not necessarily represent those of their affiliated organizations, or those of the publisher, the editors and the reviewers. Any product that may be evaluated in this article, or claim that may be made by its manufacturer, is not guaranteed or endorsed by the publisher.
The Supplementary Material for this article can be found online at: https://www.frontiersin.org/articles/10.3389/fonc.2023.1275913/full#supplementary-material
1. Torres J, Mehandru S, Colombel JF, Peyrin-Biroulet L. Crohn’s disease. Lancet (2017) 389(10080):1741–55. doi: 10.1016/S0140-6736(16)31711-1
2. Noor NM, Verstockt B, Parkes M, Lee JC. Personalised medicine in Crohn’s disease. Lancet Gastroenterol Hepatol (2020) 5(1):80–92. doi: 10.1016/S2468-1253(19)30340-1
3. Roda G, Chien Ng S, Kotze PG, Argollo M, Panaccione R, Spinelli A, et al. Crohn’s disease. Nat Rev Dis Primers (2020) 6(1):22. doi: 10.1038/s41572-020-0156-2
4. Veauthier B, Hornecker JR. Crohn’s disease: diagnosis and management. Am Fam Physician (2018) 98(11):661–9.
5. Sung H, Ferlay J, Siegel RL, Laversanne M, Soerjomataram I, Jemal A, et al. Global Cancer Statistics 2020: GLOBOCAN estimates of incidence and mortality worldwide for 36 cancers in 185 countries. CA Cancer J Clin (2021) 71(3):209–49. doi: 10.3322/caac.21660
6. Arnold M, Morgan E, Rumgay H, Mafra A, Singh D, Laversanne M, et al. Current and future burden of breast cancer: Global statistics for 2020 and 2040. Breast (2022) 66:15–23. doi: 10.1016/j.breast.2022.08.010
7. Loibl S, Poortmans P, Morrow M, Denkert C, Curigliano G. Breast cancer. Lancet (2021) 397(10286):1750–69. doi: 10.1016/S0140-6736(20)32381-3
8. Olen O, Erichsen R, Sachs MC, Pedersen L, Halfvarson J, Askling J, et al. Colorectal cancer in Crohn’s disease: a Scandinavian population-based cohort study. Lancet Gastroenterol Hepatol (2020) 5(5):475–84. doi: 10.1016/S2468-1253(20)30005-4
9. Pedersen N, Duricova D, Elkjaer M, Gamborg M, Munkholm P, Jess T. Risk of extra-intestinal cancer in inflammatory bowel disease: meta-analysis of population-based cohort studies. Am J Gastroenterol (2010) 105(7):1480–7. doi: 10.1038/ajg.2009.760
10. Atsumi T, Singh R, Sabharwal L, Bando H, Meng J, Arima Y, et al. Inflammation amplifier, a new paradigm in cancer biology. Cancer Res (2014) 74(1):8–14. doi: 10.1158/0008-5472.CAN-13-2322
11. Cole SW. Chronic inflammation and breast cancer recurrence. J Clin Oncol (2009) 27(21):3418–9. doi: 10.1200/JCO.2009.21.9782
12. Danforth DN. The role of chronic inflammation in the development of breast cancer. Cancers (Basel) (2021) 13(15):3918. doi: 10.3390/cancers13153918
13. Riegler G, Caserta L, Castiglione F, Esposito I, Valpiani D, Annese V, et al. Increased risk of breast cancer in first-degree relatives of Crohn’s disease patients. An IG-IBD study. Dig Liver Dis (2006) 38(1):18–23. doi: 10.1016/j.dld.2005.07.006
14. Pellino G, Sciaudone G, Patturelli M, Candilio G, De Fatico GS, Landino I, et al. Relatives of Crohn’s disease patients and breast cancer: an overlooked condition. Int J Surg (2014) 12(Suppl 1):S156–8. doi: 10.1016/j.ijsu.2014.05.022
15. Gong C, Xu R, Zou P, Zhang Y, Wang X. Inflammatory bowel disease and risk of breast cancer: a meta-analysis of cohort studies. Eur J Cancer Prev (2022) 31(1):54–63. doi: 10.1097/CEJ.0000000000000667
16. Hazenberg H, de Boer NKH, Mulder CJJ, Mom SH, van Bodegraven AA, Tack Md Ph DG. Neoplasia and precursor lesions of the female genital tract in IBD: epidemiology, role of immunosuppressants, and clinical implications. Inflammation Bowel Dis (2018) 24(3):510–31. doi: 10.1093/ibd/izx062
17. Annese V, Beaugerie L, Egan L, Biancone L, Bolling C, Brandts C, et al. European evidence-based consensus: inflammatory bowel disease and Malignancies. J Crohns Colitis (2015) 9(11):945–65. doi: 10.1093/ecco-jcc/jjv141
18. Hamzaoglu H, Cooper J, Alsahli M, Falchuk KR, Peppercorn MA, Farrell RJ. Safety of infliximab in Crohn’s disease: a large single-center experience. Inflammation Bowel Dis (2010) 16(12):2109–16. doi: 10.1002/ibd.21290
19. Richmond RC, Davey Smith G. Mendelian randomization: concepts and scope. Cold Spring Harb Perspect Med (2022) 12(1):a040501. doi: 10.1101/cshperspect.a040501
20. Davey Smith G, Hemani G. Mendelian randomization: genetic anchors for causal inference in epidemiological studies. Hum Mol Genet (2014) 23(R1):R89–98. doi: 10.1093/hmg/ddu328
21. Yarmolinsky J, Wade KH, Richmond RC, Langdon RJ, Bull CJ, Tilling KM, et al. Causal inference in cancer epidemiology: what is the role of Mendelian randomization? Cancer Epidemiol Biomarkers Prev (2018) 27(9):995–1010. doi: 10.1158/1055-9965.EPI-17-1177
22. Sekula P, Del Greco MF, Pattaro C, Kottgen A. Mendelian randomization as an approach to assess causality using observational data. J Am Soc Nephrol (2016) 27(11):3253–65. doi: 10.1681/ASN.2016010098
23. Emdin CA, Khera AV, Kathiresan S. Mendelian randomization. JAMA (2017) 318(19):1925–6. doi: 10.1001/jama.2017.17219
24. Burgess S, Butterworth A, Thompson SG. Mendelian randomization analysis with multiple genetic variants using summarized data. Genet Epidemiol (2013) 37(7):658–65. doi: 10.1002/gepi.21758
25. Liu JZ, van Sommeren S, Huang H, Ng SC, Alberts R, Takahashi A, et al. Association analyses identify 38 susceptibility loci for inflammatory bowel disease and highlight shared genetic risk across populations. Nat Genet (2015) 47(9):979–86. doi: 10.1038/ng.3359
26. Michailidou K, Lindstrom S, Dennis J, Beesley J, Hui S, Kar S, et al. Association analysis identifies 65 new breast cancer risk loci. Nature (2017) 551(7678):92–4. doi: 10.1038/nature24284
27. Nounu A, Kar SP, Relton CL, Richmond RC. Sex steroid hormones and risk of breast cancer: a two-sample Mendelian randomization study. Breast Cancer Res (2022) 24(1):66. doi: 10.1186/s13058-022-01553-9
28. Burgess S, Thompson SG. Use of allele scores as instrumental variables for Mendelian randomization. Int J Epidemiol (2013) 42(4):1134–44. doi: 10.1093/ije/dyt093
29. Pierce BL, Ahsan H, Vanderweele TJ. Power and instrument strength requirements for Mendelian randomization studies using multiple genetic variants. Int J Epidemiol (2011) 40(3):740–52. doi: 10.1093/ije/dyq151
30. Huang W, Xiao J, Ji J, Chen L. Association of lipid-lowering drugs with COVID-19 outcomes from a Mendelian randomization study. Elife (2021) 10:e73873. doi: 10.7554/eLife.73873
31. Feng R, Lu M, Xu J, Zhang F, Yang M, Luo P, et al. Pulmonary embolism and 529 human blood metabolites: genetic correlation and two-sample Mendelian randomization study. BMC Genom Data (2022) 23(1):69. doi: 10.1186/s12863-022-01082-6
32. Verbanck M, Chen CY, Neale B, Do R. Detection of widespread horizontal pleiotropy in causal relationships inferred from Mendelian randomization between complex traits and diseases. Nat Genet (2018) 50(5):693–8. doi: 10.1038/s41588-018-0099-7
33. Bowden J, Davey Smith G, Haycock PC, Burgess S. Consistent estimation in Mendelian randomization with some invalid instruments using a weighted median estimator. Genet Epidemiol (2016) 40(4):304–14. doi: 10.1002/gepi.21965
34. Bowden J, Davey Smith G, Burgess S. Mendelian randomization with invalid instruments: effect estimation and bias detection through Egger regression. Int J Epidemiol (2015) 44(2):512–25. doi: 10.1093/ije/dyv080
35. Hartwig FP, Davey Smith G, Bowden J. Robust inference in summary data Mendelian randomization via the zero modal pleiotropy assumption. Int J Epidemiol (2017) 46(6):1985–98. doi: 10.1093/ije/dyx102
36. Wang S, Zhu H, Pan L, Zhang M, Wan X, Xu H, et al. Systemic inflammatory regulators and risk of acute-on-chronic liver failure: A bidirectional mendelian-randomization study. Front Cell Dev Biol (2023) 11:1125233. doi: 10.3389/fcell.2023.1125233
37. Cohen JF, Chalumeau M, Cohen R, Korevaar DA, Khoshnood B, Bossuyt PM. Cochran’s Q test was useful to assess heterogeneity in likelihood ratios in studies of diagnostic accuracy. J Clin Epidemiol (2015) 68(3):299–306. doi: 10.1016/j.jclinepi.2014.09.005
38. Burgess S, Thompson SG. Interpreting findings from Mendelian randomization using the MR-Egger method. Eur J Epidemiol (2017) 32(5):377–89. doi: 10.1007/s10654-017-0255-x
39. Sanderson E. Multivariable Mendelian randomization and mediation. Cold Spring Harb Perspect Med (2021) 11(2):a038984. doi: 10.1101/cshperspect.a038984
40. Yavorska OO, Burgess S. MendelianRandomization: an R package for performing Mendelian randomization analyses using summarized data. Int J Epidemiol (2017) 46(6):1734–9. doi: 10.1093/ije/dyx034
41. Lu Y, Ma L. Investigation of the causal relationship between breast cancer and autoimmune diseases: A bidirectional mendelian randomization study. Med (Baltimore) (2023) 102(34):e34612. doi: 10.1097/MD.0000000000034612
42. Hovde O, Hoivik ML, Henriksen M, Solberg IC, Smastuen MC, Moum BA. Malignancies in patients with inflammatory bowel disease: results from 20 years of follow-up in the IBSEN study. J Crohns Colitis (2017) 11(5):571–7. doi: 10.1093/ecco-jcc/jjw193
43. Sogaard KK, Cronin-Fenton DP, Pedersen L, Sorensen HT, Lash TL. Survival in Danish patients with breast cancer and inflammatory bowel disease: a nationwide cohort study. Inflammation Bowel Dis (2008) 14(4):519–25. doi: 10.1002/ibd.20341
44. Zhou J, Yang R. Identification of key pathways and genes shared between Crohn’s disease and breast cancer using bioinformatics analysis. Oncol Lett (2020) 20(4):119. doi: 10.3892/ol.2020.11981
45. Diakos CI, Charles KA, McMillan DC, Clarke SJ. Cancer-related inflammation and treatment effectiveness. Lancet Oncol (2014) 15(11):e493–503. doi: 10.1016/S1470-2045(14)70263-3
46. Brand S. Crohn’s disease: Th1, Th17 or both? The change of a paradigm: new immunological and genetic insights implicate Th17 cells in the pathogenesis of Crohn’s disease. Gut (2009) 58(8):1152–67. doi: 10.1136/gut.2008.163667
47. Ma K, Yang L, Shen R, Kong B, Chen W, Liang J, et al. Th17 cells regulate the production of CXCL1 in breast cancer. Int Immunopharmacol (2018) 56:320–9. doi: 10.1016/j.intimp.2018.01.026
48. Asiedu MK, Ingle JN, Behrens MD, Radisky DC, Knutson KL. TGFbeta/TNF(alpha)-mediated epithelial-mesenchymal transition generates breast cancer stem cells with a claudin-low phenotype. Cancer Res (2011) 71(13):4707–19. doi: 10.1158/0008-5472.CAN-10-4554
49. Cohen EN, Gao H, Anfossi S, Mego M, Reddy NG, Debeb B, et al. Inflammation mediated metastasis: immune induced epithelial-to-mesenchymal transition in inflammatory breast cancer cells. PloS One (2015) 10(7):e0132710. doi: 10.1371/journal.pone.0132710
50. Wlodarczyk M, Sobolewska-Wlodarczyk A, Cygankiewicz AI, Jacenik D, Piechota-Polanczyk A, Stec-Michalska K, et al. G protein-coupled receptor 30 (GPR30) expression pattern in inflammatory bowel disease patients suggests its key role in the inflammatory process. A Preliminary Study J Gastrointestin Liver Dis (2017) 26(1):29–35. doi: 10.15403/jgld.2014.1121.261.gpr
51. Filardo EJ. A role for G-protein coupled estrogen receptor (GPER) in estrogen-induced carcinogenesis: Dysregulated glandular homeostasis, survival and metastasis. J Steroid Biochem Mol Biol (2018) 176:38–48. doi: 10.1016/j.jsbmb.2017.05.005
52. Jacenik D, Beswick EJ, Krajewska WM, Prossnitz ER. G protein-coupled estrogen receptor in colon function, immune regulation and carcinogenesis. World J Gastroenterol (2019) 25(30):4092–104. doi: 10.3748/wjg.v25.i30.4092
53. Prossnitz ER, Barton M. The G protein-coupled oestrogen receptor GPER in health and disease: an update. Nat Rev Endocrinol (2023) 19(7):407–24. doi: 10.1038/s41574-023-00822-7
54. Glassner KL, Abraham BP, Quigley EMM. The microbiome and inflammatory bowel disease. J Allergy Clin Immunol (2020) 145(1):16–27. doi: 10.1016/j.jaci.2019.11.003
55. Kwa M, Plottel CS, Blaser MJ, Adams S. The intestinal microbiome and estrogen receptor-positive female breast cancer. J Natl Cancer Inst (2016) 108(8):djw029. doi: 10.1093/jnci/djw029
56. Buchta Rosean C, Bostic RR, Ferey JCM, Feng TY, Azar FN, Tung KS, et al. Preexisting commensal dysbiosis is a host-intrinsic regulator of tissue inflammation and tumor cell dissemination in hormone receptor-positive breast cancer. Cancer Res (2019) 79(14):3662–75. doi: 10.1158/0008-5472.CAN-18-3464
57. Zhang J, Xia Y, Sun J. Breast and gut microbiome in health and cancer. Genes Dis (2021) 8(5):581–9. doi: 10.1016/j.gendis.2020.08.002
58. Hauser W, Janke KH, Klump B, Hinz A. Anxiety and depression in patients with inflammatory bowel disease: comparisons with chronic liver disease patients and the general population. Inflammation Bowel Dis (2011) 17(2):621–32. doi: 10.1002/ibd.21346
59. Labanski A, Langhorst J, Engler H, Elsenbruch S. Stress and the brain-gut axis in functional and chronic-inflammatory gastrointestinal diseases: A transdisciplinary challenge. Psychoneuroendocrinology (2020) 111:104501. doi: 10.1016/j.psyneuen.2019.104501
60. Ge L, Liu S, Li S, Yang J, Hu G, Xu C, et al. Psychological stress in inflammatory bowel disease: Psychoneuroimmunological insights into bidirectional gut-brain communications. Front Immunol (2022) 13:1016578. doi: 10.3389/fimmu.2022.1016578
Keywords: Crohn’s disease, breast cancer, Mendelian randomization, causal association, risk
Citation: Yu C, Xu J, Xu S, Huang Y, Tang L, Zeng X, Yu T, Chen W and Sun Z (2024) Appraising the causal association between Crohn’s disease and breast cancer: a Mendelian randomization study. Front. Oncol. 13:1275913. doi: 10.3389/fonc.2023.1275913
Received: 22 August 2023; Accepted: 27 December 2023;
Published: 09 February 2024.
Edited by:
Xuanye Cao, University of Texas MD Anderson Cancer Center, United StatesReviewed by:
Kimberly Dieudonnee van der Willik, Ikazia Ziekenhuisn Rotterdam, NetherlandsCopyright © 2024 Yu, Xu, Xu, Huang, Tang, Zeng, Yu, Chen and Sun. This is an open-access article distributed under the terms of the Creative Commons Attribution License (CC BY). The use, distribution or reproduction in other forums is permitted, provided the original author(s) and the copyright owner(s) are credited and that the original publication in this journal is cited, in accordance with accepted academic practice. No use, distribution or reproduction is permitted which does not comply with these terms.
*Correspondence: Zhengkui Sun, c3VuemhlbmdrdWlAc29odS5jb20=
†These authors have contributed equally to this work
Disclaimer: All claims expressed in this article are solely those of the authors and do not necessarily represent those of their affiliated organizations, or those of the publisher, the editors and the reviewers. Any product that may be evaluated in this article or claim that may be made by its manufacturer is not guaranteed or endorsed by the publisher.
Research integrity at Frontiers
Learn more about the work of our research integrity team to safeguard the quality of each article we publish.