- 1Department of General Surgery, The Fourth Hospital of Hebei Medical University, Shijiazhuang, China
- 2School of Information Science and Engineering, Hebei University of Science and Technology, Shijiazhuang, China
- 3Department of Gastrointestinal Surgery, The Third Hospital of Hebei Medical University, Shijiazhuang, China
- 4Department of General Surgery, The Third Hospital of Hebei Medical University, Shijiazhuang, China
- 5Department of Pathology, The Fourth Hospital of Hebei Medical University, Shijiazhuang, China
- 6The Second Hospital of Hebei Medical University, Shijiazhuang, Hebei, China
Background: We attempted to develop a progression prediction model for local advanced rectal cancer(LARC) patients who received preoperative neoadjuvant chemoradiotherapy(NCRT) and operative treatment to identify high-risk patients in advance.
Methods: Data from 272 LARC patients who received NCRT and total mesorectal excision(TME) from 2011 to 2018 at the Fourth Hospital of Hebei Medical University were collected. Data from 161 patients with rectal cancer (each sample with one target variable (progression) and 145 characteristic variables) were included. One Hot Encoding was applied to numerically represent some characteristics. The K-Nearest Neighbor (KNN) filling method was used to determine the missing values, and SmoteTomek comprehensive sampling was used to solve the data imbalance. Eventually, data from 135 patients with 45 characteristic clinical variables were obtained. Random forest, decision tree, support vector machine (SVM), and XGBoost were used to predict whether patients with rectal cancer will exhibit progression. LASSO regression was used to further filter the variables and narrow down the list of variables using a Venn diagram. Eventually, the prediction model was constructed by multivariate logistic regression, and the performance of the model was confirmed in the validation set.
Results: Eventually, data from 135 patients including 45 clinical characteristic variables were included in the study. Data were randomly divided in an 8:2 ratio into a data set and a validation set, respectively. Area Under Curve (AUC) values of 0.72 for the decision tree, 0.97 for the random forest, 0.89 for SVM, and 0.94 for XGBoost were obtained from the data set. Similar results were obtained from the validation set. Twenty-three variables were obtained from LASSO regression, and eight variables were obtained by considering the intersection of the variables obtained using the previous four machine learning methods. Furthermore, a multivariate logistic regression model was constructed using the data set; the ROC indicated its good performance. The ROC curve also verified the good predictive performance in the validation set.
Conclusions: We constructed a logistic regression model with good predictive performance, which allowed us to accurately predict whether patients who received NCRT and TME will exhibit disease progression.
1 Introduction
Colorectal cancer is the third most common cancer worldwide and the second leading cause of cancer-related deaths. In 2020, rectal cancer accounted for 6.0% of newly diagnosed cancer cases and 3.4% of cancer deaths (1). The last decades witnessed the development of multidiscipline, individualization, and precision in treatments for rectal cancer. NCRT followed by TME has been recommended for patients diagnosed with LARC, which is correlated to lower treatment-related toxicity rate, lower local recurrence rate, and higher sphincter preserve rate (2).
However, in clinical research, the sensitivity of patients with rectal cancer to preoperative neoadjuvant therapy varies significantly, and more than half of the patients are not sensitive to neoadjuvant therapy (3, 4) and exhibit disease progression after preoperative neoadjuvant therapy and operative treatment (5). Thus, we need to accurately predict disease progression in this group of patients to target the high-risk patients for focused care and related interventions.
Current methods used for predicting the outcomes of preoperative neoadjuvant therapy include MRI imaging (6), molecular marker examination (7), blood levels (8), and the assessment of pathological and clinical characteristics (9). However, the predictions are unsatisfactory and are primarily useful for determining the effects of preoperative neoadjuvant therapy. Meanwhile, no significant progress has been made in the prediction of disease progression after preoperative neoadjuvant therapy and surgical treatment. Moreover, the routine preoperative examination of patients usually involves blood tests, such as those for neutrophil or leukocyte levels, among others. The routine preoperative examination may have better effects on predicting disease progression if multiple variables, including those available from initial tests (conducted at admission) and post-neoadjuvant examination and tests, can be used comprehensively. This would help avoid the omission of important variables and the deletion or selection of critical variables for predicting disease progression after treatment.
The significance of joint work between medcine and machine learning has been more and more recognised (10). Artificial intelligence (AI) and machine learning have been widely used to screen, diagnose, and treat patients with cancer (11). The AI risk assessment of pulmonary lymph nodes is an example. Compared to traditional statistical methods, AI techniques are more effective for handling complex data (12). Moreover, AI tools can also be built to predict the prognosis of liver cancer (13), lung cancer (14), colorectal squamous cell carcinoma (15), or breast cancer (16) in patients based on pathological images or clinicopathological characteristics. AI application represents a significant trend with potential applications in predicting the outcomes of preoperative neoadjuvant therapy and disease progression after operative treatment (17).
The prediction of disease progression after preoperative neoadjuvant therapy and operative treatment is of great significance. Moreover, previous studies had reported the prediction of the effect of preoperative neoadjuvant, such as MRI (18), circulating DNA (19), tumor microsatellite stability (20), immune cell infiltration (21), etc. However, these studies only considered a few variables, and the true magnitude of the effect needed to be clarified. Chemotherapy has become one of the most important elements in the treatment of rectal cancer (22). Preoperative neoadjuvant therapy and postoperative chemotherapy can improve the prognosis of rectal cancer patients (23). The guidelind suggest the patients recieved a total durationg of 6 months before and after operation (24), we excluded the patients didn’t recieved sufficent chemotheratpy. Here, we included information on the patients collected at admission, after neoadjuvant treatment and postoperative information such as the tumor location, colonoscopy results, imaging and postoperative pathology results. We included multiple variables in the study. We further filtered eight variables using various machine learning methods for analysis, attempting to avoid the loss of important variables. We believed this would help build a prediction model with good predictive ability, which would help predict the outcomes of neoadjuvant treatment and disease progression after operative treatment.
2 Materials and methods
2.1 Patients
A retrospective study was conducted. 272 patients diagnosed with LARC, who received NCRT and underwent TME at the 4th hospital of Hebei Medical University(Shijiazhuang, Hebei, China) were enrolled from 2011-2018 were enrolled. Data included 145 clinical variables were collected.
After considering the inclusion and exclusion criteria, data from 135 patients with rectal cancer, which included 45 clinical characteristic variables, were included in the study. All patients had undergone R0 resection after NCRT. Inclusion criteria: 1) location in the rectum, within 12 cm from the anal verge; 2) pathologically malignant and diagnosed as adenocarcinoma; 3) preoperative neoadjuvant treatment before imaging diagnosis of stage II-III disease; 4) availability of complete clinical data; 5)received standard radiotherapy: 5 days a week at 1.8 Gy per day for 5;weeks to a dose of 45 Gy, followed by a boost of 5.4 Gy, for a total dose of 50.4 Gy; 6)received complete preoperative and post-operative therapy with a duration of 6 months. Exclusion criteria: 1) Concomitant with other serious diseases, such as myocardial infarction; 2) Not receiving standard NCRT; 3) Refuse follow-up; 4)refuse to receive TME after NCRT. The patients in our study recieved standard XELOX regimen in pre-operative and post-operative chemotherapy. The research scheme was approved by the Ethics Committee of the Fourth Hospital of Hebei Medical University (Approval Code: 2023KS015).
2.2 Data processing
Sample data from 272 patients with rectal cancer were screened. Data from 161 patients with rectal cancer followed up for 2 years, who subsequently underwent TME after neoadjuvant therapy, were included.
One target quantity (progression) and 145 characteristic variables were selected per sample. Concurrently, One Hot Encoding was applied to certain numerical characteristics in data processing to facilitate model training. Moreover, the KNN filling method was applied for missing data attributes, whereas SmoteTomek comprehensive sampling (25–27) was used to solve the data imbalance problem to improve the classification accuracy in a few classes. Eventually, 135 patients were selected, and the final model was constructed using four machine learning methods (ten-fold cross-validation) to screen important variables for constructing the prediction model and validating it using a ROC curve.
The 145 variables were shown as followed, Gender, age, previous medical history, chief complaint, family history, smoking history, drinking history were retrospectively collected from the medical history database. As digital rectal examination, blood test, MRI, coloscopy were perfomed both before NCRT and before TME, variables from these tests were recorded twice. In digital rectal examination, the distance between toumor and anus, whether blood was observed after examination were recorded. For the tumors failed to reach through digital rectal examination, the distance was recorded through colonscopy. Variables from coloscope include: whether stenosis, edema or mucus was observed, the morphology of tumor, the status of mucosa. The level of blood tumor biomarkers inclued CEA, CA-199, CA-724, ferroprotein, β2-microglobulin were recorded. The counting of red blood cell, white blood cell, neutrophil, lymphocyte, platelet was recorded. The serum level of albumin was also recorded. Variables from MRI inculded cricumferential invasion, tumor size, clinical TNM staging, vessel invasion. For the pathological results of coloscopy biopsy, the pathological diagnosis, tumor differentiation were recorded. The exact operating method of TME and post-operative complication was also recorded. The mutation status of KRAS, NRAS, BRAF, and the expresstion status of Her-2 MLH1, MSH2, PMS2 were record through the pathological results of the operative specimens. Variable from operative specimens also included tumor size, morphology, tumor differentiation, histological grade, pathological TNM stage, blood vessel invasion, perineural invasion, tumor regression grade. The total number of post-operative The survival and progression information was collected through telephone follow-up.
2.3 Statistical analysis
Statistical work were completed by statistical experts (School of Information Science and Engineering, Hebei University of Science and Technology). The decision tree analysis was conducted using rpart package, random forest analysis was conducted using randomForest package, SVM was conducted using e1071 package, XGBoost was conducted using xgboost package, and LASSO regression was conducted using glmnet package. The predictive ability of the prediction models was assessed based on the AUC values of the ROC curves. P<0.05 was considered statistically significant.
3 Results
3.1 Baseline clinical characteristics
We included data from 135 patients from the Fourth Hospital of Hebei Medical University who had undergone preoperative neoadjuvant therapy. Forty-five independent variables, such as gender, age, and others, were included in this study. They were randomly divided into the training and test sets in a ratio of 8:2 for subsequent analysis. There were no difference between the groups (Supplementary Table 1). The detailed information of 135 patients (with 45 variables) had been shown in Supplementary Table 2. The process was shown in Figure 1.
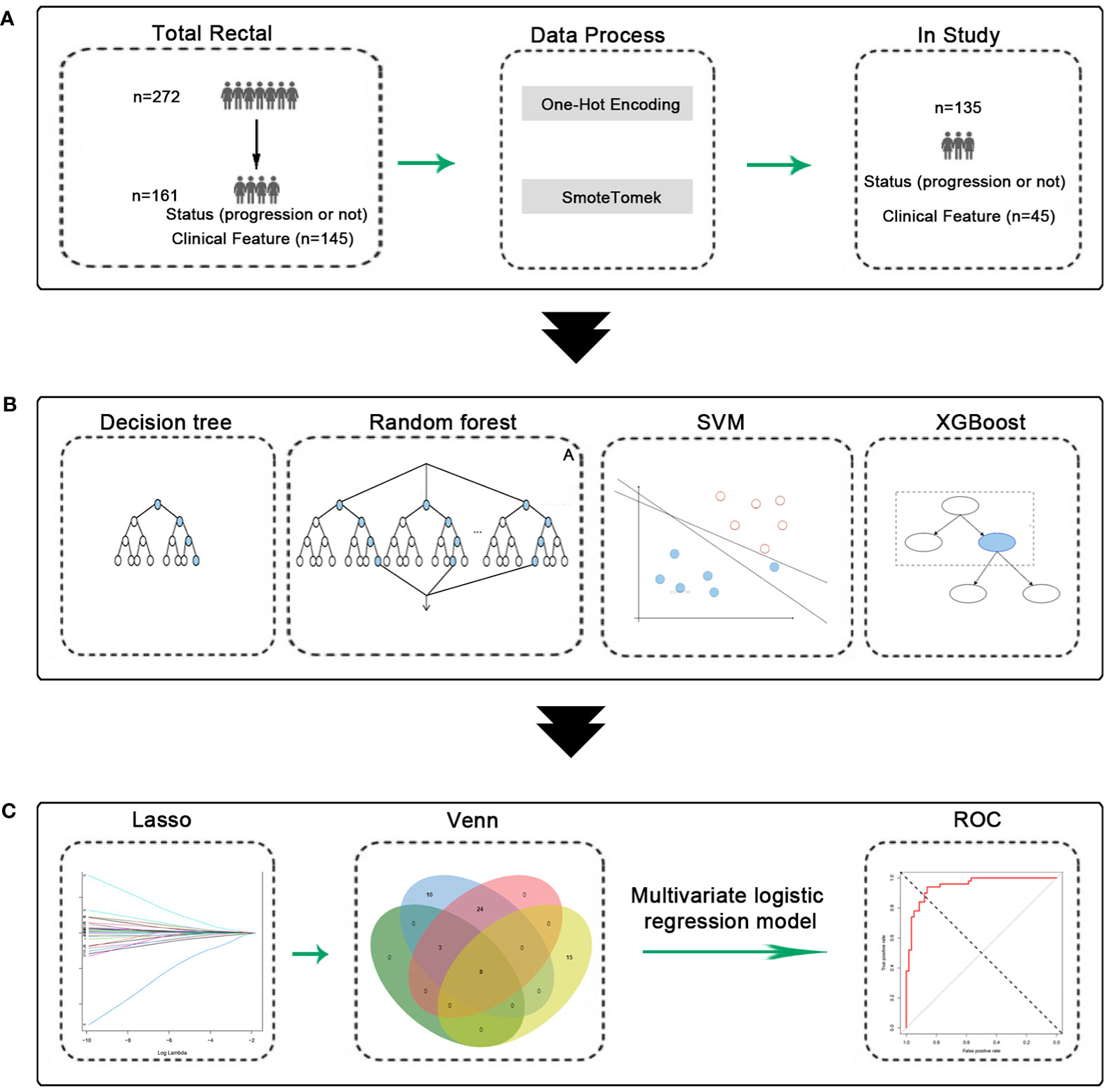
Figure 1 Experimental flow chart. (A) Data process, 135 patients were obtained. (B) Machine learning model construction and validation. (C) Construction and validation of predictive models.
3.2 Machine learning model construction and validation
All 45 baseline characteristics, including initial hospitalization data and preoperative data, were used to construct a model to predict whether the disease had progressed. Moreover, four machine learning methods were used in the training set to construct the models. In this model, as shown in Figure 2, the AUC values were 0.72 ± 0.11 for decision trees (Figure 2A), 0.97 ± 0.04 for random forests (Figure 2B), 0.89 ± 0.11 for SVM (Figure 2C), and 0.94 ± 0.10 for XGBoost (Figure 2D). To confirm the potential of the four machine learning models, we tested them in a test set and obtained similar results (Figure 3). Our results indicated the excellent predictive ability of the four machine learning models.
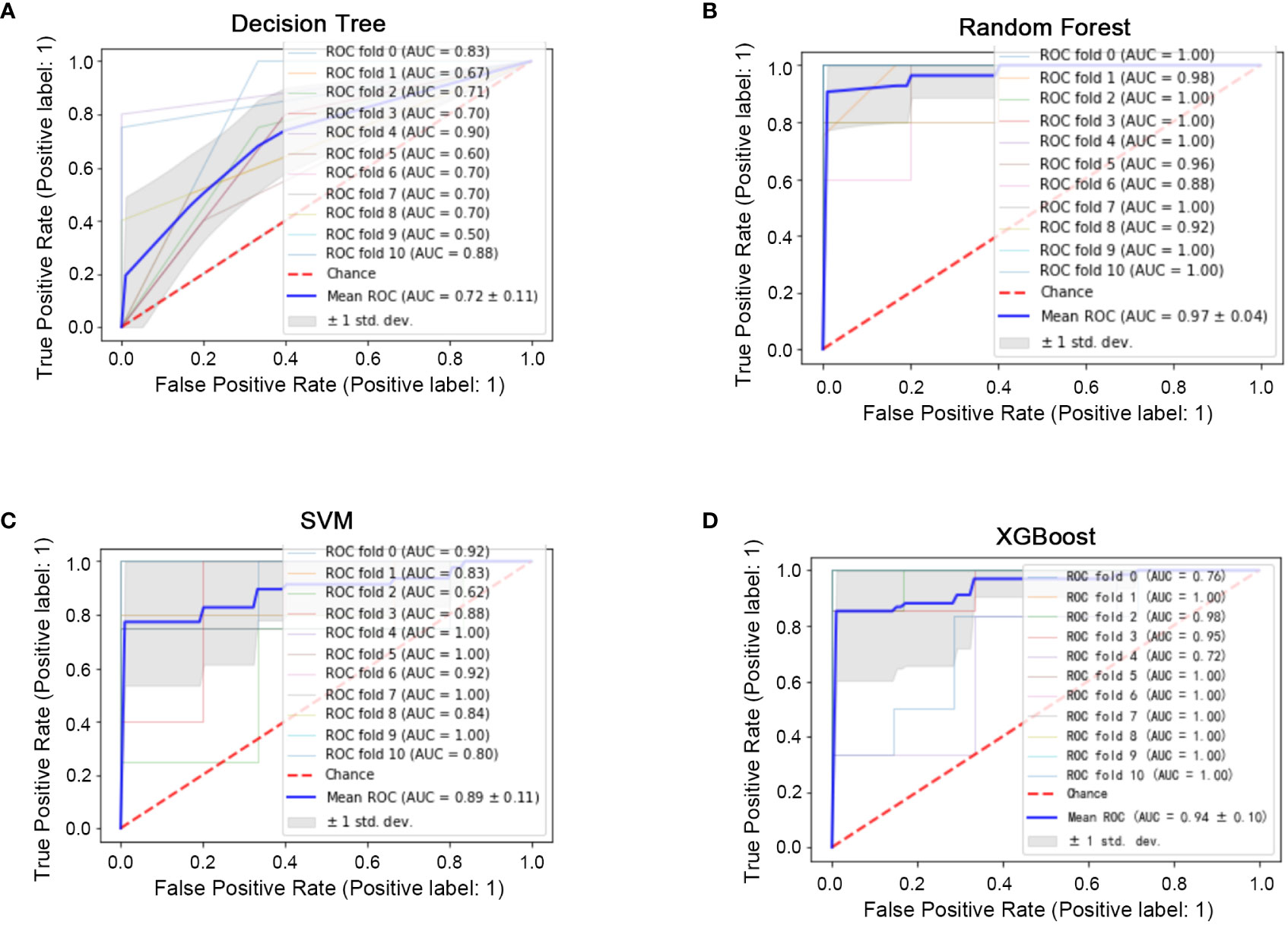
Figure 2 Machine learning model construction (ten-fold cross-validation) in the training set. (A) ROC diagram of the decision tree in the training set. (B) ROC diagram of the random forest in the training set. (C): ROC diagram of the support vector machine in the training set. (D) ROC diagram of XGBoost in the training set.
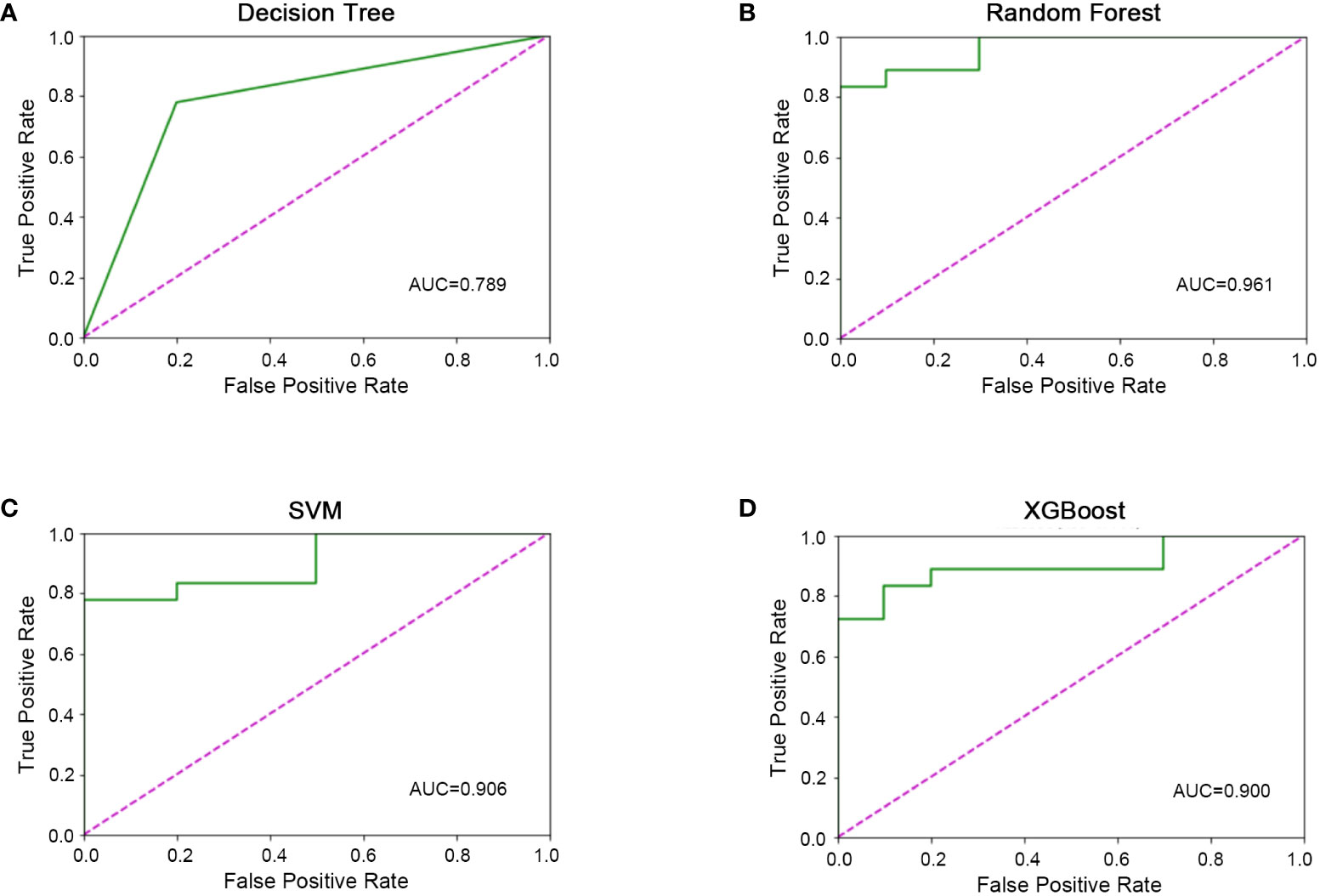
Figure 3 Machine learning model validation in the validation set. (A) ROC diagram of the decision tree in the validation set. (B) ROC diagram of the random forest in the validation set. (C) ROC diagram of support vector machine in the validation set. D) ROC diagram of XGBoost in the validation set.
3.3 Predictor construction and validation
The predictive ability of the four machine learning models werre good; however, with so many variables, it was not very convenient for practical applications. To further reduce the number of variables, we performed LASSO regression analysis, which yielded 23 variables identified (Figures 4A, B) and eight critical variables (tumor size, pre-operative serium CEA, distant metastasis in NCRT, nerve invasion, age, vascular invasion, preoperative lymph node metastasis, MLH1) were identified using a Venn diagram by four methods (Figure 4C). The MLH1-status was assessed by immunohistochemistry(IHC). These eight variables were subsequently used for multivariate logistics regression to construct a diagnostic prediction model with a discriminant optimal cutoff value of 0.314, suggesting that patients with scores <0.314 could be considered progression-free and patients with scores >0.314 could be considered to exhibit progression. In the training set, ROC analysis revealed a sensitivity of 94% and a specificity of 86.2% for the differentiation between progression and non-progression (Figure 4D upper), with an AUC value of 0.9486 (Figure 4E). Similar results were obtained in the validation set, with a sensitivity of 94.4%, a specificity of 66.7% (Figure 4D down), and an AUC value of 0.784 (Figure 4F).
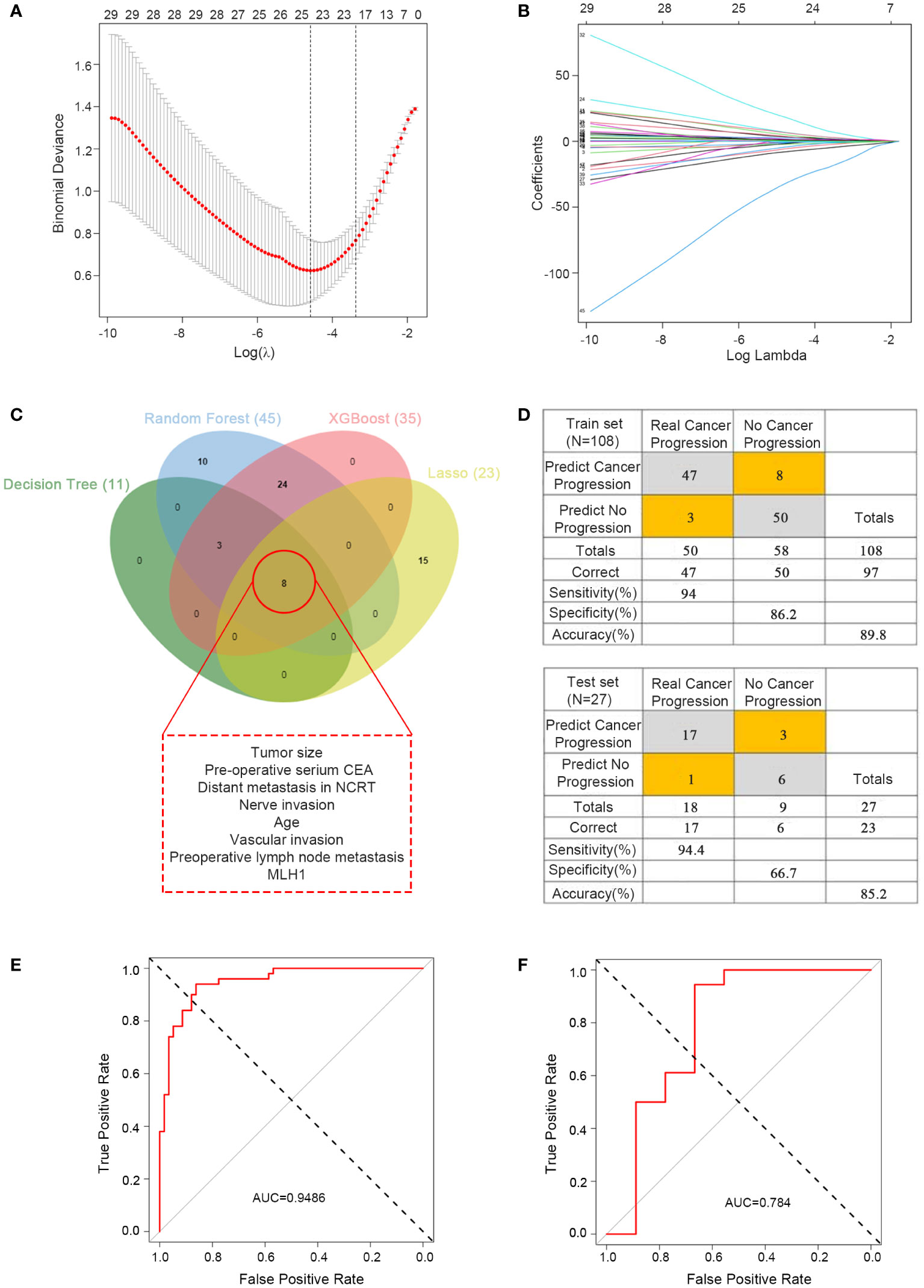
Figure 4 Predictor construction and validation. (A) Clinical characteristics of patients with rectal cancer in the LASSO model. (B) Selection of the tuning parameter (λ) in the LASSO model required cross-validation using the maximum criteria. (C) Venn diagram of the outcomes of the four machine learning methods for filtering variables. (D) Confusion matrix of binary outcomes after logistic regression for predicting patient progression in rectal cancer, the predictor for the train set (upper) and test set (lower). (E) ROC curves for predicting disease progression in patients with rectal cancer undergoing preoperative neoadjuvant therapy and after surgical treatment to distinguish whether progression; the training set. (F) ROC curves for predicting disease progression in patients with rectal cancer undergoing preoperative neoadjuvant therapy and after surgical treatment to distinguish whether progression; the test set.
4 Disscussion
Preoperative neoadjuvant chemoradiotherapy was an important part of rectal cancer treatment (28), and many previous studies had reported prediction models for the response of rectal cancer to preoperative neoadjuvant therapy (18, 29, 30). However, cases of progression after preoperative neoadjuvant therapy could not be ignored. To rule out the differences caused by chemotherapy, we ultimately included patients who had undergone sufficient chemotherapy in the study to minimize the bias caused by individual chemotherapy as much as possible. In our study, we obtained eight critical variables using four machine learning methods to construct a prediction model for progression after preoperative neoadjuvant therapy, which can reasonably predict the disease progression of patients. This can help improve the focus and increase the frequency of reviews in such cases. Additionally, once signs of progression were detected, the treatment plan could be altered immediately. This can help avoid delays in treatment and improve patient prognosis.
Machine learning have been widely applied in clinical decision-making (10). For example, machine learning had been previously applied to readmission after elective laparoscopic colorectal surgery (31). Tumor burden before and after NCRT are depcited through cTNM before NCRT stage and ypTNM respectively. However, the joint effects of pre and after NCRT stage is not well-studied. A system that is able to integret multiple information may better predict the prognosis of patients (32).
In our study, we included more than 100 variables and tested comprehensive data to avoid missing variables that can influence disease progression. To our knowledge, the variables included in our study were numerous.
We initially constructed four machine learning models. Even though the AUC values were high, they all showed good predictive functions in the training set and test set, but the value of 0.9 did not meet our requirements. Hence, we further screened the variables by LASSO regression and then using Venn diagram to further screen variables. We eventually selected eight important variables. The AUC value of the final prediction model was considerably high at 0.9486, indicating the excellent function of our model. The applicability of these eight variables was high because they were mandatory examinations or tests for patients who require hospitalization.
Feature selection plays a crucial role in the field of machine learning, as it can select the most informative features from raw data, improve model performance, reduce overfitting, and accelerate model training and prediction speed. In large-scale datasets and high-dimensional data, feature selection is particularly important because unnecessary features increase computational complexity and introduce redundant information (33, 34). When selecting univariate and multivariate regression analysis, we need to have an adequate sample size, with a positive sample size at least 10 times the number of variables. The more the better, in order to meet the meaningful results. In addition, we believed that the feature selectionn of univariate and multivariate regression carries subjectivity (subjective selection of p-value), while the feature selection of machine learning relies on computation and is more observable. In sum, univariate and multivariate regression focus more on analyzing the impact of independent variables on outcomes, while feature selection is a part of machine learning.
In the features selection for model construction, SVM was excluded from the analysis. The machine learning of this study were based on the sklearn framework. Decision trees, random forests, and XGBoost were all based on the important features of tree models, so the important features can be obtained from the model. However, SVM did not have important features in the algorithm, so important features were not be obtained. Therefore, in the selection of the variables, SVM was excluded from the analysis.
Currently, nearly all the prediction models for rectal cancer patients undergoing neoadjuvant treatment are used to predict tumor response to identify sensitive patients. Here, we aimed to predict tumor progression after neoadjuvant treatment, to identify high-risk patients. Thus, we pay more attention to these high-risk patients, benefiting for early detection and early treatment. CEA, known as a biomarker in colorectal cancer, had been reported to be associated with pathological complete remission after neoadjuvant treatment for rectal cancer, and tumor size and preoperative CEA are related to tumor downstaging (35). So tumor size and preoperative CEA had the potential to predict tumor progression. Similarly, distant metastasis, nerve invasion, age, vascular invasion, and preoperative lymph node metastasis are all related to tumor prognosis (36, 37), revealing the potential to predict tumor progression. These 7 variables are routine preoperative examination items, indicating that our model had good generality.
However, the time point at which progression occurred and was concentrated remains unascertained, which was also a limitation of this study. In our future studies, we will focus on this aspect of the research topic to determine the period in which the disease is more prone to progression. In addition, This study was a single center retrospective study, and the model constructed lacked external data validation. There was also a selection bias in this study due to the missing cases in the study. In the future, we would collaborate with other centers to further increase the sample size, validate and optimize the model constructed in this study. This will help reduce the frequency at which reviews are conducted and help focus on reviews during critical periods. This is also conducive to adjustments in treatment plans based on the availability of medical resources. In conclusion, we have constructed a model with good predictive function and wide applicability, which can help improve the focus on critical patients and their prognosis.
5 Conclusion
We constructed a logistic regression model with good predictive performance, which allowed us to accurately predict whether patients who received NCRT (sufficent standard XELOX regimen) and TME will exhibit disease progression.
Data availability statement
The original contributions presented in the study are included in the article/Supplementary Material. Further inquiries can be directed to the corresponding authors.
Ethics statement
The studies involving humans were approved by The research scheme was approved by the Ethics Committee of the Fourth Hospital of Hebei Medical University (Approval Code: 2023KS015). The studies were conducted in accordance with the local legislation and institutional requirements. Written informed consent for participation was not required from the participants or the participants’ legal guardians/next of kin because Our research was a retrospective study and did not require the written informed consent.
Author contributions
All authors contributed to the research conception and design. Conceptualization, GW, YZ and YL; methodology, YS, JH; software, YS, YZ, GW; validation, JZ; formal analysis, YT; investigation, JH, DL; resources, GW; data curation, JM; writing—original draft preparation, YY; writing—review and editing, YL; visualization, XW; supervision, JH; project administration, JH; funding acquisition, GW. All authors have read and agreed to the published version of the manuscript.
Funding
The author(s) declare financial support was received for the research, authorship, and/or publication of this article. This research was funded by Hebei Natural Science Foundation (H2020206485), (H2022206355).
Acknowledgments
Thanks to everyone who has supported and helped with this research.
Conflict of interest
The authors declare that the research was conducted in the absence of any commercial or financial relationships that could be construed as a potential conflict of interest.
Publisher’s note
All claims expressed in this article are solely those of the authors and do not necessarily represent those of their affiliated organizations, or those of the publisher, the editors and the reviewers. Any product that may be evaluated in this article, or claim that may be made by its manufacturer, is not guaranteed or endorsed by the publisher.
Supplementary material
The Supplementary Material for this article can be found online at: https://www.frontiersin.org/articles/10.3389/fonc.2023.1231508/full#supplementary-material
References
1. Sung H, Ferlay J, Siegel RL, Laversanne M, Soerjomataram I, Jemal A, et al. Global cancer statistics 2020: GLOBOCAN estimates of incidence and mortality worldwide for 36 cancers in 185 countries. CA: Cancer J Clin (2021) 71(3):209–49. doi: 10.3322/caac.21660
2. Sauer R, Becker H, Hohenberger W, Rödel C, Wittekind C, Fietkau R, et al. Preoperative versus postoperative chemoradiotherapy for rectal cancer. N Engl J Med (2004) 351(17):1731–40. doi: 10.1056/NEJMoa040694
3. Pohl M, Schmiegel W. Therapeutic strategies in diseases of the digestive tract - 2015 and beyond targeted therapies in colon cancer today and tomorrow. Dig Dis (2016) 34(5):574–9. doi: 10.1159/000445267
4. Peng SH, Mbarak HS, Li YH, Ma C, Shang QL, Chen Z, et al. Neoadjuvant intra-arterial versus intravenous chemotherapy in colorectal cancer. Med (Baltimore) (2021) 100(51):e28312. doi: 10.1097/MD.0000000000028312
5. Subbiah IM, Blackmon SH, Correa AM, Kee B, Vaporciyan AA, Swisher SG, et al. Preoperative chemotherapy prior to pulmonary metastasectomy in surgically resected primary colorectal carcinoma. Oncotarget (2014) 5(16):6584–93. doi: 10.18632/oncotarget.2172
6. Yang R, Zhao H, Wang X, Ding Z, Tao Y, Zhang C, et al. Magnetic resonance imaging radiomics modeling predicts tumor deposits and prognosis in stage T3 lymph node positive rectal cancer. Abdom Radiol (NY) (2023) 48(4):1268–79. doi: 10.1007/s00261-023-03825-0
7. Wang H, Ji D, Tian H, Gao Z, Song C, Jia J, et al. Predictive value of proteomic markers for advanced rectal cancer with neoadjuvant chemoradiotherapy. BMC Cancer (2022) 22(1):868. doi: 10.1186/s12885-022-09960-z
8. Martín-Carnicero A, Ramalle-Gomara E, Rubio-Mediavilla S, Alonso-Lago M, Zorrilla-Larraga M, Manrique-Abós , et al. Prognostic and predictive biomarkers in patients with locally advanced rectal cancer (LARC) treated with preoperative chemoradiotherapy. J Clin Med (2022) 11(20):6091. doi: 10.3390/jcm11206091
9. Karimi M, Osterlund P, Hammarström K, Imam I, Frodin JE, Glimelius B. Associations between response to commonly used neo-adjuvant schedules in rectal cancer and routinely collected clinical and imaging parameters. Cancers (Basel) (2022) 14(24):6238. doi: 10.3390/cancers14246238
10. Ichimasa K, Kudo SE, Mori Y, Misawa M, Matsudaira S, Kouyama Y, et al. Artificial intelligence may help in predicting the need for additional surgery after endoscopic resection of T1 colorectal cancer. Endoscopy (2018) 50(3):230–40. doi: 10.1055/s-0043-122385
11. Mitsala A, Tsalikidis C, Pitiakoudis M, Simopoulos C, Tsaroucha AK. Artificial intelligence in colorectal cancer screening, diagnosis and treatment. A new era. Curr Oncol (2021) 28(3):1581–607. doi: 10.3390/curroncol28030149
12. Schmidt C. M. D. Anderson breaks with IBM watson, raising questions about artificial intelligence in oncology. J Natl Cancer Inst (2017) 109(5):djx113. doi: 10.1093/jnci/djx113
13. Qu WF, Tian MX, Lu HW, Zhou YF, Liu WR, Tang Z, et al. Development of a deep pathomics score for predicting hepatocellular carcinoma recurrence after liver transplantation. Hepatol Int (2023) 17(4):927–41. doi: 10.1007/s12072-023-10511-2
14. Hu W, Zhang X, Saber A, Cai Q, Wei M, Wang M, et al. Development and validation of a nomogram model for lung cancer based on radiomics artificial intelligence score and clinical blood test data. Front Oncol (2023) 13:1132514. doi: 10.3389/fonc.2023.1132514
15. Yang Y, Yu J, Hu J, Zhou C, Niu J, Ma H, et al. A systematic and comprehensive analysis of colorectal squamous cell carcinoma: Implication for diagnosis and treatment. Cancer Med (2022) 11(12):2492–502. doi: 10.1002/cam4.4616
16. Wang J, Liu Y, Zhang R, Liu Z, Yi Z, Guan X, et al. Multi-omics fusion analysis models with machine learning predict survival of HER2-negative metastatic breast cancer: a multicenter prospective observational study. Chin Med J (Engl) (2023) 136(7):863–5. doi: 10.1097/CM9.0000000000002625
17. Colorectal Surgery Group of the Surgery Branch in the Chinese Medical Association; Beihang University State Key Laboratory of Virtual Reality Technology and Systems. Expert consensus on the surgical clinical application of rectal cancer neoadjuvant therapy effect evaluation system based on artificial intelligence platform (2022 edition). Zhonghua Wai Ke Za Zhi (2022) 60(8):732–5. doi: 10.3760/cma.j.cn112139-20220326-00125
18. Miranda J, Horvat N, Assuncao AN Jr., de M Machado FA, Chakraborty J, Pandini RV, et al. MRI-based radiomic score increased mrTRG accuracy in predicting rectal cancer response to neoadjuvant therapy. Abdom Radiol (NY) (2023) 48(6):1911–20. doi: 10.1007/s00261-023-03898-x
19. Truelsen CG, Kronborg CS, Sørensen BS, Callesen LB, Spindler KG. Circulating cell-free DNA as predictor of pathological complete response in locally advanced rectal cancer patients undergoing preoperative chemoradiotherapy. Clin Transl Radiat Oncol (2022) 36:9–15. doi: 10.1016/j.ctro.2022.06.002
20. Bando H, Tsukada Y, Inamori K, Togashi Y, Koyama S, Kotani D, et al. Preoperative Chemoradiotherapy plus Nivolumab before Surgery in Patients with Microsatellite Stable and Microsatellite Instability-High Locally Advanced Rectal Cancer. Clin Cancer Res (2022) 28(6):1136–46. doi: 10.1158/1078-0432.CCR-21-3213
21. Sekizawa K, Nakagawa K, Ichikawa Y, Suwa H, Ozawa M, Momiyama M, et al. Relationship between stromal regulatory T cells and the response to neoadjuvant chemotherapy for locally advanced rectal cancer. Surg Today (2022) 52(2):198–206. doi: 10.1007/s00595-021-02311-8
22. Tamburini E, Tassinari D, Ramundo M, De Stefano A, Viola MG, Romano C, et al. Adjuvant chemotherapy after neoadjuvant chemo-radiotherapy and surgery in locally advanced rectal cancer. A systematic review of literature with a meta-analysis of randomized clinical trials. Crit Rev Oncol Hematol (2022) 172:103627. doi: 10.1016/j.critrevonc.2022.103627
23. Bachet JB, Benoist S, Mas L, Huguet F. Neoadjuvant treatment for rectal cancer. Bull Cancer (2021) 108(9):855–67. doi: 10.1016/j.bulcan.2021.03.018
24. Benson AB, Venook AP, Al-Hawary MM, Azad N, Chen YJ, Ciombor KK, et al. Rectal cancer, version 2.2022, NCCN clinical practice guidelines in oncology. J Natl Compr Canc Netw (2022) 20(10):1139–67. doi:10.6004/jnccn.2022.0051
25. Kumar V, Lalotra GS, Sasikala P, Rajput DS, Kaluri R, Lakshmanna K, et al. Addressing binary classification over class imbalanced clinical datasets using computationally intelligent techniques. Healthcare (Basel) (2022) 10(7):1293. doi: 10.3390/healthcare10071293
26. Fitriyani NL, Syafrudinet M, Alfian G, Rhee J. Development of disease prediction model based on ensemble learning approach for diabetes and hypertension. IEEE Access (2019) 7:144777–89. doi: 10.1109/ACCESS.2019.2945129
27. Rana C, Chitre N, Poyekar B, Bide P. Stroke prediction using smote-tomek and neural network, in: 2021 12th International Conference on Computing Communication and Networking Technologies (ICCCNT), Kharagpur, India. (2021), pp. 1–5. doi: 10.1109/ICCCNT51525.2021.9579763
28. Oronsky B, Reid T, Larson C, Knox SJ. Locally advanced rectal cancer: The past, present, and future. Semin Oncol (2020) 47(1):85–92. doi: 10.1053/j.seminoncol.2020.02.001
29. Peng J, Wang W, Jin H, Qin X, Hou J, Yang Z, et al. Develop and validate a radiomics space-time model to predict the pathological complete response in patients undergoing neoadjuvant treatment of rectal cancer: an artificial intelligence model study based on machine learning. BMC Cancer (2023) 23(1):365. doi: 10.1186/s12885-023-10855-w
30. Sun Y, Zhang X, Jin C, Yue K, Sheng D, Zhang T, et al. Prospective, longitudinal analysis of the gut microbiome in patients with locally advanced rectal cancer predicts response to neoadjuvant concurrent chemoradiotherapy. J Transl Med (2023) 21(1):221. doi: 10.1186/s12967-023-04054-1
31. Francis NK, Luther A, Salib E, Allanby L, Messenger D, Allison AS, et al. The use of artificial neural networks to predict delayed discharge and readmission in enhanced recovery following laparoscopic colorectal cancer surgery. Tech Coloproctol (2015) 19(7):419–28. doi: 10.1007/s10151-015-1319-0
32. Song C, Chung JH, Kang SB, Kim DW, Oh HK, Lee HS, et al. Impact of tumor regression grade as a major prognostic factor in locally advanced rectal cancer after neoadjuvant chemoradiotherapy: A proposal for a modified staging system. Cancers (Basel) (2018) 10(9):319. doi: 10.3390/cancers10090319
33. Kourou K, Exarchos TP, Exarchos KP, Karamouzis MV, Fotiadis DI. Machine learning applications in cancer prognosis and prediction. Comput Struct Biotechnol J (2015) 13:8–17. doi: 10.1016/j.csbj.2014.11.005
34. Pudjihartono N, Fadason T, Kempa-Liehr AW, O'Sullivan JM. A review of feature selection methods for machine learning-based disease risk prediction. Front Bioinform (2022) 2:927312. doi: 10.3389/fbinf.2022.927312
35. Moureau-Zabotto L, Farnault B, de Chaisemartin C, Esterni B, Lelong B, Viret F, et al. Predictive factors of tumor response after neoadjuvant chemoradiation for locally advanced rectal cancer. Int J Radiat Oncol Biol Phys (2011) 80(2):483–91. doi: 10.1016/j.ijrobp.2010.02.025
36. Wang H, Hong R, Niu G, Hu Z, Ke C. Clinical study on risk factors related to postoperative recurrence or metastasis of rectal cancer: a retrospective cohort study. J Gastrointest Oncol (2022) 13(6):2973–88. doi: 10.21037/jgo-22-942
Keywords: deep learning, artificial intelligence, total mesorectal excision, neoadjuvant chemoradiotherapy, local advanced rectal cancer
Citation: Hu J, Sheng Y, Ma J, Tang Y, Liu D, Zhang J, Wei X, Yang Y, Liu Y, Zhang Y and Wang G (2024) Construction and validation of a progression prediction model for locally advanced rectal cancer patients received neoadjuvant chemoradiotherapy followed by total mesorectal excision based on machine learning. Front. Oncol. 13:1231508. doi: 10.3389/fonc.2023.1231508
Received: 20 June 2023; Accepted: 28 December 2023;
Published: 24 January 2024.
Edited by:
Marco Rengo, Sapienza University of Rome, ItalyReviewed by:
Cibele Masotti, Hospital Sirio Libanes, BrazilCharles Ternent, Methodist Physicians Clinic, United States
Copyright © 2024 Hu, Sheng, Ma, Tang, Liu, Zhang, Wei, Yang, Liu, Zhang and Wang. This is an open-access article distributed under the terms of the Creative Commons Attribution License (CC BY). The use, distribution or reproduction in other forums is permitted, provided the original author(s) and the copyright owner(s) are credited and that the original publication in this journal is cited, in accordance with accepted academic practice. No use, distribution or reproduction is permitted which does not comply with these terms.
*Correspondence: Yueping Liu, bGl1eXBAaGVibXUuZWR1LmNu; Yongqiang Zhang, enlxQGhlYnVzdC5lZHUuY24=; Guiying Wang, d2FuZ2d1aXlpbmdAaGVibXUuZWR1; d2FuZ2d1aXlpbmd0Z3p5QDE2My5jb20=