- 1School of Public Health, North China University of Science and Technology, Department of Hebei Key Laboratory of Medical-Industrial Integration Precision Medicine, North China University of Science and Technology Affiliated Hospital, Tangshan, Hebei, China
- 2Department of Hebei Key Laboratory of Medical-Industrial Integration Precision Medicine, North China University of Science and Technology Affiliated Hospital, Tangshan, Hebei, China
Objective: To discern long non-coding RNAs (lncRNAs) with prognostic relevance in the context of lung squamous cell carcinoma (LUSC), we intend to predict target genes by leveraging The Cancer Genome Atlas (TCGA) repository. Subsequently, we aim to investigate the proliferative potential of critical lncRNAs within the LUSC milieu.
Methods: DESeq2 was employed to identify differentially expressed genes within the TCGA database. Following this, we utilized both univariate and multivariate Cox regression analyses to identify lncRNAs with prognostic relevance. Noteworthy lncRNAs were selected for validation in cell lines. The intracellular localization of these lncRNAs was ascertained through nucleocytoplasmic isolation experiments. Additionally, the impact of these lncRNAs on cellular proliferation, invasion, and migration capabilities was investigated using an Antisense oligonucleotides (ASO) knockdown system.
Results: Multivariate Cox regression identified a total of 12 candidate genes, consisting of seven downregulated lncRNAs (BRE-AS1, CCL15-CCL14, DNMBP-AS1, LINC00482, LOC100129034, MIR22HG, PRR26) and five upregulated lncRNAs (FAM83A-AS1, LINC00628, LINC00923, LINC01341, LOC100130691). The target genes associated with these lncRNAs exhibit significant enrichment within diverse biological pathways, including metabolic processes, cancer pathways, MAPK signaling, PI3K-Akt signaling, protein binding, cellular components, cellular transformation, and other functional categories. Furthermore, nucleocytoplasmic fractionation experiments demonstrated that LINC00923 and LINC01341 are predominantly localized within the cellular nucleus. Subsequent investigations utilizing CCK-8 assays and colony formation assays revealed that the knockdown of LINC00923 and LINC01341 effectively suppressed the proliferation of H226 and H1703 cells. Additionally, transwell assays showed that knockdown of LINC00923 and LINC01341 significantly attenuated the invasive and migratory capacities of H226 and H1703 cells.
Conclusion: This study has identified 12 candidate lncRNA associated with prognostic implications, among which LINC00923 and LINC01341 exhibit potential as markers for the prediction of LUSC outcomes.
1 Introduction
Lung cancer consistently ranks as the leading cause of global cancer-related mortality (1, 2), with lung squamous cell carcinoma (LUSC) exhibiting a heightened propensity for metastasis and recurrence (3–5). In recent years, molecular targeted therapy has substantially enhanced the survival rates of patients afflicted with various malignancies. However, the progress in the realm of molecular targeted therapy for LUSC patients has been notably sluggish (6–8). In order to gain a deeper understanding of the biology of LUSC, the development of robust modeling systems assumes paramount importance (9–11).
Although long non-coding RNAs (lncRNAs) may not possess the same level of evolutionary conservation as protein-coding genes, their promoter regions manifest significant sequence conservation, underscoring the critical nature of lncRNA regulation (12–18). In healthy tissues, lncRNAs are subject to stringent regulation, but in the context of disease, they often succumb to dysregulation, culminating in aberrant expression patterns (19–23). While the mechanistic underpinnings of a subset of lncRNAs have been comprehensively elucidated in the context of LUSC, the vast majority of these molecules remain enigmatic (24–26). Consequently, the present study embarked on the identification of prognosis-related lncRNAs for LUSC, drawing upon the wealth of data within The Cancer Genome Atlas (TCGA) database. Subsequently, we conducted an extensive examination encompassing cell line models, proliferation assays, invasion assessments, and migration studies, all directed toward deciphering the potential of two upregulated lncRNAs in LUSC as predictive biomarkers.
2 Materials and methods
2.1 Sample source
Two tiers of data, denoted as Level 1 and Level 4, were retrieved from the TCGA repository. Level 4 primarily encompasses fundamental patient attributes, including sex, age, race, and the Tumor Lymph Node Metastasis (TNM) staging; whereas Level 1 provides more detailed information, including specifics such as medication dosage, treatment efficacy, radiotherapy records, and other relevant information for each follow-up day. In total, 504 LUSC tumor samples were procured for analysis. RNAseq expression values, which had been corrected utilizing Recursive Structural Equation Model (RSEM), were obtained from the Level 3 dataset of the LUSC project within the TCGA database hosted at Firehost (https://gdac.broadinstitute.org/). Subsequently, known lncRNA expression values were discerned from this dataset, and a total of 51 normal samples and 501 tumor samples were included in the ensuing analysis.
2.2 Differential analysis of lncRNA expression
DESeq2 software was employed to analyze the differential expression of lncRNAs between normal and cancer samples. The lncRNA expression values were subjected to statistical analysis with a predefined significance threshold (p < 0.05, |log2FoldChange| > 1). The software was configured accordingly to derive the differentially expressed lncRNA profiles.
2.3 Screening for lncRNA related to prognosis
We first compiled a list of lncRNAs associated with survival outcomes through single-factor Cox proportional hazard regression analysis. Subsequently, we conducted multivariable Cox proportional hazard regression analysis to identify independent prognostic factors influencing survival. Finally, a stepwise selection procedure was employed in the context of multivariable Cox proportional hazard regression analysis to identify lncRNAs with significant prognostic relevance based on their associated P- values.
2.4 Prediction of lncRNA target genes
The identification of potential lncRNA (in cis acting) target genes was performed by screening for differentially expressed genes within 10 kb upstream and downstream of the lncRNAs. This approach is grounded in the recognition that interactions between lncRNAs and their target genes typically occur in close genomic proximity. The selection of a 10-kilobase range serves to focus the research, mitigate computational complexity, and enhance prediction accuracy. In contrast, the identification of potential lncRNA target genes acting in a trans manner commenced with an initial screening using the blast algorithm (e-value < 1e-5) and was subsequently refined using RNAplex software (G<-20). It is worth noting that lncRNAs exert their influence on mRNA expression through various mechanisms, including: 1) direct cis and trans interactions with mRNAs; 2) binding to microRNAs (miRNAs), thereby impeding miRNA-mediated mRNA regulation; and 3) binding to RNA-binding proteins (RBPs), influencing RBP-mRNA interactions. The scope of this analysis was limited to the examination of RNA-seq data from the TCGA database, which exclusively provides expression profiles of lncRNAs and mRNAs. Therefore, the initial phase of analysis focused on discerning potential interactions between these molecules at the expression level through correlation analysis.
2.5 Analysis of Kyoto Encyclopedia of Genes and Genomes and Gene Ontology functional enrichment of lncRNA target genes
For KEGG analysis, the p-value was determined utilizing Fisher’s exact test. Signal transduction and disease pathways exhibiting statistical significance with a threshold of p < 0.05 were selected for further analysis. For GO analysis, the p-value was computed employing the hypergeometric distribution method. Annotations characterized by a high frequency and possessing a p-value less than 0.05 were retained for subsequent investigation.
2.6 RNA extraction and qPCR experiment
RNA extraction was performed employing the Jinbaite RNA Extraction Kit. Subsequently, DEPC water was introduced, and the concentration of RNA was quantified. Quantitative polymerase chain reaction (qPCR) experiments were executed utilizing the Zhongshi Tongchuang Reverse Transcription and Amplification Kit. The primer sequences employed are detailed in Table 1.
2.7 Nuclear cytoplasmic separation experiment
The isolation of nuclear and cytoplasmic RNA was accomplished employing Norgen’s RNA Nucleocytoplasmic Separation Kit in strict accordance with the manufacturer’s provided instructions. H226 and H1703 cells were collected into enzyme-free EP tubes, and 30 μL of solution buffer was added to each. The procedure began with an initial centrifugation step at 2000 rpm for two minutes, followed by a subsequent centrifugation at 14000 rpm for one minute. Upon removal of the supernatant, lysis buffer was introduced, and centrifugation was repeated. The supernatant, denoted as “tube 2”, was carefully transferred to a new tube, while the original EP tube’s precipitate retained the nuclear RNA and was labeled as “tube 1”. To both tube 1 and tube 2, 400μL of Buffer SK was added, followed by 200 μL of Buffer SK. Vortex mixing for 10 seconds was carried out prior to the addition of 200μL of anhydrous ethanol, followed by further vortex mixing for 10 seconds. The resultant mixture was subsequently loaded into centrifuge columns, with one column allocated for the nuclear fraction and another for the cytoplasmic fraction. Centrifugation was performed at 12000 rpm for one minute, facilitating the separation of nucleic material. Subsequently, the elution process involved the removal of the flow-through liquid from the collection tube, followed by the addition of 400uL of Wash Solution A, accompanied by centrifugation at 12000 rpm for one minute. This washing step was repeated thrice. Finally, a centrifugation at 12000 rpm for two minutes was conducted, after which the centrifuge columns were transferred to new tubes. Here, 50μL of Elution Buffer E was added, and further centrifugation ensued. The resultant eluate was collected, and the RNA concentration was measured.
2.8 Cell transfection
Preparation for transfection commenced with the addition of 500μL of Opti-MEM serum-free medium approximately 30 minutes prior to the transfection process. Transfection mixture A, intended for the introduction of one plasmid into each well of a 6-well plate, was created as follows: plasmid DNA (3 µg) was combined with Opti-MEM serum-free medium to achieve a final volume of 50 µL, and the resultant mixture was gently homogenized. Transfection mixture B was prepared by adding 3μL of Lipofectamine 2000 (Invitrogen, USA) to Opti-MEM serum-free medium until the final volume reached 50 µL. This mixture was subsequently thoroughly mixed and allowed to incubate for a duration of five minutes. The two distinct solutions, transfection mixture A and transfection mixture B, were then merged and incubated for an additional 20 minutes prior to their introduction into the target cells. Subsequently, following a 6-hour incubation period, 1.5 mL of complete medium was introduced.
2.9 CCK-8 experiment
100 μL of cells at a concentration of 1×104 were dispensed into each well of a 96-well plate, with three replicates per group, ensuring that the periphery of the plate was fully surrounded by sterile phosphate-buffered saline (PBS). Following a 48-hour incubation period, 10 μL of the CCK-8 assay solution were added to each well while taking care to prevent bubble formation. Subsequently, the 96-well plate was incubated at room temperature for one hour before being subjected to machine-based testing.
2.10 Colony formation assay
Following a 48-hour period post-transfection, 5×103 cells from each experimental group were inoculated onto individual wells of a 6-well plate, with three wells allocated per sample. Subsequently, crystal violet staining was performed once cellular clusters had formed.
2.11 Transwell assay
To conduct the invasion experiment, an extracellular matrix coating solution was meticulously prepared, and precisely 50 µL of this solution was aseptically dispensed into each well. Subsequently, a serum-free cell suspension, comprising 2×105 cells per well, was introduced into the upper compartment of the transwell system. The lower compartment was filled with a culture medium supplemented with 20% Fetal Bovine Serum (FBS). Following a 48-hour incubation period, the transwell chambers were carefully extracted. The cells were subsequently subjected to fixation using methanol for a duration of seven minutes, followed by a 7-minute staining procedure with crystal violet. Following the staining protocol, the cells underwent a thorough washing process. The membrane, bearing the adherent cells, was affixed onto a microscope slide and subsequently subjected to microscopic examination, with three random fields captured for further analysis. The migration experiment, in contrast, did not entail the utilization of matrix adhesive. However, all other procedural steps remain consistent with those employed in the invasion experiment.
2.12 Statistical analysis
In this study, statistical analyses were conducted utilizing the Student’s t-test and the χ2 test. The difference between two groups was assessed via a two-tailed t-test analysis, with a significance threshold set at p < 0.05. Survival analysis was conducted employing the Kaplan−Meier method, and intergroup differences among patients were compared utilizing the log-rank test.
3 Results
3.1 Sample source and differential lncRNA analysis
We conducted a comprehensive statistical analysis of key clinical attributes pertaining to LUSC patients, as delineated in Table 2. Principal component analysis (PCA) indicated that the selected samples could be effectively categorized into a single cohesive group for subsequent analyses, as presented in Figure 1A. Subsequently, a rigorous differential expression analysis of lncRNAs was undertaken on the samples, yielding a volcano plot that depicted the expression variations across all lncRNAs. Within this analysis, a total of 160 lncRNAs exhibited a significant upregulation, while 110 lncRNAs displayed a significant downregulation, as illustrated in Figure 1B. In order to screen for lncRNAs with potential synergistic effects or similar regulation, and to help explore the functions of lncRNAs, clustering analysis was conducted. The clustering analysis results showed the expression trend of all differential lncRNAs in all samples, as shown in Figure 1C.
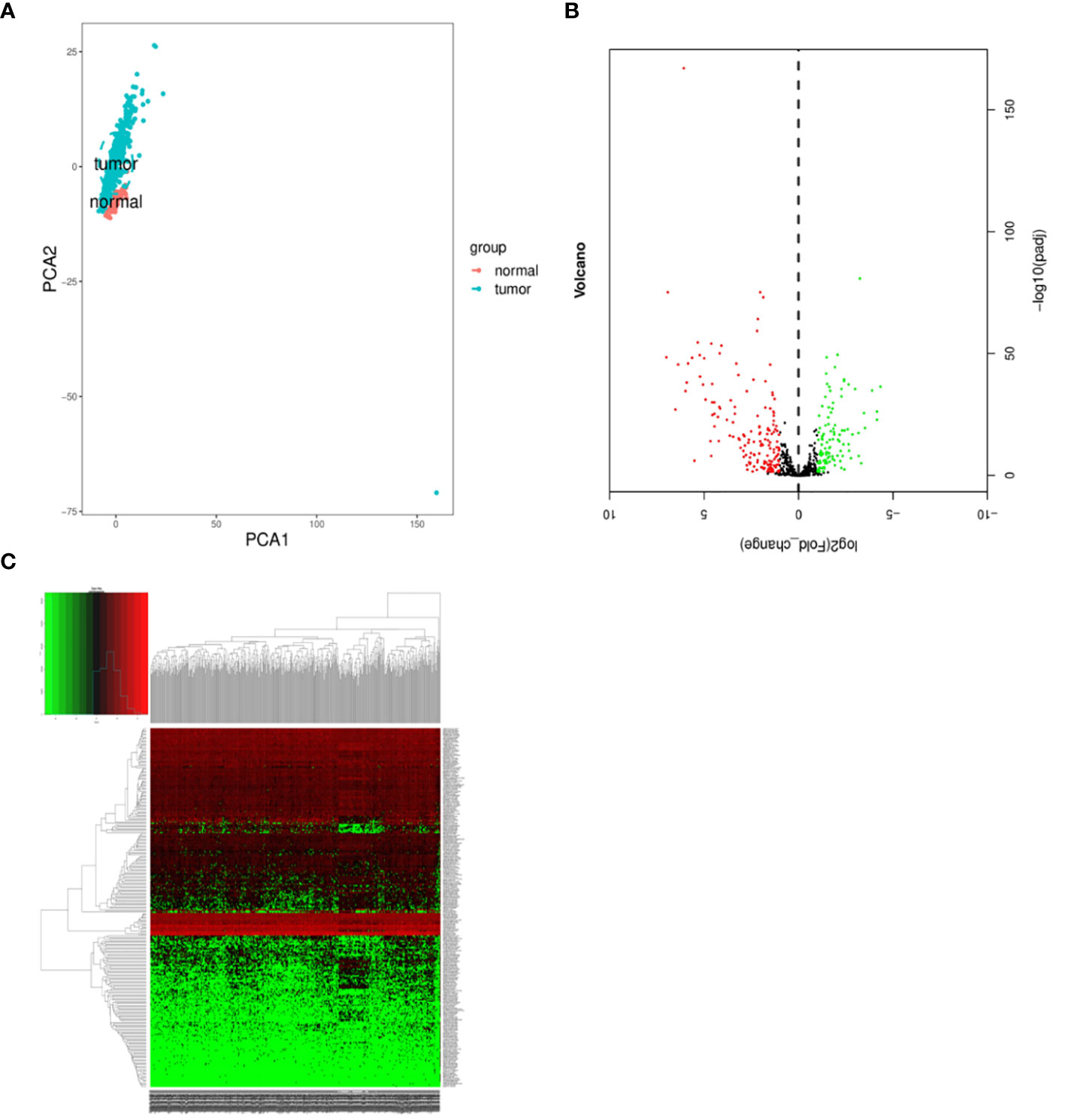
Figure 1 Differentially expressed lncRNAs in samples. (A) Principal component analysis. (B) Volcano plot visualization of differential lncRNA expression. (C) Chart depicting clustering analysis of lncRNAs. In Figure C, the horizontal axis illustrates the clustering of samples, with each column representing an individual sample. The vertical axis denotes the substantial distinctions in lncRNA clustering. The color scale reflects the abundance of lncRNA expression, where red signifies elevated lncRNA expression within the sample, while green signifies lower expression levels. Deeper shades of color indicate more pronounced differences in expression levels.
3.2 Screening and analysis of prognosis-related lncRNAs
By conducting an in-depth analysis of the correlation between overall survival (OS) and lncRNAs through univariate Cox proportional hazard regression, we successfully identified 271 lncRNAs with significant prognostic implications. Subsequently, a refined selection process involving multifactorial Cox proportional hazard regression analysis led to the identification of twelve lncRNAs. To provide insight into the expression patterns of these selected lncRNAs in LUSC and adjacent tissues, we performed t-tests and generated corresponding box plots. In the graphical representation, the designation “N” pertains to the adjacent cancer sample group, while “T” signifies the cancer sample group. Our analytical findings unveiled noteworthy disparities in the expression levels of these 12 lncRNAs between the cancer and adjacent cancer samples. Among the identified lncRNAs, seven (BRE-AS1, CCL15-CCL14, DNMBP-AS1, LINC00482, LOC100129034, MIR22HG, PRR26) exhibited significant downregulation, while five (FAM83A-AS1, LINC00628, LINC00923, LINC01341, LOC100130691) demonstrated notable upregulation, as depicted in Figure 2.
3.3 Prediction of target genes for lncRNA
Table 3 presents the predicted outcomes concerning the target genes associated with the 12 identified lncRNAs. It is noteworthy that the target genes of the differentially downregulated lncRNAs exhibited a pronounced enrichment in pivotal biological pathways, specifically those linked to metabolism, cancer, MAPK signaling, and PI3K-Akt signaling pathways (Figure 3). Conversely, the differentially upregulated lncRNAs demonstrated enriched target genes predominantly within metabolic and PI3K-Akt signaling pathways (Figure 4). Additionally, an overarching analysis of both downregulated and upregulated lncRNA target genes revealed a predominant enrichment in functions related to protein binding, cellular components, and cellular transformation, as depicted in Figures 5A, B. This extensive array of functions encompasses vital processes such as lung epithelial phosphorylation and cellular hypermetabolism, both of which play significant roles in the initiation and progression of LUSC.
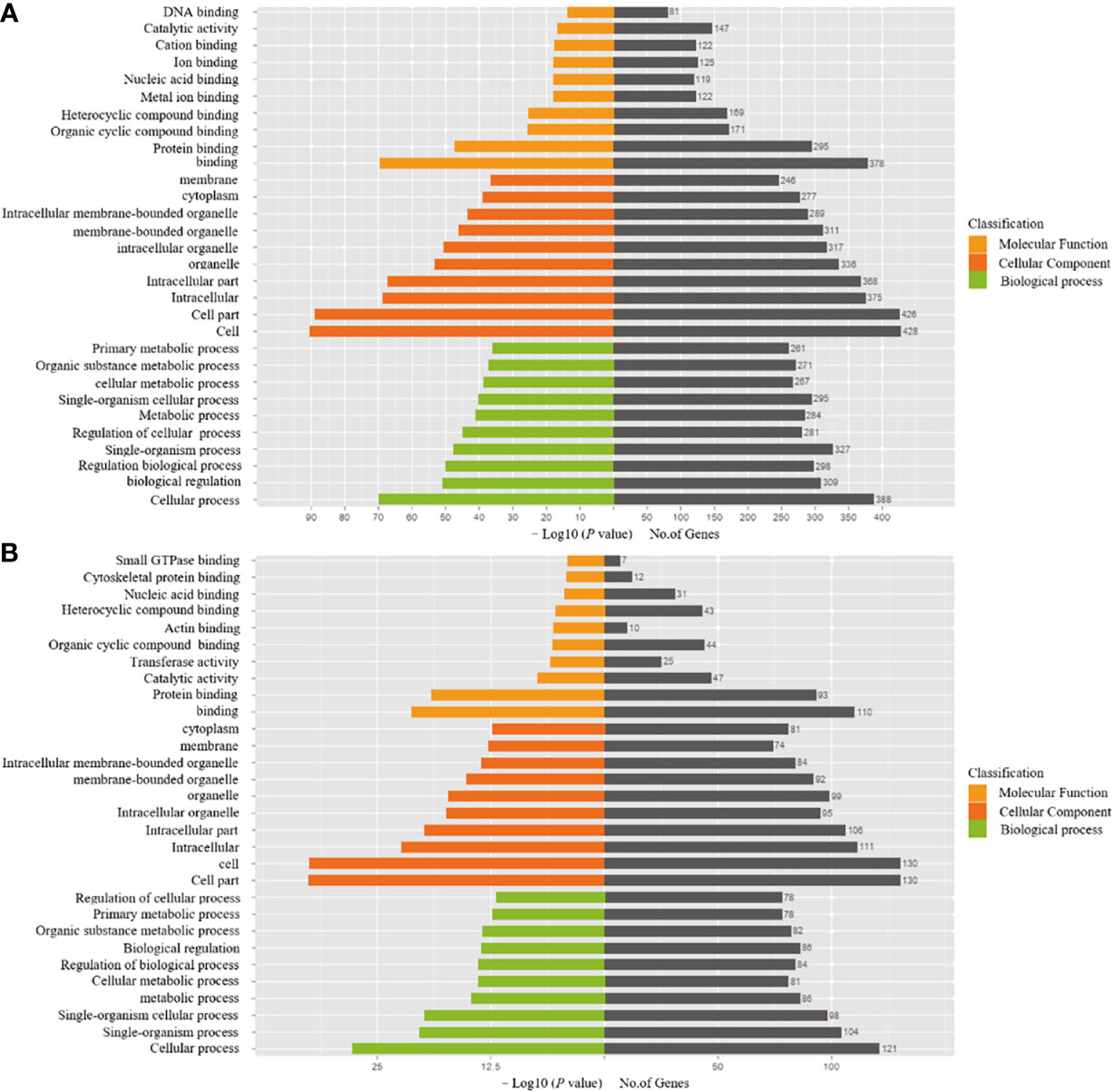
Figure 5 GO enrichment analyses of target genes of differentially expressed lncRNAs. (A) Functional analysis of differentially downregulated lncRNA target genes. (B) Functional analysis of differentially upregulated lncRNA target genes.
3.4 Verification of the proliferation ability of LINC00923 and LINC01341 in H226 cells
In our assessment of the expression profiles of BRE-AS1, CCL15-CCL14, DNMBP-AS1, LINC00482, LOC100129034, MIR22HG, PRR26, FAM83A-AS1, LINC00628, LINC00923, LINC01341, and LOC100130691 within H226 and H1703 cells, we observed that relative to normal lung epithelial cells, BRE-AS1 and CCL15-CCL14 exhibited downregulation in both H226 and H1703 cells, while LINC00923 and LINC01341 exhibited upregulation in H226 and H1703 cells, respectively. These findings are graphically represented in Figure 6. The expression levels of the remaining lncRNAs in H226 and H1703 cells exhibited slight deviations from the predicted outcomes, which could be attributed to the inherent heterogeneity among tumor cells. Given the detectability of upregulated lncRNAs in patient fluids and various samples, their study vis-à-vis their impact on tumorigenesis is often more feasible. Consequently, LINC00923 and LINC01341 were prioritized for further investigation. Initially, we selected these upregulated lncRNAs, LINC00923 and LINC01341, to assess their nucleocytoplasmic expression in H226 and H1703 cells. Our analyses indicated that LINC00923 and LINC01341 were predominantly localized within the nuclei of H226 and H1703 cells, as depicted in Figure 7. Given the conspicuous nuclear presence of LINC00923 and LINC01341, we conducted a comprehensive evaluation of their roles in the proliferation, invasion and migration ability of LINC00923 and LINC01341 in LUSC H226 and H1703 cells. Employing Antisense Oligonucleotide (ASO) primers, we embarked on a series of experiments encompassing CCK-8 assays, colony formation assays, and transwell assays. These assays were conducted to assess the proliferation, invasion, and migration capabilities of H226 and H1703 cells in response to the knockdown of LINC00923 and LINC01341. The transfection efficiency was confirmed through quantitative reverse transcription PCR (qRT-PCR), as illustrated in Figures 8A–D. Our results from the CCK-8 assay (Figures 8E–H) and the colony formation assay (Figures 8I–L) demonstrated that the downregulation of LINC00923 and LINC01341 exerted inhibitory effects on the proliferation of H226 and H1703 cells. Furthermore, our transwell experiments corroborated that the suppression of LINC00923 and LINC01341 led to attenuated invasive and migratory capabilities of H226 and H1703 cells, as illustrated in Figure 9.
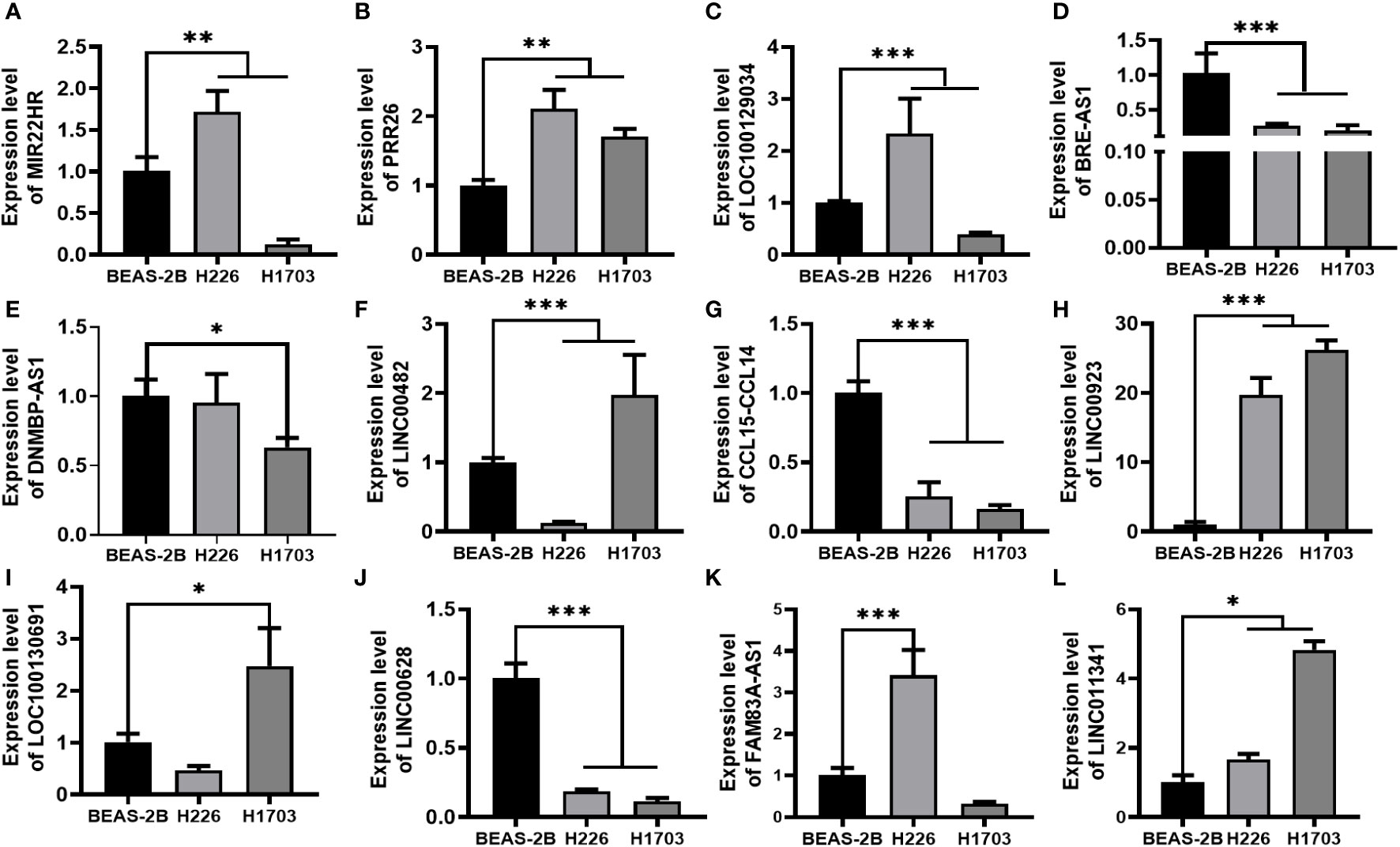
Figure 6 Verification of lncRNAs in H226 and H1703 cells. (A–L). The expression levels of MIR22HG, PRR26, LOC100129034, BRE-AS1, DNMBP-AS1, LINC00482, CCL15-CCL14, LINC00923, LOC100130691, LINC00628, FAM83A-AS1, and LINC01341 in normal lung epithelial cells BEAS-2B and lung squamous cells H226 and H1703, respectively. *P<0.05; **P<0.01; ***P<0.001.
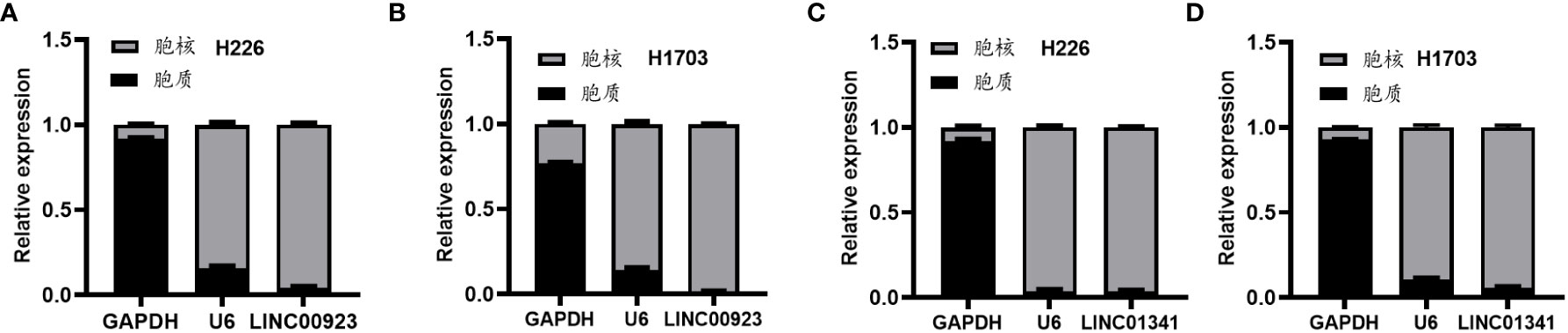
Figure 7 Illustration of the heightened expression of LINC00923 and LINC01341 within the nuclei of both H226 and H1703 cells. (A, B) The expression levels of LINC00923 in the nuclei of H226 and H1703 cells, respectively. (C, D) The expression levels of LINC01341 in the nuclei of H226 and H1703 cells, respectively.
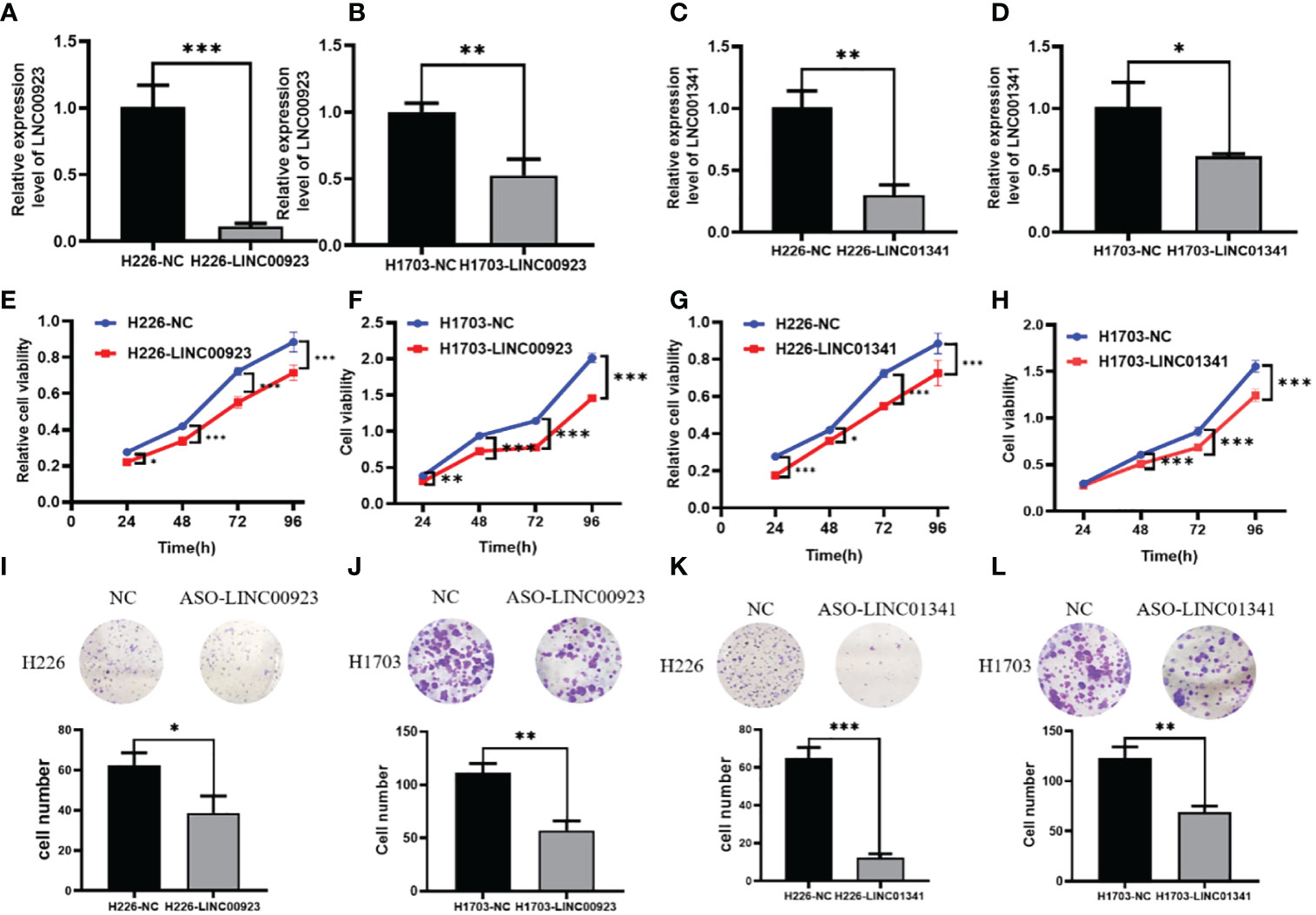
Figure 8 Illustration of the inhibitory effect of LINC00923 and LINC01341 knockdown on the proliferation of LUSC H226 and H1703 cells. (A, B) Evaluation of LINC00923 knockdown efficiency in H226 and H1703 cells. (C, D) Evaluation of LINC01341 knockdown efficiency in H226 and H1703 cells. (E, F) Assessment of proliferation capacity following LINC00923 knockdown in H226 and H1703 cells via CCK-8 assay. (G, H) Assessment of proliferation capacity following LINC01341 knockdown in H226 and H1703 cells via CCK-8 assay. (I, J) Determination of proliferation potential after LINC00923 knockdown in H226 and H1703 cells via colony formation assay. (K, L) Determination of proliferation potential after LINC01341 knockdown in H226 and H1703 cells via colony formation assay. *P<0.05; **P<0.01; ***P<0.001.
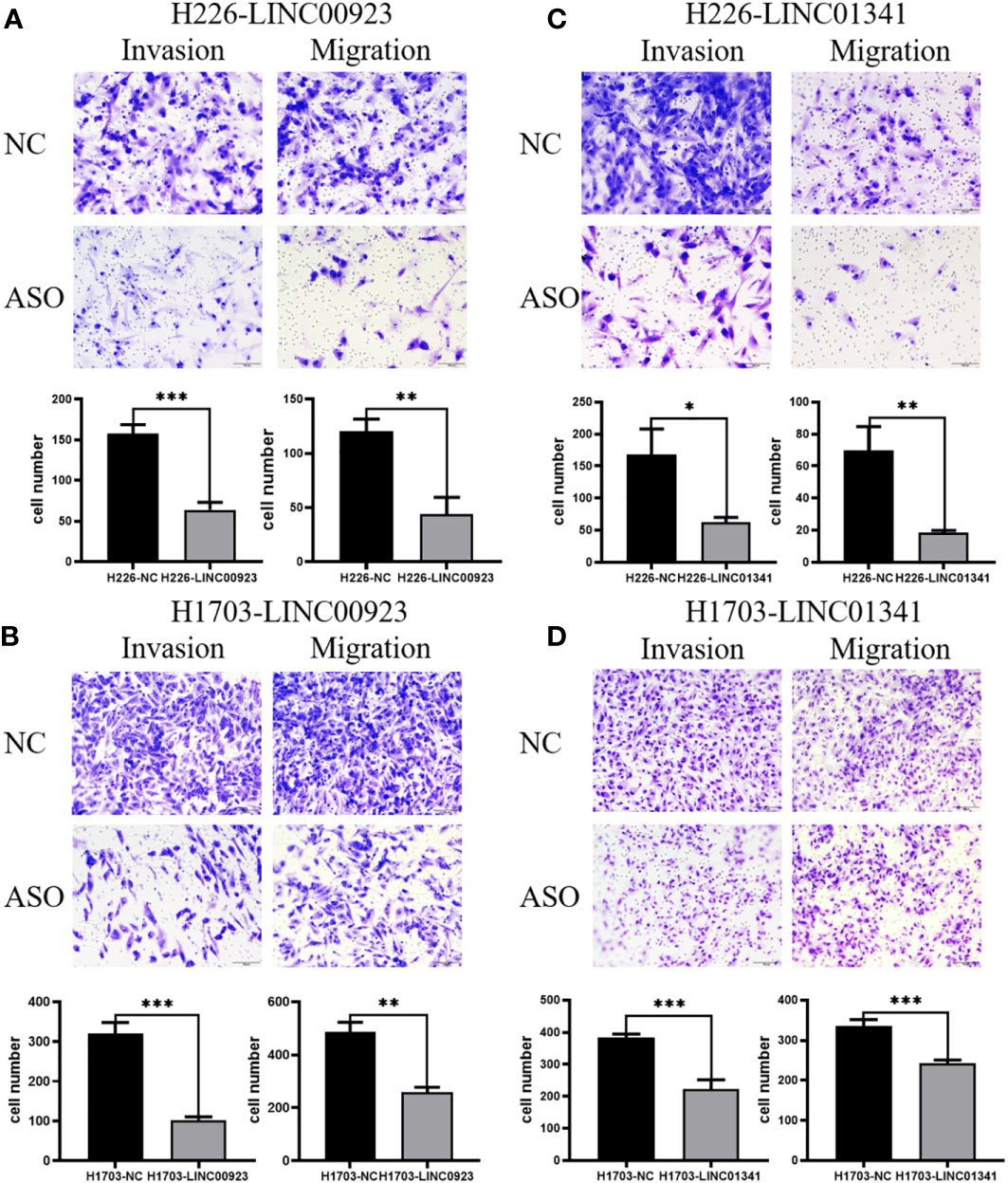
Figure 9 Illustration of the inhibitory effect of LINC00923 and LINC01341 knockdown on the invasion and migration of LUSC H226 and H1703 cells. (A, B) Evaluation of migration and invasion potential following LINC00923 knockdown in H226 and H1703 cells via transwell assay. (C, D) Assessment of migration and invasion potential following LINC01341 knockdown in H226 and H1703 cells via transwell assay. *P<0.05; **P<0.01; ***P<0.001.
4 Discussion
LUSC is characterized by a notably unfavorable prognosis, with a 5-year survival rate of merely approximately 15% due to often delayed diagnoses (27–30). Conventional diagnostic techniques exhibit limitations in terms of sensitivity and specificity, which poses challenges for early detection. Consequently, there is a pressing need for novel biomarkers that can enhance molecular diagnostics and prognostic accuracy (31–38). While proteins have historically served as diagnostic biomarkers, lncRNAs present distinctive advantages, including their inherent stability, tissue-specific expression patterns, and amenability to detection in various physiological fluids (39–42). Numerous investigations have validated the utility of lncRNAs in effectively distinguishing patients with early-stage cancer from healthy controls, showcasing their capacity to provide valuable prognostic insights pertaining to metastatic potential and recurrence likelihood (43). For instance, in the realms of oesophageal cancer (44), colorectal cancer (45), lung adenocarcinoma (46), and pancreatic cancer (47), lncRNAs have emerged as independent markers with the potential to predict disease outcomes. Consequently, the burgeoning body of evidence underscores the prospective utility of lncRNAs as markers for LUSC.
Numerous carcinogenic lncRNAs, including PITPNA-AS1 (48), lncRNA ATB (49), and LINC00173. v1 (50), are pivotal regulators in LUSC (51). Our initial step encompassed the comprehensive screening of differentially expressed lncRNAs, from which we discerned 12 exhibiting significant prognostic relevance. Within this selection, seven lncRNAs (BRE-AS1, CCL15-CCL14, DNMBP-AS1, LINC00482, LOC100129034, MIR22HG, PRR26) exhibited downregulation, while five (FAM83A-AS1, LINC00628, LINC00923, LINC01341, LOC100130691) displayed upregulation. Notably, with the exception of lncRNA MIR22HG, lncRNA BRE-AS1, lncRNA FAM83A-AS1, and LINC00628, the differential expression of the remaining lncRNAs was previously undocumented in the context of LUSC, establishing their association with prognostic outcomes for the first time. Evidently, the silencing of lncRNA MIR22HG engenders the activation of cell survival/death signaling pathways, indicating the potential of lncRNA MIR22HG as a novel diagnostic and prognostic marker for LUSC (52). Furthermore, lncRNA BRE-AS1, through the upregulation of NR4A3, elicits inhibitory effects on the growth and survival of lung adenocarcinoma cells (53). Conversely, lncRNA FAM83A-AS1 exerts a promotional influence on A549 cell progression by elevating FAM83A expression, concurrently heightening HIF-1 levels within the lung adenocarcinoma α/glycolysis axis, thereby augmenting tumoral proliferation and migration (54, 55). Additionally, studies have illuminated the epigenetic interaction of LINC00628 with the LAMA3 promoter, culminating in the development of lung adenocarcinoma (56). Noteworthy differential expression of LINC00628 between lung adenocarcinoma and LUSC has been identified, further emphasizing its prognostic relevance (57). In summary, the lncRNAs delineated in this investigation, which possess significant prognostic implications, facilitate the initiation of lung adenocarcinoma via distinct mechanistic pathways while concurrently displaying shared attributes across both lung adenocarcinoma and LUSC. This observation provides a degree of assurance regarding the accuracy and reliability of the lncRNAs identified in our study.
This study indicates that the target genes governed by lncRNAs are predominantly enriched in pivotal biological processes, including metabolism, oncogenesis, MAPK signaling, and PI3K-Akt signaling pathways, while concurrently harboring functional attributes encompassing protein binding, cellular composition, and cellular transformation. These findings substantiate the prevailing literature concerning LUSC (58, 59). Notably, prior research endeavors have unveiled that LUSC exhibits differential protein expression profiles primarily characterized by enrichments in metabolic pathways and other signal transduction cascades (60). Moreover, our in vitro cellular experiments have successfully corroborated that two lncRNAs, which were computationally predicted to exhibit heightened expression levels in LUSC, indeed manifest augmented expression in LUSC cells. Conversely, two lncRNAs forecasted to exhibit diminished expression levels in LUSC cells did, indeed, demonstrate reduced expression. Furthermore, upon the knockdown of LINC00923 and LINC01341 in H226 and H1703 cells, the cellular attributes associated with proliferation, invasion, and migration were significantly attenuated. This empirical evidence, to a certain extent, substantiates the fidelity and reliability of our prediction results.
This study has been primarily constructed utilizing data sourced exclusively from the TCGA, thereby necessitating a noteworthy caveat regarding the lack of external dataset validation, thus constituting a limitation of our investigation. Future research endeavors can overcome this limitation by undertaking comprehensive multicohort analyses that amalgamate the prognostic value of the identified lncRNAs with available expression datasets encompassing LUSC. Moreover, the current study lays a foundation for potential cellular investigations aimed at elucidating the mechanistic links between LINC00923 and LINC01341 and their putative target genes within the metabolic and PI3K-Akt signaling pathways. Such investigations would furnish additional empirical substantiation, thereby bolstering the candidacy of LINC00923 and LINC01341 as viable biomarkers for prognosticating LUSC. In summary, this study has successfully winnowed down a compendium of 12 lncRNAs exhibiting prognostic relevance. Subsequent cellular validation endeavors have identified LINC00923 and LINC01341 as prospective biomarkers with the potential to serve as predictive indicators for LUSC.
Data availability statement
The datasets presented in this study can be found in online repositories. The names of the repository/repositories and accession number(s) can be found below: https://www.ncbi.nlm.nih.gov/, https://www.jianguoyun.com/c/sd/17d0c4c/4f4303a7e2ec9502.
Author contributions
Conception and design: YC. Acquisition of data: YW and YC. Analysis and interpretation of data: YC, MZ, YZ, XS, and WK. Writing and review of the manuscript: YC and XZ. Revision of the manuscript and study supervision: GS. All authors contributed to the article and approved the submitted version.
Funding
The author(s) declare financial support was received for the research, authorship, and/or publication of this article. This work was supported by the National Natural Science Foundation of China (grant no. H82172658); Supported by the Basic Innovation Team Grant of Precision Diagnosis & Treatment of Small Cell Lung Cancer of Tangshan Medical & Engineering Integration Foundation (grant no. 21130203D); Supported by the Project of High Level Group for Research and Innovation of School of Public Health, North China University of Science and Technology (grant no. KYTD202309). The funders had no role in study design, data collection and analysis, decision to publish, or preparation of the manuscript.
Conflict of interest
The authors declare that the research was conducted in the absence of any commercial or financial relationships that could be construed as a potential conflict of interest.
Publisher’s note
All claims expressed in this article are solely those of the authors and do not necessarily represent those of their affiliated organizations, or those of the publisher, the editors and the reviewers. Any product that may be evaluated in this article, or claim that may be made by its manufacturer, is not guaranteed or endorsed by the publisher.
References
1. Siegel RL, Miller KD, Fuchs HE, Jemal A. Cancer statistics, 2022. CA Cancer J Clin (2022) 72:7–33. doi: 10.3322/caac.21708
2. Wang Y, Cheng Q, Xia Z, Zhou R, Li Y, Meng L, et al. Whole-transcriptome sequencing identifies key mRNAs, miRNAs, lncRNAs, and circRNAs associated with unexplained recurrent pregnancy loss. Cell Tissue Res (2022) 389:129–43. doi: 10.1007/s00441-022-03632-x
3. Jiang T, Shi J, Dong Z, Hou L, Zhao C, Li X, et al. Genomic landscape and its correlations with tumor mutational burden, PD-L1 expression, and immune cells infiltration in Chinese lung squamous cell carcinoma. J Hematol Oncol (2019) 12:75–87. doi: 10.1186/s13045-019-0762-1
4. Adderley H, Blackhall FH, Lindsay CR. KRAS-mutant non-small cell lung cancer: converging small molecules and immune checkpoint inhibition. EBioMedicine (2019) 41:711–16. doi: 10.1016/j.ebiom.2019.02.049
5. Stewart PA, Welsh EA, Slebos R, Fang B, Lzumi V, Chambers M, et al. Proteogenomic landscape of squamous cell lung cancer. Nat Commun (2019) 10:3578–94. doi: 10.1038/s41467-019-11452-x
6. Pan Y, Han H, Hu H, Wang H, Song Y, Hao Y, et al. KMT2D deficiency drives lung squamous cell carcinoma and hypersensitivity to RTK-RAS inhibition. Cancer Cell (2023) 41:88–105.e8. doi: 10.1016/j.ccell.2022.11.015
7. Hao B, Zhang Z, Lu Z, Xiong J, Fan T, Song C, et al. Single-cell RNA sequencing analysis revealed cellular and molecular immune profiles in lung squamous cell carcinoma. Transl Oncol (2023) 27:101568. doi: 10.1016/j.tranon.2022.101568
8. Zhang H, AbdulJabbar K, Moore DA, Akarca A, Enfield KS, Jamal-Hanjani M, et al. Spatial positioning of immune hotspots reflects the interplay between B and T cells in lung squamous cell carcinoma. Cancer Res (2023) 83:1410–25. doi: 10.1158/0008-5472.CAN-22-2589
9. Yang L, Wei S, Zhang J, Hu Q, Hu W, Cao M, et al. Construction of a predictive model for immunotherapy efficacy in lung squamous cell carcinoma based on the degree of tumor-infiltrating immune cells and molecular typing. J Trans Med (2022) 20:364. doi: 10.1186/s12967-022-03565-7
10. Gao M, Kong W, Huang Z, Xie Z. Identification of key genes related to lung squamous cell carcinoma using bioinformatics analysis. Int J Mol Sci (2020) 21:2994. doi: 10.3390/ijms21082994
11. Li N, Wang J, Zhan X. Identification of immune-related gene signatures in lung adenocarcinoma and lung squamous cell carcinoma. Front Immunol (2021) 12:752643. doi: 10.3389/fimmu.2021.752643
12. Mongelli A, Martelli F, Farsetti A, Gaetano C. The Dark That Matters: Long non-coding RNAs as master regulators of cellular metabolism in non-communicable diseases. Front Physiol (2019) 10:369. doi: 10.3389/fphys.2019.00369
13. Luo D, Deng B, Weng M, Luo Z, Nie X. A prognostic 4-lncRNA expression signature for lung squamous cell carcinoma. Artif Cells (2018) 46:1207–14. doi: 10.1080/21691401.2017.1366334
14. Canzio D, Nwakeze CL, Horta A, Rajkumar SM, Coffey EL, Duffy EE, et al. Antisense lncRNA transcription mediates DNA demethylation to drive stochastic protocadherin α promoter choice. Cell (2019) 177:639–53. doi: 10.1016/j.cell.2019.03.008
15. Joung J, Engreitz JM, Konermann S, Abudayyeh OO, Verdine VK, Aguet F, et al. Genome-scale activation screen identifies a lncRNA locus regulating a gene neighbourhood. Nature (2017) 548:343–46. doi: 10.1038/nature23451\
16. Zhang T, Su F, Lu YB, Ling XL, Dai HY, Yang TN, et al. MYC/MAX-Activated LINC00958 Promotes Lung Adenocarcinoma by oncogenic transcriptional reprogramming through HOXA1 activation[J]. Front Oncol (2022) 12:807507. doi: 10.3389/fonc.2022.807507
17. Zhang X, Wang Q, Xu Y, Wang B, Jia C, Wang L, et al. LncRNA PCAT19 negatively regulates p53 in non-small cell lung cancer[J]. Oncol Lett (2019) 18(6):6795–800. doi: 10.3892/ol.2019.11041
18. Shen Q, Sun Y, Xu S. LINC01503/miR-342-3p facilitates Malignancy in non-small-cell lung cancer cells via regulating LASP1[J]. Respir Res (2020) 21(1):235. doi: 10.1186/s12931-020-01464-3
19. Fan Y, Zhou Y, Li X, Lou M, Gao Z, Tong J, et al. Long non-coding RNA AL513318.2 as ceRNA binding to hsa-miR-26a-5p upregulates SLC6A8 expression and predicts poor prognosis in non-small lung cancer[J]. Front Oncol (2022) 12:878864. doi: 10.3389/fonc.2022.878864
20. Kolenda T, Guglas K, Kopczyńska M, Teresiak A, Blizniak R, Mackiewicz A, et al. Oncogenic role of ZFAS1 lncRNA in head and neck squamous cell carcinomas. Cells (2019) 8:366–82. doi: 10.3390/cells8040366
21. Zhan Y, Zang H, Feng J, Lu J, Chen L, Fan S. Long non-coding RNAs associated with non-small cell lung cancer. Oncotarget (2017) 8:69174–84. doi: 10.18632/oncotarget.20088
22. Liang Y, Song X, Li Y, Chen B, Zhao W, Wang L, et al. LncRNA BCRT1 promotes breast cancer progression by targeting miR-1303/PTBP3 axis[J]. Mol Cancer (2020) 19(1):85. doi: 10.1186/s12943-020-01206-5
23. Li W, Cui Y, Ma W, Wang M, Cai Y, Jiang Y. LncRNA RBPMS-AS1 promotes NRGN transcription to enhance the radiosensitivity of glioblastoma through the microRNA-301a-3p/CAMTA1 axis[J]. Transl Oncol (2021) 15(1):101282. doi: 10.1016/j.tranon.2021.101282
24. Sasa GBK, Xuan C, Chen M, Jiang Z, Ding X. Clinicopathological implications of lncRNAs, immunotherapy and DNA methylation in lung squamous cell carcinoma: a narrative review. Transl Cancer Res (2021) 10:5406–29. doi: 10.21037/tcr-21-1607
25. Nandwani A, Rathore S, Datta M. LncRNAs in cancer: Regulatory and therapeutic implications. Cancer Lett (2020) 501:162–71. doi: 10.1016/j.canlet.2020.11.048
26. Kong X, Duan Y, Sang Y, Li Y, Zhang H, Liang Y, et al. LncRNA-CDC6 promotes breast cancer progression and function as ceRNA to target CDC6 by sponging microRNA-215. J Cell Physiol (2019) 234:9105–17. doi: 10.1002/jcp.27587
27. Zhang X, Su Y, Fu X, Xiao J, Qin G, Yu M, et al. Evaluation of the prognostic value of long noncoding RNAs in lung squamous cell carcinoma. J Oncol (2022) 2022:9273628–37. doi: 10.1155/2022/9273628
28. Lai Y, Chen W, Hsu T, Lin C, Tsao Y, Wu S. Overall survival prediction of non-small cell lung cancer by integrating microarray and clinical data with deep learning. Sci Rep (2020) 10:4679–89. doi: 10.1038/s41598-020-61588-w
29. Li B, Cui Y, Diehn M, Li R. Development and validation of an individualized immune prognostic signature in early-stage non squamous non-small cell lung cancer. JAMA Oncol (2017) 3:1529–37. doi: 10.1001/jamaoncol.2017.1609
30. Lin T, Fu Y, Zhang X, Gu J, Ma X, Miao R, et al. A seven-long noncoding RNA signature predicts overall survival for patients with early stage non-small cell lung cancer. Aging (2018) 10:2356–66. doi: 10.18632/aging.101550
31. Zhang L, Peng D, Sood AK, Dang CV, Zhong X. Shedding light on the dark cancer genomes: Long noncoding RNAs as novel biomarkers and potential therapeutic targets for cancer. Mol Cancer Ther (2018) 17:1816–23. doi: 10.1158/1535-7163.MCT-18-0124
32. Esposito R, Bosch N, Lanzós A, Polidori T, Pulido-Quetglas C, Johnson R. Hacking the Cancer Genome: profiling therapeutically actionable long non-coding RNAs using CRISPR-Cas9 screening. Cancer Cell (2019) 35:545–57. doi: 10.1016/j.ccell.2019.01.019
33. Guo L, Gu J, Hou S, Liu D, Zhou M, Hua T, et al. Long non-coding RNA DANCR promotes the progression of non-small-cell lung cancer by inhibiting p21 expression[J]. Onco Targets Ther (2018) 12:135–46. doi: 10.2147/OTT.S186607
34. Lu T, Wang Y, Chen D, Liu J, Jiao W. Potential clinical application of lncRNAs in non-small cell lung cancer. Onco Targets Ther (2018) 11:8045–52. doi: 10.2147/OTT.S178431
35. Gómez-López S, Whiteman ZE, Janes SM. Mapping lung squamous cell carcinoma pathogenesis through in vitro and in vivo models. Commun Biol (2021) 4:937–51. doi: 10.1038/s42003-021-02470-x
36. Jamal-Hanjani M, Wilson GA, McGranahan N, Birkbak NJ, Watkins TBK, Veeriah S, et al. Tracking the evolution of non-small-cell lung cancer. N Engl J Med (2017) 376:2109–21. doi: 10.1056/NEJMoa1616288
37. Pan Y, Han H, Labbe KE, Zhang H, Wong K. Recent advances in preclinical models for lung squamous cell carcinoma. Oncogene (2021) 40:2817–29. doi: 10.1038/s41388-021-01723-7
38. Campbell JD, Alexandrov A, Kim J, Wala J, Berger AH, Pedamallu CS, et al. Distinct patterns of somatic genome alterations in lung adenocarcinomas and squamous cell carcinomas. Nat Genet (2016) 48:607–16. doi: 10.1038/ng.3564
39. Liu T, Han Z, Li H, Zhu Y, Sun Z, Zhu A. LncRNA DLEU1 contributes to colorectal cancer progression via activation of KPNA3. Mol Cancer (2018) 17:118–30. doi: 10.1186/s12943-018-0873-2
40. Rathinasamy B, Velmurugan BK. Role of lncRNAs in the cancer development and progression and their regulation by various phytochemicals. BioMed Pharmacother (2018) 102:242–8. doi: 10.1016/j.biopha.2018.03.077
41. Grillone K, Riillo C, Scionti F, Rocca R, Tradigo G, Guzzi PH, et al. Non-coding RNAs in cancer: platforms and strategies for investigating the genomic "dark matter". J Exp Clin Cancer Res (2020) 39:117–35. doi: 10.1186/s13046-020-01622-x
42. Lemos AEG, Matos ADR, Ferreira LB, Gimba ERP. The long non-coding RNA PCA3: an update of its functions andclinical applications as a biomarker in prostate cancer. Oncotarget (2019) 10:6589–603. doi: 10.18632/oncotarget.27284
43. Chen R, Xia W, Wang S, Xu Y, Ma Z, Xu W, et al. Long noncoding RNA SBF2-AS1 is critical for tumorigenesis of early-stage lung adenocarcinoma[J]. Mol Therapy-Nucleic Acids (2019) 16:543–53. doi: 10.1016/j.omtn.2019.04.004
44. Xie J, Jiang Y, Yuan J, Li C, Lim M, An O, et al. Super-Enhancer-Driven long non-coding RNA LINC01503, regulated by TP63, is over-expressed and oncogenic in squamous cell carcinoma. Gastroenterology (2018) 154:2137–51. doi: 10.1053/j.gastro.2018.02.018
45. Bian Z, Zhang J, Li M, Feng Y, Wang X, Zhang J, et al. LncRNA FEZF1-AS1 promotes tumor proliferation and metastasis in 2 colorectal cancer by regulating PKM2 signaling. Clin Cancer Res (2018) 24:4808–19. doi: 10.1158/1078-0432.CCR-17-2967
46. Malakar P, Stein I, Saragovi A, Winkler R, Stern-Ginossar N, Berger M, et al. Long noncoding RNA MALAT1 regulates cancer glucose metabolism by enhancing mTOR-Mediated translation of TCF7L2. Cancer Res (2019) 79:2480–93. doi: 10.1158/0008-5472.CAN-18-1432
47. Zeng Z, Xu FY, Zheng H, Cheng P, Chen Q, Ye Z, et al. LncRNA-MTA2TR functions as a promoter in pancreatic cancer via driving deacetylation-dependent accumulation of HIF-1α. Theranostics (2019) 9:5298–314. doi: 10.7150/thno.34559
48. Ren P, Xing L, Hong X, Chang L, Zhang H. LncRNA PITPNA-AS1 boosts the proliferation and migration of lung squamous cell carcinoma cells by recruiting TAF15 to stabilize HMGB3 mRNA. Cancer Med (2020) 9:7706–16. doi: 10.1002/cam4.3268
49. Li J, Xia R, Liu T, Cai X, Geng G. LncRNA-ATB promotes lung squamous carcinoma cell proliferation, migration, and invasion by targeting microRNA-590-5p/NF90 Axis. DNA Cell Biol (2020) 39:459–73. doi: 10.1089/dna.2019.5193
50. Chen J, Liu A, Wang Z, Wang B, Chai X, Lu W, et al. LINC00173.v1 promotes angiogenesis and progression of lung squamous cell carcinoma by sponging miR-511-5p to regulate VEGFA expression. Mol Cancer (2020) 19:98–116. doi: 10.1186/s12943-020-01217-2
51. Man J, Zhang Z, Dong H, Li S, Yu X, Meng L, et al. Screening and identification of key biomarkers in lung squamous cell carcinoma by bioinformatics analysis. Oncol Lett (2019) 18:5185–96. doi: 10.3892/ol.2019.10873
52. Su W, Feng S, Chen X, Yang X, Mao R, Guo C, et al. Silencing of long non-coding RNA MIR22HG triggers cell survival/death signaling via oncogenes YBX1, MET, and p21 in lung cancer. Cancer Res (2018) 78:3207–19. doi: 10.1158/0008-5472.CAN-18-0222
53. Zhang M, Wu J, Zhong W, Zhao Z, Liu Z. Long non-coding RNA BRE-AS1 represses non-small cell lung cancer cell growth and survival via up-regulating NR4A3. Arch Biochem Biophys (2018) 660:53–63. doi: 10.1016/j.abb.2018.09.013
54. Shi R, Jiao Z, Yu A, Wang T. Long noncoding antisense RNA FAM83A-AS1 promotes lung cancer cell progression by increasing FAM83A. J Cell Biochem (2019) 120:10505–12. doi: 10.1002/jcb.28336
55. Chen Z, Hu Z, Sui Q, Huang Y, Zhao M, Li M, et al. LncRNA FAM83A-AS1 facilitates tumor proliferation and the migration via the HIF-1α/ glycolysis axis in lung adenocarcinoma. Int J Biol Sci (2022) 18:522–35. doi: 10.7150/ijbs.67556
56. Xu SF, Zheng Y, Zhang L, Wang P, Niu C, Wu T, et al. Long non-coding RNA LINC00628 interacts epigenetically with the LAMA3 promoter and contributes to lung adenocarcinoma. Mol Ther Nucleic Acids (2019) 18:166–82. doi: 10.1016/j.omtn.2019.08.005
57. Liu T, Xu S, Liu X. LINC00628 is differentially expressed between lung adenocarcinoma and squamous cell carcinoma and is associated with the prognosis of NSCLC. Oncol Lett (2022) 23:55–62. doi: 10.3892/ol.2021.13173
58. Campbell JD, Yau C, Bowlby R, Liu Y, Brennan K, Fan H, et al. Genomic, pathway network, and immunologic features distinguishing squamous carcinomas. Cell Rep (2018) 23:194–212.e6. doi: 10.1016/j.celrep.2018.03.063
59. Gao L, Guo Y, Zeng J, Ma F, Luo J, Zhu H, et al. The expression, significance and function of cancer susceptibility candidate 9 in lung squamous cell carcinoma: A bioinformatics and in vitro investigation. Int J Oncol (2019) 54:1651–64. doi: 10.3892/ijo.2019.4758
Keywords: LUSC, lncRNA, LINC00923, LINC01341, TCGA
Citation: Cui Y, Wu Y, Zhang M, Zhu Y, Su X, Kong W, Zheng X and Sun G (2023) Identification of prognosis-related lncRNAs and cell validation in lung squamous cell carcinoma based on TCGA data. Front. Oncol. 13:1240868. doi: 10.3389/fonc.2023.1240868
Received: 15 June 2023; Accepted: 12 October 2023;
Published: 25 October 2023.
Edited by:
Brian D. Adams, Brain Institute of America, United StatesReviewed by:
Paolo Gandellini, University of Milan, ItalyKannappan Sriramajayam, University of Miami Health System, United States
Copyright © 2023 Cui, Wu, Zhang, Zhu, Su, Kong, Zheng and Sun. This is an open-access article distributed under the terms of the Creative Commons Attribution License (CC BY). The use, distribution or reproduction in other forums is permitted, provided the original author(s) and the copyright owner(s) are credited and that the original publication in this journal is cited, in accordance with accepted academic practice. No use, distribution or reproduction is permitted which does not comply with these terms.
*Correspondence: Guogui Sun, guogui_sun2021@sina.com