- 1Department of Breast Surgery, Liaoning Cancer Hospital & Institution, Shenyang, Liaoning, China
- 2Department of Breast Surgery, General Surgery Center, The First Hospital of Jilin University, Changchun, China
- 3School of Pharmaceutical Sciences, Wuhan University, Wuhan, China
- 4Department of Neurology and Neuroscience Center, The First Hospital of Jilin University, Changchun, China
- 5Department of Thoracic Surgery, Liaoning Cancer Hospital & Institution, Shenyang, Liaoning, China
Background: The newly discovered pan-immune-inflammation value (PIV) has been illustrated to have good prognostic value for cancer patient prognosis. However, the prognostic usefulness of PIV in breast cancer patients is unknown. As a result, to aid the clinic in providing a distinctive and trustworthy biomarker to better assess breast cancer patient’s prognosis, we conducted this meta-analysis to investigate the relationship between PIV and the survival of breast cancer patients.
Methods: We conducted a systematic search of Pubmed, Embase, the Cochrane Library, and the CNKI databases to screen for eligible studies published up to April 2023. Outcomes included overall survival (OS), progression-free survival (PFS), and pathological complete response (pCR). The hazard ratio (HR) and the corresponding 95% confidence interval (CI) were used as the indicators. STATA 15.0 software was used to perform meta-analysis, sensitivity analysis, and publication bias analysis.
Results: A total of eight articles, involving 2953 patients, met the inclusion criteria and were included in this meta-analysis. The results showed that patients with higher PIV levels had a significantly shorter OS (HR: 2.045, 95% CI: 1.355-3.086, P = 0.001) and PFS (HR: 1.466, 95% CI: 1.163-1.848, P = 0.001). Besides, the PIV value was negatively correlated with the efficacy of neoadjuvant chemotherapy. Sensitivity analysis showed that the results of this study were reliable and stable.
Conclusions: PIV has a good prognostic value in breast cancer patients and is expected to be a prognostic biomarker for breast cancer.
1 Introduction
Globally, breast cancer has surpassed all other types of cancer in frequency among women (1, 2). 2.26 million new cases of breast cancer and 680,000 deaths among women worldwide were reported in 2020 (3). In addition, the incidence of breast cancer tends to increase year over year (4). Due to the ongoing breakthrough and success of systemic therapy, the mortality rate of breast cancer patients has decreased in recent years, and patient survival has increased (5, 6). Unfortunately, a subset of patients still has a poor prognosis. It is well understood that there is significant interindividual variation in treatment response, even among patients with breast cancer at the same clinical stage who receive the same treatment (7). Because of this prognostic heterogeneity (8, 9), it is necessary to identify appropriate prognostic parameters to better predict patient prognosis, personalize breast cancer treatment, and improve survival rates.
At present, many pieces of evidence have shown that the immune-inflammatory response of the host and the tumor interact in a complicated manner (10, 11). To a certain extent, the inflammatory reaction can promote the development of cancer (12). In addition, inflammation, particularly chronic inflammation that is constantly stimulated, can lead to immune tolerance in the cells that have been wounded, allowing mutant cells to evade immune surveillance and facilitate the invasion of cancer (13). Inflammation is directly linked to the development, invasion, and metastasis of malignancies (14, 15). In recent years, the diagnostic and therapeutic value of inflammatory markers has been studied, which provides us with new research ideas for cancer treatment. Numerous inflammatory indicators, including neutrophil-to-lymphocyte ratio (NLR), platelet-to-lymphocyte ratio (PLR), and system immune-inflammatory index (SII), are highly predictive of cancer (16–20).
The-pan-immune-inflammation value (PIV), a parameter calculated from platelet, neutrophil, monocyte, and lymphocyte counts, is a newly defined inflammation-related index. PIV was initially researched in patients with metastatic colorectal cancer in 2020, and the findings demonstrated that PIV had a substantial prognostic value for patients’ survival as well as that its predictive efficiency was significantly greater than that of previously known inflammation-related markers (21).
The connection between PIV and the prognosis of breast cancer patients has been the subject of some retrospective investigations so far. However, the findings were inconsistent. Sahin et al. found that patients with low PIV had a better prognosis (22). Instead, the results of Truffi et al. showed that PIV was not linked to the prognosis of women suffering from breast cancer (23). A systematic evaluation is currently lacking, and it is still unknown what role PIV has in predicting prognosis in breast cancer. Therefore, we designed the first meta-analysis to investigate whether PIV could be a reliable prognostic biomarker in patients with breast cancer.
2 Methods
2.1 Literature search strategies
The proposal for this meta-analysis was drafted following the project guidance of the PRISMA statement (24). The PubMed, EMBASE, the Cochrane Library, and the CNKI were searched using the following keywords: “pan-immune-inflammation-value”, “PIV”, “breast neoplasms”, “breast tumor”, and “breast cancer”, in April 2023. For detailed search strategies, see Table S1. We also searched the grey literature on Google Scholar. The reference lists of the publications that meet preset criteria are manually retrieved to ensure the comprehensiveness of the data.
2.2 Inclusion and exclusion criteria
The pre-established inclusion criteria for this study were as follows: patients with a diagnosis of breast cancer; the prognostic value of PIV was assessed; any of the three outcomes, overall survival (OS), pathological complete response (pCR), and progression-free survival (PFS), was available; The clinical data were complete, and the follow-up data were complete and reliable. Apart from case reports, conference abstracts, and comments. The literature with unpublished endpoints or ambiguous statistics was also removed.
2.3 Data extraction and quality assessment
The following data were extracted from the baseline characteristics of the included studies: author, year, study region, study design, study period, sample size, patient age, treatment, cut-off, and outcome measures. To rate the caliber of observational studies, we used the Newcastle-Ottawa Scale (NOS) results. The NOS consists of three parts: selection (0-4 points), comparability (0-2 points), and outcome assessment (0-3 points). Articles with a NOS score of more than 6 were rated as high quality. Xiaoyan Qi and Qi Jin separately judged the risk of bias in the literature and double-checked the results. In the event of disagreement, it will be decided by discussion or by seeking help from a third party.
2.4 Statistical methods
All the data included in this meta-analysis were analyzed using Stata 15.0 software. For studies that provided both multivariable analysis and univariate analysis, we gave priority to extracting the former. The chi-square test was utilized to evaluate the heterogeneity between studies. When P < 0.1 and I²> 50%, it indicated the presence of heterogeneity and was appropriate to employ a random effects model, whereas the fixed effect model was applied. A sensitivity analysis was performed by removing a single study. Publication bias was assessed using Begg’s and Egger’s tests (25, 26).
3 Results
3.1 Characteristics of studies
A total of 57 replicate studies were eliminated after an initial search. With a rigorous examination of the abstracts and titles, 137 items were eliminated. The remaining 20 articles’ entire texts were then assessed in further detail. There were 8 articles involving 2953 patients (21, 22, 27–32). Figure 1 displays the PRISMA flowchart.
Table 1 lists the basic characteristics of the research that was included. Seven of the nine studies included the outcome OS, five included PFS, and two included pCR. Furthermore, four studies were undertaken in Italy, one in Japan, and two in China and Turkey. According to reports, the cutoff point for PIV was between 205.1 and 438.7. Eight articles earned NOS values ranging from 6 to 8, indicating a low probability of bias.
3.2 PIV and OS
The association between PIV and OS was examined using prognostic data from seven studies involving 2564 breast cancer patients. As shown in Figure 2, the pooled HR was 2.045 (95% CI: 1.355-3.086, P = 0.001), indicating that a high PIV increased the chance of mortality by 104.5%. We discovered significant heterogeneity amongst the included studies, so we used a random effect model (I² = 55.7%, P = 0.035).
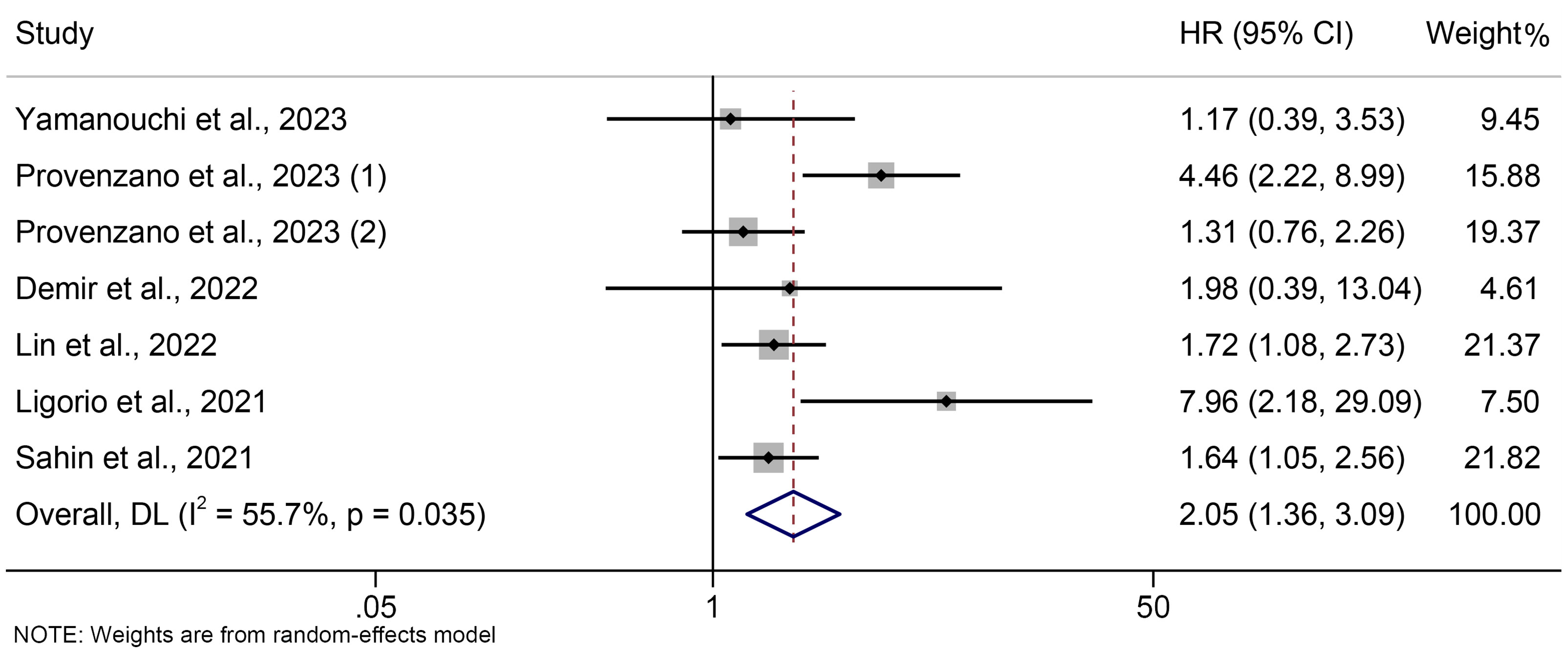
Figure 2 The association between PIV levels and OS. OS, overall survival; HR, hazard ratio; CL, confidence interval; PIV, pan-immune-inflammation value.
The subgroup analyses were carried out in line with the type of analysis. The data demonstrated that the univariate and multivariate meta-results were consistent (Figure 3).
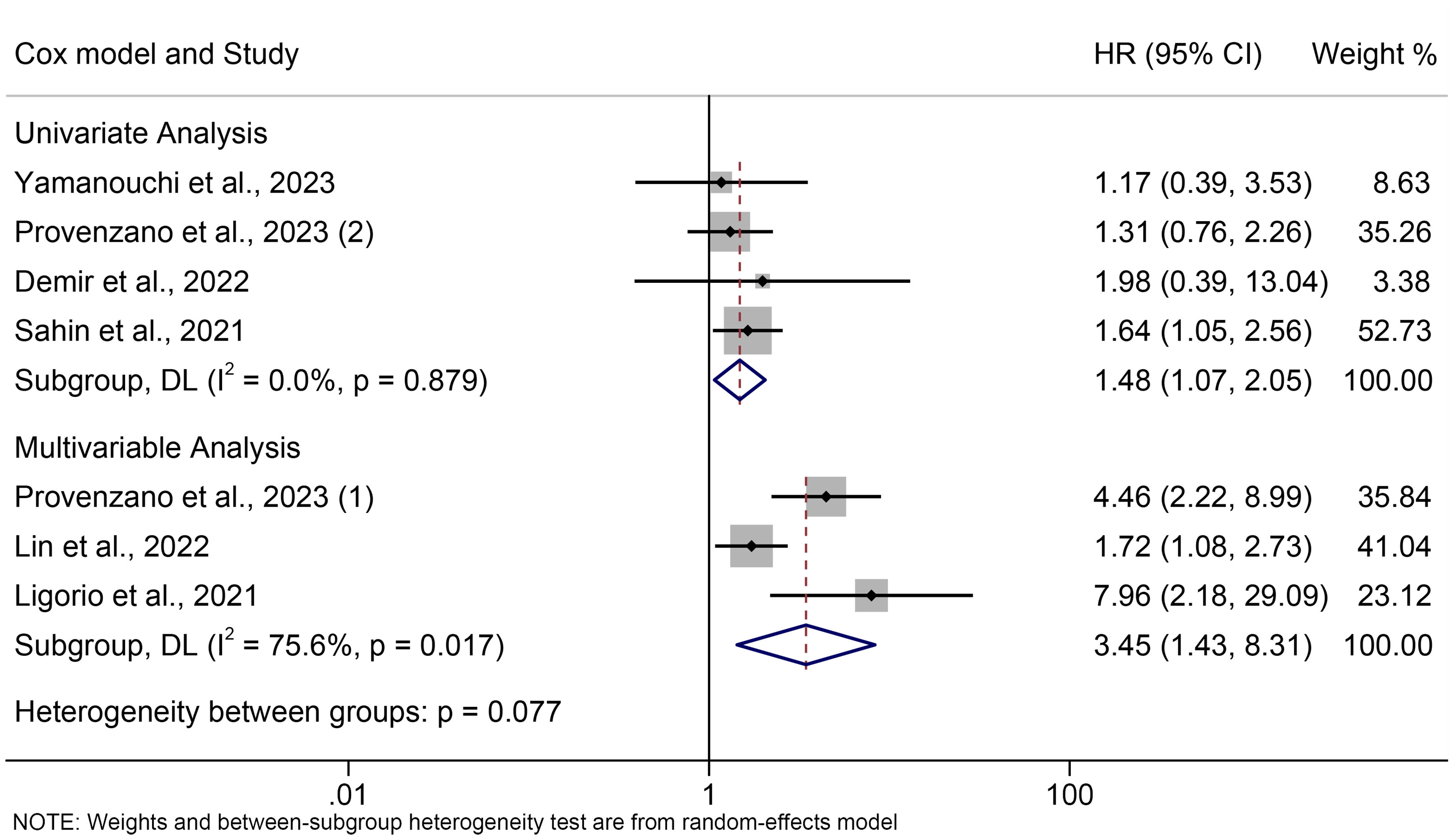
Figure 3 Subgroup analysis of the association between PIV levels and OS. OS, overall survival; HR, hazard ratio; CL, confidence interval; PIV, pan-immune-inflammation value.
3.3 PIV and PFS
Five trials involving 1191 people were analyzed to identify the relationship between PIV and PFS in breast cancer patients. A fixed effects model was applied since there was no discernible heterogeneity in the included trials (I² =21.0%, P = 0.281, Figure 4). Compared to persons with low PIV, those with high PIV had a shorter PFS, according to the assessments (HR = 1.466, 95% CI: 1.163-1.848, P = 0.001, Figure 4).
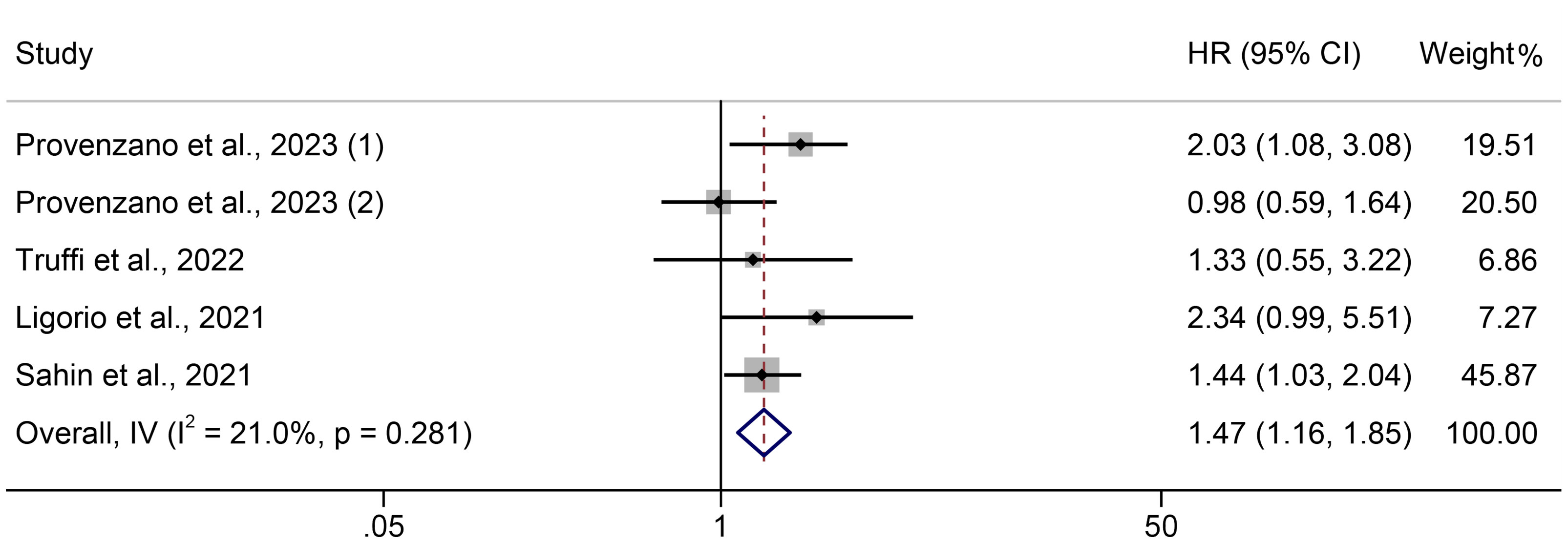
Figure 4 The association between PIV levels and PFS. PFS, progression-free survival; HR, hazard ratio; CL, confidence interval; PIV, pan-immune-inflammation value.
3.4 PIV and pCR
Two of these studies investigated the correlation between PIV and the response to neoadjuvant chemotherapy in breast cancer. In Sahin’s study, PIV was analyzed as a binary variable, while in Geng’s study, PIV was treated as a continuous variable. As a result of the significant heterogeneity between studies, the two studies could not be meta-analyzed. A systematic review was conducted to evaluate whether the evaluation of PIV can predict the pCR to neoadjuvant chemotherapy in breast cancer patients.
Sahin et al. surveyed 743 breast cancer patients in Turkey who underwent NAC and proved that PIV was an independent predictor of NAC response (OR = 3.32; 95% CI: 1.53-7.21, P = 0.002). Surprisingly, PIV was found to be superior to other blood-borne inflammation-related indicators in predicting response to NAC in Turkish women with breast cancer for the first time (22). Geng et al. gathered data from 172 breast cancer patients who underwent NAC and completed the operation. Multivariate analysis revealed that a low PIV (OR = 0.996, 95% CI = 0.993-1.000, P < 0.05) was an independent favorable factor for neoadjuvant chemotherapy efficacy (30). Both studies confirmed the predictive value of PIV for the response to neoadjuvant therapy in breast cancer.
3.5 Sensitivity analysis
The leave-one-out approach was used to conduct a sensitivity analysis. By omitting one trial at a time, we discovered that the pooled HR for OS did not change significantly, varying from 1.697 (95% CI: 1.218-2.365, after excluding Provenzano et al., 2023(1)) to 2.292 (95% CI: 1.419-3.702, after excluding Provenzano et al., 2023(2), Figure 5A). Furthermore, the pooled HR for PFS did not change considerably, ranging from 1.355 (95% CI: 1.047-1.754, after eliminating Provenzano et al., 2023(1)) to 1.626 (95% CI: 1.255-2.108, after omitting Provenzano et al., 2023(2), Figure 5B). The above results indicate that our results are robust and credible.
3.6 Publication bias
There was no evidence of publication bias for OS (Egger’s test: P = 0.364, Begg’s test: P = 0.548) or PFS (Egger’s test: P = 0.689, Begg’s test: P = 1.000). From that, we can see that our outcome was robust.
4 Discussion
The purpose of our study was to investigate the prognostic significance of PIV in patients with breast cancer. To the best of our knowledge, the present study is the first meta-analysis to evaluate the association between PIV and breast cancer survival outcomes. In this meta-analysis involving 2953 breast cancer patients, a clear relationship between higher PIV and shorter OS and PFS was observed. Of note, we also found that PIV showed excellent predictive value for NAC efficacy in breast cancer, with low levels of PIV being an independent favorable factor for NAC efficacy. Considering that PIV does not belong to the same variable type in the two included studies, resulting in too much heterogeneity and not being able to guarantee the stability of the results, only a qualitative description is carried out in this meta-analysis, which also needs to be further verified by more follow-up studies.
There is a strong link between inflammation and cancer (33, 34). On the one hand, an inflammatory response can, to a certain extent, lead to the occurrence of cancer (35, 36). On the other hand, cancer that has occurred can also stimulate the development of inflammation because of various genetic and carcinogenic changes, providing an environment for cancer development and further promoting the process of cancer (12). Inflammation can promote the proliferation and survival of malignant tumor cells, promote the generation and metastasis of blood vessels, weaken the body’s immune response, and change the body’s response to hormones and drugs. Therefore, inflammation makes survival shorter, leading to a poor patient prognosis (37–41). Although tumor activity and patient prognosis may be somewhat predicted by inflammatory conditions, the use of conventional tissue-based tumor-related biomarkers in clinical practice is constrained by issues like challenging specimen collection and high cost (42). Complete blood count (CBC)-based biomarkers were first introduced by Roman Zahorec in 2001 to address this limitation, with the advantages of easy availability and low cost (43). Counts of lymphocytes, neutrophils, platelets, and monocytes are all included in a complete blood count. Over the past decade, there has been substantial evidence that CBC-based biomarkers, such as NLR and PLR, have good prognostic value for a variety of cancers (44–47).
Some studies have found that lymphocyte has anti-tumor immune activity, can inhibit tumor cell growth, and stimulate tumor cell apoptosis (48). Neutrophils can secrete tumor growth factors, which can accelerate the proliferation and migration of tumor cells, and inhibit the anti-tumor activity of lymphocytes to a certain extent (49). Monocytes can differentiate into tumor-associated macrophages, and most of the macrophages can produce angiogenic factors to promote the formation of tumor neovascularization (50). In addition, proteases secreted by monocytes degrade the extracellular matrix to a certain extent. Platelets can enhance the defense role of tumor cells against immune cells, secrete growth factors, and release activators, thus accelerating the proliferation of tumor cells (51). PIV is a newly defined inflammation-related index. It is calculated as neutrophil count (109/L) × monocyte count (109/L) × platelet count (109/L)/lymphocyte count (109/L). As mentioned above, different blood cell populations reflect and regulate different aspects of anti-tumor resistance. In contrast to earlier indices (NLR and PLR), PIV combines all routinely assessed blood cell populations that reflect systemic inflammation and immunity. As a result, it provides a more complete picture of the host’s condition and may have a more reliable prognostic value for cancer. PIV has been shown by Ligorio et al. to be a unique and useful predictor of OS in advanced BC patients with HER-2 positivity who were given first-line trastuzumab-pertuzumab biochemotherapy (29). Sahin et al. also found that PIV had better predictive power than NLR, PLR, monocyte-to-lymphocyte ratio (MLR), and systemic immune inflammation index (SII) in breast cancer patients (22). More surprisingly, Lin et al. observed that PIV predicted OS with higher accuracy than traditional TNM staging systems (27). Therefore, compared with other biomarkers, PIV may have better prognostic value and clinical utility in breast cancer patients. In conclusion, our study finds that PIV can be a useful tool for predicting poor outcomes in breast cancer patients. Individualized and timely immunization interventions may improve outcomes in patients with high baseline PIV.
Our analysis still has certain limitations. The number of patients included in this study was relatively limited. It was also not possible to further evaluate the correlation between PIV and the prognosis of patients with different molecular subtypes of breast cancer. This meta-analysis included studies that only used PIV values at a single time point and lacked dynamic assessment, which was an important limitation. The included studies were heterogeneous in terms of tumor type and treatment type, reducing the generalizability of the results. In addition, there is a very important point: because PIV has different reference values in studies conducted in different populations around the world, there is no uniform cut-off value, which may cause the final summary results to be different from the actual value. Therefore, more high-quality studies with large sample sizes, especially multicenter RCTs, are needed to verify and refresh our conclusions. And these studies should include patients from different regions and ethnicities, and discuss uniform cutoff values to open the way for the clinical application of this biomarker.
Data availability statement
The original contributions presented in the study are included in the article/Supplementary Material. Further inquiries can be directed to the corresponding author.
Author contributions
XQ, BQ, and DL conceived and designed the study. XQ, BQ, TS, DH, HZ, YL, QJ, and MY were responsible for the collection and assembly of data, data analysis, and interpretation. XQ and BQ were involved in writing the manuscript. XQ, BQ, and DL revised the manuscript. All the work was performed under DL instructions. All authors contributed to the article and approved the submitted version.
Acknowledgments
The authors thank all the medical staff who contributed to the maintenance of the medical record database.
Conflict of interest
The authors declare that the research was conducted in the absence of any commercial or financial relationships that could be construed as a potential conflict of interest.
Publisher’s note
All claims expressed in this article are solely those of the authors and do not necessarily represent those of their affiliated organizations, or those of the publisher, the editors and the reviewers. Any product that may be evaluated in this article, or claim that may be made by its manufacturer, is not guaranteed or endorsed by the publisher.
Supplementary material
The Supplementary Material for this article can be found online at: https://www.frontiersin.org/articles/10.3389/fonc.2023.1223786/full#supplementary-material
Supplementary material 1 | The detailed search strategies for Pubmed.
References
1. Arnold M, Morgan E, Rumgay H, Mafra A, Singh D, Laversanne M, et al. Current and future burden of breast cancer: Global statistics for 2020 and 2040. Breast (2022) 66:15–23. doi: 10.1016/j.breast.2022.08.010
2. Loibl S, Poortmans P, Morrow M, Denkert C, Curigliano G. Breast cancer. Lancet (2021) 397(10286):1750–69. doi: 10.1016/S0140-6736(20)32381-3
3. Sung H, Ferlay J, Siegel RL, Laversanne M, Soerjomataram I, Jemal A, et al. Global cancer statistics 2020: GLOBOCAN estimates of incidence and mortality worldwide for 36 cancers in 185 countries. CA Cancer J Clin (2021) 71(3):209–49. doi: 10.3322/caac.21660
4. Siegel RL, Miller KD, Fuchs HE, Jemal A. Cancer statistics, 2022. CA Cancer J Clin (2022) 72(1):7–33. doi: 10.3322/caac.21708
5. Sun YS, Zhao Z, Yang ZN, Xu F, Lu HJ, Zhu ZY, et al. Risk factors and preventions of breast cancer. Int J Biol Sci (2017) 13(11):1387–97. doi: 10.7150/ijbs.21635
6. Siegel RL, Miller KD, Wagle NS, Jemal A. Cancer statistics, 2023. CA Cancer J Clin (2023) 73(1):17–48. doi: 10.3322/caac.21763
7. Parekh T, Dodwell D, Sharma N, Shaaban AM. Radiological and pathological predictors of response to neoadjuvant chemotherapy in breast cancer: A brief literature review. Pathobiology (2015) 82(3-4):124–32. doi: 10.1159/000433582
8. Yeo SK, Guan JL. Breast cancer: multiple subtypes within a tumor? Trends Cancer (2017) 3(11):753–60. doi: 10.1016/j.trecan.2017.09.001
9. Li X, Zhou J, Xiao M, Zhao L, Zhao Y, Wang S, et al. Uncovering the subtype-specific molecular characteristics of breast cancer by multiomics analysis of prognosis-associated genes, driver genes, signaling pathways, and immune activity. Front Cell Dev Biol (2021) 9:689028. doi: 10.3389/fcell.2021.689028
10. Zhao H, Wu L, Yan G, Chen Y, Zhou M, Wu Y, et al. Inflammation and tumor progression: signaling pathways and targeted intervention. Signal Transduct Target Ther (2021) 6(1):263. doi: 10.1038/s41392-021-00658-5
11. Grivennikov SI, Wang K, Mucida D, Stewart CA, Schnabl B, Jauch D, et al. Adenoma-linked barrier defects and microbial products drive IL-23/IL-17-mediated tumour growth. Nature (2012) 491(7423):254–8. doi: 10.1038/nature11465
12. Swierczak A, Mouchemore KA, Hamilton JA, Anderson RL. Neutrophils: important contributors to tumor progression and metastasis. Cancer Metastasis Rev (2015) 34(4):735–51. doi: 10.1007/s10555-015-9594-9
13. Yuan Q, Deng D, Pan C, Ren J, Wei T, Wu Z, et al. Integration of transcriptomics, proteomics, and metabolomics data to reveal HER2-associated metabolic heterogeneity in gastric cancer with response to immunotherapy and neoadjuvant chemotherapy. Front Immunol (2022) 13:951137. doi: 10.3389/fimmu.2022.951137
14. Greten FR, Grivennikov SI. Inflammation and cancer: triggers, mechanisms, and consequences. Immunity (2019) 51(1):27–41. doi: 10.1016/j.immuni.2019.06.025
15. Mantovani A. Cancer: inflammation by remote control. Nature (2005) 435(7043):752–3. doi: 10.1038/435752a
16. Moon G, Noh H, Cho IJ, Lee JI, Han A, Noh H, Cho IJ, Lee JI, Han A. Prediction of late recurrence in patients with breast cancer: elevated neutrophil to lymphocyte ratio (NLR) at 5 years after diagnosis and late recurrence. Breast Cancer (2020) 27(1):54–61. doi: 10.1007/s12282-019-00994-z
17. Li S, Xu X, Liang D, Tian G, Song S, He Y. [Prognostic value of blood neutrophil-to-lymphocyte ratio (NLR) and platelet-to-lymphocyte ratio (PLR) in patients with gastric cancer]. Zhonghua Zhong Liu Za Zhi (2014) 36(12):910–5.
18. Ding P, Guo H, Sun C, Yang P, Kim NH, Tian Y, et al. Combined systemic immune-inflammatory index (SII) and prognostic nutritional index (PNI) predicts chemotherapy response and prognosis in locally advanced gastric cancer patients receiving neoadjuvant chemotherapy with PD-1 antibody sintilimab and XELOX: a prospective study. BMC Gastroenterol (2022) 22(1):121. doi: 10.1186/s12876-022-02199-9
19. Jin J, Yang L, Liu D, Li W. Association of the neutrophil to lymphocyte ratio and clinical outcomes in patients with lung cancer receiving immunotherapy: a meta-analysis. BMJ Open (2020) 10(6):e035031. doi: 10.1136/bmjopen-2019-035031
20. Savioli F, Morrow ES, Dolan RD, Romics L, Lannigan A, Edwards J, et al. Prognostic role of preoperative circulating systemic inflammatory response markers in primary breast cancer: meta-analysis. Br J Surg (2022) 109(12):1206–15. doi: 10.1093/bjs/znac319
21. Fucà G, Guarini V, Antoniotti C, Morano F, Moretto R, Corallo S, et al. The Pan-Immune-Inflammation Value is a new prognostic biomarker in metastatic colorectal cancer: results from a pooled-analysis of the Valentino and TRIBE first-line trials. Br J Cancer (2020) 123(3):403–9. doi: 10.1038/s41416-020-0894-7
22. Şahin AB, Cubukcu E, Ocak B, Deligonul A, Oyucu Orhan S, Tolunay S, et al. Low pan-immune-inflammation-value predicts better chemotherapy response and survival in breast cancer patients treated with neoadjuvant chemotherapy. Sci Rep (2021) 11(1):14662. doi: 10.1038/s41598-021-94184-7
23. Truffi M, Sottotetti F, Gafni N, Albasini S, Piccotti F, Morasso C, et al. Prognostic potential of immune inflammatory biomarkers in breast cancer patients treated with neoadjuvant chemotherapy. Cancers (Basel) (2022) 14(21):5287. doi: 10.3390/cancers14215287
24. Moher D, Liberati A, Tetzlaff J, Altman DG. Preferred reporting items for systematic reviews and meta-analyses: the PRISMA Statement. Open Med (2009) 3(3):e123–30.
25. Zhang L, Jin Q, Chai D, Kuang T, Li C, Guan Y, et al. The correlation between probiotic use and outcomes of cancer patients treated with immune checkpoint inhibitors. Front Pharmacol (2022) 13:937874. doi: 10.3389/fphar.2022.937874
26. Zhang L, Chen C, Chai D, Li C, Kuang T, Liu L, et al. Effects of PPIs use on clinical outcomes of urothelial cancer patients receiving immune checkpoint inhibitor therapy. Front Pharmacol (2022) 13:1018411. doi: 10.3389/fphar.2022.1018411
27. Lin F, Zhang LP, Xie SY, Huang HY, Chen XY, Jiang TC, et al. Pan-immune-inflammation value: A new prognostic index in operative breast cancer. Front Oncol (2022) 12:830138. doi: 10.3389/fonc.2022.830138
28. Demir H, Demirci A, Eren SK, Beypinar I, Davarcı SE, Baykara M, et al. A new prognostic index in young breast cancer patients. J Coll Physicians Surg Pak (2022) 32(1):86–91. doi: 10.29271/jcpsp.2022.01.86
29. Ligorio F, Fucà G, Zattarin E, Lobefaro R, Zambelli L, Leporati R, et al. The pan-immune-inflammation-value predicts the survival of patients with human epidermal growth factor receptor 2 (HER2)-positive advanced breast cancer treated with first-line taxane-trastuzumab-pertuzumab. Cancers (Basel) (2021) 13(8):1964. doi: 10.3390/cancers13081964
30. Geng Y. Correlation between peripheral blood Inflammation Indexes and efficacy of neoadjuvant chemotherapy for breast cancer. Jilin University (2022).
31. Provenzano L, Lobefaro R, Ligorio F, Zattarin E, Zambelli L, Sposetti C, et al. The pan-immune-inflammation value is associated with clinical outcomes in patients with advanced TNBC treated with first-line, platinum-based chemotherapy: an institutional retrospective analysis. Ther Adv Med Oncol (2023) 15:17588359231165978. doi: 10.1177/17588359231165978
32. Yamanouchi K, Murakami S, Sato A, Ogawa S, Shinagawa H, Kamohara Y, et al. Integrated evaluation of inflammatory, nutritional, and sarcopenia markers to predict survival in metastatic breast cancer patients. In Vivo (2023) 37(2):811–7. doi: 10.21873/invivo.13146
33. Balkwill F, Mantovani A. Inflammation and cancer: back to Virchow? Lancet (2001) 357(9255):539–45. doi: 10.1016/S0140-6736(00)04046-0
34. Mantovani A, Allavena P, Sica A, Balkwill F. Cancer-related inflammation. Nature (2008) 454(7203):436–44. doi: 10.1038/nature07205
35. Guthrie GJ, Charles KA, Roxburgh CS, Horgan PG, McMillan DC, Clarke SJ. The systemic inflammation-based neutrophil-lymphocyte ratio: experience in patients with cancer. Crit Rev Oncol Hematol (2013) 88(1):218–30. doi: 10.1016/j.critrevonc.2013.03.010
36. Digifico E, Balinzo S, Belgiovine C. The dark side of the force: when the immune system is the fuel of tumor onset. Int J Mol Sci (2021) 22(3):1224. doi: 10.3390/ijms22031224
37. Candido J, Hagemann T. Cancer-related inflammation. J Clin Immunol (2013) 33 Suppl 1:S79–84. doi: 10.1007/s10875-012-9847-0
38. Balkwill F. Immunology for the next generation. Nat Rev Immunol (2005) 5(6):509–12. doi: 10.1038/nri1628
39. Yu PF, Huang Y, Han YY, Lin LY, Sun WH, Rabson AB, et al. TNFα-activated mesenchymal stromal cells promote breast cancer metastasis by recruiting CXCR2(+) neutrophils. Oncogene (2017) 36(4):482–90. doi: 10.1038/onc.2016.217
40. Ye JH, Wang XH, Shi JJ, Yin X, Chen C, Chen Y, et al. Tumor-associated macrophages are associated with response to neoadjuvant chemotherapy and poor outcomes in patients with triple-negative breast cancer. J Cancer (2021) 12(10):2886–92. doi: 10.7150/jca.47566
41. Shibutani M, Maeda K, Nagahara H, Fukuoka T, Nakao S, Matsutani S, et al. The peripheral monocyte count is associated with the density of tumor-associated macrophages in the tumor microenvironment of colorectal cancer: a retrospective study. BMC Cancer (2017) 17(1):404. doi: 10.1186/s12885-017-3395-1
42. Marrugo-Ramírez J, Mir M, Samitier J. Blood-based cancer biomarkers in liquid biopsy: A promising non-invasive alternative to tissue biopsy. Int J Mol Sci (2018) 19(10):2877. doi: 10.3390/ijms19102877
43. Zahorec R. Ratio of neutrophil to lymphocyte counts–rapid and simple parameter of systemic inflammation and stress in critically ill. Bratisl Lek Listy (2001) 102(1):5–14.
44. Zhu Y, Zhou S, Liu Y, Zhai L, Sun X. Prognostic value of systemic inflammatory markers in ovarian Cancer: a PRISMA-compliant meta-analysis and systematic review. BMC Cancer (2018) 18(1):443. doi: 10.1186/s12885-018-4318-5
45. Cho U, Park HS, Im SY, Yoo CY, Jung JH, Suh YJ, et al. Prognostic value of systemic inflammatory markers and development of a nomogram in breast cancer. PloS One (2018) 13(7):e0200936. doi: 10.1371/journal.pone.0200936
46. Sakane T, Okuda K, Matsui T, Oda R, Tatematsu T, Yokota K, et al. Prognostic value of systemic inflammatory markers and the nutrition status in thymic epithelial tumors with complete resection. Thorac Cancer (2022) 13(15):2127–33. doi: 10.1111/1759-7714.14485
47. Kim HJ, Lee KH, Shim HJ, Hwang EC, Choi YD, Bang H, et al. Prognostic significance of the neutrophil-lymphocyte ratio and platelet-lymphocyte ratio in neuroendocrine carcinoma. Chonnam Med J (2022) 58(1):29–36. doi: 10.4068/cmj.2022.58.1.29
48. Galli F, Aguilera JV, Palermo B, Markovic SN, Nisticò P, Signore A. Relevance of immune cell and tumor microenvironment imaging in the new era of immunotherapy. J Exp Clin Cancer Res (2020) 39(1):89. doi: 10.1186/s13046-020-01586-y
49. Xiong S, Dong L, Cheng L. Neutrophils in cancer carcinogenesis and metastasis. J Hematol Oncol (2021) 14(1):173. doi: 10.1186/s13045-021-01187-y
50. Petty AJ, Yang Y. Tumor-associated macrophages: implications in cancer immunotherapy. Immunotherapy (2017) 9(3):289–302. doi: 10.2217/imt-2016-0135
Keywords: pan-immune-inflammation value, breast cancer, prognosis, clinical utility, meta-analysis
Citation: Qi X, Qiao B, Song T, Huang D, Zhang H, Liu Y, Jin Q, Yang M and Liu D (2023) Clinical utility of the pan-immune-inflammation value in breast cancer patients. Front. Oncol. 13:1223786. doi: 10.3389/fonc.2023.1223786
Received: 16 May 2023; Accepted: 14 August 2023;
Published: 30 August 2023.
Edited by:
Eriko Katsuta, Yokohama City University Graduate School of Medicine, JapanReviewed by:
Foteinos-Ioannis Dimitrakopoulos, University of Patras, GreeceJuan Caballero, European Bioinformatics Institute (EMBL-EBI), United Kingdom
Copyright © 2023 Qi, Qiao, Song, Huang, Zhang, Liu, Jin, Yang and Liu. This is an open-access article distributed under the terms of the Creative Commons Attribution License (CC BY). The use, distribution or reproduction in other forums is permitted, provided the original author(s) and the copyright owner(s) are credited and that the original publication in this journal is cited, in accordance with accepted academic practice. No use, distribution or reproduction is permitted which does not comply with these terms.
*Correspondence: Delong Liu, bGl1ZGwxMDAyQDE2My5jb20=
†These authors have contributed equally to this work