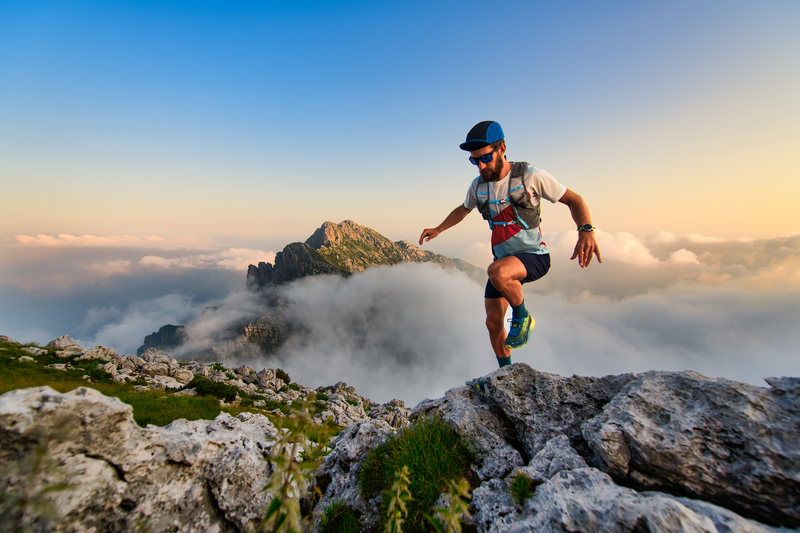
95% of researchers rate our articles as excellent or good
Learn more about the work of our research integrity team to safeguard the quality of each article we publish.
Find out more
ORIGINAL RESEARCH article
Front. Oncol. , 06 November 2023
Sec. Breast Cancer
Volume 13 - 2023 | https://doi.org/10.3389/fonc.2023.1193927
Introduction: Triple-negative breast cancer (TNBC) is a heterogeneous disease associated with a poor prognosis. Delaying in time to start adjuvant chemotherapy (TTC) has been related to an increased risk of distant recurrence-free survival (DRFS). We aimed to develop a prognostic model to estimate the effects of delayed TTC among TNBC risk subgroups.
Materials and methods: We analyzed 687 TNBC patients who received adjuvant chemotherapy at the Instituto Nacional de Enfermedades Neoplasicas (Lima, Peru). Database was randomly divided to create a discovery set (n=344) and a validation set (n=343). Univariate and multivariate Cox regression models were performed to identify prognostic factors for DRFS. Risk stratification was implemented through two models developed based on proportional hazard ratios from significant clinicopathological characteristics. Subpopulation treatment effect pattern plot (STEPP) analysis was performed to determine the best prognostic cut-off points for stratifying TNBC subgroups according to risk scores and estimate Kaplan-Meier differences in 10-year DRFS comparing TTC (≤30 vs.>30 days).
Results: In univariate analysis, patients aged ≥70 years (HR=4.65; 95% CI: 2.32-9.34; p=<0.001), those at stages pT3-T4 (HR=3.28; 95% CI: 1.57-6.83; p=0.002), and pN2-N3 (HR=3.00; 95% CI: 1.90-4.76; p=<0.001) were notably associated with higher risk. STEPP analysis defined three risk subgroups for each model. Model N°01 categorized patients into low (score: 0–31), intermediate (score:32–64), and high-risk (score: 65–100) cohorts; meanwhile, Model N°02: low (score: 0–26), intermediate (score: 27–55), and high (score: 56–100). Kaplan-Meier plots showed that in the discovery set, patients with TTC>30 days experienced a 17.5% decrease in 10-year DRFS rate (95%CI=6.7-28.3), and the impact was more remarkable in patients who belong to the high-risk subgroup (53.3% decrease in 10 years-DRFS rate). Similar results were found in the validation set.
Conclusions: We developed two prognostic models based on age, pT, and pN to select the best one to classify TNBC. For Model N°02, delayed adjuvant chemotherapy conferred a higher risk of relapse in patients ≥70 years and who were characterized by pT3/T4 and pN2/N3. Thus, more efforts should be considered to avoid delayed TTC in TNBC patients, especially those in high-risk subgroups.
Triple-negative breast cancer (TNBC) is a heterogeneous and complex disease characterized by the absence of estrogen receptors (ER), progesterone receptors (PR), and HER2 amplification (1, 2). TNBC could be classified into six different subtypes regarding transcriptional profiling: two basal-like (BL1 and BL2), an immunomodulatory (IM), a mesenchymal (M), a mesenchymal stem-like (MSL), and a luminal AR (LAR) subtype (3).
TNBC represents 10%-20% of all BCs worldwide (4, 5), which amounts to nearly 200,000 cases each year (6). A high prevalence of TNBC has been reported among Hispanic, Afro-American, premenopausal, or carriers of BRCA gene mutations (7, 8). In Latin America, the incidence of TNBC has been described in several countries, such as Mexico (23.1%), Venezuela (24.6%), Brazil (27%), and Peru (21.3%) (9, 10). TNBC patients from these countries usually face socioeconomic barriers to timely access to specialized cancer treatment (1, 11, 12).
TNBC also exhibits a higher probability of early tumor relapse, metastases, and death than patients with other BC subtypes (13, 14). Despite advances in BC treatments, including targeted therapies and immunotherapies, the management of TNBC remains particularly challenging. Unlike hormone receptor-positive or HER2-positive subtypes, TNBC lacks well-defined molecular targets for therapy, limiting the treatment options mainly to chemotherapy (5).
For TNBC patients, treatment mainly consists of surgery and chemotherapy (15). Adjuvant chemotherapy is the standard of care for this BC subtype because it helps to reduce the risk of a distant recurrence and improves overall survival (OS) (16, 17). Yu et al. (2013) reported that the 5-year OS for TNBC patients receiving adjuvant chemotherapy was 85% compared to 76% in those who did not (HR=0.65; 95% CI=0.52-0.81; p<0.001) (18).
In recent years, delayed initiation of adjuvant chemotherapy (TTC) has been associated with a worse prognosis (5). Among TNBC patients, TTC > 4 weeks has been associated with an 89% increase in the risk of recurrence (95% CI= 1.09-3.27; p=0.024) and a 2.49 times increase in the risk of death (95%CI= 1.30-4.76; p=0.006) compared to those with TTC ≤ 4 weeks (18).
Considering the limited access to treatment and irregular outcomes for TNBC patients, conducting studies to select patients who promptly need adjuvant chemotherapy is essential. A Subpopulation Treatment Effect Pattern Plot (STEPP) analysis is an alternative approach to estimating interactions between treatment and a continuous covariate of interest (19). Kaplan-Meier plots provided by this method allow defining cut-off points to identify subpopulations that may respond differently to a treatment. By stratifying patients into risk groups, STEPP can provide insights into personalized treatment decisions, helping optimize the benefit-risk balance for each patient.
In this current study, we aimed to develop a simple and easy-to-use prognostic model based on clinical characteristics to evaluate the effect of TTC on relapse risk among TNBC subgroups.
A retrospective study reported 2007 cases of TNBC diagnosed between January 2000 and December 2014 at the National Institute of Neoplastic Diseases (INEN) in Lima, Peru. Immunohistochemistry (IHC) and fluorescent in situ hybridization (FISH) techniques were utilized to identify TNBC patients.
Antibodies Estrogen Anti-Receptor (Clone 1D5, Dako), Progesterone Anti-Receptor (Clone PGR636, Dako), and Anti-HER2/neu (A0485, Dako) were used to assess IHC analysis. A tumor was defined as negative for either ER or PR if less than 10% of its cells showed any level of nuclear staining. HER2 status was determined following the American Society of Clinical Oncology and College of American Pathologists (ASCO-CAP) guidelines. FISH results corroborated the absence of HER2 protein overexpression. More details about the cohort design have already been reported in previous literature (20).
We only included TNBC patients (stage I to III) who underwent surgery as the first treatment and then received adjuvant chemotherapy. Male patients, inflammatory breast tumor cases, and patients lacking information about tumor size, surgery type, chemotherapy, or surgery dates were excluded. TNBC patients treated with neoadjuvant chemotherapy were not considered in this study.
Medical records were reviewed to obtain information on clinical variables such as age, diagnosis period (2000 – 2004, 2005 – 2009, 2010 – 2014), pathological tumor size (pT) and lymph nodes (pN), histological grade, vascular permeability, and treatment completion. Incomplete chemotherapy was defined as patients receiving less than four cycles of anthracyclines. DRFS was estimated in years from the initial intervention (surgery) to the date of documentation of distant recurrence or last contact with the patients. TTC was calculated by the days between surgery and the first dose of adjuvant chemotherapy. TTC variable was: ≤30 and >30 days.
Database was randomly divided to create a discovery (n=344) and validation set (n=343). Discovery set was used to calculate the proportional hazard ratios strongly associated with DRFS. Univariate and multivariate Cox regression models were conducted to create adjusted models. Clinical characteristics included in those models, such as age, pT and pN, had to be statistically significant in univariate and multivariate Cox analyses. Subsequently, log-rank tests were performed to determine whether HRs from the variables selected were constant over time.
Linear models were developed based on the summation of proportional hazard ratios from the clinicopathological characteristics of each patient (21, 22). Risk scores from all patients were scaled from 0 to 100. STEPP method was used to define appropriate cut-off values to split patients into groups with different DRFS probabilities and estimate the effect of TTC on those risk subgroups, which were based on Kaplan-Meier estimations of 10-year DRFS (y-axis) throughout the composite risk score (x-axis). In previous research, STEPP was also used to determine cut-off values and divided patients with diffuse and/or intestinal-type gastric cancer in risk groups for peritoneal recurrence using R (23). STEPP parameters had a window size of 160 (r2) and 30 (r1) patients with a recommended number of permutations of 2500 for stable results (24). Standard errors at 95%CI were considered to assess the distant recurrence effect.
Subsequently, survival analyses were performed to determine whether the subgroups created by the STEPP analysis were statistically significant to DRFS. Additionally, composite risk values from the discovery and validation sets were compared to clinical stages using multivariate Cox analysis to evaluate which represents a major significant risk in DRFS. Data were analyzed with R software version 4.0.3 using the packages “survival” (version 3.5-5), “survminer” (version 0.4.9), and “stepp” (version 3.2.5).
The study was approved by the Ethics Review Board of the Instituto Nacional de Enfermedades Neoplasicas (INEN 22-03) and conducted in compliance with all relevant ethical guidelines.
In this study, a total of 687 TNBC patients were included. Median age of the population was 48.0 (IQR: 41.0, 57.0) years. Most of them were determined to have a pT2 (62.6%), pN0 (48.8%), and G3 (81.0%). A higher proportion of patients were found to be aged 41-59 years (n=388, 56.5%) and diagnosed between 2005-2009 (n=287, 41.8%). Similar trends were reported in the discovery set (n=344) and validation set (n=343; Table 1).
Univariate and multivariate Cox analysis for clinicopathological characteristics related to DRFS showed that only age, pT, and pN were significantly associated with recurrence (Table 2). Both age stratifications were significant for the outcome. For Univariate analysis, clinicopathologic characteristics that contributed to a higher risk for recurrence were age ≥ 70 years (vs. ≤40/41-59/60-69; HR=4.65; 95%CI:2.32-9.34; p=<0.001), stage pT3-T4 (vs. pT1/T2; HR=3.28; 95%CI:1.57-6.83; p=0.002), followed by pN2-N3 (vs. pN0/N1; HR=3.00, 95%CI:1.90-4.76; p=<0.001) and age ≥ 60 years (vs. ≤40/41-59; HR=2.38; 95%CI:1.36-4.18; p=0.003; Table 2). Evaluation of the proportional hazards (PH) assumption determined that HRs of the three characteristics (age, pT, and pN) were constant over time (Figure S1).
Table 2 Univariate and multivariate analysis of TNBC patients and characteristics clinicopathologic related to DRFS in the discovery set.
The median follow-up period for the discovery set was 9.6 years. Regression models were described according to proportional hazard ratios from the Multivariate analyses:
Equations indicated that the composite risk scores could vary according to the profile of each patient (i). Scores of all patients were scaled from 0 to 100, where 100 implied the patient obtained the maximum value of the equation. According to the STEPP methodology, patients were stratified into three subgroups based on their composite risk values.
For Model N°01, the median composite risk values for the discovery set were 46 (IQR: 30, 55). Low (score: 0 – 31), intermediate (score: 32 – 64), and high risk (score: 65 – 100) (Figure 1A; Table 3). For Model N°02, the median composite risk values were 36 (IQR: 25, 53) and also could classify patients with a low (score: 0 – 26), intermediate (score: 27 – 55), and high risk (score: 56 – 100) (Figure 1B; Table 3). The overall decrease for 10-year DRFS among patients who started adjuvant treatment >30 days vs. those who started ≤ 30 days was 17.5% ± 10.8% for both models (Table 3).
Figure 1 STEPP plots of 10-year DRFS, histograms and Kaplan-Meier curves according to composite risk scores in subgroups according to TTC for the discovery set. (A) Model N°01. (B) Model N°2.
Table 3 10-year DRFS (%). For each analysis, results show three subpopulations at either extreme of the STEPP (lowest and highest composite risk values) as well as the intermediate STEPP subpopulations for both discovery and validation sets.
However, the effects may vary depending on each subpopulation created by the STEPP methodology. For Model N°01, it could be observed that patients who belong to the higher risk subgroup and started chemotherapy ≤30 days experienced an increment in 53.3% ± 28.8% of 10-year DRFS vs. those who initiated adjuvant >30 days (Table 3). Alternatively, in the low-risk subgroup, the DRFS variation was marginal (1.9% ± 13.7%), implying that those with lower risk characteristics in the composite value have similar effects even if they started their adjuvant treatment ≤30 days or >30 days. In Model N°02, the high-risk subgroup initiating chemotherapy within the same temporal frame experienced a 10-year DRFS of 53.4% ± 49.9%, also in contrast to those who initiated treatment later (Table 3). Low-risk subgroup also displayed minimal changes in DRFS, regardless of TTC. In both models, high-risk individuals delaying treatment beyond 30 days could be considered critical regarding the prognostic risk of recurrence.
In Model N°01, Cox Univariate analysis revealed significant risk stratification among patients into three subgroups: Low vs. Intermediate (HR=1.90; 95% CI= 1.17-3.08; p=0.010) and Low vs. High (HR=4.58; 95% CI=2.77-7.56; p=<0.001; Figure 1A; Table 4). Similarly, in Model N°02, significant risk differentiation was observed: Low vs. Intermediate (HR=1.82; 95% CI=1.13-2.95; p=0.015) and Low vs. High (HR=5.29; 95% CI=3.22-8.69; p=<0.001; Figure 1B; Table 4).
Table 4 Univariate Cox analysis of TNBC patients’ distant recurrence in discovery and validation set regarding risk subgroups.
The median follow-up period for the validation set was 10.1 years, and regarding its composite risk values, for Model N°01, the median score was 46 (IQR: 30 - 55). Regarding Model N°02, the median score was 36 (IQR: 25 - 49). Cut-off points for the risk groups from both models were the same as in the discovery set (Figure 2; Table 3).
Figure 2 STEPP plots of 10-year DRFS, histograms and Kaplan-Meier curves according to composite risk scores in subgroups according to TTC for the validation set. (A) Model N°01. (B) Model N°2.
In Model N°01, high-risk patients initiating chemotherapy after 30 days exhibited a 43.8% ± 26.0% absolute decrease in 10-year DRFS rate compared to those who started before 30 days (Table 3). For the low-risk population with delayed treatment, the rate dropped by 5.3% ± 12.1%. Conversely, in Model N°02, the high-risk patients experienced a 22.7% ± 27.6% absolute decrease in the 10-year DRFS rate under the same conditions, while the low-risk group remained consistent at a 5.3% ± 12.1% reduction. The effect was more pronounced for high-risk patients with delayed initiation in both discovery and validation sets for both models (Figures 1, 2).
The risk subgroups were also significant in the validation set for Model N°01: Low vs. Intermediate (HR=2.03; 95%CI=1.25-3.31; p=0.005) and Low vs. High (HR=3.50; 95%CI=2.03-6.05; p=<0.001; Figure 2A; Table 4). However, Model N°02 seems to be a better classifier than the previous one: Low vs. Intermediate (HR=2.48; 95%CI=1.49-4.15; p<0.001) and Low vs. High (HR=4.97; 95%CI=2.79-8.89; p=<0.001; Figure 2B; Table 4).
We developed a prognostic model using clinicopathological characteristics to estimate the individual patient’s risk of distant recurrence and to identify risk subgroups. The relationship between a delay in TTC and a worse prognosis in TNBC has been shown in previous studies (18, 20, 25). However, heterogeneity among TNBC patients requires individual decisions for treatments. The prognostic model developed in this study is based on clinicopathological characteristics that can be easily found in the clinical records of patients with TNBC, such as age, pT, and pN.
The classification of risk subgroups using prognostic models and the STEPP methodology has been developed for clinical trials and treatments for breast cancer (26, 27). For example, among premenopausal women with HR-positive/HER2-negative early breast cancer, a prognostic model based on clinicopathological characteristics was used to identify high-risk subgroups of recurrence. STEPP analysis demonstrated that high-risk patients experienced an absolute decrease of 5% for 8-year distant recurrence–free interval (DRFI) and 5-year breast cancer–free interval (BCFI) when treated with exemestane+OFS vs. tamoxifen+OFS/ (21, 22). It should be remarked that STEPP is an exploratory tool (28). It means that its application is not limited to clinical trials but rather to determining specific cut-points in the range of values of the covariate of interest (29). Thus, STEPP methodology is an alternative approach to identify interactions between treatment and covariates, define risk subgroups based on a continuous covariate (e.g., risk score/composite risk value), and evaluate the treatment effect (28).
In the discovery set for Model N°01, three significant risk subgroups for distant recurrence were identified: Low (score: 0 – 31), intermediate (score: 32 – 64), and high risk (score: 65 – 100). Regarding Model N°02, three significant risk subgroups were also presented: Low (score: 0 – 26), intermediate (score: 27 – 55), and high risk (score: 56 – 100). Subsequently, the classification of these subgroups was corroborated in the validation set for each model. Our discovery and validation sets showed that the effect in 10-year DRFS of adjuvant chemotherapy initiation >30 days compared to ≤30 days depending on the risk score. According to the TTC, patients classified as low or intermediate risk appeared to have the same effect in 10-year DRFS.In contrast, patients classified as high risk and who start chemotherapy >30 days appear to have a more significant decrease in 10-year DRFS compared to those who began earlier.
In previous studies, no relationship had been found between TTC and worse outcomes when all types of breast cancer were included in the cohort (30, 31). A Kaplan-Meier analysis showed no patterns in delaying the start of chemotherapy for more than four weeks, which indicates no significantly increased hazard ratio in the overall survival (32). Other studies supported that chemotherapy more than 12 weeks from surgery remained significantly associated with inferior survival (33, 34). Meanwhile, another one concluded that a delay of 120 days from diagnosis to chemotherapy was associated with worse outcomes (HR=1.29; 95%CI:1.22–1.37; P<0.001) (35). As has been described, there is no explicit agreement on the ideal time range for TTC so that patients with breast cancer have better outcomes.
However, studies with TNBC patients suggest that 30 days is a meaningful indicator of the quality of cancer care. A recent study reported that adjuvant chemotherapy initiation after 30 days was shown to be associated with a decrease in 10-overall survival in TNBC patients (33). An evaluation of 6827 patients, including TNBC patients and those with HER2+ treated with trastuzumab, showed that patients who started chemotherapy 61 days after surgery had worse survival compared to those who started treatment in the first 30 days after surgery (25). In this context, it appears necessary to make efforts so TNBC patients can start their adjuvant therapies as soon as possible.
Although there has been increased awareness and efforts for health education and promotion in the Latin American region, access to specialized cancer centers and coverage of drug health insurance is limited (36, 37),. This situation hinders the first contact with the health service and the initiation of treatment, which is why the process takes about seven months in countries such as Brazil and Mexico (38). Likewise, a study of Colombian patients associated a higher socioeconomic and educational level with a decreased time to diagnosis and treatment (39).
Regarding adjuvant treatment, the proportion of locally advanced breast cancer (LABC) patients that initiate adjuvant chemotherapy after eight weeks is higher in public hospitals in countries of Latin America such as Bolivia (52.0%), Peru (40.0%), Colombia (25.0%) and Mexico (12.5%), compared to private hospitals in Chile (20.0%) and Peru (20.0%) (40). Furthermore, South America was the region with the most extended approval period for the Adjuvant Treatment Optimization Trial of Lapatinib and Trastuzumab (on average 236 days), while Europe and North America were the fastest (26 days) (41).
The COVID-19 pandemic has highlighted structural problems and inequities in the healthcare system. A multicenter descriptive study showed that, during the pandemic, 51.6% of Peruvian cancer patients experienced a delay in their therapy, 42.5% had their treatment rescheduled, and 30.6% were unable to see a specialist after their diagnosis (42). In Brazil, the trend appears to be similar: 40% of patients reported a delay in cancer treatment or changes in treatment strategy (43–45).
Due to previously mentioned barriers, only 27.5% of Peruvian patients with TNBC had a TTC ≤ 30 days (20). Proper segmentation of patients with TNBC will allow the risk of patients to be evaluated and a treatment strategy that fits their condition. The current study allows physicians to estimate individual risk based on age, pT, and pN to suggest who should urgently start adjuvant chemotherapy.
In conclusion, we developed two predictive models based on age, pT, and pN. Model N°02 was the best one to classify TNBC patients’ candidates for adjuvant chemotherapy into three subgroups: Low (score: 0 – 26), intermediate (score: 27 – 55), and high risk (score: 56 – 100). High-risk patients with TNBC who started their treatment >30 days experienced a decrease from 75.0% to 21.6% in 10-year DRFS, compared to those who started<30 days. This trend was confirmed in the validation cohort (58.8% to 36.1%). Thus, we suggest using our model to bring additional efforts to high-risk patients with TNBC to avoid increasing their relapse probabilities.
The original contributions presented in the study are included in the article/Supplementary Material. Further inquiries can be directed to the corresponding author.
The study was approved by the Ethics Review Board of the Instituto Nacional de Enfermedades Neoplasicas (INEN 22-03) and conducted in compliance with all relevant ethical guidelines.
Conception and design: HG, YF, JP, ZM. Administrative support: ZM, YF. Collection and assembly of data: ZM. Data analysis and interpretation: ZM, YF, JP, HG. Manuscript writing: All authors. Final approval of manuscript: All authors. Accountable for all aspects of the work: All authors. All authors contributed to the article and approved the submitted version.
The research was auto-financially supported by authors.
The authors thank the patients, caregivers, and medical staff involved in the treatment of these patients from the Instituto Nacional de Enfermedades Neoplásicas.
The authors declare that the research was conducted in the absence of any commercial or financial relationships that could be construed as a potential conflict of interest.
All claims expressed in this article are solely those of the authors and do not necessarily represent those of their affiliated organizations, or those of the publisher, the editors and the reviewers. Any product that may be evaluated in this article, or claim that may be made by its manufacturer, is not guaranteed or endorsed by the publisher.
The Supplementary Material for this article can be found online at: https://www.frontiersin.org/articles/10.3389/fonc.2023.1193927/full#supplementary-material
1. Vallejos C, Gómez H, Cruz WR, Pinto JA, Dyer R, Velarde R, et al. Breast cancer classification according to immunohistochemistry markers: subtypes and association with clinicopathologic variables in a Peruvian hospital database. Clin Breast Cancer (2010) 10(4):294–300. doi: 10.3816/cbc.2010.n.038
2. Zevallos A, Bravo L, Bretel D, Paez KJ, Infante U, Cardenas NK, et al. The hispanic landscape of triple negative breast cancer. Crit Rev Oncol Hematol (2020) 155:103094. doi: 10.1016/J.CRITREVONC.2020.103094
3. Lehmann BD, Pietenpol JA, Tan AR. Triple-negative breast cancer: molecular subtypes and new targets for therapy. Am Soc Clin Oncol Educ Book (2015) 35:e31–9. doi: 10.14694/edbook_am.2015.35.e31
4. Nwagu GC, Bhattarai S, Swahn M, Ahmed S, Aneja R. Prevalence and mortality of triple-negative breast cancer in west Africa: biologic and sociocultural factors. JCO Glob Oncol (2021)(7), 1129–40. doi: 10.1200/go.21.00082
5. Almansour NM. Triple-negative breast cancer: A brief review about epidemiology, risk factors, signaling pathways, treatment and role of artificial intelligence. Front Mol Biosci (2022) 9:836417/BIBTEX. doi: 10.3389/FMOLB.2022.836417/BIBTEX
6. Chavez KJ, Garimella SV, Lipkowitz S. Triple negative breast cancer cell lines: one tool in the search for better treatment of triple negative breast cancer. Breast Dis (2011) 32(1–2):35. doi: 10.3233/BD-2010-0307
7. Moore-Smith L, Forero-Torres A, Stringer-Reasor E. Future developments in neoadjuvant therapy for triple-negative breast cancer. Surg Clin North Am (2018) 98(4):773–85. doi: 10.1016/J.SUC.2018.04.004
8. Carey LA, Perou CM, Livasy C, Dressler LG, Cowan DP, Conway K, et al. Race, breast cancer subtypes, and survival in the Carolina Breast Cancer Study. JAMA (2006) 295(21):2492–502. doi: 10.1001/jama.295.21.2492
9. AL Amaral IVSK. Triple negative breast cancer in Brazilian women without metastasis to axillary lymph nodes: ten-year survival and prognostic factors. Br J Med Med Res (2013) 3(4):880–96. doi: 10.9734/bjmmr/2013/2544
10. Pinto JA, Araujo JM, Cardenas NK, Morante Z, Doimi FD, Vidaurre T, et al. A prognostic signature based on three-genes expression in triple-negative breast tumours with residual disease. NPJ Genomic Med (2016) 1(1):1–7. doi: 10.1038/npjgenmed.2015.15
11. Whitesell M, Al-Najar S, Bowser G, Brown MA. Cultural and environmental factors of triple-negative breast carcinomas. Cancer Res J (2014) 2(2):18. doi: 10.11648/J.CRJ.20140202.12
12. Wang Z, Dong Y, Lu Z, Chen Z. Socioeconomic status variables contribute to the disparities in female triple negative breast cancer outcome in theUnited States, 2011-2015: A population study based on NCI Surveillance, Epidemiology and End Results (SEER) database. J Clin Oncol (2021) 39(15_suppl):6521–1. doi: 10.1200/JCO.2021.39.15_suppl.6521
13. Dawson SJ, Provenzano E, Caldas C. Triple negative breast cancers: clinical and prognostic implications. Eur J Cancer (2009) 45(Suppl 1):27–40. doi: 10.1016/S0959-8049(09)70013-9
14. Dent R, Trudeau M, Pritchard KI, Hanna W, Kahn HK, Sawka C, et al. Triple-negative breast cancer: clinical features and patterns of recurrence. Clin Cancer Res (2007) 13(15):4429–34. doi: 10.1158/1078-0432.ccr-06-3045
15. Waks AG, Winer EP. Breast cancer treatment: A review. JAMA (2019) 321(3):288–300. doi: 10.1001/JAMA.2018.19323
16. Albain K, Davies C, Godwin J, Gray R, Pan H, Clarke M, et al. Comparisons between different polychemotherapy regimens for early breast cancer: meta-analyses of long-term outcome among 100,000 women in 123 randomised trials. Lancet (2012) 379(9814):432–44. doi: 10.1016/S0140-6736(11)61625-5
17. Curigliano G, Burstein HJ, Winer EP, Gnant M, Dubsky P, Loibl S, et al. De-escalating and escalating treatments for early-stage breast cancer: the St. Gallen International Expert Consensus Conference on the Primary Therapy of Early Breast Cancer 2017. Ann Oncol (2017) 28(8):1700–12. doi: 10.1093/ANNONC/MDX308
18. Cai L, Tong Y, Zhu X, Shen K, Zhu J, Chen X. Prolonged time to adjuvant chemotherapy initiation was associated with worse disease outcome in triple negative breast cancer patients. Sci Rep (2020) 10(1):1–11. doi: 10.1038/s41598-020-64005-4
19. Lazar AA, Cole BF, Bonetti M, Gelber RD. Evaluation of treatment-effect heterogeneity using biomarkers measured on a continuous scale: subpopulation treatment effect pattern plot. J Clin Oncol (2010) 28(29):4539–44. doi: 10.1200/JCO.2009.27.9182
20. Morante Z, Ruiz R, Araujo JM, Pinto JA, Cruz-Ku G, Urrunaga-Pastor D, et al. Impact of the delayed initiation of adjuvant chemotherapy in the outcome of triple negative breast cancer. Clin Breast Cancer (2021) 21(3):239–246.e4. doi: 10.1016/J.CLBC.2020.09.008
21. Pagani O, Francis PA, Fleming GF, Walley BA, Viale G, Colleoni M, et al. Absolute improvements in freedom from distant recurrence to tailor adjuvant endocrine therapies for premenopausal women: Results from TExT and Soft. J Clin Oncol (2020) 38(12):1293–303. doi: 10.1200/JCO.18.01967
22. Regan MM, Francis PA, Pagani O, Fleming GF, Walley BA, Viale G, et al. Absolute benefit of adjuvant endocrine therapies for premenopausal women with hormone receptor-positive, human epidermal growth factor receptor 2-negative early breast cancer: TEXT and SOFT trials. J Clin Oncol (2016) 34(19):2221–30. doi: 10.1200/JCO.2015.64.3171
23. Qu H, Shi R, Yang B, Chen X, Song Y, He L, et al. HAND2-AS1, PRKAA2 and VLDLR predict the risk of peritoneal metastasis in gastric cancer of different Lauren types based on STEPP analysis. BIOCELL (2022) 46(3):721–35. doi: 10.32604/BIOCELL.2021.015867
24. R: Analyze survival data using Kaplan-Meier method. Available at: https://search.r-project.org/CRAN/refmans/stepp/html/analyze.KM.stepp.html (Accessed Oct. 04, 2023).
25. De Melo Gagliato D, Gonzalez-Angulo AM, Lei X, Theriault RL, Giordano SH, Valero V, et al. Clinical impact of delaying initiation of adjuvant chemotherapy in patients with breast cancer. J Clin Oncol (2014) 32(8):735–44. doi: 10.1200/JCO.2013.49.7693
26. Lazar AA, Bonetti M, Cole BF, Yip WK, Gelber RD. Identifying treatment effect heterogeneity in clinical trials using subpopulations of events: STEPP. Clin Trials (2016) 13(2):169–79. doi: 10.1177/1740774515609106
27. Wang XV, Cole B, Bonetti M, Gelber RD. Meta-STEPP: subpopulation treatment effect pattern plot for individual patient data meta-analysis. Stat Med (2016) 35(21):3704–16. doi: 10.1002/SIM.6958
28. Yip WK, Bonetti M, Cole BF, Barcella W, Wang XV, Lazar A, et al. Subpopulation Treatment Effect Pattern Plot (STEPP) analysis for continuous, binary and count outcomes. Clin Trials (2016) 13(4):382–90. doi: 10.1177/1740774516643297
29. Pogue-Geile KL, Kim C, Jeong JH, Tanaka N, Bandos H, Gavin PG, et al. Predicting degree of benefit from adjuvant trastuzumab in NSABP trial B-31. J Natl Cancer Inst (2013) 105(23):1782–8. doi: 10.1093/JNCI/DJT321
30. Brazda A, Estroff J, Euhus D, Leitch AM, Huth J, Andrews V, et al. Delays in time to treatment and survival impact in breast cancer. Ann Surg Oncol (2010) 17(Suppl 3). doi: 10.1245/S10434-010-1250-6
31. Jara Sánchez C, Ruiz A, Martín M, Antón A, Munárriz B, Plazaola A, et al. Influence of timing of initiation of adjuvant chemotherapy over survival in breast cancer: a negative outcome study by the Spanish Breast Cancer Research Group (GEICAM). Breast Cancer Res Treat (2007) 101(2):215–23. doi: 10.1007/S10549-006-9282-0
32. Cold S, Düring M, Ewertz M, Knoop A, Møller S. Does timing of adjuvant chemotherapy influence the prognosis after early breast cancer? Results of the Danish Breast Cancer Cooperative Group (DBCG). Br J Cancer (2005) 93(6):627. doi: 10.1038/SJ.BJC.6602734
33. Lohrisch C, Paltiel C, Gelmon K, Speers C, Taylor S, Barnett J, et al. Impact on survival of time from definitive surgery to initiation of adjuvant chemotherapy for early-stage breast cancer. J Clin Oncol (2006) 24(30):4888–94. doi: 10.1200/JCO.2005.01.6089
34. Hershman DL, Wang X, McBride R, Jacobson JS, Grann VR, Neugut AI. Delay of adjuvant chemotherapy initiation following breast cancer surgery among elderly women. Breast Cancer Res Treat (2006) 99(3):313–21. doi: 10.1007/S10549-006-9206-Z
35. Kupstas AR, Hoskin TL, Day CN, Habermann EB, Boughey JC. Effect of surgery type on time to adjuvant chemotherapy and impact of delay on breast cancer survival: A national cancer database analysis. Ann Surg Oncol 2019 26:10 (2019) 26(10):3240–9. doi: 10.1245/S10434-019-07566-7
36. Alarcon-Rozas AE, Villacres K, Cardenas R. Perceptions of breast and cervix cancer prevention in two populations of women in Peru: Impact of knowledge and access to health service. J Clin Oncol (2005) 23(16_suppl):6120–0. 0. doi: 10.1200/jco.2005.23.16_6120
37. Justo N, Wilking N, Jönsson B, Luciani S, Cazap E. A review of breast cancer care and outcomes in Latin America. Oncologist (2013) 18(3):248–56. doi: 10.1634/THEONCOLOGIST.2012-0373
38. The Economist Intelligence Unit, “Control del cáncer, acceso y desigualdad en América Latina: Una historia de luces y sombras . Available at: https://www.iccp-portal.org/system/files/resources/Cancer_control_access_and_inequality_in_Latin_America_SPANISH.pdf (Accessed Mar. 11, 2022).
39. Piñeros M, Sánchez R, Perry F, García OA, Ocampo R, Cendales R. Delay for diagnosis and treatment of breast cancer in Bogotá, Colombia. Salud Publica Mex (2011) 53(6):478–85.
40. Pinto JA, Pinillos L, Villarreal‐Garza C, Morante Z, Villarán MV, Mejía G, et al. Barriers in Latin America for the management of locally advanced breast cancer. Ecancermedicalscience (2019) 13. doi: 10.3332/ECANCER.2019.897
41. Metzger-Filho O, de Azambuja E, Bradbury I, Saini KS, Bines J, Simon SD, et al. Analysis of regional timelines to set up a global phase III clinical trial in breast cancer: the adjuvant lapatinib and/or trastuzumab treatment optimization experience. Oncologist (2013) 18(2):134–40. doi: 10.1634/THEONCOLOGIST.2012-0342
42. Valdiviezo N, Alcarraz C, Castro D, Salas R, Begazo-Mollo V, Galvez-Villanueva M, et al. Oncological care during first Peruvian national emergency COVID-19 pandemic: A multicentric descriptive study. Cancer Manag Res (2022) 14:1075–85. doi: 10.2147/CMAR.S350038
43. Araujo SEA, Leal A, Centrone AFY, Teich VD, Malheiro DT, Cypriano AS, et al. Impact of COVID-19 pandemic on care of oncological patients: experience of a cancer center in a Latin American pandemic epicenter. Einstein (Sao Paulo) (2020) 19:eAO6282. doi: 10.31744/EINSTEIN_JOURNAL/2021AO6282
44. Lin DD, Meghal T, Murthy PM, Mo L, D’Silva A, Huang Y, et al. Chemotherapy treatment modifications during the COVID-19 outbreak at a community cancer center in New York City. JCO Glob Oncol (2020) 6:1298–305. doi: 10.1200/go.20.00309
Keywords: breast cancer, subpopulation treatment effect pattern plot, triple negative breast cancer, adjuvant chemotherapy, prognostic factor analysis
Citation: Morante Z, Ferreyra Y, Pinto JA, Valdivieso N, Castañeda C, Vidaurre T, Valencia G, Rioja P, Fuentes H, Cotrina JM, Neciosup S and Gomez HL (2023) Subpopulation treatment effect pattern plot analysis: a prognostic model for distant recurrence-free survival to estimate delayed adjuvant chemotherapy initiation effect in triple-negative breast cancer. Front. Oncol. 13:1193927. doi: 10.3389/fonc.2023.1193927
Received: 26 March 2023; Accepted: 18 October 2023;
Published: 06 November 2023.
Edited by:
Louis W. C. Chow, Organization for Oncology and Translational Research (OOTR), Hong Kong SAR, ChinaReviewed by:
Toshima Z. Parris, University of Gothenburg, SwedenCopyright © 2023 Morante, Ferreyra, Pinto, Valdivieso, Castañeda, Vidaurre, Valencia, Rioja, Fuentes, Cotrina, Neciosup and Gomez. This is an open-access article distributed under the terms of the Creative Commons Attribution License (CC BY). The use, distribution or reproduction in other forums is permitted, provided the original author(s) and the copyright owner(s) are credited and that the original publication in this journal is cited, in accordance with accepted academic practice. No use, distribution or reproduction is permitted which does not comply with these terms.
*Correspondence: Henry L. Gomez, aGdvbWV6QGdlY29wZXJ1Lm9yZw==
Disclaimer: All claims expressed in this article are solely those of the authors and do not necessarily represent those of their affiliated organizations, or those of the publisher, the editors and the reviewers. Any product that may be evaluated in this article or claim that may be made by its manufacturer is not guaranteed or endorsed by the publisher.
Research integrity at Frontiers
Learn more about the work of our research integrity team to safeguard the quality of each article we publish.