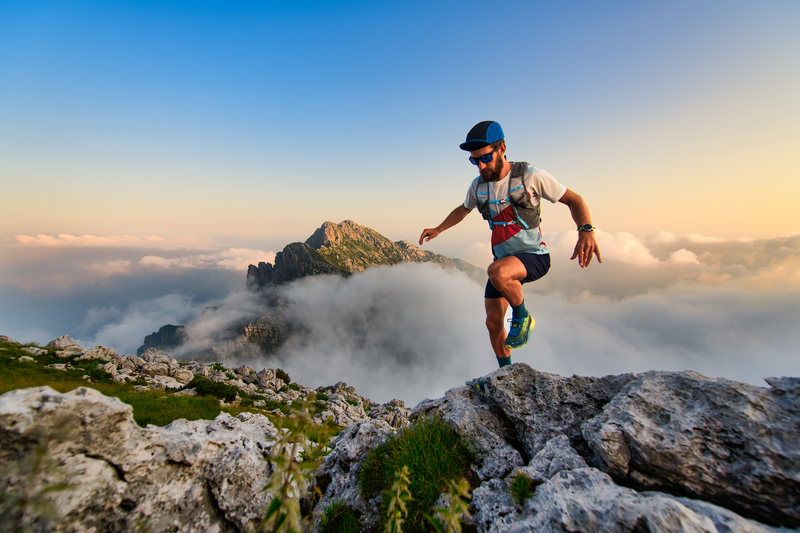
95% of researchers rate our articles as excellent or good
Learn more about the work of our research integrity team to safeguard the quality of each article we publish.
Find out more
ORIGINAL RESEARCH article
Front. Oncol. , 30 August 2023
Sec. Cancer Molecular Targets and Therapeutics
Volume 13 - 2023 | https://doi.org/10.3389/fonc.2023.1238613
Introduction: Lymphoma is a common canine cancer with translational relevance to human disease. Diffuse large B-cell lymphoma (DLBCL) is the most frequent subtype, contributing to almost fifty percent of clinically recognized lymphoma cases. Identifying new biomarkers capable of early diagnosis and monitoring DLBCL is crucial for enhancing remission rates. This research seeks to advance our knowledge of the molecular biology of DLBCL by analyzing the expression of microRNAs, which regulate gene expression by negatively impacting gene expression via targeted RNA degradation or translational repression. The stability and accessibility of microRNAs make them appropriate biomarkers for the diagnosis, prognosis, and monitoring of diseases.
Methods: We extracted and sequenced microRNAs from ten fresh-frozen lymph node tissue samples (six DLBCL and four non-neoplastic).
Results: Small RNA sequencing data analysis revealed 35 differently expressed miRNAs (DEMs) compared to controls. RT-qPCR confirmed that 23/35 DEMs in DLBCL were significantly upregulated (n = 14) or downregulated (n = 9). Statistical significance was determined by comparing each miRNA's average expression fold-change (2-Cq) between the DLCBL and healthy groups by applying the unpaired parametric Welch's 2-sample t-test and false discovery rate (FDR). The predicted target genes of the DEMs were mainly enriched in the PI3K-Akt-MAPK pathway.
Discussion: Our data point to the potential value of miRNA signatures as diagnostic biomarkers and serve as a guideline for subsequent experimental studies to determine the targets and functions of these altered miRNAs in canine DLBCL.
Lymphomas are a complex, heterogeneous group of hematopoietic malignancies with similarities between dogs and humans (1), accounting for 7- 24% of all cancers in dogs and approximately 83% of all hematopoietic cancers (2). Canine lymphomas microscopically resemble some forms of non-Hodgkin lymphomas in humans and respond similarly to standard treatments (3). Diffuse large B-cell lymphoma (DLBCL) is humans’ most frequent aggressive lymphoma subtype, representing almost 30% of NHL cases (4). DLBCL is also the most reported subtype of lymphoma in dogs, followed by peripheral T-cell lymphoma not otherwise specified, nodal T-zone lymphoma, T-lymphoblastic lymphoma, and marginal zone lymphoma (5).
Several approaches are used to treat DLBCL, depending on the cancer stage and response to treatment. CHOP-based chemotherapy (cyclophosphamide, doxorubicin, vincristine, prednisone) (6, 7) is the standard treatment for DLBCL, with overall survival times varying from 10 to 14 months (7). CHOP therapy is not curative; but diminishes the cancer burden, resulting in partial to complete remission (8). Nevertheless, lymphoma patients eventually experience cancer relapse, resulting from the inability of chemotherapy to eradicate the subclinical disease. Earlier detection of DLCBL may improve the chances of therapeutic success. Thus, there is a continuous search for additional biomarkers capable of early detecting and monitoring responses to treatment in dogs with DLBCL.
The diagnosis of DLBCL relies on histologic evaluation of a tissue biopsy in conjunction with immunohistochemical (IHC) or flow cytometry analysis (9). This workflow is sufficient to confirm the diagnosis; however, it may be challenging to differentiate reactive lymph nodes from DLCBL in some cases (9). PCR for antigen receptor rearrangements (PARR) assays to determine clonality assays aid in a more accurate diagnosis (10); however, the workflow becomes laborious and expensive. A diagnostic test capable of detecting DLBCL and identifying prognostically relevant patient subgroups would substantially simplify the ability to diagnose and treat DLBCL correctly.
This study aims to broaden our understanding of the molecular biology of canine DLBCL by investigating the role of gene expression regulators called microRNAs (miRNA) as possible diagnostic biomarkers in DLBCL. MicroRNAs are small non-coding RNAs (11) that negatively modulate gene expression (12–14); they are incorporated into the RNA silencing complex and, in the case of perfect complementarity at the 3’ untranslated region with the mRNA target, induce targeted mRNA degradation (15). In the case of imperfect complementarity, miRNAs may block gene expression at the translational level (13, 16–19). One miRNA can target various genes, including transcriptional activators and repressors, thereby regulating physiological and pathological processes (18, 20). Mature miRNAs are abundant in the cell cytoplasm and circulate extracellularly in bodily fluids such as plasma and serum (21, 22) packaged in lipoprotein complexes, which protect their degradation by ribonucleases (23, 24), permitting their uptake by distant cells, where they also contribute to cell-to-cell communication (17, 25).
Aberrant expression of miRNA has been seen in numerous diseases, including cancers (17, 26, 27). Most miRNA genes occupy mutable sites within the genome. These regions are also enriched in other cancer-related genes (26, 28); consequently, miRNAs have drawn considerable attention as potential cancer biomarkers. MicroRNAs can be used as diagnostic and prognostic biomarkers to detect disease subtypes (29, 30). They can also be measured sequentially to evaluate the evolution in disease status over time (30–33) or the response to treatment (34), as they play crucial roles in regulating cancer progression.
Compared to healthy dogs, the miRNA profile of the canine DLBCL-affected dogs is not thoroughly studied. This study investigates the differentially expressed miRNAs in canine DLBCL using fresh frozen lymph node samples from six DLBCL dog patients and four normal healthy dogs. This study enhances our understanding of the canine DLBCL miRNAs’ signature profile, which could be a valuable tool in disease detection and progression in dogs. This study also improves our understanding of the disease mechanism, molecular pathways, biomarkers discovery, dysregulated miRNAs, and personalized medicine.
Ten fresh-frozen lymph node biopsy specimens were used in this study. Six of these samples were affected by DLBCL, and 4 were histologically normal lymph nodes. Dogs with DLBCL were presented to the Purdue University Veterinary Teaching Hospital (PUVTH), West Lafayette, IN, from 2013 to 2014 for cancer diagnosis, staging, and treatment. All samples from dogs with DLCBL were collected by surgical biopsy of an affected peripheral lymph node (incisional wedge biopsy or surgical extirpation of an entire lymph node) while the dogs were under general anesthesia. The diagnosis of DLBCL was made by integrating histopathology and immunohistochemistry results confirming the expression of CD79a and/or Pax-5 and lack of expression of CD3 by the neoplastic cells. The controls used in the study were four fresh-frozen, non-neoplastic lymph nodes collected from purpose-bred research dogs from a commercial vendor (Covance Research Products, Inc.). with no substantial clinical, hematologic, or biochemical abnormalities. These dogs were humanely euthanatized in academic surgical laboratory courses taught to veterinary students at the Purdue College of Veterinary Medicine. Animal medical history and test results of DLBCL cases are provided (Supplementary Table 1A). The demographic data of healthy cases are demonstrated (Supplementary Table 1B). The samples were evaluated and classified by a board-certified pathologist according to the WHO criteria of 2008. All the samples for this project were collected with informed consent from the dogs’ owners, and procedures for sample collection were approved by the Purdue Animal Care and Use Committee (protocols #1708001607 and #1111000308). All lymph node samples were frozen in liquid nitrogen immediately after harvesting and stored at -80°C until total miRNA extraction.
RNA extraction was performed using the Monarch® Total RNA Miniprep Kit (NEB, Ipswich, MA, USA). Briefly, the fresh-frozen samples were quickly thawed in an equal volume of the Monarch® DNA/RNA Protection Reagent and homogenized using a vortex. Then, 10 µL of Proteinase K was added to the mixture. After a brief vortex, the samples were incubated at room temperature for 30 minutes; then, an equal amount of isopropanol was added, followed by a quick vortex. The mixture was passed through an RNA purification column and washed twice using a 500 µL RNA Wash Buffer. Subsequently, DNase I treatment was used to remove residual gDNA. A 500 µL RNA Priming Buffer was added for RNA binding, followed by a few washing steps. Afterward, the total RNA was eluted with 100 µL nuclease-free water, and the RNA samples were stored at -80°C. Before sequencing, the quantity and quality of total RNA were assessed by UV® spectrophotometry (NanoDrop, ThermoFisher Scientific, Waltham, MA, USA). The quantification of small RNAs was assisted by a spectrofluorometer (Qubit 4 Fluorometer, ThermoFisher Scientific, Waltham, MA, USA) using Invitrogen™ Qubit™ microRNA Assay Kits (ThermoFisher Scientific, Waltham, MA, USA) as demonstrated (Supplementary Table 2).
The extracted total RNA from each sample was sequenced (Novogene Co., Ltd., Beijing, China). RNA degradation and contamination were examined on 1% agarose gels. RNA purity was tested utilizing the Nanophotometer® UV spectrophotometer (IMPLEN, Westlake Village, CA, USA). RNA integrity and quantification were measured utilizing the RNA Nano 6000 Assay Kit of the Agilent Bioanalyzer 2100 system (Agilent Technologies, Santa Clara, CA, USA).
The small RNA library construction used 3 μg of total RNA per sample. Following the manufacturer’s recommendations, sequencing libraries were produced using NEBNext® Multiplex Small RNA Library Prep Set (NEB, Ipswich, MA, USA) for Illumina®, and index codes were added to allow attribution of sequences to samples. First-strand cDNA was performed using M-MuLV Reverse Transcriptase (RNase H–), PCR amplification using LongAmp Taq 2X Master Mix, SR Primer for Illumina, and index (X) primer. The purified PCR products were examined on an 8% polyacrylamide gel (100V, 80 min). DNA fragments equivalent to 140~160 bp (the length of small non-coding RNA plus the 3’ and 5’ adaptors) were excised and suspended in an eight μL elution buffer. Lastly, library quality was evaluated using DNA High Sensitivity Chips on the Agilent Bioanalyzer 2100 system (Agilent Technologies, Santa Clara, CA, USA).
Customized Perl (https://www.perl.org/get.html) and Python (https://www.python.org/downloads/) scripts were used to analyze the raw fastq files. The fraction of reads with < 1% (Q20) and 0.1% (Q30) error, GC-content, were calculated after cleaning up reads with ploy-N, 5’ adapter contaminants without 3’ adapter, or the insert tag, in addition to reads containing poly A, T, G, C, or having low-quality as shown (Supplementary Tables 3, 4).
The small RNA sequences were mapped to the reference sequence using Bowtie 0.12.9 (35), as shown (Supplementary Table 5). For known miRNAs, miRBase20.0 and modified software miRDeep2_0_0_5 were used as a reference (36). Small RNA tags were mapped to RepeatMasker open-4.0.3 and Rfam (37) databases to remove tags originating from repetitive sequences, rRNA, tRNA, snRNA, and snoRNA. The software package miRDeep2 (36) and the characteristics of the hairpin structure of miRNA precursor were integrated to predict potential novel miRNAs.
Expression levels were assessed as transcripts per million (TPM) using the following criteria (38): (1) Normalization formula: Normalized expression = mapped read count/total reads*1000000. (2) Differential expression analysis of the two groups (DLBCL vs. healthy) is displayed (Supplementary Table 6) and was achieved using the DESeq2 v1.12.0 and R package (1.8.3) (39). The False Discovery Rate (FDR) rate was controlled by adjusting the P-values using the Benjamini & Hochberg (BH) method implemented in DESeq2. Adjusted P-values of <0.05 and the fold change of > 2 were used as the threshold for significantly differential expression. Predicting the target gene of miRNA was performed by miRanda3.3a (40).
Gene Ontology (GO) enrichment analysis was used to identify conserved biological processes, molecular functions, and cellular compartments related to the predicted gene targets of the differentially expressed miRNAs (DEMs). The GOseq R package (Release 2.12) based on Wallenius non-central hypergeometric distribution (41) and adjusted P-values BH of <0.05, which adjusts for gene length bias, was implemented for enrichment analysis.
Over-represented pathways were identified based on the predicted target genes of the DEMs and pathways defined in the Kyoto Encyclopedia of Genes and Genomes (KEGG) (41). KOBAS V3.0 software was used to test the statistical enrichment of the target gene candidates in KEGG pathways (42). To graphically visualize the enrichment pathway, ShinyGO 0.75 (43) was used to annotate pathways from multiple resources: GO, KEGG, Reactome (44, 45), PANTHER (46), and WikiPathways (47). The KEGG diagram was retrieved using KEGG and path view (48, 49).
Following the manufacturer’s protocol, the first-strand cDNA synthesis was performed using the miRCURY LNA RT Kit (QIAGEN, Germantown, MD, USA). UniSp6 RNA spike-in, the reverse transcription control, was diluted and added to the mix as instructed. Total RNA was diluted to 50 ng/μL in RNase−free water. The total volume of the reaction mixture (10 μL) was kept on ice to avoid RNA degradation. The reaction mixture consisted of 2 μL of 5x miRCURY SYBR® Green Reaction Buffer, 4.5 μl RNase-free Water, 1 μL of 10x miRCURY RT Enzyme Mix, 2 μL of the diluted RNA, and 0.5 μL of RNA synthetic spike−in (UniSp6). Reverse-transcription thermal cycling was performed as instructed: incubation for 1 h at 42°C, followed by reverse transcriptase enzyme inactivation for 5 min at 95°C, then immediate cooling to 4°C. The synthesized cDNA was diluted, and quantitative RT-PCR was performed on a QuantStudio3 PCR system (ThermoFisher Scientific, Waltham, MA, USA). The miRCURY LNA (50) miRNA Custom PCR Panel (QIAGEN, Germantown, MD, USA) was used to quantify the pre-selected miRNAs (Table 1). Each sample was measured on a separate plate; in each plate, 44 miRNAs, RNUB6, UniSp6 (cDNA synthesis control), UniSp3 (RT-qPCR positive control and inter-plate calibrator (IPC), and non-template control (negative control) were measured. The cDNA dilution was 1:80, according to the manufacturer’s instructions. Relative miRNA expression levels
RT-qPCR normalization for reducing technical variation was performed to validate the were calculated by the Livak method 2-ΔΔcq (51).
The applied criteria in selecting reference candidates for normalizing the exported calibrated quantification cycles (Cq) are shown (Supplementary Table 7). First, a set of miRNA candidates was selected manually based on the lowest coefficient of variation (CV%) (52, 53), followed by using RefFinder (https://www.heartcure.com.au/reffinder/) (54). This web-based tool uses an ensemble approach based on several computational algorithms (geNorm (55), NormFinder (56), the comparative Delta-Cq method (57), and BestKeeper (58)) to rank potential reference miRNAs according to their stability of expression as demonstrated. Each program ranks and ascribes weights to the tested miRNAs. The geometric mean of those weights is calculated and used as the final weight (Table 2).
All statistical analyses were performed using R version 4.1.2. Unpaired parametric Welch’s 2- sample t-test was used to explore the differences in the fold-change (2-ΔΔcq) in the expression of each miRNA in the DLCBL and healthy groups. To address the multiple testing problem, which results in increased false-positive rates, P-values were corrected (59) using Benjamini–Hochberg (FDR) with P-values treated according to their ranks (60). The adjusted P-values less than 0.05 were used to indicate significance.
The mean concertation of the total RNA extracted was 1354.33 ± 759.65 ng/µL measured by UV spectrophotometer. The ratio 260/280nm absorbance ratio was 2.1 ± 0.01 (Supplementary Table 2).
The mean number of sRNA reads was 475,87,913, with an average of 96.75% of sRNA retained after quality trimming and adapter removal for all samples. A summary of the overall cleaning process and mapping statistics of the reads are shown (Supplementary Table 5). A total of 320 miRNAs was detected and the cluster analysis of all the differentially expressed miRNAs from the healthy and DLBCL samples are shown on the criteria of a fold change ≥2 or ≤- 2 and p-value <0.05 and presented in a heat map (Figure 1). Twenty-four miRNAs were upregulated, and 11 downregulated in DLBCL relative to normal lymph nodes (35 total DEMs) are presented in a volcano blot (Figure 2).
Figure 1 sRNA-Seq heatmap of the expressed miRNAs (DEMs). Red represents high expression; blue represents low expression. The color intensity from red to blue represents the log10 (TPM+1) value from large to small.
Figure 2 sRNA-Seq volcano plot of the differentially expressed miRNAs (DEMs). Red represents high expression; green represents low expression.
Gene ontology enrichment showed that the predicted top 20 GO terms target genes regulated by the DEMs are mainly implicated in the regulation of signal transduction biological process (Figure 3A), cytoskeleton and cytoplasmic vesicle cellular compartments (Figure 3B), and carbohydrate and nucleotide binding molecular function (Figure 3C). Pathway enrichment KEGG (Figure 4) uncovered that the DEMs predicted targets were primarily involved in metabolic pathways; Pathways in cancer (Figure S1), PI3K-Akt Pathway (Figure S2), and MAPK signaling pathway (Figure S3). Reactome pathways revealed DEMs targets mainly involved in the immune system, small molecule transport, and cytokine signaling in the immune system (Figure S4). In contrast, for PANTHER and wikiPathways, the integrin signaling pathway (Figures S5A, B) and MAPK signaling pathway (Figure S3) had the most prominent DEMs predicted targets, respectively.
Figure 3 Gene ontological classifications of the differentially expressed miRNAs (DEMs) targets by sRNA-Seq between DLBCL and healthy group. The differentially expressed genes are grouped into three GO terms, (A) Biological process, (B) Cellular components, and (C) Molecular functions. The abscissa represents the number of annotated genes enriched primarily in each GO term.
Figure 4 Kyoto Encyclopedia of Genes and Genomes (KEGG) annotation of the differentially expressed miRNAs (DEMs) in DLBCL. Each circle represented a KEGG pathway, the name of which was shown on the left legend. Abscissa showed the number of genes enriched in each pathway. The bigger the fold enrichment is, the more significant the pathway. The color of the barplot represented negative log10 of the false discover rate (FDR) (red: high, blue: low).
The mean concertation of the total RNA extracted was 193.25 ± 124.2 ng/µL as measured by UV spectrophotometry. The 260/280 nm absorbance ratio was 1.966 ± 0.0302, while the mean concentration of miRNAs measured by spectrofluorometer was 67.622 ± 46.8 ng/µL, as shown in (Supplementary Table 2).
The cDNA synthesis control, UniSp6, was amplified at 20.9 Cq ( ± 0.45) in all samples. The qPCR positive inter-plate control, UniSp3, was amplified in all samples, and Cqs calibrated accordingly for all samples to 21.638 to limit run-to-run deviation. The average Cq values for each tested miRNA and RefFinder recommended three references (miR-361-5p, miR-101, and miR-29c-3p) with their geometric mean ranking values (1.32, 1.86, and 2.06) and average Cq of 28.12, 26.61, and 23.86, respectively are shown (Figure 5, Supplementary Table 7). The relative expression in DLBCL and healthy group of each miRNA was analyzed individually compared to the geometric mean of the expression level of miR-361-5p, miR-101, and miR-29c-3p in each sample by Welch’s 2-sample t-test (Figure 6 and Table 3). We confirmed the miRNA sequence result for 23/35 DEMs after applying cut-off FDR <0.05 and fold change < 2 (Figures 7A, B). Fourteen of the 23 DEMs were upregulated, and nine were downregulated in DLBCL relative to the normal lymph node. Specifically, we observed the dysregulation of 23 miRNAs that regulate two axes in DLBCL progression. The first axis promotes uncontrolled lymphoid proliferation, cellular growth, and apoptosis inhibition; this axis is regulated by oncomiRs (18, 19, 61), such as miR-17/92, miR-31, miR-34a, miR-106a, miR-451,miR-192-5p, and miR-1839, which we found to be overexpressed. The second axis is associated with the downregulation of the tumor suppressor miRNAs (62–64), such as miR-146a, miR-150, miR-151, miR-152, miR-216b, miR-217, and miR-885.
Figure 5 The ranking of candidate reference genes is based on the geometric mean of the ranking values by RefFinder. The results showed that miR-361-5p, miR-101, and miR-29c-3p are the top three stably expressed miRNAs that can be used as reference miRNAs for the normalization of the experimental data.
Figure 6 Estimated mean (± 95%) ΔΔCq values for expression of 23 selected miRNAs in healthy (n = 4) and DLBCL (n = 6) groups. Lighter points show individual samples. FDR-adjusted P-values for two-sample t-tests are ≤ 0.048 in all cases.
Table 3 Two-sample t-test results for differences in ΔΔCq between Healthy (n = 4) and DLBCL (n = 6) groups in 44 miRNAs, with FDR adjustment (lowest P-values first).
Figure 7 (A) q-PCR volcano plot showing FDR-adjusted, negative log10 P-value versus the estimated difference between mean ΔΔCq values in the DLBCL group (n = 6) relative to the healthy group (n = 4) for 45 micro-RNA markers. Labeled points highlight statistically significant differences in expression. Points with lighter outer rings are also significant using a more conservative Bonferroni-Holm correction. (B) qPCR heatmap of 23 differentially expressed miRNAs identified as significant in FDR-corrected two-sample t-tests. The colored bar at the top of the plot distinguishes normal subjects (beige) from cancer subjects (grey).
Small RNA-Seq has made distinctive contributions to discovering and quantifying miRNAs, investigating their differential expression contributing to tumorigenesis, and identifying highly similar miRNA family members that vary by a single nucleotide. The main focus of this research is to validate the sRNA-Seq results using RT-qPCR and explore the roles of miRNAs and different signaling pathways.
The DEMs observed herein are mainly predicted to regulate the RAS signaling pathways. The activation of receptor tyrosine kinase stimulates RAS to initiate two major downstream pathways: the phosphatidylinositol-3-kinase (PI3K)/serine-threonine kinases (AKT) (65) and mitogen-activated protein kinases (MAPK). Those, in turn, may result in the activation of C-Myc and transcription factor NF-κB, also enriched by the DEMs. Moreover, two miRNAs involved in the p53 pathway are reported. The disturbed regulation of these pathways may stimulate lymphoid cell proliferation growth and inhibition of apoptosis, as shown in other cancers (65–67).
The PI3K/AKT signaling cascade is one of the most significant intracellular signaling pathways, commonly activated in various malignancies (68). In health, the PI3K/AKT signaling pathway involves multiple biological functions, including cell growth and fate. Disruption of this pathway affects cell growth, development, metabolic activity, and cytoskeletal remodeling, resulting in cancer cell survival and therapy resistance (69–71). We found that miR-151, miR-192-5p, miR-152, and miR-885-5p are involved in this pathway and are dysregulated in canine DLBCL compared to lymph node tissue from healthy dogs. The miR-151 downregulation is also reported in other cancers, including Epstein-Barr virus-induced DLBCL (72). We also verified the downregulation of another tumor suppressor, miR-152, which exerts its function through its antiproliferative activity (73), repressing the PI3K signaling pathway (74). The phosphatase and tensin homolog (PTEN) inhibit the PI3K/AKT pathway with vital anticancer activity; we report the downregulation of miR-885-p, reported to activate the PI3K/AKT pathway by inhibiting PTEN (75). We also report the upregulation of miR-192-5p, which is also significantly overexpressed in the low-grade B-cell lymphoma, Waldenstrom Macroglobulinemia (76, 77), suggesting an oncogenic function. In addition, miR-144 was upregulated in canine DLBCL. The role is miR144-3p was investigated in other cancers, where the upregulation of miR-144-3p inhibits PTEN, promoting apoptosis of cancer cells (78–80). Noteworthy miR-451 is also upregulated in this study in canine DLBCL. The tuning function of both miRNAs has been reported in tumorigenesis (81), and its expression is reportedly altered in various cancers (82–84).
The other downstream arm of the RAS pathway is the activation of MAPK. These protein kinases auto-phosphorylate their serine and threonine residues to induce or inhibit their target (85), regulating essential biological functions such as cellular division, death, oxidative stress, and immune response (86, 87). We found that miR-146a and miR-31, involved in this pathway, are dysregulated in canine DLBCL compared to lymph node tissue from healthy dogs. The expression of miR-146a is altered in various cancers (88, 89) and reportedly targets IRAK1 and TRAF6, leading to the inactivation of the inflammatory, tumorigenic NF-kB signaling pathway (90, 91), apoptosis stimulation, and cancer cell proliferation reduction (92). Function studies are needed to determine the role of miR-146a in canine DLBCL. We also verified miR-31 overexpression in the DLBCL group compared to controls. The intricate dual function of miR-31 has been studied in various cancers (93–97). As a tumor suppressor, miR-31 affects genes implicated in PI3K/AKT and DNA repair, while its upregulation activates other pathways, including WNT, Hippo, and NF-κB (98). Given its upregulation in this study, miR-31 may act as an oncomiR in canine DLBC lymphoma; however, further studies are needed to elucidate its regulatory role in the carcinogenesis of this tumor.
Activation of signal transduction pathways such as RAS-PI3K/AKT and RAS-MAPK will converge on the nucleus to activate transcription factors responsible for diverse cellular functions. The MYC proto-oncogene rapidly responds to the stimulation of the RAS-MAPK pathway. The C-Myc signaling pathway regulates critical cellular functions, including division, diversification, cell signaling, metabolic activity, and cell death. Substantial evidence supports that abnormal MYC expression drives tumor onset and progression (99) and links it to all defining features of cancer (100, 101). Notably, we verified the overexpression of the oncogenic polycistronic cluster miR-17/92 (miR-17, miR-18a, miR-19b, miR-20a, and miR-92a), also known as oncomiR-1 (102, 103) and its paralogue miR-106a/363 (miR-106a, and miR-92a) (104) in canine DLBCL compared to healthy lymph nodes. OncomiR-1 is frequently dysregulated in canine and human DLBCL (105–108). OncomiR-1 is a direct downstream target of Myc (109). Myc regulates and activates oncomiR-1 that, in turn, targets and inhibits PTEN (110–112), consequently activating AKT (113, 114), as well as attenuates the proapoptotic protein BimL (115, 116) or transcription factor E2F (117, 118) hence, preventing apoptosis and DNA repair. Strong evidence suggests that the aberrant expression of oncomiR-1 leads to cancer development, including B-cell lymphoma in humans (119–121) and murine models (102, 103, 122). In line with previous studies, we observed the overexpression of miR-19b/20a (111, 123), which is known for repressing apoptosis and inducing malignant transformation by activating the mammalian target of the rapamycin (mTOR) pathway (124–126) and by suppressing PTEN (123, 127, 128).
Along with our previous study (129), sRNA-Seq and RT-qPCR quantification verified the upregulation of miR-34a in canine DLBCL (120, 129–131). This finding does not corroborate studies in human cancers where miR-34 is downregulated (132). This finding is explained by Christofferson and collaborators (2010), who elucidated the role of miR-34a in cellular senescence where it was activated independently of p53, the upstream regulator, to inhibit MYC through utilizing another pathway that involves the ETS transcription factor ELK1 (133, 134). We also report the downregulation of the tumor suppressor miR-150, a regulator of MYC (135). The effect of increased expression of miR-150 in reducing tumor growth has been investigated in many cancers (136–138). Xiao and collaborators (2016) investigated the regulatory role between miR-150 and c-Myb in B-cell development in vivo (139, 140) and concluded that miR-150 is expressed in mature lymphocytes and directly targets and activates c-Myc (141). The interplay between miR-150 and c-Myb can result in a wide range of changes, such as a severe block of B-cell development, deletion of the c-Myb, or one of the mature B-cell subsets expansion in case of miR-150 deficiency (142). Function studies of miR-150 are warranted in canine DLBCL.
Nuclear factor-κB (NF-κB) belongs to a family of inducible transcription factors that regulate many genes involved in immune and inflammatory responses. We report the downregulation of miR-217 in canine DLBCL, in agreement with what is reported in many cancers (143–145). Although the function of miR-217 is not fully established in B-cell lymphomas, the overexpression of miR-217-5p significantly decreased breast cancer cell proliferation, invasion, migration, and suppressed epithelial-to-mesenchymal transition due to the inhibition of the NF-κB signaling pathway by directly targeting metadherin (MTDH) (146) which is also known as astrocyte elevated gene 1 (AEG1) or LYRIC (Lysine Rich CEACAM1) (147). In laryngeal cancer, miR-217 exerts an anti-metastatic and antiproliferative effect via repression of its downstream target genes MTDH and the programmed cell death protein 1-ligand 1 (PD1-L1) (148) at the translational level. Interestingly, the increased expression of MTDH may also be driven by the PI3K/ARK, MAPK, Myc, and Wnt/β-catenin pathways (147, 149). It is noteworthy that given the multiple pathways interactions, oncogenic roles, and involvement in the chemoresistance of MTDH/LYRIC, there is an increased interest in investigating this molecule as a potential therapeutic target in cancer (147).
We also report two dysregulated miRNAs in canine DLBCL involved in the p53 pathway: miR-664 (upregulated) and miR-379-5p (downregulated). The p53 protein responds to a wide range of cellular stress signals (150) by regulating cell cycle and DNA repair, enhancing cell senescence (151) and apoptosis, and altering the cellular environment by changing the extracellular matrix and angiogenesis in a specific tissue location (152). Thus, the p53 pathway is tightly regulated by positive or negative feedback loops (152). The upregulation of miR-664 is also reported in human lung cancer cells (153), osteosarcoma (154), and hepatocellular carcinoma, where it was associated with poor prognosis (155). In squamous cell carcinoma, miR-664 upregulation promotes cell proliferation, migration, and invasion by targeting interferon regulatory factor 2 (IRF2), which inhibits p53 expression (156). Conversely, a tumor suppressor function has been shown in cutaneous malignant melanoma (157) and breast cancer (158). The oncogenic function is canine DLBCL is likely, but studies are needed to determine if similar mechanisms and targets apply to this tumor. The predicted dysregulated miRNA-mediated pathways in canine DLBCL need confirmation of the inverse correlation to important targets in tumor tissues to substantiate the pathway analysis results. Thus, our subsequent studies include the integration of transcriptome and proteome data.
Our study shows the downregulation of miR-129-5p in the DLBCL samples. Consistent results have been observed in other relevant research studies; gastric cancer (159, 160), colorectal cancer (161, 162), liver cancer (163), endometrial cancer (164), and esophageal cancer (165, 166). The miR-129 promoter hypermethylation (162, 167) allows the overexpression of SOX4 and, subsequently, tumor initiation, progression, and metastasis (164, 167)
Limitations of the study include a variation in the miRNA expression observed within the DLBCL group (Figure 1, Supplementary Table 1); this is explained by the heterogeneous nature of lymphomas even within the same type and subtypes, and corroborated by differences in mitotic index, staging, and progression-free survival time (168). Two biologically distinct groups are observed when focusing on validated DEMs between healthy and DLBCL patients (Figure 7B). Despite the heterogeneity of this disease, this set of 23 miRNAs can differentiate DLBCL from healthy patients. Further analyses with a larger cohort of DLBCL cases are needed to determine if DEMs within DLBCL patients may be markers to subtype or infer prognostication.
Unlike human studies, our study compared fresh frozen lymph nodes from canine DLBCL patients to healthy controls instead of reactive lymph nodes. Biopsy for histopathology of reactive lymph nodes is not commonly performed in a clinical setting in dogs due to concerns about the dog’s comfort, the invasiveness of the procedure, the possibility of complications, and the dog owners’ decision. A few studies on human patients revealed altered expression of miRNAs in reactive lymph nodes (169–173). These miRNAs may regulate immune cell activities and lead to lymph node reactivity. Thus, our subsequent studies will focus on a large prospective cohort of patients exploring different sample types (i.e., fine-needle aspirate) and the ability of the reported DEMs to discriminate DLBCL from other lymphomas and reactive lymph nodes.
Identifying biomarkers that allow earlier diagnosis of DLBCL is critical to improving treatment outcomes. Our study revealed 24 upregulated and 11 downregulated miRNAs in canine DLBCL relative to normal lymph nodes by sRNA-Seq, totaling 35 DEMs. From those, 23 DEMS were validated by RT-qPCR, 14 were upregulated, and nine were downregulated. Our results hold the promise of miRNAs as diagnostic biomarkers for DLBCL. Many DEMs identified herein are reportedly dysregulated in human DLBCL, such as the well-described miR-17/92 cluster, and miRNAs miR-106a, miR-451a, and miR-31 that were upregulated. Moreover, miR-150, miR-151, and miR-152 are downregulated in DLBCL in dogs and humans. Two DEMs (miR-216b and miR-1839) are reported for the first time to be differentially expressed between DLBCL and healthy controls in dogs. KEGG enrichment pathway analysis showed that the predicted target genes regulated by DEMs are mainly implicated in metabolic pathways, pathways in cancer, PI3K-AKT pathway, and MAPK signaling pathway. Our study serves as a building block for subsequent studies that will validate the diagnostic utility of this set of DEMs in a larger, prospective cohort of patients. The ability of this set of DEMs to distinguish DLBCL from other lymphoma subtypes and reactive lymph nodes will also be explored in future work. Our study serves as a guideline for subsequent experimental studies to determine the targets and functions of these altered miRNAs in canine DLBCL.
The original contributions presented in the study are publicly available. This data can be found here: https://www.ncbi.nlm.nih.gov/geo/query/acc.cgi?acc=GSE240893.
The animal studies were approved by The Purdue Animal Care and Use Committee (protocols #1708001607 and #1111000308). The studies were conducted in accordance with the local legislation and institutional requirements. Written informed consent was obtained from the owners for the participation of their animals in this study.
Conceptualization: NE, MC, and AS. Data curation: NE and MG. Formal analysis: NE, NL, and MG. Investigation: NE, MC, MG, and AS. Methodology: NE, MG, and AS. Project administration: AS. Resources: AS, NE. Software: NE, MG, and ES. Supervision: MC and AS. Validation: NE and ES. Writing original draft: NE, ES, NL, and AS. Writing, review & editing: NE, ES, MC, MG, NL, and AS. All authors have read and agreed to the published version of the manuscript.
This work was supported by the Purdue Research Foundation (PRF) of Purdue University and the Doberman Pinscher Health Foundation.
The authors declare that the research was conducted in the absence of any commercial or financial relationships that could be construed as a potential conflict of interest.
All claims expressed in this article are solely those of the authors and do not necessarily represent those of their affiliated organizations, or those of the publisher, the editors and the reviewers. Any product that may be evaluated in this article, or claim that may be made by its manufacturer, is not guaranteed or endorsed by the publisher.
The Supplementary Material for this article can be found online at: https://www.frontiersin.org/articles/10.3389/fonc.2023.1238613/full#supplementary-material
1. Ito D, Frantz AM, Modiano JF. Canine lymphoma as a comparative model for human non-hodgkin lymphoma: recent progress and applications. Veterinary Immunol immunopathology (2014) 159(3-4):192–201. doi: 10.1016/j.vetimm.2014.02.016
2. Vail DM, Thamm DH, Liptak JM. Hematopoietic tumors. withrow and MacEwen's small animal clinical. Oncology (2019), 688–772. doi: 10.1016/B978-0-323-59496-7.00033-5
3. Dias JNR, André AS, Aguiar SI, Gil S, Tavares L, Aires-da-Silva F. Immunotherapeutic strategies for canine lymphoma: Changing the odds against non-hodgkin lymphoma. Front Vet Sci (2021) 8:621758. doi: 10.3389/fvets.2021.621758
4. van Leeuwen MT, Turner JJ, Joske DJ, Falster MO, Srasuebkul P, Meagher NS, et al. Lymphoid neoplasm incidence by WHO subtype in australia 1982-2006. Int J Cancer (2014) 135(9):2146–56. doi: 10.1002/ijc.28849
5. Valli VE, San Myint M, Barthel A, Bienzle D, Caswell J, Colbatzky F, et al. Classification of canine malignant lymphomas according to the world health organization criteria. Vet Pathol (2011) 48(1):198–211. doi: 10.1177/0300985810379428
6. Garrett LD, Thamm DH, Chun R, Dudley R, Vail DM. Evaluation of a 6-month chemotherapy protocol with no maintenance therapy for dogs with lymphoma. J Vet Intern Med (2002) 16(6):704–9. doi: 10.1892/0891-6640(2002)016<0704:eoacpw>2.3.co;2
7. Keller ET, MacEwen EG, Rosenthal RC, Helfand SC, Fox LE. Evaluation of prognostic factors and sequential combination chemotherapy with doxorubicin for canine lymphoma. J Vet Intern Med (1993) 7(5):289–95. doi: 10.1111/j.1939-1676.1993.tb01021.x
8. Sato M, Yamazaki J, Goto-Koshino Y, Setoguchi A, Takahashi M, Baba K, et al. Minimal residual disease in canine lymphoma: An objective marker to assess tumour cell burden in remission. Vet J (2016), 215:38–42. doi: 10.1016/j.tvjl.2016.05.012
9. Aresu L, Martini V, Rossi F, Vignoli M, Sampaolo M, Aricò A, et al. Canine indolent and aggressive lymphoma: clinical spectrum with histologic correlation. Vet Comp Oncol (2015) 13(4):348–62. doi: 10.1111/vco.12048
10. Burnett RC, Vernau W, Modiano JF, Olver CS, Moore PF, Avery AC. Diagnosis of canine lymphoid neoplasia using clonal rearrangements of antigen receptor genes. Vet Pathol (2003) 40(1):32–41. doi: 10.1354/vp.40-1-32
11. He L, Hannon GJ. MicroRNAs: small RNAs with a big role in gene regulation. Nat Rev Genet (2004) 5(7):522–31. doi: 10.1038/nrg1379
12. Ambros V. MicroRNA pathways in flies and worms: growth, death, fat, stress, and timing. Cell (2003) 113(6):673–6. doi: 10.1016/s0092-8674(03)00428-8
13. Bartel DP. MicroRNAs: genomics, biogenesis, mechanism, and function. Cell (2004) 116(2):281–97. doi: 10.1016/s0092-8674(04)00045-5
14. Lai EC. microRNAs: runts of the genome assert themselves. Curr Biol (2003) 13(23):R925–36. doi: 10.1016/j.cub.2003.11.017
15. van den Berg A, Mols J, Han J. RISC-target interaction: cleavage and translational suppression. Biochim Biophys Acta (2008) 1779(11):668–77. doi: 10.1016/j.bbagrm.2008.07.005
16. Wienholds E, Plasterk RH. MicroRNA function in animal development. FEBS Lett 579(26):5911–22. doi: 10.1016/j.febslet.2005.07.070
17. Metias SM, Lianidou E, Yousef GM. MicroRNAs in clinical oncology: at the crossroads between promises and problems. J Clin Pathol (2009) 62(9):771–6. doi: 10.1136/jcp.2009.064717
18. Manikandan J, Aarthi JJ, Kumar SD, Pushparaj PN. Oncomirs: the potential role of non-coding microRNAs in understanding cancer. Bioinformation (2008) 2(8):330–4. doi: 10.6026/97320630002330
19. Esquela-Kerscher A, Slack FJ. Oncomirs - microRNAs with a role in cancer. Nat Rev Cancer (2006) 6(4):259–69. doi: 10.1038/nrc1840
20. Jovanovic M, Hengartner MO. miRNAs and apoptosis: RNAs to die for. Oncogene (2006) 25(46):6176–87. doi: 10.1038/sj.onc.1209912
21. Chen X, Ba Y, Ma L, Cai X, Yin Y, Wang K, et al. Characterization of microRNAs in serum: a novel class of biomarkers for diagnosis of cancer and other diseases. Cell Res (2008) 18(10):997–1006. doi: 10.1038/cr.2008.282
22. Mitchell PS, Parkin RK, Kroh EM, Fritz BR, Wyman SK, Pogosova-Agadjanyan EL, et al. Circulating microRNAs as stable blood-based markers for cancer detection. Proc Natl Acad Sci USA (2008) 105(30):10513–8. doi: 10.1073/pnas.0804549105
23. Creemers EE, Tijsen AJ, Pinto YM. Circulating microRNAs: novel biomarkers and extracellular communicators in cardiovascular disease? Circ Res (2012) 110(3):483–95. doi: 10.1161/circresaha.111.247452
24. Sadik N, Cruz L, Gurtner A, Rodosthenous RS, Dusoswa SA, Ziegler O, et al. Extracellular RNAs: A new awareness of old perspectives. Methods Mol Biol (2018) 1740:1–15. doi: 10.1007/978-1-4939-7652-2_1
25. Kosaka N, Yoshioka Y, Hagiwara K, Tominaga N, Katsuda T, Ochiya T. Trash or treasure: extracellular microRNAs and cell-to-cell communication. Front Genet (2013) 4:173. doi: 10.3389/fgene.2013.00173
26. Barbarotto E, Schmittgen TD, Calin GA. MicroRNAs and cancer: profile, profile, profile. Int J Cancer (2008) 122(5):969–77. doi: 10.1002/ijc.23343
27. Bonneau E, Neveu B, Kostantin E, Tsongalis GJ, De Guire V. How close are miRNAs from clinical practice? a perspective on the diagnostic and therapeutic market. Ejifcc (2019) 30(2):114–27.
28. Lawrie CH. MicroRNAs and lymphomagenesis: a functional review. Br J Haematol (2013) 160(5):571–81. doi: 10.1111/bjh.12157
29. Lan H, Lu H, Wang X, Jin H. MicroRNAs as potential biomarkers in cancer: opportunities and challenges. BioMed Res Int (2015) 2015:125094. doi: 10.1155/2015/125094
30. Bidarra D, Constâncio V, Barros-Silva D, Ramalho-Carvalho J, Moreira-Barbosa C, Antunes L, et al. Circulating MicroRNAs as biomarkers for prostate cancer detection and metastasis development prediction. Front Oncol (2019) 9:900. doi: 10.3389/fonc.2019.00900
31. To KK, Tong CW, Wu M, Cho WC. MicroRNAs in the prognosis and therapy of colorectal cancer: From bench to bedside. World J Gastroenterol (2018) 24(27):2949–73. doi: 10.3748/wjg.v24.i27.2949
32. Tan W, Liang G, Xie X, Jiang W, Tan L, Sanders AJ, et al. Incorporating MicroRNA into molecular phenotypes of circulating tumor cells enhances the prognostic accuracy for patients with metastatic breast cancer. Oncologist (2019) 24(11):e1044–54. doi: 10.1634/theoncologist.2018-0697
33. Krishnan P, Ghosh S, Wang B, Li D, Narasimhan A, Berendt R, et al. Next generation sequencing profiling identifies miR-574-3p and miR-660-5p as potential novel prognostic markers for breast cancer. BMC Genomics (2015), 16:735. doi: 10.1186/s12864-015-1899-0
34. Martinez-Gutierrez AD, Catalan OM, Vázquez-Romo R, Porras Reyes FI, Alvarado-Miranda A, Lara Medina F, et al. miRNA profile obtained by next-generation sequencing in metastatic breast cancer patients is able to predict the response to systemic treatments. Int J Mol Med (2019) 44(4):1267–80. doi: 10.3892/ijmm.2019.4292
35. Langmead B, Trapnell C, Pop M, Salzberg SL. Ultrafast and memory-efficient alignment of short DNA sequences to the human genome. Genome Biol (2009) 10(3):R25. doi: 10.1186/gb-2009-10-3-r25
36. Friedländer MR, Mackowiak SD, Li N, Chen W, Rajewsky N. miRDeep2 accurately identifies known and hundreds of novel microRNA genes in seven animal clades. Nucleic Acids Res (2012) 40(1):37–52. doi: 10.1093/nar/gkr688
37. Kalvari I, Nawrocki EP, Ontiveros-Palacios N, Argasinska J, Lamkiewicz K, Marz M, et al. Rfam 14: expanded coverage of metagenomic, viral and microRNA families. Nucleic Acids Res (2021) 49(D1):D192–d200. doi: 10.1093/nar/gkaa1047
38. Zhou L, Chen J, Li Z, Li X, Hu X, Huang Y, et al. Integrated profiling of microRNAs and mRNAs: microRNAs located on Xq27.3 associate with clear cell renal cell carcinoma. PloS One (2010) 5(12):e15224. doi: 10.1371/journal.pone.0015224
39. Love MI, Huber W, Anders S. Moderated estimation of fold change and dispersion for RNA-seq data with DESeq2. Genome Biol (2014) 15(12):550. doi: 10.1186/s13059-014-0550-8
40. Enright AJ, John B, Gaul U, Tuschl T, Sander C, Marks DS. MicroRNA targets in drosophila. Genome Biol (2003) 5(1):R1. doi: 10.1186/gb-2003-5-1-r1
41. Young MD, Wakefield MJ, Smyth GK, Oshlack A. Gene ontology analysis for RNA-seq: accounting for selection bias. Genome Biol (2010) 11(2):R14. doi: 10.1186/gb-2010-11-2-r14
42. Mao X, Cai T, Olyarchuk JG, Wei L. Automated genome annotation and pathway identification using the KEGG orthology (KO) as a controlled vocabulary. Bioinformatics (2005) 21(19):3787–93. doi: 10.1093/bioinformatics/bti430
43. Ge SX, Jung D, Yao R. ShinyGO: a graphical gene-set enrichment tool for animals and plants. Bioinformatics (2020) 36(8):2628–9. doi: 10.1093/bioinformatics/btz931
44. Jassal B, Matthews L, Viteri G, Gong C, Lorente P, Fabregat A, et al. The reactome pathway knowledgebase. Nucleic Acids Res (2020) 48(D1):D498–d503. doi: 10.1093/nar/gkz1031
45. Fabregat A, Jupe S, Matthews L, Sidiropoulos K, Gillespie M, Garapati P, et al. The reactome pathway knowledgebase. Nucleic Acids Res (2018) 46(D1):D649–55. doi: 10.1093/nar/gkx1132
46. Mi H, Thomas P. PANTHER pathway: an ontology-based pathway database coupled with data analysis tools. Methods Mol Biol (2009) 563:123–40. doi: 10.1007/978-1-60761-175-2_7
47. Martens M, Ammar A, Riutta A, Waagmeester A, Slenter Denise N, Hanspers K, et al. WikiPathways: connecting communities. Nucleic Acids Res (2021) 49(D1):D613–21. doi: 10.1093/nar/gkaa1024
48. Kanehisa M, Furumichi M, Sato Y, Ishiguro-Watanabe M, Tanabe M. KEGG: integrating viruses and cellular organisms. Nucleic Acids Res (2021) 49(D1):D545–51. doi: 10.1093/nar/gkaa970
49. Luo W, Brouwer C. Pathview: an R/Bioconductor package for pathway-based data integration and visualization. Bioinformatics (2013) 29(14):1830–1. doi: 10.1093/bioinformatics/btt285
50. Jensen SG, Lamy P, Rasmussen MH, Ostenfeld MS, Dyrskjøt L, Orntoft TF, et al. Evaluation of two commercial global miRNA expression profiling platforms for detection of less abundant miRNAs. BMC Genomics (2011) 12:435. doi: 10.1186/1471-2164-12-435
51. Livak KJ, Schmittgen TD. Analysis of relative gene expression data using real-time quantitative PCR and the 2(-delta delta C(T)) method. Methods (2001) 25(4):402–8. doi: 10.1006/meth.2001.1262
52. Mestdagh P, Van Vlierberghe P, De Weer A, Muth D, Westermann F, Speleman F, et al. A novel and universal method for microRNA RT-qPCR data normalization. Genome Biol (2009) 10(6):R64. doi: 10.1186/gb-2009-10-6-r64
53. Mar JC, Kimura Y, Schroder K, Irvine KM, Hayashizaki Y, Suzuki H, et al. Data-driven normalization strategies for high-throughput quantitative RT-PCR. BMC Bioinf (2009) 10:110. doi: 10.1186/1471-2105-10-110
54. Xie F, Xiao P, Chen D, Xu L, Zhang B. miRDeepFinder: a miRNA analysis tool for deep sequencing of plant small RNAs. Plant Mol Biol (2012). doi: 10.1007/s11103-012-9885-2
55. Vandesompele J, De Preter K, Pattyn F, Poppe B, Van Roy N, De Paepe A, et al. Accurate normalization of real-time quantitative RT-PCR data by geometric averaging of multiple internal control genes. Genome Biol (2002) 3(7):Research0034. doi: 10.1186/gb-2002-3-7-research0034
56. Andersen CL, Jensen JL, Ørntoft TF. Normalization of real-time quantitative reverse transcription-PCR data: a model-based variance estimation approach to identify genes suited for normalization, applied to bladder and colon cancer data sets. Cancer Res (2004) 64(15):5245–50. doi: 10.1158/0008-5472.can-04-0496
57. Silver N, Best S, Jiang J, Thein SL. Selection of housekeeping genes for gene expression studies in human reticulocytes using real-time PCR. BMC Mol Biol (2006) 7:33. doi: 10.1186/1471-2199-7-33
58. Pfaffl MW, Tichopad A, Prgomet C, Neuvians TP. Determination of stable housekeeping genes, differentially regulated target genes and sample integrity: BestKeeper–excel-based tool using pair-wise correlations. Biotechnol Lett (2004) 26(6):509–15. doi: 10.1023/b:bile.0000019559.84305.47
59. Diz AP, Carvajal-Rodríguez A, Skibinski DO. Multiple hypothesis testing in proteomics: a strategy for experimental work. Mol Cell Proteomics (2011) 10(3):M110.004374. doi: 10.1074/mcp.M110.004374
60. Benjamini Y, Hochberg Y. Controlling the false discovery rate: A practical and powerful approach to multiple testing. J R Stat Soc Ser B (Methodological) (1995) 57(1):289–300.
61. Cho WCS. OncomiRs: the discovery and progress of microRNAs in cancers. Mol Cancer (2007) 6(1):60. doi: 10.1186/1476-4598-6-60
62. Otmani K, Lewalle P. Tumor suppressor miRNA in cancer cells and the tumor microenvironment: Mechanism of deregulation and clinical implications. Front Oncol (2021) 11:708765. doi: 10.3389/fonc.2021.708765
63. Bueno MJ, Malumbres M. MicroRNAs and the cell cycle. Biochim Biophys Acta (2011) 1812(5):592–601. doi: 10.1016/j.bbadis.2011.02.002
64. Winter J, Jung S, Keller S, Gregory RI, Diederichs S. Many roads to maturity: microRNA biogenesis pathways and their regulation. Nat Cell Biol (2009) 11(3):228–34. doi: 10.1038/ncb0309-228
65. Osaki M, Oshimura M, Ito H. PI3K-akt pathway: its functions and alterations in human cancer. Apoptosis (2004) 9(6):667–76. doi: 10.1023/B:APPT.0000045801.15585.dd
66. Goodnow CC. Multistep pathogenesis of autoimmune disease. Cell (2007) 130(1):25–35. doi: 10.1016/j.cell.2007.06.033
67. Hanahan D, Weinberg RA. The hallmarks of cancer. Cell (2000) 100(1):57–70. doi: 10.1016/s0092-8674(00)81683-9
68. Liu P, Cheng H, Roberts TM, Zhao JJ. Targeting the phosphoinositide 3-kinase pathway in cancer. Nat Rev Drug Discovery (2009) 8(8):627–44. doi: 10.1038/nrd2926
69. Alzahrani AS. PI3K/Akt/mTOR inhibitors in cancer: At the bench and bedside. Semin Cancer Biol (2019) 59:125–32. doi: 10.1016/j.semcancer.2019.07.009
70. Burris HA 3rd. Overcoming acquired resistance to anticancer therapy: focus on the PI3K/AKT/mTOR pathway. Cancer Chemother Pharmacol (2013) 71(4):829–42. doi: 10.1007/s00280-012-2043-3
71. Pérez-Ramírez C, Cañadas-Garre M, Molina M, Faus-Dáder MJ, Calleja-Hernández M. PTEN and PI3K/AKT in non-small-cell lung cancer. Pharmacogenomics (2015) 16(16):1843–62. doi: 10.2217/pgs.15.122
72. Andrade TA, Evangelista AF, Campos AH, Poles WA, Borges NM, Camillo CM, et al. A microRNA signature profile in EBV+ diffuse large b-cell lymphoma of the elderly. Oncotarget (2014) 5(23):11813–26. doi: 10.18632/oncotarget.2952
73. Liu X, Li J, Qin F, Dai S. miR-152 as a tumor suppressor microRNA: Target recognition and regulation in cancer. Oncol Lett (2016) 11(6):3911–6. doi: 10.3892/ol.2016.4509
74. Ma J, Yao Y, Wang P, Liu Y, Zhao L, Li Z, et al. MiR-152 functions as a tumor suppressor in glioblastoma stem cells by targeting krüppel-like factor 4. Cancer Lett (2014) 355(1):85–95. doi: 10.1016/j.canlet.2014.09.012
75. Jiang Z, Cui H, Zeng S, Li L. miR-885-5p inhibits invasion and metastasis in gastric cancer by targeting malic enzyme 1. DNA Cell Biol (2021) 40(5):694–705. doi: 10.1089/dna.2020.6478
76. Bouyssou JM, Liu CJ, Bustoros M, Sklavenitis-Pistofidis R, Aljawai Y, Manier S, et al. Profiling of circulating exosomal miRNAs in patients with waldenström macroglobulinemia. PloS One (2018) 13(10):e0204589. doi: 10.1371/journal.pone.0204589
77. Trino S, Lamorte D, Caivano A, De Luca L, Sgambato A, Laurenzana I. Clinical relevance of extracellular vesicles in hematological neoplasms: from liquid biopsy to cell biopsy. Leukemia (2021) 35(3):661–78. doi: 10.1038/s41375-020-01104-1
78. Cao HL, Gu MQ, Sun Z, Chen ZJ. miR-144-3p contributes to the development of thyroid tumors through the PTEN/PI3K/AKT pathway. Cancer Manag Res (2020) 12:9845–55. doi: 10.2147/cmar.s265196
79. Yuan X, Pan J, Wen L, Gong B, Li J, Gao H, et al. MiR-144-3p enhances cardiac fibrosis after myocardial infarction by targeting PTEN. Front Cell Dev Biol (2019) 7:249. doi: 10.3389/fcell.2019.00249
80. Murphy CP, Li X, Maurer V, Oberhauser M, Gstir R, Wearick-Silva LE, et al. MicroRNA-mediated rescue of fear extinction memory by miR-144-3p in extinction-impaired mice. Biol Psychiatry (2017) 81(12):979–89. doi: 10.1016/j.biopsych.2016.12.021
81. Wang T, Wu F, Yu D. miR-144/451 in hematopoiesis and beyond. ExRNA 1(1):16. doi: 10.1186/s41544-019-0035-8
82. Kanaoka R, Iinuma H, Dejima H, Sakai T, Uehara H, Matsutani N, et al. Usefulness of plasma exosomal MicroRNA-451a as a noninvasive biomarker for early prediction of recurrence and prognosis of non-small cell lung cancer. Oncology (2018) 94(5):311–23. doi: 10.1159/000487006
83. Kawamura S, Iinuma H, Wada K, Takahashi K, Minezaki S, Kainuma M, et al. Exosome-encapsulated microRNA-4525, microRNA-451a and microRNA-21 in portal vein blood is a high-sensitive liquid biomarker for the selection of high-risk pancreatic ductal adenocarcinoma patients. J Hepatobiliary Pancreat Sci (2019) 26(2):63–72. doi: 10.1002/jhbp.601
84. Goto T, Fujiya M, Konishi H, Sasajima J, Fujibayashi S, Hayashi A, et al. An elevated expression of serum exosomal microRNA-191, - 21, -451a of pancreatic neoplasm is considered to be efficient diagnostic marker. BMC Cancer (2018) 18(1):116. doi: 10.1186/s12885-018-4006-5
85. Johnson GL, Lapadat R. Mitogen-activated protein kinase pathways mediated by ERK, JNK, and p38 protein kinases. Science (2002) 298(5600):1911–2. doi: 10.1126/science.1072682
86. Arthur JS, Ley SC. Mitogen-activated protein kinases in innate immunity. Nat Rev Immunol (2013) 13(9):679–92. doi: 10.1038/nri3495
87. Soares-Silva M, Diniz FF, Gomes GN, Bahia D. The mitogen-activated protein kinase (MAPK) pathway: Role in immune evasion by trypanosomatids. Front Microbiol (2016) 7:183. doi: 10.3389/fmicb.2016.00183
88. He L, Wang Z, Zhou R, Xiong W, Yang Y, Song N, et al. Dexmedetomidine exerts cardioprotective effect through miR-146a-3p targeting IRAK1 and TRAF6 via inhibition of the NF-κB pathway. BioMed Pharmacother (2021) 133:110993. doi: 10.1016/j.biopha.2020.110993
89. Liu R, Liu C, Chen D, Yang WH, Liu X, Liu CG, et al. FOXP3 controls an miR-146/NF-κB negative feedback loop that inhibits apoptosis in breast cancer cells. Cancer Res (2015) 75(8):1703–13. doi: 10.1158/0008-5472.can-14-2108
90. Su YL, Wang X, Mann M, Adamus TP, Wang D, Moreira DF, et al. Myeloid cell-targeted miR-146a mimic inhibits NF-κB-driven inflammation and leukemia progression in vivo. Blood (2020) 135(3):167–80. doi: 10.1182/blood.2019002045
91. Zilahi E, Tarr T, Papp G, Griger Z, Sipka S, Zeher M. Increased microRNA-146a/b, TRAF6 gene and decreased IRAK1 gene expressions in the peripheral mononuclear cells of patients with sjögren's syndrome. Immunol Lett (2012) 141(2):165–8. doi: 10.1016/j.imlet.2011.09.006
92. Garo LP, Ajay AK, Fujiwara M, Gabriely G, Raheja R, Kuhn C, et al. MicroRNA-146a limits tumorigenic inflammation in colorectal cancer. Nat Commun (2021) 12(1):2419. doi: 10.1038/s41467-021-22641-y
93. Soheilyfar S, Velashjerdi Z, Sayed Hajizadeh Y, Fathi Maroufi N, Amini Z, Khorrami A, et al. In vivo and in vitro impact of miR-31 and miR-143 on the suppression of metastasis and invasion in breast cancer. J Buon (2018) 23(5):1290–6.
94. Zhao L, Sun Y, Hou Y, Peng Q, Wang L, Luo H, et al. MiRNA expression analysis of cancer-associated fibroblasts and normal fibroblasts in breast cancer. Int J Biochem Cell Biol (2012) 44(11):2051–9. doi: 10.1016/j.biocel.2012.08.005
95. Kent OA, Mendell JT, Rottapel R. Transcriptional regulation of miR-31 by oncogenic KRAS mediates metastatic phenotypes by repressing RASA1. Mol Cancer Res (2016) 14(3):267–77. doi: 10.1158/1541-7786.mcr-15-0456
96. Pedroza-Torres A, Fernández-Retana J, Peralta-Zaragoza O, Jacobo-Herrera N, Cantú de Leon D, Cerna-Cortés JF, et al. A microRNA expression signature for clinical response in locally advanced cervical cancer. Gynecol Oncol (2016) 142(3):557–65. doi: 10.1016/j.ygyno.2016.07.093
97. Zeljic K, Jovanovic I, Jovanovic J, Magic Z, Stankovic A, Supic G. MicroRNA meta-signature of oral cancer: evidence from a meta-analysis. Ups J Med Sci (2018) 123(1):43–9. doi: 10.1080/03009734.2018.1439551
98. Sur D, Cainap C, Burz C, Havasi A, Chis IC, Vlad C, et al. The role of miRNA -31-3p and miR-31-5p in the anti-EGFR treatment efficacy of wild-type k-RAS metastatic colorectal cancer. is it really the next best thing in miRNAs? J Buon (2019) 24(5):1739–46.
99. Carabet LA, Rennie PS, Cherkasov A. Therapeutic inhibition of myc in cancer. structural bases and computer-aided drug discovery approaches. Int J Mol Sci (2018) 20(1). doi: 10.3390/ijms20010120
100. Hanahan D, Weinberg RA. Hallmarks of cancer: the next generation. Cell (2011) 144(5):646–74. doi: 10.1016/j.cell.2011.02.013
101. Gabay M, Li Y, Felsher DW. MYC activation is a hallmark of cancer initiation and maintenance. Cold Spring Harb Perspect Med (2014) 4(6). doi: 10.1101/cshperspect.a014241
102. Ventura A, Young AG, Winslow MM, Lintault L, Meissner A, Erkeland SJ, et al. Targeted deletion reveals essential and overlapping functions of the miR-17 through 92 family of miRNA clusters. Cell (2008) 132(5):875–86. doi: 10.1016/j.cell.2008.02.019
103. Xiao C, Srinivasan L, Calado DP, Patterson HC, Zhang B, Wang J, et al. Lymphoproliferative disease and autoimmunity in mice with increased miR-17-92 expression in lymphocytes. Nat Immunol (2008) 9(4):405–14. doi: 10.1038/ni1575
104. Mogilyansky E, Rigoutsos I. The miR-17/92 cluster: a comprehensive update on its genomics, genetics, functions and increasingly important and numerous roles in health and disease. Cell Death Differ (2013) 20(12):1603–14. doi: 10.1038/cdd.2013.125
105. Dal Bo M, Bomben R, Hernández L, Gattei V. The MYC/miR-17-92 axis in lymphoproliferative disorders: A common pathway with therapeutic potential. Oncotarget (2015) 6(23):19381–92. doi: 10.18632/oncotarget.4574
106. Fassina A, Marino F, Siri M, Zambello R, Ventura L, Fassan M, et al. The miR-17-92 microRNA cluster: a novel diagnostic tool in large b-cell malignancies. Lab Invest (2012) 92(11):1574–82. doi: 10.1038/labinvest.2012.129
107. Jin HY, Oda H, Lai M, Skalsky RL, Bethel K, Shepherd J, et al. MicroRNA-17~92 plays a causative role in lymphomagenesis by coordinating multiple oncogenic pathways. EMBO J (2013) 32(17):2377–91. doi: 10.1038/emboj.2013.178
108. Lawrie CH, Soneji S, Marafioti T, Cooper CD, Palazzo S, Paterson JC, et al. MicroRNA expression distinguishes between germinal center b cell-like and activated b cell-like subtypes of diffuse large b cell lymphoma. Int J Cancer (2007) 121(5):1156–61. doi: 10.1002/ijc.22800
110. Dhawan A, Scott JG, Harris AL, Buffa FM. Pan-cancer characterisation of microRNA across cancer hallmarks reveals microRNA-mediated downregulation of tumour suppressors. Nat Commun 9(1):5228. doi: 10.1038/s41467-018-07657-1
111. Olive V, Bennett MJ, Walker JC, Ma C, Jiang I, Cordon-Cardo C, et al. miR-19 is a key oncogenic component of mir-17-92. Genes Dev (2009) 23(24):2839–49. doi: 10.1101/gad.1861409
112. Jiang P, Rao EY, Meng N, Zhao Y, Wang JJ. MicroRNA-17-92 significantly enhances radioresistance in human mantle cell lymphoma cells. Radiat Oncol (2010) 5:100. doi: 10.1186/1748-717x-5-100
113. Fang LL, Wang XH, Sun BF, Zhang XD, Zhu XH, Yu ZJ, et al. Expression, regulation and mechanism of action of the miR-17-92 cluster in tumor cells (Review). Int J Mol Med (2017) 40(6):1624–30. doi: 10.3892/ijmm.2017.3164
114. Jiang C, Bi C, Jiang X, Tian T, Huang X, Wang C, et al. The miR-17~92 cluster activates mTORC1 in mantle cell lymphoma by targeting multiple regulators in the STK11/AMPK/TSC/mTOR pathway. Br J Haematol (2019) 185(3):616–20. doi: 10.1111/bjh.15591
115. Wang Z, Malone MH, He H, McColl KS, Distelhorst CW. Microarray analysis uncovers the induction of the proapoptotic BH3-only protein bim in multiple models of glucocorticoid-induced apoptosis. J Biol Chem (2003) 278(26):23861–7. doi: 10.1074/jbc.M301843200
116. Jelínková I, Šafaříková B, Vondálová Blanářová O, Skender B, Hofmanová J, Sova P, et al. Platinum(IV) complex LA-12 exerts higher ability than cisplatin to enhance TRAIL-induced cancer cell apoptosis via stimulation of mitochondrial pathway. Biochem Pharmacol (2014) 92(3):415–24. doi: 10.1016/j.bcp.2014.09.013
117. O'Donnell KA, Wentzel EA, Zeller KI, Dang CV, Mendell JT. C-myc-regulated microRNAs modulate E2F1 expression. Nature (2005) 435(7043):839–43. doi: 10.1038/nature03677
118. Zhang Y, Zhang A, Shen C, Zhang B, Rao Z, Wang R, et al. E2F1 acts as a negative feedback regulator of c-Myc-induced hTERT transcription during tumorigenesis. Oncol Rep (2014) 32(3):1273–80. doi: 10.3892/or.2014.3287
119. Alsaadi M, Khan MY, Dalhat MH, Bahashwan S, Khan MU, Albar A, et al. Dysregulation of miRNAs in DLBCL: Causative factor for pathogenesis, diagnosis and prognosis. Diagnostics (Basel) (2021) 11(10). doi: 10.3390/diagnostics11101739
120. Meng Y, Quan L, Liu A. Identification of key microRNAs associated with diffuse large b-cell lymphoma by analyzing serum microRNA expressions. Gene (2018) 642:205–11. doi: 10.1016/j.gene.2017.11.022
121. Labi V, Schoeler K, Melamed D. miR-17∼92 in lymphocyte development and lymphomagenesis. Cancer Lett (2019) 446:73–80. doi: 10.1016/j.canlet.2018.12.020
122. Solé C, Arnaiz E, Lawrie CH. MicroRNAs as biomarkers of b-cell lymphoma. biomark Insights (2018) 13:1177271918806840. doi: 10.1177/1177271918806840
123. Yuan J, Su Z, Gu W, Shen X, Zhao Q, Shi L, et al. MiR-19b and miR-20a suppress apoptosis, promote proliferation and induce tumorigenicity of multiple myeloma cells by targeting PTEN. Cancer biomark (2019) 24(3):279–89. doi: 10.3233/cbm-182182
124. Aoki M, Fujishita T. Oncogenic roles of the PI3K/AKT/mTOR axis. Curr Top Microbiol Immunol (2017) 407:153–89. doi: 10.1007/82_2017_6
125. Düvel K, Yecies JL, Menon S, Raman P, Lipovsky AI, Souza AL, et al. Activation of a metabolic gene regulatory network downstream of mTOR complex 1. Mol Cell (2010) 39(2):171–83. doi: 10.1016/j.molcel.2010.06.022
126. Zhang J, Wang L, Jiang J, Qiao Z. The lncRNA SNHG15/miR-18a-5p axis promotes cell proliferation in ovarian cancer through activating Akt/mTOR signaling pathway. J Cell Biochem (2020) 121(12):4699–710. doi: 10.1002/jcb.29474
127. Gao X, Qin T, Mao J, Zhang J, Fan S, Lu Y, et al. PTENP1/miR-20a/PTEN axis contributes to breast cancer progression by regulating PTEN via PI3K/AKT pathway. J Exp Clin Cancer Res (2019) 38(1):256. doi: 10.1186/s13046-019-1260-6
128. Li RK, Gao J, Guo LH, Huang GQ, Luo WH. PTENP1 acts as a ceRNA to regulate PTEN by sponging miR-19b and explores the biological role of PTENP1 in breast cancer. Cancer Gene Ther (2017) 24(7):309–15. doi: 10.1038/cgt.2017.29
129. Elshafie NO, Nascimento NCD, Lichti NI, Kasinski AL, Childress MO, Santos APD. MicroRNA biomarkers in canine diffuse large b-cell lymphoma. Vet Pathol (2021) 58(1):34–41. doi: 10.1177/0300985820967902
130. Craig KKL, Wood GA, Keller SM, Mutsaers AJ, Wood RD. MicroRNA profiling in canine multicentric lymphoma. PloS One (2019) 14(12):e0226357. doi: 10.1371/journal.pone.0226357
131. Hershkovitz-Rokah O, Geva P, Salmon-Divon M, Shpilberg O, Liberman-Aronov S. Network analysis of microRNAs, genes and their regulation in diffuse and follicular b-cell lymphomas. Oncotarget (2018) 9(8):7928–41. doi: 10.18632/oncotarget.23974
132. Kalfert D, Ludvikova M, Pesta M, Ludvik J, Dostalova L, Kholová I. Multifunctional roles of miR-34a in cancer: A review with the emphasis on head and neck squamous cell carcinoma and thyroid cancer with clinical implications. Diagnostics (Basel) (2020) 10(8). doi: 10.3390/diagnostics10080563
133. Christoffersen NR, Shalgi R, Frankel LB, Leucci E, Lees M, Klausen M, et al. p53-independent upregulation of miR-34a during oncogene-induced senescence represses MYC. Cell Death Differ (2010) 17(2):236–45. doi: 10.1038/cdd.2009.109
134. Raucci A, Macrì F, Castiglione S, Badi I, Vinci MC, Zuccolo E. MicroRNA-34a: the bad guy in age-related vascular diseases. Cell Mol Life Sci (2021) 78(23):7355–78. doi: 10.1007/s00018-021-03979-4
135. Ziel-Swier L, Liu Y, Seitz A, de Jong D, Koerts J, Rutgers B, et al. The role of the MYC/miR-150/MYB/ZDHHC11 network in hodgkin lymphoma and diffuse large b-cell lymphoma. Genes (Basel) (2022) 13(2). doi: 10.3390/genes13020227
136. Lee KH, Lee JK, Choi DW, Do IG, Sohn I, Jang KT, et al. Postoperative prognosis prediction of pancreatic cancer with seven microRNAs. Pancreas (2015) 44(5):764–8. doi: 10.1097/mpa.0000000000000346
137. Dezhong L, Xiaoyi Z, Xianlian L, Hongyan Z, Guohua Z, Bo S, et al. miR-150 is a factor of survival in prostate cancer patients. J buon (2015) 20(1):173–9.
138. Yokobori T, Suzuki S, Tanaka N, Inose T, Sohda M, Sano A, et al. MiR-150 is associated with poor prognosis in esophageal squamous cell carcinoma via targeting the EMT inducer ZEB1. Cancer Sci (2013) 104(1):48–54. doi: 10.1111/cas.12030
139. Xiao C, Calado DP, Galler G, Thai TH, Patterson HC, Wang J, et al. MiR-150 controls b cell differentiation by targeting the transcription factor c-myb. Cell (2016) 165(4):1027. doi: 10.1016/j.cell.2016.04.056
140. Xiao C, Calado DP, Galler G, Thai TH, Patterson HC, Wang J, et al. MiR-150 controls b cell differentiation by targeting the transcription factor c-myb. Cell (2007) 131(1):146–59. doi: 10.1016/j.cell.2007.07.021
141. Cogswell JP, Cogswell PC, Kuehl WM, Cuddihy AM, Bender TM, Engelke U, et al. Mechanism of c-myc regulation by c-myb in different cell lineages. Mol Cell Biol (1993) 13(5):2858–69. doi: 10.1128/mcb.13.5.2858-2869.1993
142. Thomas MD, Kremer CS, Ravichandran KS, Rajewsky K, Bender TP. C-myb is critical for b cell development and maintenance of follicular b cells. Immunity (2005) 23(3):275–86. doi: 10.1016/j.immuni.2005.08.005
143. Zhao W, Wang X, Jiang Y, Jia X, Guo Y. miR-217-5p inhibits invasion and metastasis of prostate cancer by targeting clusterin. Mamm Genome (2021) 32(5):371–80. doi: 10.1007/s00335-021-09874-4
144. Xing X, An M, Chen T. LncRNA SNHG20 promotes cell proliferation and invasion by suppressing miR-217 in ovarian cancer. Genes Genomics (2021) 43(9):1095–104. doi: 10.1007/s13258-021-01138-4
145. Wang S, Tong H, Su T, Zhou D, Shi W, Tang Z, et al. CircTP63 promotes cell proliferation and invasion by regulating EZH2 via sponging miR-217 in gallbladder cancer. Cancer Cell Int (2021) 21(1):608. doi: 10.1186/s12935-021-02316-w
146. Yang L, Liu S, Xu B, Wang M, Kong X, Song Z. miR-217-5p suppresses epithelial-mesenchymal transition and the NF-κB signaling pathway in breast cancer via targeting of metadherin. Oncol Lett (2022) 23(5):162. doi: 10.3892/ol.2022.13282
147. Dhiman G, Srivastava N, Goyal M, Rakha E, Lothion-Roy J, Mongan NP, et al. Metadherin: A therapeutic target in multiple cancers. Front Oncol (2019) 9:349. doi: 10.3389/fonc.2019.00349
148. Miao S, Mao X, Zhao S, Song K, Xiang C, Lv Y, et al. miR-217 inhibits laryngeal cancer metastasis by repressing AEG-1 and PD-L1 expression. Oncotarget (2017) 8(37):62143–53. doi: 10.18632/oncotarget.19121
149. Hu G, Wei Y, Kang Y. The multifaceted role of MTDH/AEG-1 in cancer progression. Clin Cancer Res (2009) 15(18):5615–20. doi: 10.1158/1078-0432.ccr-09-0049
150. Vogelstein B, Lane D, Levine AJ. Surfing the p53 network. Nature (2000) 408(6810):307–10. doi: 10.1038/35042675
151. Jin S, Levine AJ. The p53 functional circuit. J Cell Sci (2001) 114(Pt 23):4139–40. doi: 10.1242/jcs.114.23.4139
152. Harris SL, Levine AJ. The p53 pathway: positive and negative feedback loops. Oncogene (2005) 24(17):2899–908. doi: 10.1038/sj.onc.1208615
153. Zhu X, Ju S, Yuan F, Chen G, Shu Y, Li C, et al. microRNA-664 enhances proliferation, migration and invasion of lung cancer cells. Exp Ther Med (2017) 13(6):3555–62. doi: 10.3892/etm.2017.4433
154. Bao Y, Chen B, Wu Q, Hu K, Xi X, Zhu W, et al. Overexpression of miR-664 is associated with enhanced osteosarcoma cell migration and invasion ability via targeting SOX7. Clin Exp Med (2017) 17(1):51–8. doi: 10.1007/s10238-015-0398-6
155. Wang X, Zhou Z, Zhang T, Wang M, Xu R, Qin S, et al. Overexpression of miR-664 is associated with poor overall survival and accelerates cell proliferation, migration and invasion in hepatocellular carcinoma. Onco Targets Ther (2019) 12:2373–81. doi: 10.2147/ott.s188658
156. Li X, Zhou C, Zhang C, Xie X, Zhou Z, Zhou M, et al. MicroRNA-664 functions as an oncogene in cutaneous squamous cell carcinomas (cSCC) via suppressing interferon regulatory factor 2. J Dermatol Sci (2019) 94(3):330–8. doi: 10.1016/j.jdermsci.2019.05.004
157. Ding Z, Jian S, Peng X, Liu Y, Wang J, Zheng L, et al. Loss of MiR-664 expression enhances cutaneous malignant melanoma proliferation by upregulating PLP2. Med (Baltimore) (2015) 94(33):e1327. doi: 10.1097/md.0000000000001327
158. Wu L, Li Y, Li J, Ma D. MicroRNA-664 targets insulin receptor substrate 1 to suppress cell proliferation and invasion in breast cancer. Oncol Res (2019) 27(4):459–67. doi: 10.3727/096504018x15193500663936
159. Katada T, Ishiguro H, Kuwabara Y, Kimura M, Mitui A, Mori Y, et al. microRNA expression profile in undifferentiated gastric cancer. Int J Oncol (2009) 34(2):537–42.
160. Tsai KW, Wu CW, Hu LY, Li SC, Liao YL, Lai CH, et al. Epigenetic regulation of miR-34b and miR-129 expression in gastric cancer. Int J Cancer (2011) 129(11):2600–10. doi: 10.1002/ijc.25919
161. Karaayvaz M, Zhai H, Ju J. miR-129 promotes apoptosis and enhances chemosensitivity to 5-fluorouracil in colorectal cancer. Cell Death Dis (2013) 4(6):e659. doi: 10.1038/cddis.2013.193
162. Bandres E, Agirre X, Bitarte N, Ramirez N, Zarate R, Roman-Gomez J, et al. Epigenetic regulation of microRNA expression in colorectal cancer. Int J Cancer (2009) 125(11):2737–43. doi: 10.1002/ijc.24638
163. Liu Y, Hei Y, Shu Q, Dong J, Gao Y, Fu H, et al. VCP/p97, down-regulated by microRNA-129-5p, could regulate the progression of hepatocellular carcinoma. PloS One (2012) 7(4):e35800. doi: 10.1371/journal.pone.0035800
164. Vervoort SJ, van Boxtel R, Coffer PJ. The role of SRY-related HMG box transcription factor 4 (SOX4) in tumorigenesis and metastasis: friend or foe? Oncogene (2013) 32(29):3397–409. doi: 10.1038/onc.2012.506
165. Chen X, Hu H, Guan X, Xiong G, Wang Y, Wang K, et al. CpG island methylation status of miRNAs in esophageal squamous cell carcinoma. Int J Cancer (2012) 130(7):1607–13. doi: 10.1002/ijc.26171
166. Kang M, Li Y, Liu W, Wang R, Tang A, Hao H, et al. miR-129-2 suppresses proliferation and migration of esophageal carcinoma cells through downregulation of SOX4 expression. Int J Mol Med (2013) 32(1):51–8. doi: 10.3892/ijmm.2013.1384
167. Fesler A, Zhai H, Ju J. miR-129 as a novel therapeutic target and biomarker in gastrointestinal cancer. Onco Targets Ther (2014) 7:1481–5. doi: 10.2147/ott.s65548
168. Aresu L. Canine lymphoma, more than a morphological diagnosis: What we have learned about diffuse large b-cell lymphoma. Front Vet Sci (2016) 3:77. doi: 10.3389/fvets.2016.00077
169. Asmar F, Hother C, Kulosman G, Treppendahl MB, Nielsen HM, Ralfkiaer U, et al. Diffuse large b-cell lymphoma with combined TP53 mutation and MIR34A methylation: Another "double hit" lymphoma with very poor outcome? Oncotarget (2014) 5(7):1912–25. doi: 10.18632/oncotarget.1877
170. Liu YP, Hu H, Xu F, Wen JJ. [Relation of MiR-34a expression in diffuse large b cell lymphoma with clinical prognosis]. Zhongguo Shi Yan Xue Ye Xue Za Zhi (2017) 25(2):455–9. doi: 10.7534/j.issn.1009-2137.2017.02.026
171. Wang WL, Yang C, Han XL, Wang R, Huang Y, Zi YM, et al. MicroRNA-23a expression in paraffin-embedded specimen correlates with overall survival of diffuse large b-cell lymphoma. Med Oncol (2014) 31(4):919. doi: 10.1007/s12032-014-0919-2
172. Han B, Gao ZD, Wang HX, Wang ZH, Fan CB, Liu JL, et al. [Expression of MiR-155 in tissue of patients with diffuse large b-cell lymphoma and its effect on cell biological characteristics]. Zhongguo Shi Yan Xue Ye Xue Za Zhi (2019) 27(2):445–51. doi: 10.19746/j.cnki.issn.1009-2137.2019.02.022
Keywords: diffuse large B-cell lymphoma, multicentric, miRNAs, cancer biomarkers, miRNome, sRNA sequencing, canine DLBCL
Citation: Elshafie NO, Gribskov M, Lichti NI, Sayedahmed EE, Childress MO and dos Santos AP (2023) miRNome expression analysis in canine diffuse large B-cell lymphoma. Front. Oncol. 13:1238613. doi: 10.3389/fonc.2023.1238613
Received: 12 June 2023; Accepted: 04 August 2023;
Published: 30 August 2023.
Edited by:
Brian D. Adams, Brain Institute of America, United StatesReviewed by:
Ghanbar Mahmoodi Chalbatani, Tehran University of Medical Sciences, IranCopyright © 2023 Elshafie, Gribskov, Lichti, Sayedahmed, Childress and dos Santos. This is an open-access article distributed under the terms of the Creative Commons Attribution License (CC BY). The use, distribution or reproduction in other forums is permitted, provided the original author(s) and the copyright owner(s) are credited and that the original publication in this journal is cited, in accordance with accepted academic practice. No use, distribution or reproduction is permitted which does not comply with these terms.
*Correspondence: Andrea P. dos Santos, c2FudG9zMUBwdXJkdWUuZWR1
Disclaimer: All claims expressed in this article are solely those of the authors and do not necessarily represent those of their affiliated organizations, or those of the publisher, the editors and the reviewers. Any product that may be evaluated in this article or claim that may be made by its manufacturer is not guaranteed or endorsed by the publisher.
Research integrity at Frontiers
Learn more about the work of our research integrity team to safeguard the quality of each article we publish.