- 1Division of Hematology and Oncology, Department of Medicine, University of Washington, Seattle, WA, United States
- 2Clinical Research Division, Fred Hutchinson Cancer Center, Seattle, WA, United States
- 3Division of Myeloma, Department of Hematology & Hematopoietic Cell Transplantation, City of Hope, CA, United States
Traditional prognostic models for newly diagnosed patients with multiple myeloma (MM), including International Staging System criteria and number of high-risk chromosomal abnormalities, are based on disease characteristics at diagnosis. However, the identification of patients at risk of more rapidly progressive MM is inherently a dynamic assessment. In a subset of patients with MM, adverse disease biology only becomes evident after the failure of first-line therapy. We define this entity as functional high-risk MM (FHRMM), encompassing relapse within 18 months of treatment initiation and/or within 12 months of frontline autologous stem cell transplantation. FHRMM is not adequately captured by traditional prognostic models, and there is a need for better understanding of mechanisms or risk factors for early relapse or progression. In this review, we explore potential definitions of FHRMM before delving into its underlying drivers based on genetic, transcriptomic, and immune cell profiling studies. Emerging data suggest that specific features of both myeloma cells and immune cells can enable the FHRMM phenotype. We conclude our review by discussing ongoing and future studies that seek to identify and intervene upon patients with FHRMM preemptively.
Introduction
Multiple myeloma (MM), a malignant neoplasm of plasma cells, is marked by considerable heterogeneity in outcomes after diagnosis and initiation of frontline therapy. With Revised International Staging System (R-ISS) criteria, 5-year rates of progression-free survival (PFS) for newly diagnosed patients range from 55% to 36% to 24% depending on staging parameters at diagnosis (1). Similarly, for patients with MM receiving modern induction therapy with four-drug regimens, the presence of high-risk cytogenetics at diagnosis modulates the degree of benefit that patients receive from the addition of novel agents (2–4). The presence of 2 or more high risk chromosomal features at diagnosis appears to confer a particularly negative prognosis, even in the era of quadruplet induction followed by autologous stem cell transplantation (ASCT) (2, 5). Other poor prognostic markers detectable at diagnosis include primary plasma cell leukemia, anaplastic morphology, and soft-tissue extramedullary disease (EMD) (6–8).
In contrast, functional high-risk multiple myeloma (FHRMM) is only defined through dynamic assessment of disease kinetics after treatment initiation. At its broadest level, FHRMM could potentially include both patients with primary refractory MM as well as patients with early relapse following first-line therapy. In this Review, we focus on the second definition of FHRMM with an emphasis on early relapse. Although the definition of early relapse varies between studies as shown in Table 1 (9–21), we define FHRMM as progressive disease (PD) within 18 months of treatment initiation and/or PD within 12 months of frontline ASCT (10, 11, 13, 16–21). As illustrated in Figure 1, these studies have uniformly shown that FHRMM status following frontline therapy is associated with worsened overall survival (OS) thereafter (9–21).
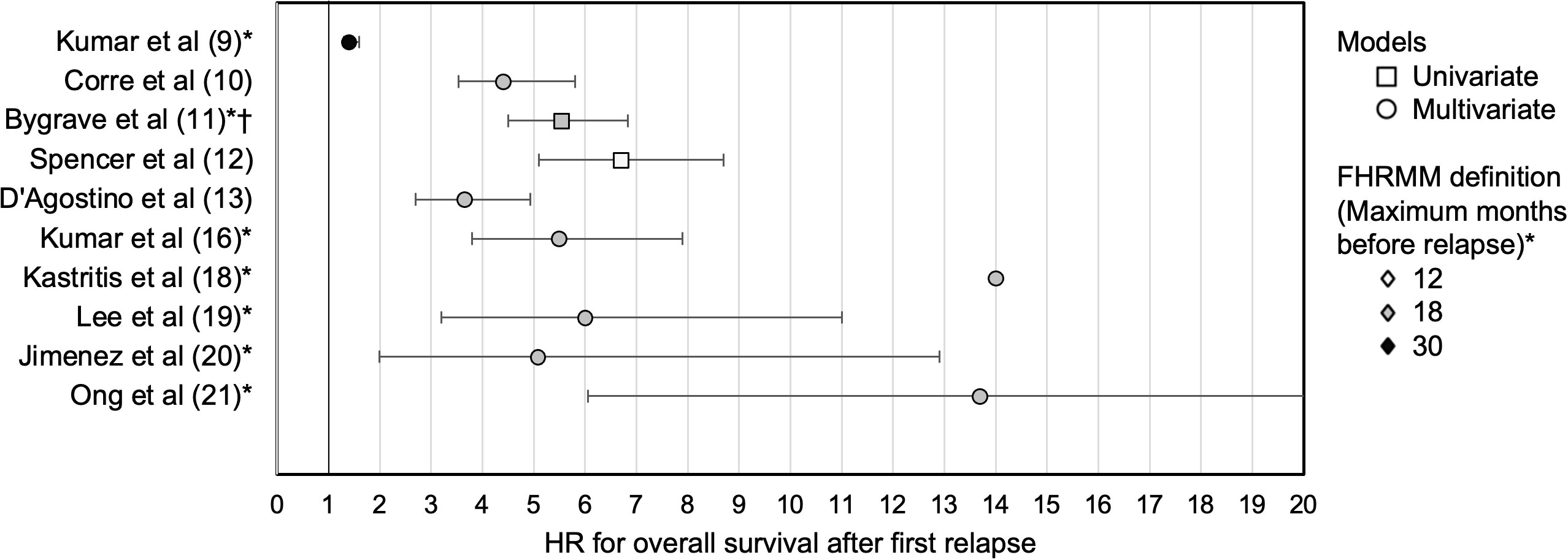
Figure 1 Studies of FHRMM and hazard ratios for overall survival. For studies from Table 1, HR with 95% CIs are depicted for overall survival following first relapse for patients with FHRMM versus patients with later relapses. Studies where HR and 95% CI were not reported are not shown. * 6 months added to PD criteria for FHRMM if defined from ASCT rather than start of therapy. † RR reported. ASCT, autologous stem cell transplant; CI, confidence interval; FHRMM, functional high-risk multiple myeloma; HR, hazard ratio; PD, progressive disease; RR, relative risk.
Given these findings, multiple groups have attempted to identify clinical risk factors that can predict FHRMM status following frontline therapy (22–31). Potential risk factors from these studies include R-ISS staging (or specific components thereof), performance status, specific myeloma-defining events, suboptimal induction regimens, or suboptimal depths of response. However, these scoring systems vary widely in terms of FHRMM definitions, relevant covariates, and analytic performance. One such calculator from the GIMEMA group, the Simplified Score to Predict Early Relapse in Multiple Myeloma (S-ERMM), has shown conflicting results in different datasets (23, 27, 29). As such, better tools to define patients at high risk of early relapse are needed. In this Review, we explore emerging evidence from genomic, transcriptomic, and immune cell profiling studies to identify these patients. Pre-clinical and translational studies suggest that both myeloma cells and immune cells play large roles in enabling early relapse. Our review of the multi-omic landscape of FHRMM highlights the need for both more precise prognostic models to capture the full spectrum of risk in MM as well as future studies that intervene on FHRMM biology before clinical relapse occurs.
Myeloma cell biology in functional high-risk myeloma
Gene expression profiling (GEP) assays, which look at the expression of targeted genes by levels of messenger RNA (mRNA) expression within MM cells, may be able to predict FHRMM. Two such GEP tools, the GEP70/UAMS70 assay (marketed as MyPRS) and the EMC92 assay (marketed as SKY92), are commercially available in the United States (US). In one study of 94 patients using the MyPRS assay, rates of relapse within 12 months of induction were 28% in patients with high-risk MyPRS scores versus only 2% in patients with low-risk MyPRS scores (32). Interestingly, early relapse occurred in 30% of patients with high-risk MyPRS scores and low-risk conventional cytogenetics; in contrast, no patients with low-risk MyPRS scores and high-risk cytogenetics had early relapse. In a secondary analysis of the Myeloma XI trial, early relapse occurred in over a third of patients with high-risk SKY92 scores regardless of whether they received post-transplant lenalidomide or not (33). Another 17-gene panel, the REL-17 signature, has been validated to predict patients with >60% probability of relapsing within 12 months of ASCT (17). However, it is worth noting that the routine use of these tests in the US has historically been limited by issues around insurance reimbursement (34, 35).
In addition to these multi-gene panels, specific genes associated with cell growth or tumor suppression are often implicated in the FHRMM phenotype. Several groups have sought to identify culpable genes or gene families using GEP as well as more comprehensive genomic sequencing. For example, a recent analysis of genomic data from the Multiple Myeloma Research Foundation CoMMpass dataset demonstrated that genes involved in the IL-6/JAK/STAT3 signaling pathway are associated with FHRMM (36). Genes associated with glycolysis, hypoxia tolerance, and oxidative stress are enriched in FHRMM as well (36–38). Unsurprisingly, DNA damage repair pathways including TP53 (whether mutated or lost) are disrupted in patients with FHRMM at higher proportions as compared to patients with relapse occurring at later timeframes (13, 37, 38). In one study comparing FHRMM patients at first relapse versus heavily pre-treated non-FHRMM patients (with a median of 5 prior lines of therapy), biallelic inactivation of TP53 or RB1 was more common (44% versus 30%) in the FHRMM subgroup than the heavily pre-treated subgroup (38).
Beyond genes focused on cell growth or tumor suppression, a few miscellaneous genes additionally warrant discussion. Mutations in genes involved with epithelial-mesenchymal transitions may predispose patients to early relapse: for example, integrin-ɑ8 has been found to be enriched in FHRMM and drives MM cell proliferation and invasion (38, 39). The noncoding RNA transcript MALAT1, which may similarly be involved in this process, is found at higher levels among patients with early relapse (40). Other noncoding mRNA transcripts, for example miR-181a which is found on chromosome 1q, have also been implicated in this setting (41). High levels of miR-193a-5p circulating in peripheral blood are conversely associated with lower rates of early relapse, although this may be a function of this particular transcript’s association with proteasome inhibitor sensitivity (42). Some of these findings may have relevance to conventional markers of disease aggressiveness, for example mesenchymal transition as a driver of EMD. However, further research is needed to validate these associations.
Immune cell biology in functional high-risk myeloma
The tumor immune microenvironment (TME) plays a key role in MM pathogenesis and is also important in mediating the risk of relapse after effective therapy (43). Recent analyses have demonstrated that, with time and exposure to different MM-directed therapies, the TME evolves just as MM cells themselves evolve. Specifically, in a study of 39 patients including newly diagnosed patients and triple-class-refractory patients, TME analyses using mass cytometry, cytokines, and RNA sequencing of bone marrow samples demonstrate that the TME becomes less functional over time: in particular, increasing numbers of senescent T cells and fewer early-memory T cells are detected during later lines of therapy (44). As detailed below, several studies have examined immune cell subsets in similar detail with an emphasis on early relapse after induction therapy or after ASCT. These types of analyses can help us better understand TME-based drivers of FHRMM that are not reliably captured by traditional prognostic models.
Most research to date with regard to immune cell profiling in MM has focused on T-cell types and phenotypes. For example, in one study of bone marrow aspirates from newly diagnosed patients with MM, PFS was almost three times worse in the subset of patients with regulatory T-cell (Treg) frequencies above the median (45). These patients also had higher levels of PD-1 and LAG-3 expression (markers of T-cell exhaustion) as well. Specific to FHRMM, an analysis of marrow-infiltrating T-cells at Day +100 after ASCT identified a distinct subgroup with shorter PFS; this subgroup had increased Treg cells and greater levels of regulatory markers such as ICOS, PD-1, and LAG-3 on effector cells (46). Moving beyond Treg cells, a xenograft model of ASCT has found that inhibitory receptor expression on CD8+ T cells as well as downregulation of the costimulatory receptor CD226 (DNAM-1) were also associated with MM progression (47). In this study, these DNAM-1 negative CD8+ T cells had an exhausted phenotype with increased TIGIT and PD-1 expression. In an analysis of post-ASCT lymphocyte composition and function involving 55 patients, patients who ultimately relapsed after ASCT had greater numbers of exhausted CD8+ T cells (with upregulation of CD57 and PD-1) detectable even prior to clinical detection of PD (48). One study of 58 MM patients identified distinct TME patterns after ASCT via cytometry with time of flight (CyTOF) analyses and found that those with increased levels of naïve and terminally differentiated T cells had comparatively worse outcomes regardless of the presence of high-risk chromosomal abnormalities (49).
The contribution of B cells to the FHRMM phenotype is less well defined. While regulatory B-cells (Breg) are found at higher concentrations within the bone marrow of newly diagnosed MM patients as compared to patients in remission or healthy volunteers, Breg cells are typically eradicated by therapy and remain undetectable at relapse (50, 51). Similarly, B-cell subset analyses did not play a significant role in identifying unique TME clusters that predict good versus poor responses to therapy (44). This general lack of impact may be related to global B-cell suppression and dysfunction that occurs early in MM pathogenesis in most cases, even for patients without clear immunoparesis (44, 52). Alternatively, because low Breg frequencies are associated with worsened OS but not worsened PFS in elderly patients with MM, B cell subset analyses may be more pertinent to other competing disease processes (e.g., infectious complications) than they are to the kinetics of MM progression (53, 54).
In contrast to B cells, natural killer (NK) lymphocytes clearly play important roles in the immune response against MM (55–57). As such, it is unsurprising that the dynamics of NK cell reconstitution following transplantation has been associated with outcomes in MM. In a study of 114 patients with MM, patients with low NK cell counts in peripheral blood (<100 cells per microliter) 1 month post-ASCT had worsened PFS compared to patients with NK cell counts of 100-200 per microliter (58). Similarly, an analysis of adaptive NK cells (long-lived NK cells with properties of immunologic memory against viruses such as cytomegalovirus) showed that greater absolute numbers of adaptive NKs was associated with a lower risk of relapse following ASCT (59). In brief, lower numbers of NK cells during post-ASCT immune reconstitution may be associated with greater risk of relapse. Conversely, higher levels of NK cells three months after ASCT is associated with higher rates of measurable residual disease (MRD) negativity (60). In addition to quantitative NK cell frequencies, NK cell phenotypes may also play a role as well: in one study, patients with higher levels of terminally differentiated NK cells after ASCT had worsened PFS than those with more immature NK cells (56).
Non-lymphoid immune cells may also enable or protect against FHRMM in certain cases, although conclusions are difficult to draw given the complex web of myeloid-lineage cellular interactions in the tumor microenvironment. For example, in a detailed analysis of patient marrow samples following quadruplet induction, MRD negativity was associated with higher levels of monocytes as well as plasmacytoid dendritic cells within the bone marrow (61). In contrast, high levels of circulating monocytes have been a poor prognostic feature in several studies (62, 63). The types of monocytes within the blood and marrow may matter as much as their quantities, given that non-classical monocytes (CD16+CD14dim) may drive myeloma cell growth and increased tumor burden (64, 65). In contrast, classical monocytes (CD14+CD16-) may have the opposite effect (66, 67). Differentiated monocytes show a similar range of behaviors depending on their phenotype, as evidenced by the presence of M2-polarized tumor-associated macrophages (associated with immunosuppression) as a marker of earlier relapses (68, 69). However, even pro-inflammatory M1-polarized macrophages may lead to downstream promotion of stem-like MM progenitor cells and bortezomib resistance through complex cytokine signaling pathways (70). Evidently, more studies of monocyte-lineage cells and their overlapping impacts on the FHRMM phenotype are required.
Finally, serum cytokine levels may also play a role in risk stratification for FHRMM. In an analysis of 188 Chinese patients with newly diagnosed MM, baseline interleukin (IL)-10 levels over 169.96 pg/ml were associated with inferior PFS and OS (71). In a retrospective study, serum IL-2, IL-4, IL-6, IL-10, IL-17a, TNF-alpha, and IFN-gamma levels were markedly elevated in newly diagnosed MM patients compared to healthy controls; in a multivariate analysis, IL-6 and IL-17a were prognostic factors for survival (72). Soluble IL-2 receptor (sIL-2r), which normally stimulates lymphocyte proliferation after binding IL-2, is generally released from lymphocytes via cleavage of the alpha chain (CD25) and is increased in several malignancies (73). sIL-2r competes for binding of IL-2 with the IL-2 receptor and thus may play a role in blocking anti-tumor immune based activities. In an analysis of 88 patients with newly diagnosed MM, sIL-2r levels were an independent risk factor for PFS (74).
Taken together, several studies have shown an important role for immune cells within the TME with respect to treatment failure and progression. However, these TME characteristics are not reliably captured by our current risk-stratification systems for MM. Most studies have focused on Treg frequencies or markers of T-cell exhaustion such as PD-1 or TIGIT, but NK cell properties in the post-ASCT setting impact the risk of early relapse as well. Finally, levels of specific serum cytokines, initially altered in newly diagnosed MM compared to healthy controls, may also be prognostic for survival. The interplay between adverse features of immune cells and myeloma cells remains unclear: is T-cell exhaustion an independent phenomenon in patients with FHRMM, or is it being driven by biological features of MM cells that remain incompletely characterized? Regardless, incorporating these TME factors as novel biomarkers for early progression may help better refine prognostic models and address the unmet need around FHRMM identification.
Mitigating the risk of functional high-risk myeloma
Strategies to identify a distinct FHRMM phenotype through multi-omic analyses of both myeloma cells and immune cells are only a first step; ideally, patients should also be managed differently once FHRMM is recognized clinically. Such FHRMM-specific management should emphasize not just more aggressive therapy, but rather more personalized therapy tailored to FHRMM’s unique disease and immune biology as summarized in this review. In Table 2, we outline four potential strategies to intervene on these patient populations both before and after their diagnosis of FHRMM, spanning from improved patient counseling to enhanced surveillance to altered therapeutic strategies. Given the paucity of published evidence surrounding the drivers of FHRMM, robust trials are still needed before these therapeutic strategies can be widely adopted.
Firstly, for patients at higher risk of FHRMM based on the factors identified in Figure 2, can we modify their treatment approach a priori during frontline therapy? Many trials have already employed multi-drug maintenance or consolidation therapy for patients with cytogenetically defined high-risk disease at diagnosis (76, 77). More recently, the MUKnine OPTIMUM trial included a high-risk SKY92 GEP profile alongside ultra-high-risk cytogenetics or plasma cell leukemia as eligibility criteria for an intensive induction and extended consolidation regimen (78). With regard to FHRMM, retrospective studies have identified the use of multi-drug maintenance or post-ASCT consolidation as protective factors against early relapse (13, 18). Given that GEP assays are not always available or reimbursable, at least three risk calculators (S-ERMM, CIBMTR, and EBMT) based on easily obtainable baseline parameters may be able to guide FHRMM-oriented decision making in future trials (22–31). Conversely, to the extent that patients who achieve deep remissions or MRD negativity during first-line therapy are less likely to experience rapid relapses thereafter, predictive calculators to identify these patients may also identify patients who will be at low risk of FHRMM (79, 80). As of now, we suggest that these calculators be used to guide further prospective studies before they are routinely deployed in clinical care to guide the escalation or de-escalation of frontline treatment.
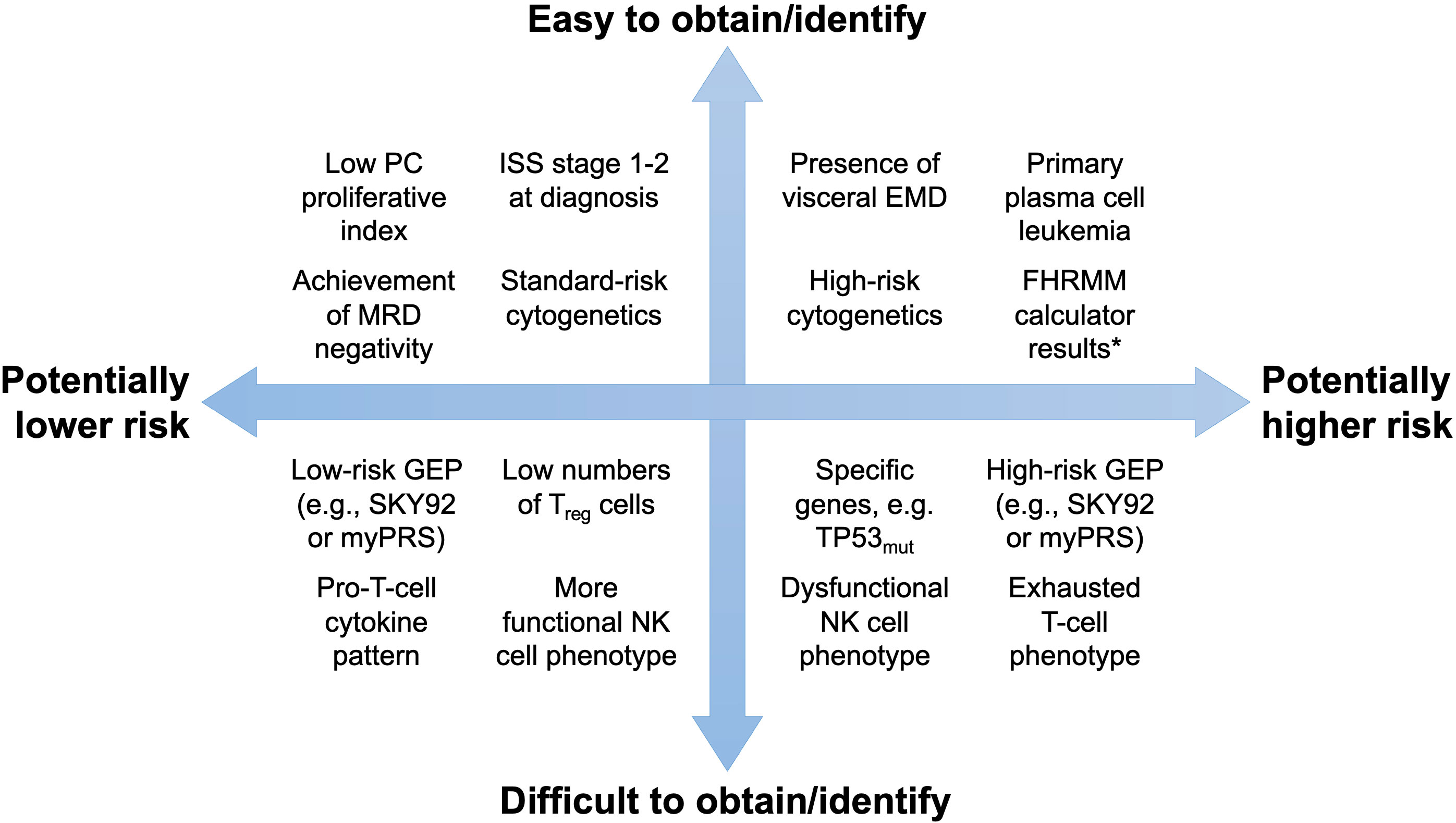
Figure 2 Potential tools to predict the FHRMM phenotype. Tests and tools are organized by their ability to predict the FHRMM phenotype (x-axis) as well as their ability to be obtained or identified in routine clinical practice (y-axis). * For example, the S-ERMM, EBMT, and CIBMTR calculators summarized in this review. EMD, extramedullary disease; FHRMM, functional high-risk multiple myeloma; GEP, gene expression profiling; ISS, International Staging System; MRD, measurable residual disease; NK, natural killer; PC, plasma cell; TP53mut, mutated TP53 gene.
Secondly, for patients who are suspected of having an FHRMM phenotype, should we screen these patients more aggressively for relapse during the maintenance phase of therapy? Enhanced surveillance with more frequent cross-sectional imaging or bone marrow biopsies may theoretically lead to relapse detection earlier, although the clinical benefit of this has not yet been established. Emerging serum biomarkers may be able to predict PD months before it occurs clinically, for example rising levels of proteins such as DKK1 and sclerostin involved in pathologic bone remodeling (81). Increasing levels of soluble B-cell maturation antigen, a known biomarker of plasma cell burden, may be able to identify impending relapse as well (82). Finally, several groups have investigated the implications of increasing levels of MRD burden on sequential bone marrow biopsies as a harbinger of relapse (83–85). For patients suspected to have an FHRMM phenotype and a correspondingly higher risk of relapse in any given time period, longitudinal assessments of these emerging biomarkers may be justified and even cost-effective.
Thirdly, should we change our approach to next-line therapies once early relapse has occurred? Several studies have found that subsequent PFS and OS are poor in FHRMM (9–21). On a simple level, more drugs in combination are superior when it comes to partially mitigating this risk of poor outcomes in FHRMM. For example, secondary analyses of the randomized ASPIRE and IKEMA trials demonstrate that triplets outperform doublets with regard to PFS among patients with FHRMM at study enrollment (86, 87). In this vein, the ongoing multi-arm MyDrug study conducted by the Multiple Myeloma Research Foundation (clinicaltrials.gov ID: NCT03732703) utilizes mutational analysis with targeted therapies in multi-drug regimens specifically for patients with FHRMM (75). Similarly, the Australasian Myeloma Research Consortium is running the IBIS trial of isatuximab and dexamethasone alongside the cereblon E3 ligase modulator (CELMoD) ibderdomide in patients with FHRMM as well (88). We eagerly await the results of these and other investigations in this space.
Alternatively, given the contribution of immune cell dysfunction within the TME as described above, adoptive cellular immunotherapy may play a particularly important role in managing FHRMM. Idecabtagene vicleucel and ciltacabtagene autoleucel, two chimeric antigen receptor T-cell (CAR-T) therapies currently approved in the US for patients who have received 4+ prior lines of therapy, have been studied as second-line therapy in FHRMM through the KarMMa-2A and CARTITUDE-2B trials, respectively (89, 90). In both single-arm cohorts, these CAR-T therapies behaved comparably to the corresponding KarMMa and CARTITUDE-1 trials involving more heavily pre-treated patients (91, 92). This suggests that CAR-T therapy may be a valuable option for selected patients with FHRMM as second-line therapy. Even allogeneic stem cell transplantation, a modality rarely used in the modern era, may have a role in selected patients with FHRMM (93).
Finally, can precision medicine be used some day to target specific pathways implicated in FHRMM? For example, the aforementioned JAK/STAT3 pathway has been shown to be activated preferentially in cells from patients with FHRMM (36, 37). Ruxolitinib, a known inhibitor of the JAK/STAT3 pathway approved for myelofibrosis, has been shown in a Phase 1 trial to lead to responses in approximately a third of patients with relapsed/refractory MM (94). Interestingly, preclinical studies suggest that ruxolitinib may also lower PD-L1 expression on MM cells and interfere with other pro-growth pathways (95, 96). For patients with FHRMM in the setting of mutations in genes such as RAF or the CDK family, the ongoing MyDrug study is investigating the addition of targeted therapies in addition to anti-MM regimens (75). In patients with slow NK cell reconstitution after ASCT, autologous NK cell infusions (after ex vivo expansion) or NK-specific cytokine agonists may be therapeutic options as well (97, 98). With regard to reducing T cell dysfunction, pre-clinical models suggest that TIGIT inhibition on T cells may work to prevent relapse (47, 99, 100). At least one clinical trial (clinicaltrials.gov ID NCT05289492) of TIGIT inhibition in relapsed MM is under way (101). As a caveat, checkpoint inhibition in MM has a mixed history given the premature closure of the KEYNOTE-183 and KEYNOTE-185 trials for excess risk (102, 103). As such, future strategies of checkpoint inhibitors in FHRMM should ideally be paired with advanced diagnostic tools to better select patients who are more likely to benefit from these targeted approaches.
Discussion
In clinical practice, one of the most vexing characteristics of FHRMM is its unexpectedness. For patients with known risk factors such as high-risk cytogenetics, treatment is often tailored to their aggressive phenotype in terms of consideration of strategies such as tandem ASCT or dual PI-IMID maintenance. In contrast, FHRMM often occurs in patients without such risk factors. As shown in Table 3, approximately 20-40% of patients with FHRMM have ISS stage 1 disease (10, 11, 13, 14, 18, 19). Similarly, in several studies, over half of patients with FHRMM have standard-risk cytogenetics at diagnosis (10, 13, 18, 19, 104). These are patients for whom several years of remission after frontline therapy might have been expected, and early relapse is devastating in its own right but moreover because FHRMM confers a poor prognosis thereafter. Better identification of these patients a priori is an important first step toward trials of preemptive treatment intensification, novel therapeutic agents, or precision oncology approaches as outlined in this review.
Considering all the factors reviewed thus far, two overarching conclusions can be drawn about FHRMM and risk assessment in MM. First, assessing disease risk is a dynamic process that current prognostic models do not fully capture. Most of our prognostic models in plasma cell disorders, both in active MM and in precursor conditions such as smoldering myeloma, focus on disease parameters at diagnosis and not their kinetics over time. FHRMM is by definition a longitudinal diagnosis, and further studies of patients with FHRMM are required to create dynamic risk assessment systems accordingly. Second, given that we often change our treatment approaches for patients with traditionally defined high-risk MM, FHRMM arguably represents a shortcoming of our current risk models. As noted in Table 3, over half of patients who ultimately develop FHRMM are misclassified as having standard-risk disease biology at diagnosis based on the absence of selected cytogenetic abnormalities. Incorporation of novel biomarkers outlined into traditional staging models may help better stratify these patients to avoid undertreatment of patients at high risk of developing FHRMM.
Importantly, we intentionally defined FHRMM narrowly by focusing on early relapse after induction therapy and/or ASCT. However, if characterized more broadly to include any upstaging of risk based on disease kinetics over time, FHRMM could also include patients with primary refractory MM as well as patients with insufficient responses to induction therapy. Patients with primary refractory MM have poor outcomes even in the modern era of CD38-targeted monoclonal antibody incorporation into treatment regimens (106–109). Encouragingly, use of ASCT in the second line for these patients despite frontline refractoriness has recently been associated with improved outcomes (110). Failure to achieve at least a partial response with induction therapy has similarly been associated with worsened outcomes in several studies, although data do not generally support treatment intensification outside of planned ASCT when this occurs (111, 112). Whether the underlying disease and TME biology of these scenarios is similar to the pathophysiology of early relapse discussed in this review is unknown.
A key limitation of our review is its focus on genomic, transcriptomic, and immune drivers of FHRMM. Other diagnostic tools may play a key role in identifying FHRMM as well. For example, the plasma cell proliferative index, while not technically a genomic test per se, has been shown to predict early relapse among patients who do not achieve a complete response after ASCT (113). However, this test is not routinely available outside of select US institutions. Given the increasing sensitivity of peripheral blood flow cytometry assays, multiple groups have also demonstrated that circulating plasma cells are a predictor of early relapse whether detected at diagnosis or during stem cell collection (37, 114–116). Advanced imaging modalities such as whole-body diffusion-weighted magnetic resonance imaging may play a role in FHRMM prognostication as well, given that persistence of focal lesions after induction may be indicative of a worsened prognosis (117, 118). Finally, organoid-based models and ex vivo assays to assess drug sensitivity may also be helpful in determining the causes of treatment failure in earlier lines of therapy (119–122) Further research is needed to validate these tools and increase their availability for MM physicians across the globe.
In conclusion, FHRMM is a dynamically defined state of high risk defined by multiple factors. FHRMM is unequivocally associated with worsened OS after its emergence, but identification of these patients a priori remains a challenge with conventional risk prognostication systems. Both myeloma cell factors and immune cell factors have been implicated in the pathophysiology of early relapse. Larger multi-omic prospective data sets in newly diagnosed MM and early relapsed MM, building on some of the data presented in this review, will help refine prognostic models for these patients in coming years. Thankfully, studies of both diagnostic strategies to identify FHRMM as well as therapeutic strategies to mitigate these patients’ subsequently poor prognoses are under way.
Author contributions
RB and AC conceptualized the research idea. All authors contributed to the article and approved the submitted version.
Funding
The authors declare that no financial support was received for the research, authorship, and/or publication of this article.
Acknowledgments
The authors wish to acknowledge Meral Beksaç MD (Department of Hematology, Ankara University, Turkey) for unpublished context around risk stratification systems for early relapse in MM.
Conflict of interest
RB: Consultancy: BMS, Janssen, Pfizer, Genentech/Roche, Sanofi Pasteur, SparkCures; Research Funding: Pack Health. KC: Research funding: BMS. SL: Consultancy: Abbvie, Cellectar, Janssen; Research Funding: BMS. AC: Consultancy: BMS, Adaptive Biotechnologies; Research Funding: Adaptive Biotechnologies, Harpoon, Nektar, BMS, Janssen, Sanofi, Abbvie.
Publisher’s note
All claims expressed in this article are solely those of the authors and do not necessarily represent those of their affiliated organizations, or those of the publisher, the editors and the reviewers. Any product that may be evaluated in this article, or claim that may be made by its manufacturer, is not guaranteed or endorsed by the publisher.
References
1. Palumbo A, Avet-Loiseau H, Oliva S, Lokhorst HM, Goldschmidt H, Rosinol L, et al. Revised international staging system for multiple myeloma: A report from international myeloma working group. J Clin Oncol (2015) 33(26):2863–9. doi: 10.1200/JCO.2015.61.2267
2. Voorhees PM, Kaufman JL, Laubach JP, Sborov DW, Reeves B, Rodriguez C, et al. Daratumumab, lenalidomide, bortezomib, and dexamethasone for transplant-eligible newly diagnosed multiple myeloma: the GRIFFIN trial. Blood (2020) 136(8):936–45. doi: 10.1182/blood.2020005288
3. Moreau P, Attal M, Hulin C, Arnulf B, Belhadj-Merzoug K, Benboubker L, et al. Bortezomib, thalidomide, and dexamethasone with or without daratumumab before and after autologous stem-cell transplantation for newly diagnosed multiple myeloma (CASSIOPEIA): a randomised, open-label, phase 3 study. Lancet (2019) 394:29–38. doi: 10.1016/S0140-6736(19)31240-1
4. Goldschmidt H, Mai EK, Bertsch U, Fenk R, Nievergall E, Tichy D, et al. Addition of isatuximab to lenalidomide, bortezomib, and dexamethasone as induction therapy for newly diagnosed, transplantation-eligible patients with multiple myeloma (GMMG-HD7): part 1 of an open-label, multicentre, randomised, active-controlled, phase 3 trial. Lancet Haematol (2022) 9:e810–e21. doi: 10.1016/S2352-3026(22)00263-0
5. Costa LJ, Chhabra S, Medvedova E, Dholaria BR, Schmidt TM, Godby KN, et al. Daratumumab, carfilzomib, lenalidomide, and dexamethasone with minimal residual disease response-adapted therapy in newly diagnosed multiple myeloma. J Clin Oncol (2022) 40(25):2901–12. doi: 10.1200/JCO.21.01935
6. Nowakowski GS, Witzig TE, Dingli D, Tracz MJ, Gertz MA, Lacy MQ, et al. Circulating plasma cells detected by flow cytometry as a predictor of survival in 302 patients with newly diagnosed multiple myeloma. Blood (2005) 106(7):2276–9. doi: 10.1182/blood-2005-05-1858
7. Pour L, Sevcikova S, Greslikova H, Kupska R, Majkova P, Zahradova L, et al. Soft-tissue extramedullary multiple myeloma prognosis is significantly worse in comparison to bone-related extramedullary relapse. Haematologica (2014) 99(2):360–4. doi: 10.3324/haematol.2013.094409
8. Gagelmann N, Eikema DJ, Koster L, Netelenbos T, McDonald A, Stoppa AM, et al. Impact of newly diagnosed extramedullary myeloma on outcome after first autograft followed by maintenance: A CMWP-EBMT study. Eur J Haematol (2023) 111(2):181–90. doi: 10.1111/ejh.13981
9. Kumar SK, Dispenzieri A, Fraser R, Mingwei F, Akpek G, Cornell R, et al. Early relapse after autologous hematopoietic cell transplantation remains a poor prognostic factor in multiple myeloma but outcomes have improved over time. Leukemia (2018) 32(4):986–95. doi: 10.1038/leu.2017.331
10. Corre J, Montes L, Martin E, Perrot A, Cailot D, Leleu X, et al. Early relapse after autologous transplant for myeloma is associated with poor survival regardless of cytogenetic risk. Haematologica (2020) 105(9):e480–3. doi: 10.3324/haematol.2019.236588
11. Bygrave C, Pawlyn C, Davies F, Craig Z, Cairns D, Hockaday A, et al. Early relapse after high-dose melphalan autologous stem cell transplant predicts inferior survival and is associated with high disease burden and genetically high-risk disease in multiple myeloma. Br J Haematol (2021) 193(3):551–5. doi: 10.1111/bjh.16793
12. Spencer A, Mollee P, Blacklock HA, Harrison S, Quach H, Ho PJ, et al. Real-world outcome for newly diagnosed patients with functional high-risk myeloma - a myeloma and related diseases registry analysis. Blood (2019) 134(Supplement_1):269. doi: 10.1182/blood-2019-129431
13. D'Agostino M, Zaccaria GM, Ziccheddu B, Rustad EH, Genuardi E, Capra A, et al. Early relapse risk in patients with newly diagnosed multiple myeloma characterized by next-generation sequencing. Clin Cancer Res (2020) 26(18):4832–41. doi: 10.1158/1078-0432.CCR-20-0951
14. Helm-Petersen S, Sorrig R, Klausen TW, Preiss B, Frolund UC, Helleberg C, et al. Early relapsed disease of multiple myeloma following up-front HDM-ASCT: a study based on the Danish Multiple Myeloma Registry in the period 2005 to 2014. Leukemia (2018) 32(9):2054–7. doi: 10.1038/s41375-018-0057-5
15. Majithia N, Rajkumar SV, Lacy MQ, Buadi FK, Dispenzieri A, Gertz MA, et al. Early relapse following initial therapy for multiple myeloma predicts poor outcomes in the era of novel agents. Leukemia (2016) 30(11):2208–13. doi: 10.1038/leu.2016.147
16. Kumar S, Mahmood ST, Lacy MQ, Dispenzieri A, Hayman SR, Buadi FK, et al. Impact of early relapse after auto-SCT for multiple myeloma. Bone Marrow Transplant (2008) 42(6):413–20. doi: 10.1038/bmt.2008.180
17. Wu P, Walker BA, Broyl A, Kaiser M, Johnson DC, Kuiper R, et al. A gene expression based predictor for high risk myeloma treated with intensive therapy and autologous stem cell rescue. Leuk Lymphoma (2015) 56(3):594–601. doi: 10.3109/10428194.2014.911863
18. Kastritis E, Roussou M, Eleutherakis-Papaiakovou E, Gavriatopoulou M, Migkou M, Gika D, et al. Early relapse after autologous transplant is associated with very poor survival and identifies an ultra-high-risk group of patients with myeloma. Clin Lymphoma Myeloma Leuk (2020) 20(7):445–52. doi: 10.1016/j.clml.2019.10.014
19. Lee H, Duggan P, Chaudhry A, Neri P, Tay J, Rashid-Kolvear F, et al. Early relapse for multiple myeloma patients undergoing single autologous stem cell therapy: A single-center experience. Clin Lymphoma Myeloma Leuk (2018) 18(1):e69–75. doi: 10.1016/j.clml.2017.10.009
20. Jimenez-Zepeda VH, Reece DE, Trudel S, Chen C, Tiedemann R, Kukreti V. Early relapse after single auto-SCT for multiple myeloma is a major predictor of survival in the era of novel agents. Bone Marrow Transplant (2015) 50(2):204–8. doi: 10.1038/bmt.2014.237
21. Ong SY, de Mel S, Chen YX, Ooi MG, Surendran S, Lin A, et al. Early relapse post autologous transplant is a stronger predictor of survival compared with pretreatment patient factors in the novel agent era: analysis of the Singapore Multiple Myeloma Working Group. Bone Marrow Transplant (2016) 51(7):933–7. doi: 10.1038/bmt.2016.43
22. Zhou H, Jian Y, Du J, Liu J, Zhang Z, Yang G, et al. Prognostic nomogram for multiple myeloma early relapse after autologous stem cell transplant in the novel agent era. Cancer Med (2023) 12(8):9085–6. doi: 10.1002/cam4.5630
23. Zaccaria GM, Bertamini L, Petrucci MT, Offidani M, Corradini P, Capra A, et al. Development and validation of a simplified score to predict early relapse in newly diagnosed multiple myeloma in a pooled dataset of 2,190 patients. Clin Cancer Res (2021) 27(13):3695–703. doi: 10.1158/1078-0432.CCR-21-0134
24. Kubasch AS, Grieb N, Oeser A, Neumuth T, Yomade O, Hochhaus A, et al. Predicting early relapse for patients with multiple myeloma through machine learning. Blood (2021) 138(Supplement 1):2953–. doi: 10.1182/blood-2021-151195
25. Dhakal B, D'Souza A, Callander N, Chhabra S, Fraser R, Davila O, et al. Novel prognostic scoring system for autologous hematopoietic cell transplantation in multiple myeloma. Br J Haematol (2020) 191(3):442–52. doi: 10.1111/bjh.16987
26. Wei H, Sun Z, Ye X, Yu J, Ye Y, Wang Z. Establishment of a prediction model for disease progression within one year in newly diagnosed multiple myeloma patients. Hematology (2022) 27(1):575–82. doi: 10.1080/16078454.2022.2067940
27. Beksac M, Seval GC, Bozdag SC, Toprak SK, Yuksel MK, Topcuoglu P, et al. P19 - External validation of three existing early myeloma relapse scores (by EBMT, CIBMTR, and GIMEMA) in a single center shows major differences. Hemasphere (2023) 7(Suppl):20. doi: 10.1097/01.HS9.0000936204.00101.29
28. Beksac M, Iacobelli S, Koster L, Cornelissen J, Griskevicius L, Rabin NK, et al. An early post-transplant relapse prediction score in multiple myeloma: a large cohort study from the chronic Malignancies working party of EBMT. Bone Marrow Transplant (2023) 58(8):916–23. doi: 10.1038/s41409-023-01999-1
29. Slade M, Fiala M, Kelley S, Crees ZD, Schroeder MA, Stockerl-Goldstein K, et al. Evaluation of the simplified score to predict early relapse in multiple myeloma (S-ERMM) in the MMRF CoMMpass study. Leuk Res (2023) 127:107037. doi: 10.1016/j.leukres.2023.107037
30. Awada H, Hajj Ali A, Anwer F, Sauter C, Williams L, Khouri J. Validation of the CIBMTR scoring system in predicting early relapse of multiple myeloma after autologous hematopoietic cell transplant. Bone Marrow Transplant (2023) 58(9):1057–8. doi: 10.1038/s41409-023-02024-1
31. Manier S, Dimopoulos M, Hulin C, Leleu X, Delforge M, Weisel K, et al. Predictors of lenalidomide refractory relapse timing with newly diagnosed multiple myeloma: A FIRST trial subanalysis. Clin Lymphoma Myeloma Leuk (2023) 23(9):e297–306.e1. doi: 10.1016/j.clml.2023.06.002
32. Manasanch E, Berrios D, Fountain E, Claussen CM, Chaung T, Kaufman GP, et al. Gene expression profiling predicts relapse-free and overall survival in newly diagnosed myeloma patients treated with novel therapies. Br J Haematol (2021) 192(4):e115–e20. doi: 10.1111/bjh.17344
33. Shah V, Sherborne AL, Johnson DC, Ellis S, Price A, Chowdhury F, et al. Predicting ultrahigh risk multiple myeloma by molecular profiling: an analysis of newly diagnosed transplant eligible myeloma XI trial patients. Leukemia (2020) 34(11):3091–6. doi: 10.1038/s41375-020-0750-z
34. Davies FE, Pawlyn C, Usmani SZ, San-Miguel JF, Einsele H, Boyle EM, et al. Perspectives on the risk-stratified treatment of multiple myeloma. Blood Cancer Discovery (2022) 3(4):273–84. doi: 10.1158/2643-3230.BCD-21-0205
35. Hresko A, Haga SB. Insurance coverage policies for personalized medicine. J Pers Med (2012) 2(4):201–16. doi: 10.3390/jpm2040201
36. Soekojo CY, Chung TH, Furqan MS, Chng WJ. Genomic characterization of functional high-risk multiple myeloma patients. Blood Cancer J (2022) 12(1):24. doi: 10.1038/s41408-021-00576-3
37. Wang YT, Chu B, Zhou TG, Lu MQ, Shi L, Gao S, et al. Clinical and genomic characterization of Chinese patients with functional high-risk multiple myeloma: A real-world validation study. Front Oncol (2023) 13:1110693. doi: 10.3389/fonc.2023.1110693
38. Poos AM, Giesen N, Catalano C, Paramasivam N, Huebschmann D, John L, et al. Comprehensive comparison of early relapse and end-stage relapsed refractory multiple myeloma. Blood (2020) 136(Supplement 1):1–. doi: 10.1182/blood-2020-141611
39. Ryu J, Koh Y, Park H, Kim DY, Kim DC, Byun JM, et al. Highly expressed integrin-alpha8 induces epithelial to mesenchymal transition-like features in multiple myeloma with early relapse. Mol Cells (2016) 39(12):898–908. doi: 10.14348/molcells.2016.0210
40. Cho S, Chang YC, Chang C, Lin S, Liu Y, Hsiao H, et al. MALAT1 long non-coding RNA is overexpressed in multiple myeloma and may serve as a marker to predict disease progression. BMC Cancer (2014) 4:809. doi: 10.1186/1471-2407-14-809
41. Papadimitriou MA, Papanota AM, Adamopoulos PG, Pilala KM, Liacos CI, Malandrakis P, et al. miRNA-seq and clinical evaluation in multiple myeloma: miR-181a overexpression predicts short-term disease progression and poor post-treatment outcome. Br J Cancer (2022) 126(1):79–90. doi: 10.1038/s41416-021-01602-8
42. Park SS, Lim JY, Kim TW, Ko YH, Jeon WJ, Lee SY, et al. Predictive impact of circulating microRNA-193a-5p on early relapse after autologous stem cell transplantation in patients with multiple myeloma. Br J Haematol (2020) 189(3):518–23. doi: 10.1111/bjh.16413
43. Kawano Y, Moschetta M, Manier S, Glavey S, Görgün GT, Roccaro AM, et al. Targeting the bone marrow microenvironment in multiple myeloma. Immunol Rev (2015) 263(1):160–72. doi: 10.1111/imr.12233
44. Visram A, Dasari S, Anderson E, Kumar S, Kourelis TV. Relapsed multiple myeloma demonstrates distinct patterns of immune microenvironment and Malignant cell-mediated immunosuppression. Blood Cancer J (2021) 11(3):45. doi: 10.1038/s41408-021-00440-4
45. Alrasheed N, Lee L, Ghorani E, Henry JY, Conde L, Chin M, et al. Marrow-infiltrating regulatory T cells correlate with the presence of dysfunctional CD4+PD-1+ Cells and inferior survival in patients with newly diagnosed multiple myeloma. Clin Cancer Res (2020) 26(13):3443–54. doi: 10.1158/1078-0432.Ccr-19-1714
46. Lee L, Alrasheed N, Khandelwal G, Fitzsimons E, Richards H, Wilson W, et al. Increased immune-regulatory receptor expression on effector T cells as early indicators of relapse following autologous stem cell transplantation for multiple myeloma. Front Immunol (2021) 12:618610. doi: 10.3389/fimmu.2021.618610
47. Minnie SA, Kuns RD, Gartlan KH, Zhang P, Wilkinson AN, Samson L, et al. Myeloma escape after stem cell transplantation is a consequence of T-cell exhaustion and is prevented by TIGIT blockade. Blood (2018) 132(16):1675–88. doi: 10.1182/blood-2018-01-825240
48. Chung DJ, Pronschinske KB, Shyer JA, Sharma S, Leung S, Curran SA, et al. T-cell exhaustion in multiple myeloma relapse after autotransplant: optimal timing of immunotherapy. Cancer Immunol Res (2016) 4(1):61–71. doi: 10.1158/2326-6066.CIR-15-0055
49. Parmar H, Gertz M, Anderson EI, Kumar S, Kourelis TV. Microenvironment immune reconstitution patterns correlate with outcomes after autologous transplant in multiple myeloma. Blood Adv (2021) 5(7):1797–804. doi: 10.1182/bloodadvances.2020003857
50. Zhang L, Tai YT, Ho M, Xing L, Chauhan D, Gang A, et al. Regulatory B cell-myeloma cell interaction confers immunosuppression and promotes their survival in the bone marrow milieu. Blood Cancer J (2017) 7(3):e547. doi: 10.1038/bcj.2017.24
51. Bartosinska J, Purkot J, Karczmarczyk A, Chojnacki M, Zaleska J, Wlasiuk P, et al. Differential function of a novel population of the CD19+CD24hiCD38hi bregs in psoriasis and multiple myeloma. Cells (2021) 10(2):411. doi: 10.3390/cells10020411
52. Rawstron AC, Davies FE, Owen RG, English A, Pratt G, Child JA, et al. B-lymphocyte suppression in multiple myeloma is a reversible phenomenon specific to normal B-cell progenitors and plasma cell precursors. Br J Haematol (1998) 100(1):176–83. doi: 10.1046/j.1365-2141.1998.00525.x
53. Tang W, Li Y, Zou Z, Cui J, Wang F, Zheng Y, et al. A stratified therapeutic model incorporated with studies on regulatory B cells for elderly patients with newly diagnosed multiple myeloma. Cancer Med (2023) 12(3):3054–67. doi: 10.1002/cam4.5228
54. Wang C, Xu H, Gao R, Leng F, Huo F, Li Y, et al. CD19(+)CD24(hi)CD38(hi) regulatory B cells deficiency revealed severity and poor prognosis in patients with sepsis. BMC Immunol (2022) 23(1):54. doi: 10.1186/s12865-022-00528-x
55. Venglar O, Bago JR, Motais B, Hajek R, Jelinek T. Natural killer cells in the Malignant niche of multiple myeloma. Front Immunol (2021) 12:816499. doi: 10.3389/fimmu.2021.816499
56. Orrantia A, Terren I, Astarloa-Pando G, Gonzalez C, Uranga A, Mateos-Mazon JJ, et al. NK cell reconstitution after autologous hematopoietic stem cell transplantation: association between NK cell maturation stage and outcome in multiple myeloma. Front Immunol (2021) 12:748207. doi: 10.3389/fimmu.2021.748207
57. Fionda C, Stabile H, Molfetta R, Soriani A, Bernardini G, Zingoni A, et al. Translating the anti-myeloma activity of Natural Killer cells into clinical application. Cancer Treat Rev (2018) 70:255–64. doi: 10.1016/j.ctrv.2018.10.005
58. Rueff J, Medinger M, Heim D, Passweg J, Stern M. Lymphocyte subset recovery and outcome after autologous hematopoietic stem cell transplantation for plasma cell myeloma. Biol Blood Marrow Transplant (2014) 20(6):896–9. doi: 10.1016/j.bbmt.2014.03.007
59. Merino AM, Mehta RS, Luo X, Kim H, De For T, Janakiram M, et al. Early adaptive natural killer cell expansion is associated with decreased relapse after autologous transplantation for multiple myeloma. Transplant Cell Ther (2021) 27(4):310.e1–.e6. doi: 10.1016/j.jtct.2020.10.023
60. Keruakous AR, Asch A, Aljumaily R, Zhao D, Yuen C. Prognostic impact of natural killer cell recovery on minimal residual disease after autologous stem cell transplantation in multiple myeloma. Transpl Immunol (2022) 71:101544. doi: 10.1016/j.trim.2022.101544
61. Maura F, Boyle EM, Diamond B, Blaney P, Ghamlouch H, Ziccheddu B, et al. Genomic and immune signatures predict sustained MRD negativity in newly diagnosed multiple myeloma patients treated with daratumumab, carfilzomib, lenalidomide, and dexamethasone (D-KRd). Blood (2021) 138(Supplement 1):325. doi: 10.1182/blood-2021-149112
62. Shin SJ, Roh J, Kim M, Jung MJ, Koh YW, Park CS, et al. Prognostic significance of absolute lymphocyte count/absolute monocyte count ratio at diagnosis in patients with multiple myeloma. Korean J Pathol (2013) 47(6):526–33. doi: 10.4132/KoreanJPathol.2013.47.6.526
63. Dosani T, Covut F, Beck R, Driscoll JJ, de Lima M, Malek E. Significance of the absolute lymphocyte/monocyte ratio as a prognostic immune biomarker in newly diagnosed multiple myeloma. Blood Cancer J (2017) 7(6):e579. doi: 10.1038/bcj.2017.60
64. Sponaas AM, Moen SH, Liabakk NB, Feyzi E, Holien T, Kvam S, et al. The proportion of CD16(+)CD14(dim) monocytes increases with tumor cell load in bone marrow of patients with multiple myeloma. Immun Inflammation Dis (2015) 3(2):94–102. doi: 10.1002/iid3.53
65. Zahran AM, Nafady-Hego H, Moeen SM, Eltyb HA, Wahman MM, Nafady A. Higher proportion of non-classical and intermediate monocytes in newly diagnosed multiple myeloma patients in Egypt: A possible prognostic marker. Afr J Lab Med (2021) 10(1):129. doi: 10.4102/ajlm.v10i1.1296
66. Damasceno D, Almeida J, Teodosio C, Sanoja-Flores L, Mayado A, Perez-Pons A, et al. Monocyte subsets and serum inflammatory and bone-associated markers in monoclonal gammopathy of undetermined significance and multiple myeloma. Cancers (Basel) (2021) 13(6):1454. doi: 10.3390/cancers13061454
67. Coffey DG, Maura F, Gonzalez-Kozlova E, Diaz-Mejia JJ, Luo P, Zhang Y, et al. Immunophenotypic correlates of sustained MRD negativity in patients with multiple myeloma. Nat Commun (2023) 14(1):5335. doi: 10.1038/s41467-023-40966-8
68. Suyani E, Sucak GT, Akyurek N, Sahin S, Baysal NA, Yagci M, et al. Tumor-associated macrophages as a prognostic parameter in multiple myeloma. Ann Hematol (2013) 92(5):669–77. doi: 10.1007/s00277-012-1652-6
69. Sun J, Park C, Guenthner N, Gurley S, Zhang L, Lubben B, et al. Tumor-associated macrophages in multiple myeloma: advances in biology and therapy. J Immunother Cancer (2022) 10(4):e003975. doi: 10.1136/jitc-2021-003975
70. Beyar-Katz O, Magidey K, Reiner-Benaim A, Barak N, Avivi I, Cohen Y, et al. Proinflammatory macrophages promote multiple myeloma resistance to bortezomib therapy. Mol Cancer Res (2019) 17(11):2331–40. doi: 10.1158/1541-7786.MCR-19-0487
71. Wang H, Wang L, Chi PD, Wang WD, Chen XQ, Geng QR, et al. High level of interleukin-10 in serum predicts poor prognosis in multiple myeloma. Br J Cancer (2016) 114(4):463–8. doi: 10.1038/bjc.2016.11
72. Gu J, Huang X, Zhang Y, Bao C, Zhou Z, Jin J. Cytokine profiles in patients with newly diagnosed multiple myeloma: Survival is associated with IL-6 and IL-17A levels. Cytokine (2021) 138:155358. doi: 10.1016/j.cyto.2020.155358
73. Bien E, Balcerska A. Serum soluble interleukin 2 receptor alpha in human cancer of adults and children: a review. Biomarkers (2008) 13(1):1–26. doi: 10.1080/13547500701674063
74. Wang L, Wang J-h, Liu W-j, Wang W-d, Wang H, Chen X-q, et al. High level of soluble interleukin-2 receptor in serum predicts treatment resistance and poor progression-free survival in multiple myeloma. Ann Hematol (2017) 96(12):2079–88. doi: 10.1007/s00277-017-3125-4
75. Kumar SK, Hofmeister CC, Biran N, Kaur G, Ye JC, Zonder JA, et al. The mydrug platform trial: efficacy and safety of daratumumab, ixazomib, pomalidomide, and dexamethasone in patients with functionally high-risk multiple myeloma. Blood (2022) 140(Supplement 1):4434–5. doi: 10.1182/blood-2022-163737
76. Nooka AK, Kaufman JL, Muppidi S, Langston A, Heffner LT, Gleason C, et al. Consolidation and maintenance therapy with lenalidomide, bortezomib and dexamethasone (RVD) in high-risk myeloma patients. Leukemia (2014) 28(3):690–3. doi: 10.1038/leu.2013.335
77. Gay F, Musto P, Rota-Scalabrini D, Bertamini L, Belotti A, Galli M, et al. Carfilzomib with cyclophosphamide and dexamethasone or lenalidomide and dexamethasone plus autologous transplantation or carfilzomib plus lenalidomide and dexamethasone, followed by maintenance with carfilzomib plus lenalidomide or lenalidomide alone for patients with newly diagnosed multiple myeloma (FORTE): a randomised, open-label, phase 2 trial. Lancet Oncol (2021) 22(12):1705–20. doi: 10.1016/S1470-2045(21)00535-0
78. Kaiser MF, Hall A, Walker K, Sherborne A, De Tute RM, Newnham N, et al. Daratumumab, cyclophosphamide, bortezomib, lenalidomide, and dexamethasone as induction and extended consolidation improves outcome in ultra-high-risk multiple myeloma. J Clin Oncol (2023) 41(23):3945–55. doi: 10.1200/JCO.22.02567
79. Guerrero C, Puig N, Cedena MT, Goicoechea I, Perez C, Garces JJ, et al. A machine learning model based on tumor and immune biomarkers to predict undetectable MRD and survival outcomes in multiple myeloma. Clin Cancer Res (2022) 28(12):2598–609. doi: 10.1158/1078-0432.CCR-21-3430
80. Maura F, Rajanna AR, Derkach A, Ziccheddu B, Weinhold N, Maclachlan KH, et al. Individualized treatment-adjusted risk stratification in newly diagnosed multiple myeloma. Blood (2022) 140(Supplement 1):1561–3. doi: 10.1182/blood-2022-160215
81. Mabille C, Ruyssen-Witrand A, Degboe Y, Gennero I, Loiseau HA, Roussel M, et al. DKK1 and sclerostin are early markers of relapse in multiple myeloma. Bone (2018) 113:114–7. doi: 10.1016/j.bone.2017.10.004
82. Shen Y, Liu J, Wang B, Zhang Y, Xu Y, Wang X, et al. Serum soluble BCMA can be used to monitor relapse of multiple myeloma patients after chimeric antigen receptor T-cell immunotherapy. Curr Res Transl Med (2023) 71(2):103378. doi: 10.1016/j.retram.2023.103378
83. Martinez-Lopez J, Wong SW, Shah N, Bahri N, Zhou K, Sheng Y, et al. Clinical value of measurable residual disease testing for assessing depth, duration, and direction of response in multiple myeloma. Blood Adv (2020) 4(14):3295–301. doi: 10.1182/bloodadvances.2020002037
84. Mohan M, Kendrick S, Szabo A, Yarlagadda N, Atwal D, Pandey Y, et al. Clinical implications of loss of bone marrow minimal residual disease negativity in multiple myeloma. Blood Adv (2022) 6(3):808–17. doi: 10.1182/bloodadvances.2021005822
85. Diamond B, Korde N, Lesokhin AM, Smith EL, Shah U, Mailankody S, et al. Dynamics of minimal residual disease in patients with multiple myeloma on continuous lenalidomide maintenance: a single-arm, single-centre, phase 2 trial. Lancet Haematol (2021) 8(6):e422–e32. doi: 10.1016/s2352-3026(21)00130-7
86. Mateos MV, Goldschmidt H, San-Miguel J, Mikhael J, DeCosta L, Zhou L, et al. Carfilzomib in relapsed or refractory multiple myeloma patients with early or late relapse following prior therapy: A subgroup analysis of the randomized phase 3 ASPIRE and ENDEAVOR trials. Hematol Oncol (2018) 36(2):463–70. doi: 10.1002/hon.2499
87. Facon T, Moreau P, Baker R, Pour L, Min C-K, Leleu X, et al. Isatuximab plus carfilzomib and dexamethasone in patients with early versus late relapsed multiple myeloma: ikema subgroup analysis. Blood (2022) 140(Supplement 1):1820–2. doi: 10.1182/blood-2022-159105
88. Australasian Myeloma Research Consortium. AMaRC 20-01 - STUDY. An immuno-therapeutic salvage strategy for 'functional' high-risk (FHR) multiple myeloma incorporating Iberdomide, Isatuximab and Dexamethasone (IBIS) (2023). Available at: https://www.amarconline.org/clinical-trials/ibis.
89. Usmani S, Patel K, Hari P, Berdeja J, Alsina M, Vij R, et al. KarMMa-2 cohort 2a: efficacy and safety of idecabtagene vicleucel in clinical high-risk multiple myeloma patients with early relapse after frontline autologous stem cell transplantation. Blood (2022) 140(Supplement 1):875–7. doi: 10.1182/blood-2022-162469
90. Van De Donk NWCJ, Agha M, Cohen AD, Cohen YC, Anguille S, Kerre T, et al. Ciltacabtagene autoleucel (Cilta-cel), a BCMA-directed CAR-T cell therapy, in patients with multiple myeloma (MM) and early relapse after initial therapy: CARTITUDE-2 cohort B 18-month follow-up. Blood (2022) 140(Supplement 1):7536–7. doi: 10.1182/blood-2022-159169
91. Munshi NC, Anderson LD Jr., Shah N, Madduri D, Berdeja J, Lonial S, et al. Idecabtagene vicleucel in relapsed and refractory multiple myeloma. N Engl J Med (2021) 384(8):705–16. doi: 10.1056/NEJMoa2024850
92. Lin Y, Martin TG, Usmani SZ, Berdeja JG, Jakubowiak AJ, Agha ME, et al. CARTITUDE-1 final results: Phase 1b/2 study of ciltacabtagene autoleucel in heavily pretreated patients with relapsed/refractory multiple myeloma. J Clin Oncol (2023) 41(Suppl_16):8009. doi: 10.1200/JCO.2023.41.16_suppl.8009
93. Randall K, Kaparou M, Xenou E, Paneesha S, Kishore B, Kanellopoulos A, et al. Reduced-intensity conditioning allogeneic transplantation after salvage treatment with DT-PACE in myeloma patients relapsing early after autologous transplant. Eur J Haematol (2017) 99(4):300–5. doi: 10.1111/ejh.12917
94. Berenson JR, Martinez D, Safaie T, Boccia R, Yang H, Moezi M, et al. Ruxolitinib and methylprednisolone for treatment of patients with relapsed/refractory multiple myeloma. Br J Haematol (2023) 200(6):722–30. doi: 10.1111/bjh.18593
95. Chen H, Li M, Ng N, Yu E, Bujarski S, Yin Z, et al. Ruxolitinib reverses checkpoint inhibition by reducing programmed cell death ligand-1 (PD-L1) expression and increases anti-tumour effects of T cells in multiple myeloma. Br J Haematol (2021) 192(3):568–76. doi: 10.1111/bjh.17282
96. Kusoglu A, Bagca B, Ay NPO, Saydam G, Avci CB. Ruxolitinib regulates the autophagy machinery in multiple myeloma cells. Anticancer Agents Med Chem (2020) 20(18):2316–23. doi: 10.2174/1871520620666200218105159
97. Nahi H, Chrobok M, Meinke S, Gran C, Marquardt N, Afram G, et al. Autologous NK cells as consolidation therapy following stem cell transplantation in multiple myeloma. Cell Rep Med (2022) 3(2):100508. doi: 10.1016/j.xcrm.2022.100508
98. Fernandez RA, Mayoral JE, Pierre-Louis L, Yao Y, Xu Y, Mu S, et al. Improving NK cell function in multiple myeloma with NKTR-255, a novel polymer-conjugated human IL-15. Blood Adv (2023) 7(1):9–19. doi: 10.1182/bloodadvances.2022007985
99. Minnie SA, Waltner OG, Ensbey KS, Olver SD, Collinge AD, Sester DP, et al. TIGIT inhibition and lenalidomide synergistically promote antimyeloma immune responses after stem cell transplantation in mice. J Clin Invest (2023) 133(4):e157907. doi: 10.1172/JCI157907
100. Guillerey C, Harjunpaa H, Carrie N, Kassem S, Teo T, Miles K, et al. TIGIT immune checkpoint blockade restores CD8+ T-cell immunity against multiple myeloma. Blood (2018) 132(16):1689–94. doi: 10.1182/blood-2018-01-825265
101. Moreau P, Holmberg L, Meuleman N, Graham P, De Henau O, Driessens G, et al. TIG-007: study of EOS884448/GSK4428859A alone, and in combination with iberdomide with or without dexamethasone, in participants with relapsed or refractory multiple myeloma. Blood (2021) 138(Supplement 1):2745–. doi: 10.1182/blood-2021-152395
102. Usmani SZ, Schjesvold F, Oriol A, Karlin L, Cavo M, Rifkin RM, et al. Pembrolizumab plus lenalidomide and dexamethasone for patients with treatment-naive multiple myeloma (KEYNOTE-185): a randomised, open-label, phase 3 trial. Lancet Haematol (2019) 6(9):e448–e58. doi: 10.1016/s2352-3026(19)30109-7
103. Mateos MV, Blacklock H, Schjesvold F, Oriol A, Simpson D, George A, et al. Pembrolizumab plus pomalidomide and dexamethasone for patients with relapsed or refractory multiple myeloma (KEYNOTE-183): a randomised, open-label, phase 3 trial. Lancet Haematol (2019) 6(9):e459–e69. doi: 10.1016/S2352-3026(19)30110-3
104. Kansagra A, Hansen E, Belli AJ, Goran S, Wang C-K. Evaluation of functional and cytogenetic high-risk multiple myeloma using real-world data. Blood (2021) 138(Supplement 1):4093–. doi: 10.1182/blood-2021-149724
105. Panopoulou A, Easdale S, Ethell M, Nicholson E, Potter M, Giotas A, et al. Impact of ultra high-risk genetics on real-world outcomes of transplant-eligible multiple myeloma patients. Hemasphere (2023) 7(2):e831. doi: 10.1097/HS9.0000000000000831
106. Cohen YC, Zada M, Wang SY, Bornstein C, David E, Moshe A, et al. Identification of resistance pathways and therapeutic targets in relapsed multiple myeloma patients through single-cell sequencing. Nat Med (2021) 27(3):491–503. doi: 10.1038/s41591-021-01232-w
107. Mithraprabhu S, Reynolds J, Turner R, Quach H, Horvath N, Kerridge I, et al. Circulating tumour DNA analysis predicts relapse and improves risk stratification in primary refractory multiple myeloma. Blood Cancer J (2023) 13(1):25. doi: 10.1038/s41408-023-00796-9
108. Majithia N, Rajkumar SV, Lacy MQ, Buadi FK, Dispenzieri A, Gertz MA, et al. Outcomes of primary refractory multiple myeloma and the impact of novel therapies. Am J Hematol (2015) 90(11):981–5. doi: 10.1002/ajh.24131
109. Jurczyszyn A, Waszczuk-Gajda A, Castillo JJ, Krawczyk K, Stork M, Pour L, et al. Primary refractory multiple myeloma: a real-world experience with 85 cases. Leuk Lymphoma (2020) 61(12):2868–75. doi: 10.1080/10428194.2020.1788014
110. Charalampous C, Goel U, Kapoor P, Binder M, Buadi FK, Cook J, et al. Outcomes of patients with primary refractory multiple myeloma in the era of triplet and quadruplet induction therapy. Blood Adv (2023) 7(16):4371–80. doi: 10.1182/bloodadvances.2023009681
111. Banerjee R, Williams L, Mikhael JR. Should I stay or should I go (to transplant)? Managing insufficient responses to induction in multiple myeloma. Blood Cancer J (2023) 13(1):89. doi: 10.1038/s41408-023-00864-0
112. Vij R, Kumar S, Zhang MJ, Zhong X, Huang J, Dispenzieri A, et al. Impact of pretransplant therapy and depth of disease response before autologous transplantation for multiple myeloma. Biol Blood Marrow Transplant (2015) 21(2):335–41. doi: 10.1016/j.bbmt.2014.10.023
113. Sidiqi MH, Aljama MA, Jevremovic D, Morice WG, Timm M, Buadi FK, et al. Plasma cell proliferative index post-transplant is a powerful predictor of prognosis in myeloma patients failing to achieve a complete response. Bone Marrow Transplant (2019) 54(3):442–7. doi: 10.1038/s41409-018-0280-8
114. Cowan AJ, Stevenson PA, Libby EN, Becker PS, Coffey DG, Green DJ, et al. Circulating plasma cells at the time of collection of autologous PBSC for transplant in multiple myeloma patients is a negative prognostic factor even in the age of post-transplant maintenance therapy. Biol Blood Marrow Transplant (2018) 24(7):1386–91. doi: 10.1016/j.bbmt.2018.02.017
115. Gay F, Zaccaria GM, Offidani M, Vozella F, Becco P, Belotti A, et al. Integrative analysis of baseline prognostic features and achievement of minimal residual disease negativity as predictors of early relapse in transplant-eligible multiple myeloma patients. Blood (2019) 134(Supplement_1):605–. doi: 10.1182/blood-2019-125588
116. Xia Y, Shen N, Zhang R, Wu Y, Shi Q, Li J, et al. High-risk multiple myeloma predicted by circulating plasma cells and its genetic characteristics. Front Oncol (2023) 13:1083053. doi: 10.3389/fonc.2023.1083053
117. Belotti A, Ribolla R, Cancelli V, Villanacci A, Angelini V, Chiarini M, et al. Predictive role of diffusion-weighted whole-body MRI (DW-MRI) imaging response according to MY-RADS criteria after autologous stem cell transplantation in patients with multiple myeloma and combined evaluation with MRD assessment by flow cytometry. Cancer Med (2021) 10(17):5859–65. doi: 10.1002/cam4.4136
118. Rasche L, Alapat D, Kumar M, Gershner G, McDonald J, Wardell CP, et al. Combination of flow cytometry and functional imaging for monitoring of residual disease in myeloma. Leukemia (2019) 33(7):1713–22. doi: 10.1038/s41375-018-0329-0
119. Silva A, Silva MC, Sudalagunta P, Distler A, Jacobson T, Collins A, et al. An ex vivo platform for the prediction of clinical response in multiple myeloma. Cancer Res (2017) 77(12):3336–51. doi: 10.1158/0008-5472.CAN-17-0502
120. Bonolo de Campos C, Meurice N, Petit JL, Polito AN, Zhu YX, Wang P, et al. "Direct to Drug" screening as a precision medicine tool in multiple myeloma. Blood Cancer J (2020) 10(5):54. doi: 10.1038/s41408-020-0320-7
121. Coffey DG, Cowan AJ, DeGraaff B, Martins TJ, Curley N, Green DJ, et al. High-throughput drug screening and multi-omic analysis to guide individualized treatment for multiple myeloma. JCO Precis Oncol (2021) 5:PO.20.00442. doi: 10.1200/PO.20.00442
Keywords: myeloma, induction therapy, cytogenetics, functional, high-risk
Citation: Banerjee R, Cicero KI, Lee SS and Cowan AJ (2023) Definers and drivers of functional high-risk multiple myeloma: insights from genomic, transcriptomic, and immune profiling. Front. Oncol. 13:1240966. doi: 10.3389/fonc.2023.1240966
Received: 15 June 2023; Accepted: 18 September 2023;
Published: 02 October 2023.
Edited by:
Saad Zafar Usmani, Levine Cancer Institute, United StatesReviewed by:
Daisy Alapat, University of Arkansas for Medical Sciences, United StatesUrvi Shah, Memorial Sloan Kettering Cancer Center, United States
Copyright © 2023 Banerjee, Cicero, Lee and Cowan. This is an open-access article distributed under the terms of the Creative Commons Attribution License (CC BY). The use, distribution or reproduction in other forums is permitted, provided the original author(s) and the copyright owner(s) are credited and that the original publication in this journal is cited, in accordance with accepted academic practice. No use, distribution or reproduction is permitted which does not comply with these terms.
*Correspondence: Rahul Banerjee, cmFodWwuYmFuZXJqZWUubWRAZ21haWwuY29t
†ORCID: Rahul Banerjee, orcid.org/0000-0003-3781-5441