- 1Health Management Center, Ningbo Women and Children’s Hospital, Ningbo, China
- 2Department of Internal Medicine, Ningbo Women and Children’s Hospital, Ningbo, China
- 3Department of Obstetrics, Ningbo Women and Children’s Hospital, Ningbo, China
Background: Diabetes has been related to a higher risk of breast cancer (BC) in women. However, it remains unknown whether the incidence of BC is increased in women with prediabetes. A systematic review and meta-analysis was therefore performed to evaluate the relationship between prediabetes and risk of BC.
Methods: Observational studies with longitudinal follow-up relevant to the objective were found via searching Medline, Embase, Cochrane Library, and Web of Science. A fixed- or random-effects model was used to pool the results depending on heterogeneity.
Results: Eight prospective cohort studies and two nest case-control studies were included. A total of 1069079 community women were involved, and 72136 (6.7%) of them had prediabetes at baseline. During a mean duration follow-up of 9.6 years, 9960 (0.93%) patients were diagnosed as BC. Pooled results with a fixed-effects model showed that women with prediabetes were not associated with a higher incidence of BC as compared to those with normoglycemia (risk ratio: 0.99, 95% confidence interval: 0.93 to 1.05, p = 0.72) with mild heterogeneity (p for Cochrane Q test = 0.42, I2 = 3%). Subgroup analyses showed that study characteristics such as study design, menopausal status of the women, follow-up duration, diagnostic criteria for prediabetes, methods for validation of BC cases, and study quality scores did not significantly affect the results (p for subgroup analyses all > 0.05).
Conclusion: Women with prediabetes may not be associated with an increased risk of BC as compared to women with normoglycemia.
Introduction
Breast cancer (BC) is a highly prevalent malignancy among women worldwide, with approximately 1.4 million new diagnoses annually (1, 2). Established risk factors for BC include aging, family history of BC, and reproductive factors such as early menarche, late menopause, late age at first pregnancy, and low parity etc. (3). Early detection of BC is critical in preventing the disease, thus identifying populations at higher risk for its development is imperative (4).
Accumulating evidence suggests that hyperglycemia may have an adverse effect on BC incidence and prognosis (5, 6). A recent meta-analysis with 30 studies showed that patient with type 2 diabetes (T2D) were more likely to be diagnosed with BC as compared to those without T2D (7). Moreover, preexisting T2D has been also suggested to be a risk factor of poor survival of patients with BC (8). In the realm of glycemic metabolism research, the notion of prediabetes has emerged in recent decades as a means of characterizing a state of intermediate hyperglycemia that falls between normoglycemia and diabetes (9). Prediabetes is clinically defined by the presence of impaired glucose tolerance (IGT), impaired fasting glucose (IFG), and mildly elevated glycated hemoglobin (HbA1c) (10). As per established guidelines, IGT is diagnosed when plasma glucose concentrations range from 7.8-11.0 mmol/L after a 2-hour testing period of an oral glucose tolerance test. The definition of IFG is contingent upon the adoption of either the World Health Organization (WHO) or the 2003 American Diabetes Association (ADA) guideline definition, which respectively stipulate fasting plasma glucose (FPG) range of 6.1 to 6.9 mmol/L and 5.6 to 6.9 mmol/L (11). Furthermore, the American Diabetes Association (ADA) and the National Institute for Health and Care Excellence (NICE) also have classified HbA1c levels of 5.7–6.4% or 6.0–6.4% as prediabetic (12, 13). Prior researches have established a correlation between prediabetes and an elevated likelihood of experiencing cardiovascular events (14, 15), akin to the association observed with diabetes. However, the potential connection between prediabetes and an augmented risk of BC remains uncertain. Consequently, in the present study, a systematic review and meta-analysis was carried out to elucidate the relationship between prediabetes and the incidence of BC in female adult population.
Methods
The PRISMA 2020 (16, 17) statement and Cochrane Handbook (18) were followed in this systematic review and meta-analysis.
Database search
In order to identify studies that met the meta-analysis’ objectives, the following terms were combined (1): “prediabetes” OR “pre-diabetes” OR “prediabetic” OR “pre-diabetic” OR “prediabetic state” OR “borderline diabetes” OR “impaired fasting glucose” OR “impaired glucose tolerance” OR “IFG” OR “IGT” OR “fasting glucose” OR “HbA1c” (2); “breast”; and (3) “neoplasms” OR “carcinoma” OR “cancer” OR “tumor” OR “malignancy”. In the search, the dates of databases creation and the date of last search (April 12, 2023) were taken into consideration. Our selection criteria were limited to studies conducted on humans and published in English as full-length papers. Additionally, we manually checked the references of the related original and review articles to identify the original studies that were not included.
Study identification
The PICOS criteria were followed in determining study selection criteria.
(1) P (Participants): Women without a known diagnosis of cancer at baseline.
(2) I (Intervention): Women with prediabetes at baseline. The diagnosis of prediabetes was in accordance with the criteria used in the original studies.
(3) C (Control): Women with normoglycemia at baseline.
(4) O (Outcome): The incidence of BC during follow-up durations, compared between women with prediabetes and women with normoglycemia.
(5) S (Study design): Observational studies that follow patients over time, including cohort studies, post-hoc analyses of clinical trials, and nested case-control studies.
Reviews, meta-analyses, editorials, studies enrolling patients with known cancer at baseline, studies without longitudinal follow-up, studies did not investigate prediabetes as exposure, or studies with no relevant outcomes were excluded.
Study quality assessment and data extraction
For the purpose of assessing the study quality, the Newcastle–Ottawa Scale (NOS) (19) was used, which was composite of three domains involving defining groups of the study, comparing groups between them, and validating outcomes. The NOS incorporates nine criteria, and each study receives one point if it meets a specific criterion. As detailed above, two authors conducted electronic database searches, extracted study data independently, and assessed study quality independently. Disagreements between the two authors should be discussed in order to resolve them. The data collected were (1): study information (authors, countries, publication year, and study design) (2); sources and sample sizes of the included female population, and their mean ages (3); diagnostic criteria for prediabetes and numbers of participants with prediabetes at baseline (4); follow-up durations, number of women who were diagnosed as BC during follow-up, and methods for validating the outcomes; and (5) variables included in the multivariate regression analysis which was used for the analysis of the association between prediabetes and risks of BC.
Statistical methods
Risk ratios (RRs) and 95% confidence intervals (CIs) were used to assess the association between prediabetes and risk of BC. For variance stabilization and normalization, we performed a logarithmical transformation followed by a calculation of the RRs and standard errors (SE) (18). An evaluation of heterogeneity was conducted using the Cochrane Q test and an I2 statistic (20). If I2 > 50%, heterogeneity was considered significant. A fixed-effects model was used to pool the results if heterogeneity among the included studies was not significant; otherwise, a random-effects model was used (18). Sensitivity analysis by excluding one dataset at a time was used to examine the stability of the finding. Subgroup analysis was carried out to evaluate whether the results were significantly affected by predefined study characteristics, such as study design, menopausal status of the women, follow-up duration, diagnostic criteria for prediabetes, methods for validation of BC cases, and study quality scores. In order to reflect publication bias, funnel plots were constructed and symmetry was examined visually. In addition, publication bias was simultaneously evaluated using Egger’s regression asymmetry test (21). The RevMan (Version 5.1; Cochrane Collaboration, Oxford, UK) and Stata (version 12.0; Stata Corporation, College Station, TX) software were employed for the statistical analyses.
Results
Database search results
An overview of the database search process is shown in Figure 1. As a result of the initial literature search, 881 articles were found; after excluding duplications, 709 articles remained. As a result of screening the titles and abstracts, an additional 673 studies were excluded from the meta-analysis. A full-text review was conducted on the remaining 36 studies, of which 26 were further excluded for the reasons listed in Figure 1. As a final step, ten observational studies (22–31) were used for this meta-analysis.
Characteristics of the included studies
Characteristics of the included studies are displayed in Table 1. Overall, eight prospective cohort studies (22–29) and two nest case-control studies (30, 31) were included. These studies were published between 2005 and 2022, and performed in Korea, Austria, the United States, Japan, Sweden, Canada, and the United Kingdom. A total of 1,069,079 community-derived women were included, with the mean ages of 37 to 65 years. Prediabetes was defined as IFG in five studies (22, 23, 25, 26, 28), as IFG and/or IGT in one study (24), and as HbA1c of 5.7~6.4% in four studies (27, 29–31). Accordingly, 72136 (6.7%) of the included participants had prediabetes at baseline. The mean follow-up durations were 6 to 37 years in the studies. During a mean duration follow-up of 9.6 years, 9960 (0.93%) patients were diagnosed as BC. Validation of BC was evidenced via national cancer registries in five studies (22–24, 26, 29), and via medical records in the other five studies (25, 27, 28, 30, 31). Variables such as age, body mass index, smoking, and alcohol drinking were adjusted in the multivariate regression models when the association between prediabetes and the risk of BC was analyzed in each study. A good quality study was indicated by a NOS range of eight to nine stars (Table 2).
Association between prediabetes and the incidence of BC
Since two of the included studies reported data according to the age of the included women, and another two studies according to the menopausal status of the included women separately, these datasets were included in the meta-analysis independently. Overall, 15 datasets from ten studies were available for the meta-analysis (22–31). Mild heterogeneity was observed among the included studies (p for Cochrane Q test = 0.42, I2 = 3%). Pooled results with a fixed-effects model showed that women with prediabetes were not associated with a higher incidence of BC as compared to those with normoglycemia (RR: 0.99, 95% CI: 0.93 to 1.05, p = 0.72; Figure 2). Sensitivity analysis by excluding one dataset at a time showed similar results (RR: 0.97 to 1.01, p all > 0.05; Figure 3). Subgroup analyses showed that study characteristics such as study design (p for subgroup difference = 0.77, Figure 4A), menopausal status of the women (p for subgroup difference = 0.07, Figure 4B), diagnostic criteria for prediabetes (p for subgroup difference = 0.15, Figure 5A), follow-up duration (p for subgroup difference = 0.27, Figure 5B), methods for validation of BC cases (p for subgroup difference = 0.92, Figure 6A), and study quality scores (p for subgroup difference = 0.20, Figure 6B) did not significantly affect the results.
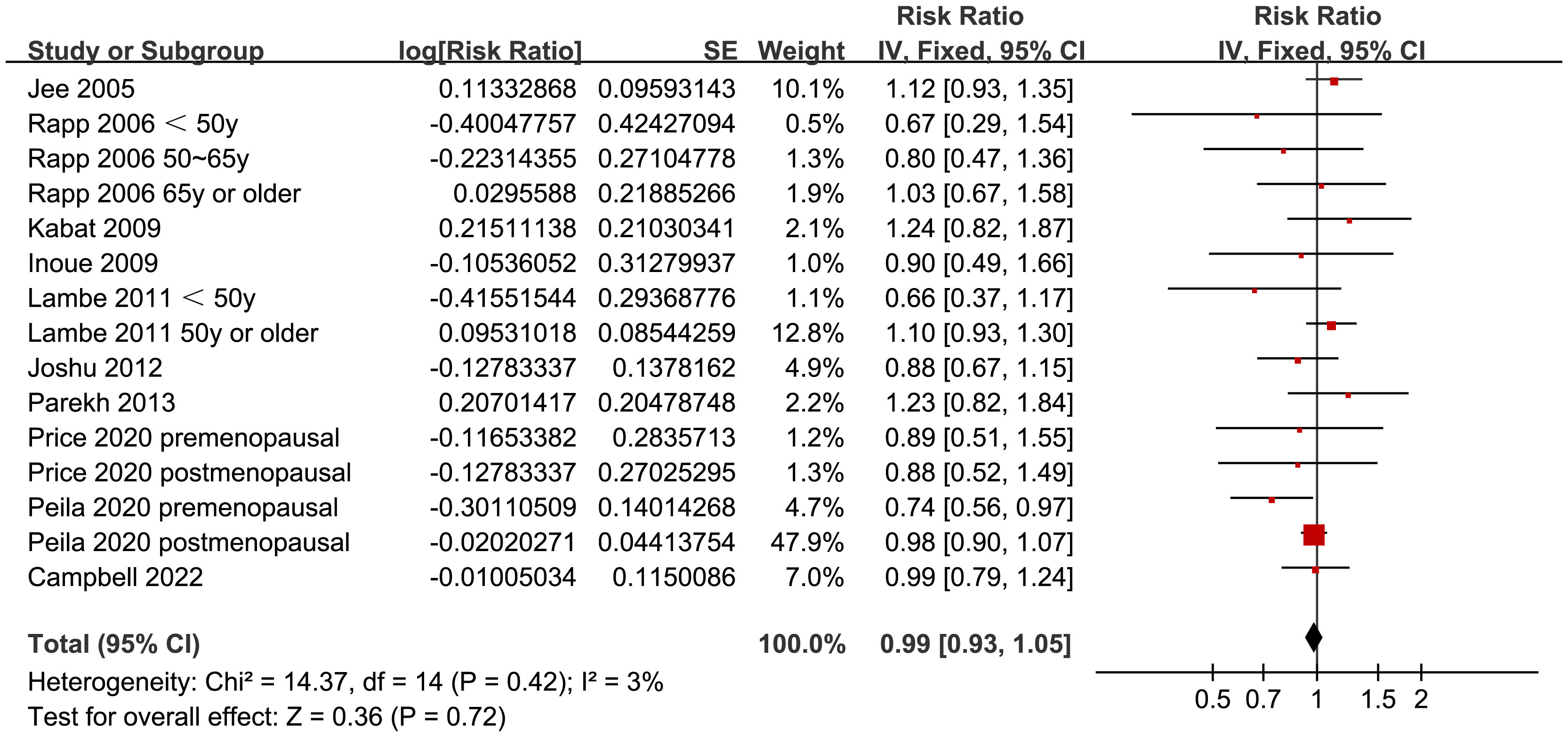
Figure 2 Forest plots for the meta-analysis of the association between prediabetes and the incidence of BC.
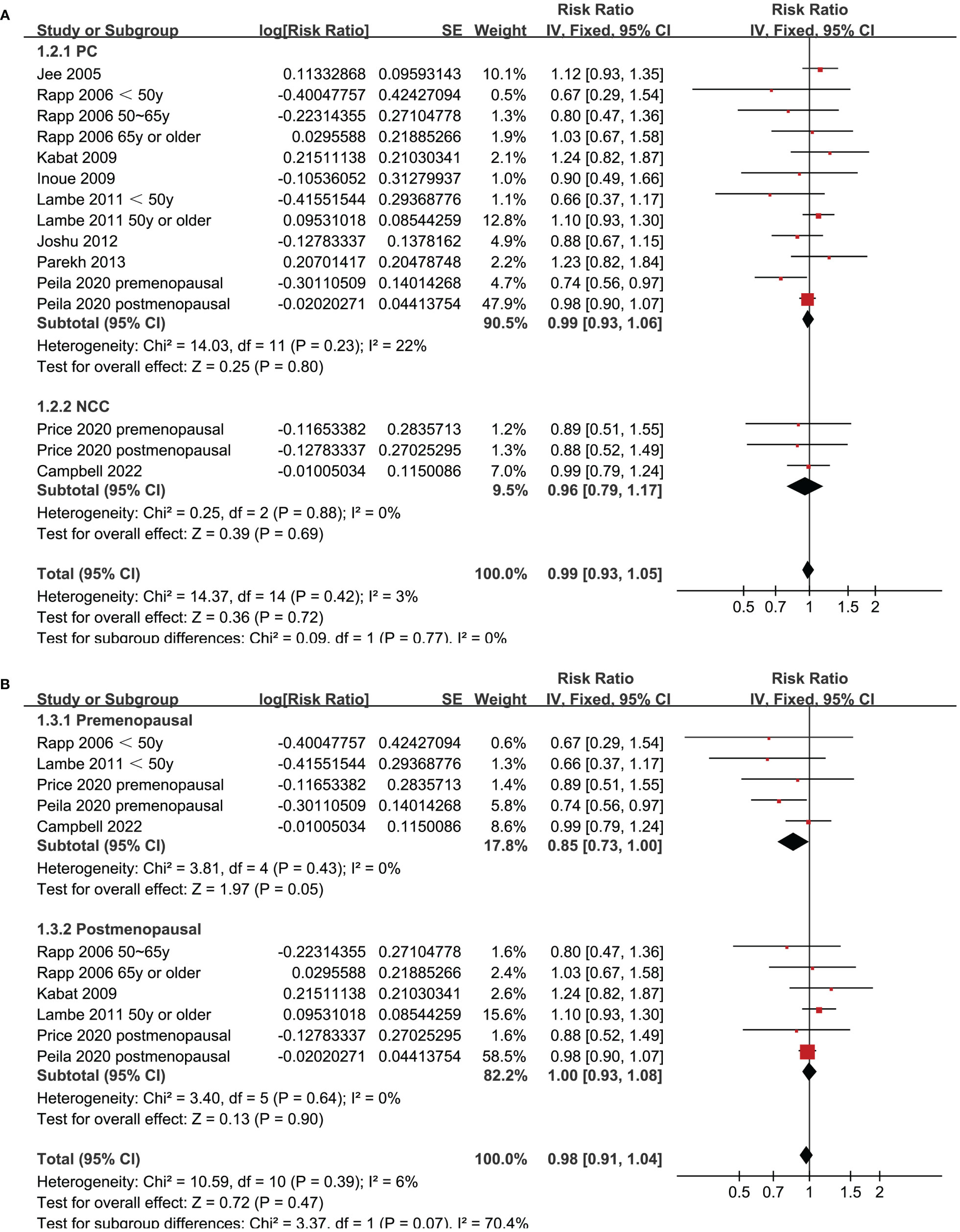
Figure 4 Forest plots for the subgroup analyses of the association between prediabetes and the incidence of BC. (A), subgroup analyses according to study design; and (B), subgroup analyses according to menopausal status of the women.
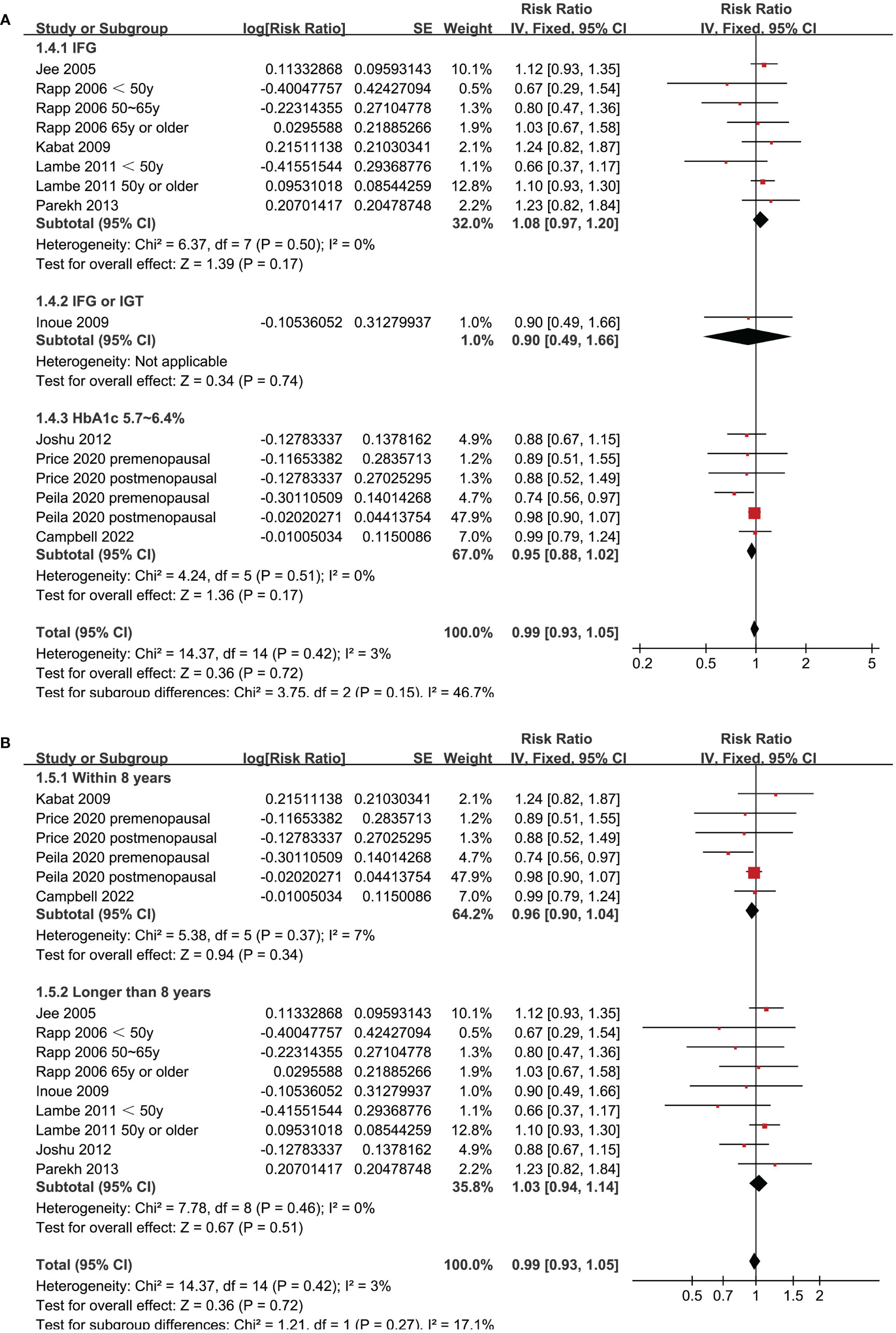
Figure 5 Forest plots for the subgroup analyses of the association between prediabetes and the incidence of BC. (A), subgroup analyses according to definition of prediabetes; and (B), subgroup analyses according to follow-up duration.
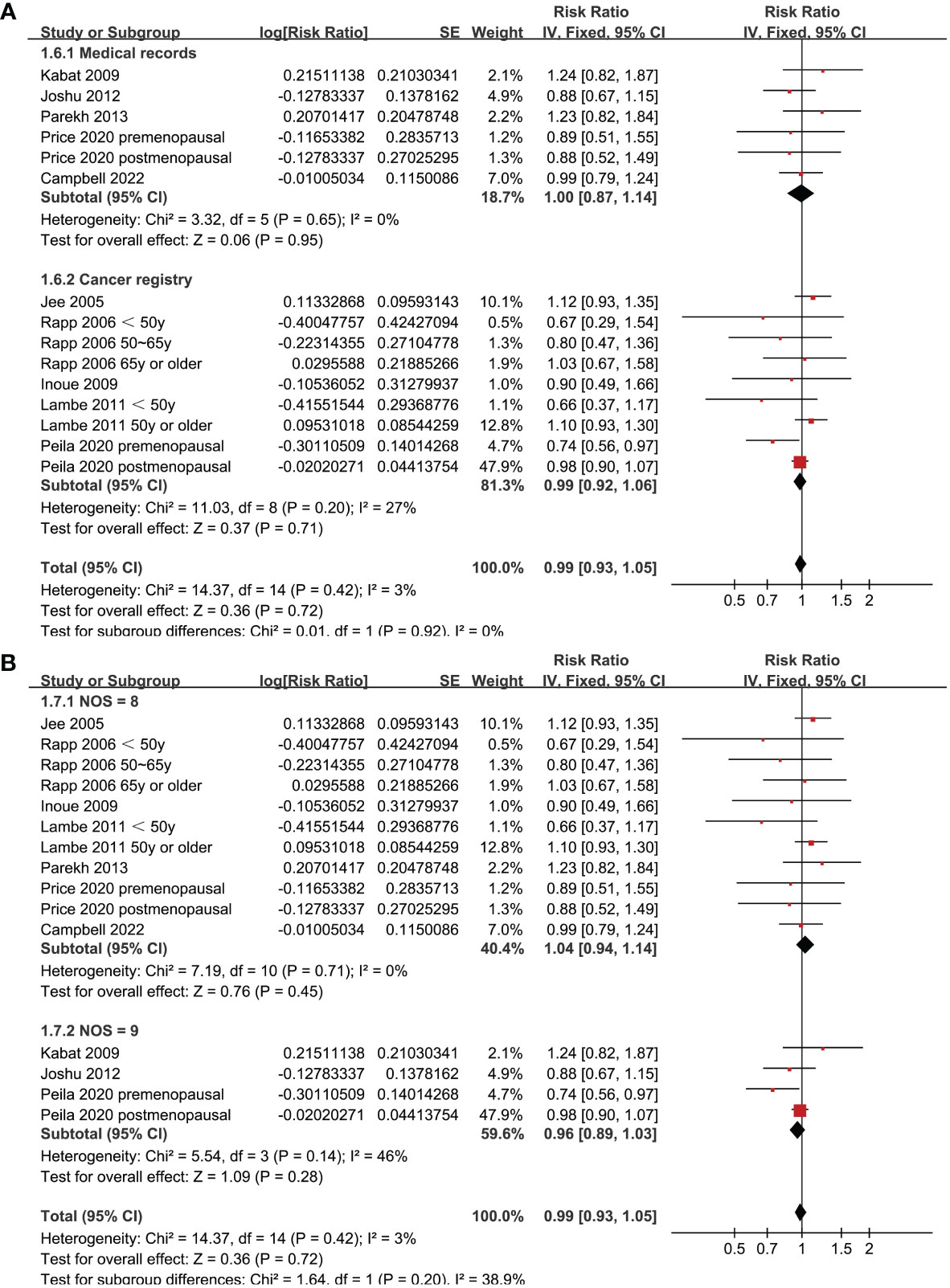
Figure 6 Forest plots for the subgroup analyses of the association between prediabetes and the incidence of BC. (A), subgroup analyses according to methods for validation of BC; and (B), subgroup analyses according to the study quality scores.
Publication bias
Figure 7 shows the funnel plots regarding the association between prediabetes and the incidence of BC. According to visual inspection, the plots are symmetrical, which suggests that high risk of publication bias is unlikely. Additionally, Egger’s regression tests indicated a low risk of publication bias (p = 0.52).
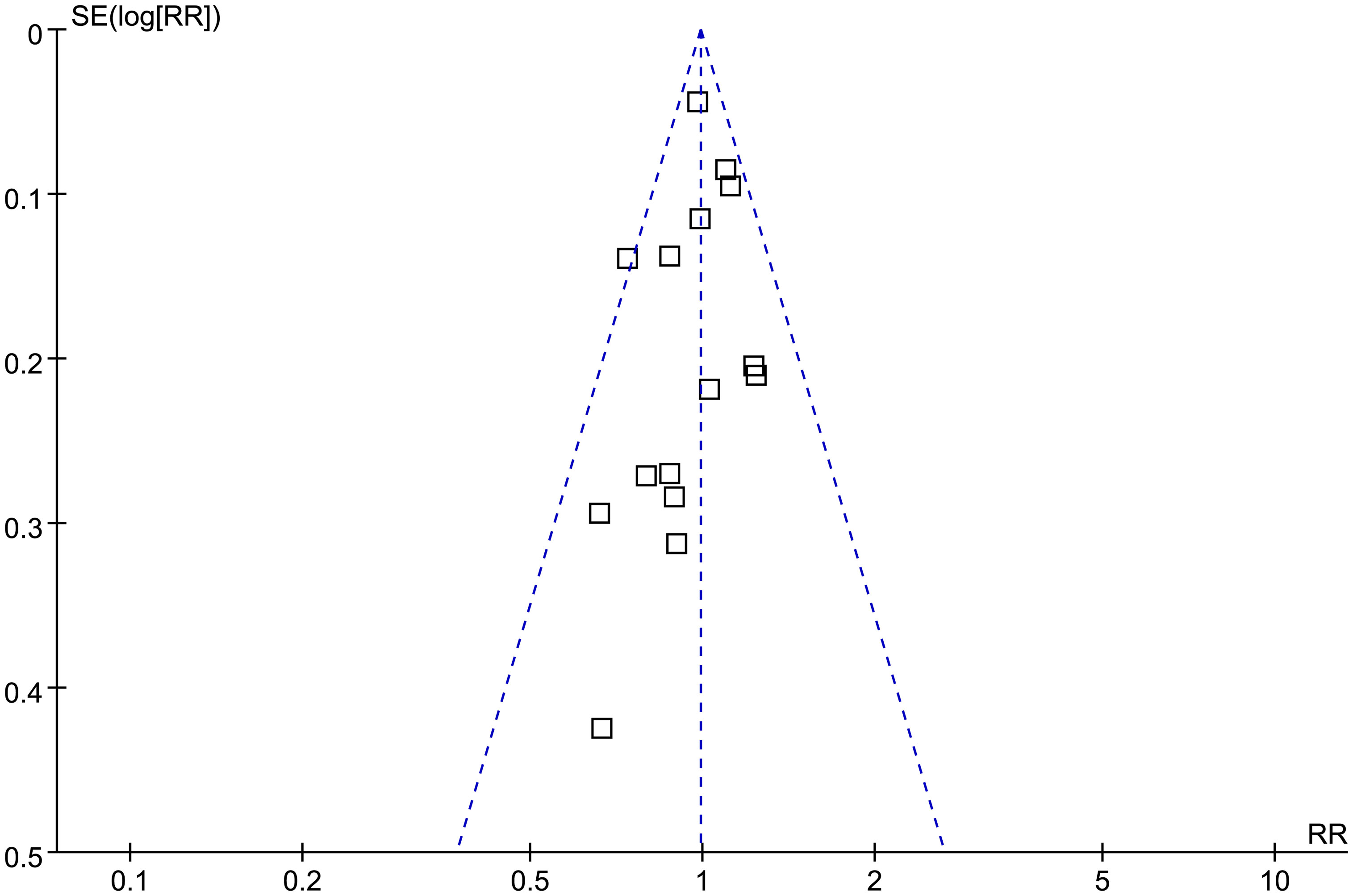
Figure 7 Funnel plots for the publication bias underlying the meta-analysis of the association between prediabetes and the incidence of BC.
Discussion
Based on this meta-analysis, women with prediabetes were shown to be not associated with an increased incidence of BC compared to controls with normoglycemia. Further sensitivity analyses by omitting one study at a time showed consistent results. Subsequent subgroup analyses showed that the results were not significantly by differences of study characteristics such as study design, menopausal status of the women, follow-up durations, definition of prediabetes, methods for the validation of BC cases, or study quality scores. As a result, these results indicate that prediabetes may not be a risk factor of BC in women.
Few previous meta-analyses have evaluated the association between prediabetes and risk of BC. Although an early meta-analysis incorporating the evidence from 16 cohort studies that found that overall prediabetes may be associated with an increased risk of cancer, subsequent subgroup analysis showed that the association may be site-specific according to different cancers (32). As for the subgroup analysis for BC, four cohort studies were included and the pooled results suggest that prediabetes may be associated with a higher risk of BC. However, besides studies reporting the incidence of BC, the authors also included a study that reported BC related mortality, which may confound the results of the meta-analysis (32). Some methodological strength should be noticed in the current systematic review and meta-analysis as compared to the previous one. For example, a comprehensive literature search in four widely used electronic databases was performed, which retrieved ten observational studies according to the aim of the meta-analysis. In addition, only studies reporting BC incidence were included, and studies reporting BC related mortality was excluded. This is important because the two outcomes are not always consistent because BC related mortality could also be influenced by therapeutic factors. Moreover, multivariate regression analyses were used among all of the included studies, and the results were independent of the potential confounding factors such as age, BMI, family history of BC, smoking and alcohol drinking etc. Finally, the stability and robustness of the finding was further confirmed by the consistent results in sensitivity and subgroup analyses. Collectively, results of the meta-analysis suggest that based on the findings from current epidemiological studies, prediabetes may not be a risk factor of BC in women.
Although it is shown in recent studies that the global burdens of premenopausal and postmenopausal BC have been both raising in recent decades, the risk factors of premenopausal and postmenopausal BC could be different (33). In this meta-analysis, a subgroup analysis according to the menopausal status of the women suggested that prediabetes presented a trend of lowered risk of BC in premenopausal women (RR: 0.85, 95% CI: 0.73 to 1.00, p = 0.05), but not in postmenopausal women (RR: 1.00, 95% CI: 0.93 to 1.08, p = 0.90). Although the between-group difference was not statistically significant (p = 0.07), it suggested that prediabetes might be a protective factor for BC in premenopausal women. A similar effect to prediabetes has been suggested by an early study evaluating the influence of metabolic syndrome (MetS) on the risk of BC (34). This meta-analysis included 17 follow-up studies showed that MetS was associated with an increased risk of BC in postmenopausal women, but significantly reduced breast cancer risk in premenopausal women (34). The underlying mechanisms are not clear at current stage (35). From our perspective, this might be explained by the potential role of insulin on ovarian androgen synthesis in premenopausal women. Prediabetes is characterized by hyperinsulinemia and insulin resistance. It is speculated that insulin’s stimulating effect on ovarian androgen synthesis may lead to ovarian hyperandrogenism (36), which in turn may reduce the risk of BC in premenopausal women (37). Large-scale prospective studies are needed to validate the influence of menopausal status on the association between prediabetes and BC, and determined to mechanisms involved.
In addition, subgroup analysis also suggested that the difference of the definition of prediabetes did not significantly affect the association between prediabetes and the risk of BC. Nevertheless, the results should be interpreted cautiously because none of the included studies defined prediabetes as IGT in these studies. In a recent meta-analysis, different definitions and diagnostic criteria were found to affect the association of prediabetes with diabetes risk (38). Therefore, further studies are needed to clarify if different definition and diagnostic criteria for prediabetes could affect the association between prediabetes and BC.
This study also has limitations. First, we could not determine whether the association was consistent across pathological types of BC. In addition, although all selected studies utilized multivariate regression analysis, residual confounding factors could not be excluded, such as the potential influences of dietary and other lifestyle factors that are related to the risk of BC. Finally, as mentioned previously, it remains to determine if difference in menopausal status and definition of prediabetes may affect the results of the meta-analysis.
Conclusion
Based on the meta-analysis, prediabetes may not be associated with an increased incidence of BC in women. However, it remains to be investigated if the conclusion is universal in pre and postmenopausal women, and in prediabetes with different definitions and diagnostic criteria.
Data availability statement
The original contributions presented in the study are included in the article/Supplementary Material. Further inquiries can be directed to the corresponding authors.
Author contributions
JL designed the study. JL and RT performed literature review, study identification, quality evaluation, and data collection. JL and ZL performed statistical analysis and interpreted the results. JL drafted the manuscript. All authors revised the manuscript. All authors contributed to the article and approved the submitted version.
Funding
This study was supported by the Medical and Health Science and Technology Project of Zhejiang Province (2021ZH005) and the Project of Ningbo Leading Medical & Health Discipline, China (No. 2010-S04).
Conflict of interest
The authors declare that the research was conducted in the absence of any commercial or financial relationships that could be construed as a potential conflict of interest.
Publisher’s note
All claims expressed in this article are solely those of the authors and do not necessarily represent those of their affiliated organizations, or those of the publisher, the editors and the reviewers. Any product that may be evaluated in this article, or claim that may be made by its manufacturer, is not guaranteed or endorsed by the publisher.
References
1. Siegel RL, Miller KD, Wagle NS, Jemal A. Cancer statistics, 2023. CA Cancer J Clin (2023) 73(1):17–48. doi: 10.3322/caac.21763
2. Tao X, Li T, Gandomkar Z, Brennan PC, Reed WM. Incidence, mortality, survival, and disease burden of breast cancer in China compared to other developed countries. Asia Pac J Clin Oncol (2023). doi: 10.1111/ajco.13958
3. Sun YS, Zhao Z, Yang ZN, Xu F, Lu HJ, Zhu ZY, et al. Risk factors and preventions of breast cancer. Int J Biol Sci (2017) 13(11):1387–97. doi: 10.7150/ijbs.21635
4. Pashayan N, Antoniou AC, Ivanus U, Esserman LJ, Easton DF, French D, et al. Personalized early detection and prevention of breast cancer: ENVISION consensus statement. Nat Rev Clin Oncol (2020) 17(11):687–705. doi: 10.1038/s41571-020-0388-9
5. Kang C, LeRoith D, Gallagher EJ. Diabetes, obesity, and breast cancer. Endocrinology (2018) 159(11):3801–12. doi: 10.1210/en.2018-00574
6. Qiu J, Zheng Q, Meng X. Hyperglycemia and chemoresistance in breast cancer: from cellular mechanisms to treatment response. Front Oncol (2021) 11:628359. doi: 10.3389/fonc.2021.628359
7. Lu Y, Hajjar A, Cryns VL, Trentham-Dietz A, Gangnon RE, Heckman-Stoddard BM, et al. Breast cancer risk for women with diabetes and the impact of metformin: A meta-analysis. Cancer Med (2022) 12(10):11703–18. doi: 10.1002/cam4.5545
8. Zhao XB, Ren GS. Diabetes mellitus and prognosis in women with breast cancer: A systematic review and meta-analysis. Med (Baltimore). (2016) 95(49):e5602. doi: 10.1097/MD.0000000000005602
9. Tabak AG, Herder C, Rathmann W, Brunner EJ, Kivimaki M. Prediabetes: a high-risk state for diabetes development. Lancet (2012) 379(9833):2279–90. doi: 10.1016/S0140-6736(12)60283-9
10. Echouffo-Tcheugui JB, Perreault L, Ji L, Dagogo-Jack S. Diagnosis and management of prediabetes: A review. JAMA (2023) 329(14):1206–16. doi: 10.1001/jama.2023.4063
11. Hostalek U. Global epidemiology of prediabetes - present and future perspectives. Clin Diabetes Endocrinol (2019) 5:5. doi: 10.1186/s40842-019-0080-0
12. Chatterton H, Younger T, Fischer A, Khunti K. Risk identification and interventions to prevent type 2 diabetes in adults at high risk: summary of NICE guidance. BMJ (2012) 345:e4624. doi: 10.1136/bmj.e4624
13. American Diabetes Association Professional Practice Committee. 2. Classification and diagnosis of diabetes: standards of medical care in diabetes-2019. Diabetes Care (2019) 42(Suppl 1):S13–28. doi: 10.2337/dc22-S002
14. Cai X, Zhang Y, Li M, Wu JH, Mai L, Li J, et al. Association between prediabetes and risk of all cause mortality and cardiovascular disease: updated meta-analysis. BMJ (2020) 370:m2297. doi: 10.1136/bmj.m2297
15. Schlesinger S, Neuenschwander M, Barbaresko J, Lang A, Maalmi H, Rathmann W, et al. Prediabetes and risk of mortality, diabetes-related complications and comorbidities: umbrella review of meta-analyses of prospective studies. Diabetologia (2022) 65(2):275–85. doi: 10.1007/s00125-021-05592-3
16. Page MJ, McKenzie JE, Bossuyt PM, Boutron I, Hoffmann TC, Mulrow CD, et al. The PRISMA 2020 statement: an updated guideline for reporting systematic reviews. BMJ (2021) 372:n71. doi: 10.1136/bmj.n71
17. Page MJ, Moher D, Bossuyt PM, Boutron I, Hoffmann TC, Mulrow CD, et al. PRISMA 2020 explanation and elaboration: updated guidance and exemplars for reporting systematic reviews. BMJ (2021) 372:n160. doi: 10.1136/bmj.n160
18. Higgins J, Thomas J, Chandler J, Cumpston M, Li T, Page M, et al. Cochrane Handbook for Systematic Reviews of Interventions version 6.2. The Cochrane Collaboration, London, UK (2021). Available at: www.training.cochrane.org/handbook.
19. Wells GA, Shea B, O’Connell D, Peterson J, Welch V, Losos M, et al. The Newcastle-Ottawa Scale (NOS) for assessing the quality of nonrandomised studies in meta-analyses (2010). Available at: http://www.ohri.ca/programs/clinical_epidemiology/oxford.asp.
20. Higgins JP, Thompson SG. Quantifying heterogeneity in a meta-analysis. Stat Med (2002) 21(11):1539–58. doi: 10.1002/sim.1186
21. Egger M, Davey Smith G, Schneider M, Minder C. Bias in meta-analysis detected by a simple, graphical test. BMJ (1997) 315(7109):629–34. doi: 10.1136/bmj.315.7109.629
22. Jee SH, Ohrr H, Sull JW, Yun JE, Ji M, Samet JM. Fasting serum glucose level and cancer risk in Korean men and women. JAMA (2005) 293(2):194–202. doi: 10.1001/jama.293.2.194
23. Rapp K, Schroeder J, Klenk J, Ulmer H, Concin H, Diem G, et al. Fasting blood glucose and cancer risk in a cohort of more than 140,000 adults in Austria. Diabetologia (2006) 49(5):945–52. doi: 10.1007/s00125-006-0207-6
24. Inoue M, Noda M, Kurahashi N, Iwasaki M, Sasazuki S, Iso H, et al. Impact of metabolic factors on subsequent cancer risk: results from a large-scale population-based cohort study in Japan. Eur J Cancer Prev (2009) 18(3):240–7. doi: 10.1097/CEJ.0b013e3283240460
25. Kabat GC, Kim M, Chlebowski RT, Khandekar J, Ko MG, McTiernan A, et al. A longitudinal study of the metabolic syndrome and risk of postmenopausal breast cancer. Cancer Epidemiol Biomarkers Prev (2009) 18(7):2046–53. doi: 10.1158/1055-9965.EPI-09-0235
26. Lambe M, Wigertz A, Garmo H, Walldius G, Jungner I, Hammar N. Impaired glucose metabolism and diabetes and the risk of breast, endometrial, and ovarian cancer. Cancer Causes Control. (2011) 22(8):1163–71. doi: 10.1007/s10552-011-9794-8
27. Joshu CE, Prizment AE, Dluzniewski PJ, Menke A, Folsom AR, Coresh J, et al. Glycated hemoglobin and cancer incidence and mortality in the Atherosclerosis in Communities (ARIC) Study, 1990-2006. Int J Cancer. (2012) 131(7):1667–77. doi: 10.1002/ijc.27394
28. Parekh N, Lin Y, Vadiveloo M, Hayes RB, Lu-Yao GL. Metabolic dysregulation of the insulin-glucose axis and risk of obesity-related cancers in the Framingham heart study-offspring cohort (1971-2008). Cancer Epidemiol Biomarkers Prev (2013) 22(10):1825–36. doi: 10.1158/1055-9965.EPI-13-0330
29. Peila R, Rohan TE. Diabetes, glycated hemoglobin, and risk of cancer in the UK biobank study. Cancer Epidemiol Biomarkers Prev (2020) 29(6):1107–19. doi: 10.1158/1055-9965.EPI-19-1623
30. Price TR, Friedenreich CM, Robson PJ, Li H, Brenner DR. High-sensitivity C-reactive protein, hemoglobin A1c and breast cancer risk: a nested case-control study from Alberta’s Tomorrow Project cohort. Cancer Causes Control. (2020) 31(12):1057–68. doi: 10.1007/s10552-020-01329-6
31. Campbell PT, Newton CC, Jacobs EJ, McCullough ML, Wang Y, Rees-Punia E, et al. Prospective associations of hemoglobin A(1c) and c-peptide with risk of diabetes-related cancers in the Cancer Prevention Study-II Nutrition Cohort. Cancer Res Commun (2022) 2(7):653–62. doi: 10.1158/2767-9764.CRC-22-0082
32. Huang Y, Cai X, Qiu M, Chen P, Tang H, Hu Y. Prediabetes and the risk of cancer: a meta-analysis. Diabetologia (2014) 57(11):2261–9. doi: 10.1007/s00125-014-3361-2
33. Heer E, Harper A, Escandor N, Sung H, McCormack V, Fidler-Benaoudia MM. Global burden and trends in premenopausal and postmenopausal breast cancer: a population-based study. Lancet Glob Health (2020) 8(8):e1027–e37. doi: 10.1016/S2214-109X(20)30215-1
34. Guo M, Liu T, Li P, Wang T, Zeng C, Yang M, et al. Association between metabolic syndrome and breast cancer risk: an updated meta-analysis of follow-up studies. Front Oncol (2019) 9:1290. doi: 10.3389/fonc.2019.01290
35. Lian K, Feng H, Liu S, Wang K, Liu Q, Deng L, et al. Insulin quantification towards early diagnosis of prediabetes/diabetes. Biosens Bioelectron. (2022) 203:114029. doi: 10.1016/j.bios.2022.114029
36. Xu Y, Qiao J. Association of insulin resistance and elevated androgen levels with polycystic ovarian syndrome (PCOS): A review of literature. J Healthc Eng. (2022) 2022:9240569. doi: 10.1155/2022/9240569
37. Clendenen TV, Ge W, Koenig KL, Afanasyeva Y, Agnoli C, Brinton LA, et al. Breast cancer risk prediction in women aged 35-50 years: impact of including sex hormone concentrations in the Gail model. Breast Cancer Res (2019) 21(1):42. doi: 10.1186/s13058-019-1126-z
38. Lee CMY, Colagiuri S, Woodward M, Gregg EW, Adams R, Azizi F, et al. Comparing different definitions of prediabetes with subsequent risk of diabetes: an individual participant data meta-analysis involving 76 513 individuals and 8208 cases of incident diabetes. BMJ Open Diabetes Res Care (2019) 7(1):e000794. doi: 10.1136/bmjdrc-2019-000794
Keywords: breast cancer, prediabetes, incidence, risk factor, meta-analysis
Citation: Lin J, Tu R and Lu Z (2023) Prediabetes and the risk of breast cancer: a meta-analysis. Front. Oncol. 13:1238845. doi: 10.3389/fonc.2023.1238845
Received: 12 June 2023; Accepted: 31 August 2023;
Published: 18 September 2023.
Edited by:
Maria Rosaria De Miglio, University of Sassari, ItalyReviewed by:
Hojat Dehghanbanadaki, Tehran University of Medical Sciences, IranSubhash Kumar Tripathi, Seattle Children’s Research Institute, United States
Copyright © 2023 Lin, Tu and Lu. This is an open-access article distributed under the terms of the Creative Commons Attribution License (CC BY). The use, distribution or reproduction in other forums is permitted, provided the original author(s) and the copyright owner(s) are credited and that the original publication in this journal is cited, in accordance with accepted academic practice. No use, distribution or reproduction is permitted which does not comply with these terms.
*Correspondence: Jing Lin, amluZ2xpbl9uYndjQDIxY24uY29t; Zhai’e Lu, bHV6aGFpZV9uYndjQDIxY24uY29t