- 1School of Biomedical Engineering, Air Force Medical University, Shaanxi, China
- 2Shaanxi Provincial Key Laboratory of Bioelectromagnetic Detection and Intelligent Perception, Shaanxi, China
Cancer growing in hollow organs has become a serious threat to human health. The accurate T-staging of hollow organ cancers is a major concern in the clinic. With the rapid development of medical imaging technologies, radiomics has become a reliable tool of T-staging. Due to similar growth characteristics of hollow organ cancers, radiomics studies of these cancers can be used as a common reference. In radiomics, feature-based and deep learning-based methods are two critical research focuses. Therefore, we review feature-based and deep learning-based T-staging methods in this paper. In conclusion, existing radiomics studies may underestimate the hollow organ wall during segmentation and the depth of invasion in staging. It is expected that this survey could provide promising directions for following research in this realm.
1 Introduction
Cancer occurrence in hollow organs, such as gastric cancer (GC), colorectal cancer (CRC), esophageal cancer (EC), cervical cancer (CC), and bladder cancer (BC), poses a significant threat to human health. Global cancer statistics in 2022 reveal that hollow organ cancers rank fifth and fourth among the top ten cancers in terms of incidence and mortality, respectively (1). Despite being inconspicuous and difficult to detect in their early stages, hollow organ cancers often advance to the late stages by the time symptoms manifest (2–6).
Early screening and diagnosis are crucial for reducing morbidity and mortality associated with these cancers due to their slow progression. For instance, the implementation of screening for CRC in the United States led to a reduction of over 40% in its incidence and mortality (7). Current screening and diagnostic methods include fecal-occult-blood screening (8), blood tests for tumor markers (9), optical endoscopy (10), and imaging tests (11). However, the low specificity of the former two methods renders their results only as reference points (12). Although optical endoscopy is the clinical gold standard for hollow organ cancer diagnosis, its invasive nature can result in organ damage, such as infections, perforations, and hemorrhages (13). Noninvasive imaging techniques, such as MRI, CT, and US, have shown significant potential for cancer screening and diagnosis (14).
For the diagnosis of hollow organ cancers, T-staging plays an integral role, significantly impacting treatment decisions and prognoses. For example, T2 or below-stage BC patients may undergo cystoscopic resection, while those with more advanced T-stages may require complete cystectomy (15). In the case of EC, the selection of radiotherapy and chemotherapy is determined by whether the tumor is beyond T2-stage (16). Similar considerations apply to other hollow organ cancers (17–19). Radiomics methods offer the ability to quantify tumors through the extraction of extensive features and evaluate tumor heterogeneity objectively (14), surpassing subjective interpretations by medical professionals. Consequently, imaging-based radiomics studies have gained attention from researchers.
Given their clinical significance, consolidating and mutually referencing individual studies on hollow organ cancers becomes necessary for accurate diagnosis. In this review, we systematically describe and compare feature-based and deep learning-based methods sequentially, based on their respective feature extraction techniques. By analyzing their strengths and limitations, we aim to provide a comprehensive overview of existing studies and propose corresponding insights and limitations. Ultimately, our review aims to guide downstream diagnosis of hollow organ cancers and inspire subsequent radiomics studies to address these limitations progressively, thus developing more effective solutions.
2 Review methodology
Peer-reviewed papers were collected primarily through online digital libraries such as PubMed, ScienceDirect, Springer and IEEE Xplore. In addition, the Google Scholar search engine was used to search for pertinent publications. Considering their high incidence and mortality, we used the five following hollow organ cancers as examples: GC, CRC, EC, CC, and BC. For each disease, we mainly introduce their feature-based and deep learning-based T-staging methods. Therefore, the search keywords used included ‘radiomics’, ‘hollow organ cancer’, ‘segmentation’, ‘T-staging’, ‘gastric cancer’, ‘colorectal cancer’, ‘esophageal cancer’, ‘cervical cancer’ and ‘bladder cancer’. Only papers that included medical images were considered. The papers that were collected for review were published between 2012 and 2023, since imaging-based radiomics started receiving attention in 2012.
During the literature review, the following items were considered:
● Objective of the paper (learning tasks);
● Visual input (the kind of medical images);
● Methods used (segmentation methods, feature extraction methods, classification methods);
● Results or findings (the results obtained).
3 The pipeline of radiomics for T-staging
The pipeline of radiomics for tumor staging is shown in Figure 1A (14, 20). In feature-based methods, segmentation is the most basic step. We introduce three different types of segmentation techniques: manual, semiautomatic, and automatic segmentation in this paper. For tumor staging, many radiomics studies are based on conventional handcrafted features, i.e., shape, texture, statistics, wavelets, etc. (15, 21) The features are then screened to reduce the risk of overfitting the classifier, after which the features are classified. This is the standard pipeline for feature-based radiomics. For deep learning-based algorithms, on the other hand, the original image is frequently used as input, and stage prediction is conducted directly through an end-to-end structure.
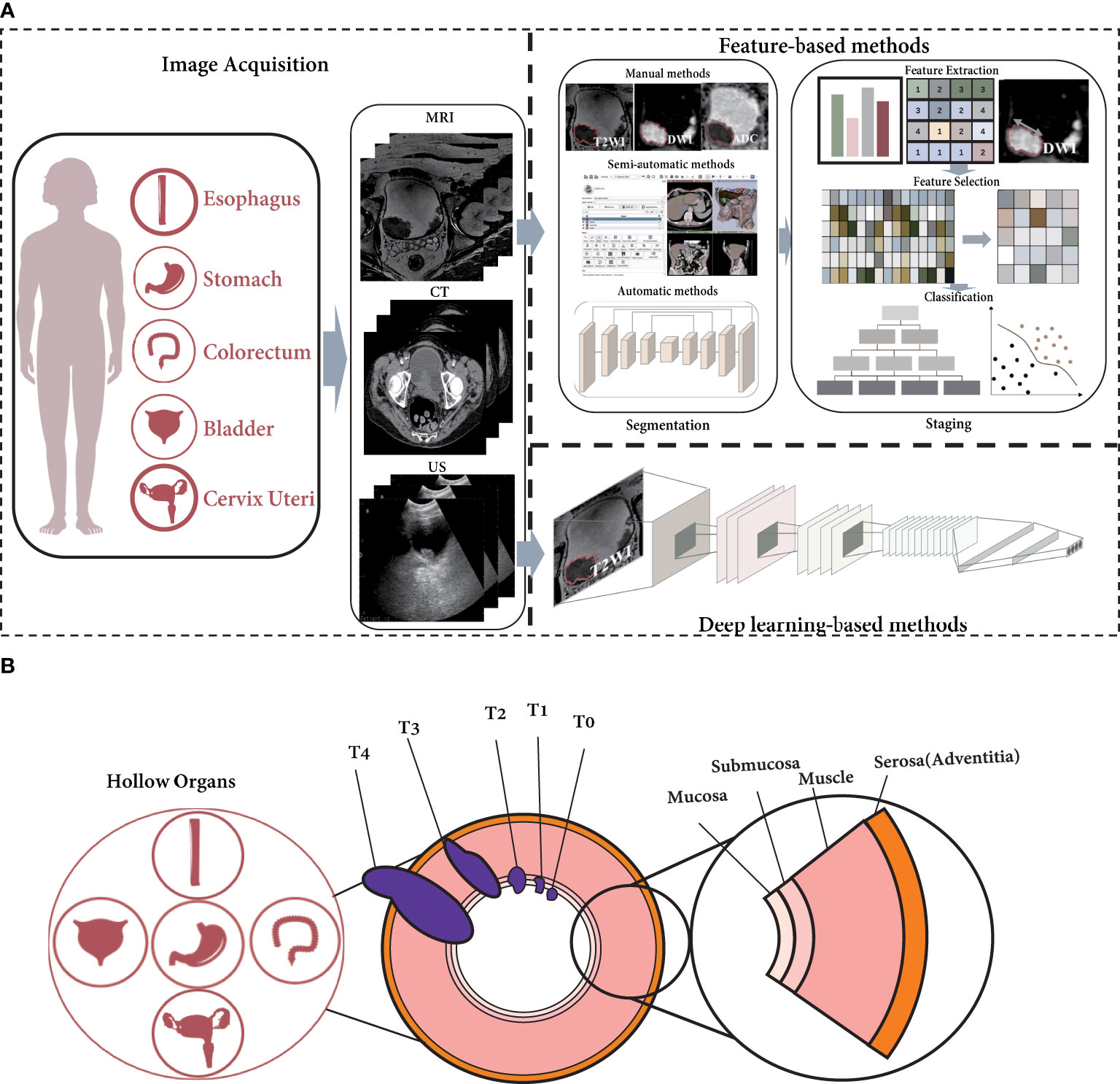
Figure 1 The current radiomics pipeline is not appropriate for hollow organ cancers with specific growth characteristics. (A) The pipeline of radiomics for T-staging. (B) The growth of cancer in hollow organs invade the organ walls.
However, hollow organ tumors adhere to the inner surface of the hollow organ when they occur, invasively grow toward the outer surface, and metastasize to the lymph nodes, as shown in Figure 1B. According to the eighth edition of the American Joint Committee on Cancer (AJCC) Staging Manual (22, 23), the depth of the tumor-invasive hollow organ wall is an important criterion for T-staging. (The T-staging of hollow organ cancers are listed in Table S1) In addition, the manual recommends the use of imaging modalities for staging diagnosis (23). As a result, T-staging is often not only related to the tumor territory seen in imaging; the depth of invasion is also an important factor.
For feature-based methods, the first step is usually to segment the tumor region (14). Because in many cancers, tumor size is a key factor, as in breast cancer and hepatobiliary cancer (23–25). However, unlike these solid organ tumors, in hollow organ tumors, the depth of tumor invasion is an important indicator for staging (23, 26–28). Therefore, general radiomics approaches for the T-staging of solid organ cancers are not fully applicable to that of hollow organ cancers. The relationship between tumor stage and depth of invasion determines that the segmentation of organ wall is also important. In radiomics studies of hollow organ cancers, the tumor and organ wall should be segmented first, and then the tumor stage can be estimated.
For deep learning-based methods, since there is no need to segment the region of interest (ROI), the segmentation of tumor and organ wall is not necessary (29, 30). However, end-to-end structures are often disfavored for their inexplicability. Therefore, for hollow organ cancers, how to integrate the depth of tumor invasion into tumor stage as an auxiliary task may be an issue that researchers need to be considered.
4 Segmentation for feature-based methods
4.1 Manual methods
Regarding the segmentation of the ROI, in most radiomics studies, it is still necessary to invite professional medical staff to manually outline it. For instance, in Ba-Ssalamah et al. (31), a radiologist was invited to manually delineate the ROI. In Ahn et al. (32), the radiologist also asked for help from another individual to confirm the manual result, which ensured intra-observer agreement. In addition, in Liu et al. (33), two radiologists jointly segmented the ROI manually. Similarly, in Wu et al. (34), multiple people participated in manual delineation, but they invited additional experts to confirm the individual delineation results to ensure inter-observer consistency. Therefore, regardless of whether manual delineation involves a single person or multiple people, the consistency guarantee of the delineation needs to be emphasized.
4.2 Semiautomatic methods
A specific software can be used to aid in the segmentation of a target regions or volumes. Usually, the software reduces labor use based on the key points of manual drawing and the differences around the pixels. For instance, 3D slicer (35), and PMOD 3.6 (36) can be used to segment the ROI or volume of interest (VOI). There are also several semiautomatic methods that can be used by artificially setting thresholds or initial shapes, such as those described in Dong et al. (37); Mu et al. (38); Xu et al. (39). In these works, we also determined whether the surrounding tissue was considered, since these segmentation methods were applied in hollow organ cancers. According to our statistics, the surrounding tissues were also segmented in Shen et al. (40); Mu et al. (38). However, the researchers did not segment the organ wall. With their methods, the depth of invasion could not be fully represented, which may result in inaccurate downstream T-staging.
4.3 Automatic methods
Many researchers expect to find a completely automatic method. This kind of method does not require manual intervention and learns the required segmentation area through a deep network. In these works, various deep networks are employed, such as two-stream 3D CNNs (41), and U-Net (42), and progressive dilated CNNs (43). In these works, several researchers segmented the ROI of each slice from a 2D perspective, as in Lin et al. (42); Dolz et al. (43). Others intended to segment the VOI from a 3D perspective (41, 44). With 2D methods, it is necessary to comprehensively analyze the multi-slice segmentation results to obtain the depth of invasion for T-staging. With 3D methods, it is more straightforward to estimate the depth of invasion by voxels.
From aforementioned statements, manual segmentation or semiautomatic segmentation using software is used as the gold standard, and the performance of these methods does not need to be considered; rather, quantitative metrics can be applied. For consistency, we suppose that it is necessary for manual and semiautomatic methods that require human involvement to ensure the accuracy of segmentation. Due to the lack of human intervention, fully automated methods do not require consistency checks. Since hollow organ tumor growth will appear to be infiltrative, we believe that the inclusion of the hollow wall is necessary to accurately estimate its invasion, as in the studies described in Liu et al. (45); Wang et al. (46). These items are summarized in Table S2.
5 Staging for feature-based methods
hollow organ cancers that have been staged via feature-based methods include EC (34, 47), GC (33, 48, 49), CRC (17, 21), CC (38), and BC (15, 46, 50). In these works, GC and BC were more commonly staged. The reason may be that the volume of the wall tissue in these two organs is relatively empty compared to that of the tumor, and the tumor and the wall tissue can be clearly distinguished by imaging. The esophagus and cervix are relatively narrow, and there are many folds in the colorectum, making the tumor and organ wall less obvious on imaging.
When staging a tumor, most studies try to distinguish tumors less advanced or more advanced than stage II, e.g., Liang et al. (17); Liu et al. (48); Wu et al. (34); Mu et al. (38); Wang et al. (49). The main reason is that the two types of treatment methods are different. Resection is regarded as the most common treatment option for patients with a tumor stage less advanced than T2, while chemotherapy is generally the main treatment option for patients with a tumor stage more advanced than T2 (51). For BC studies, researchers are more concerned about local excision surgery or total excision surgery, so more detailed T2 staging results is necessary (nonmuscle invasive vs. muscle invasive).
Concerning imaging studies, different studies involve various modalities. As a convenient and accessible imaging modality, CT is widely used, as in Liang et al. (17); Li et al. (21); Wu et al. (34); Wang et al. (49). Diffusion-weighted imaging (DWI) is another imaging modality that is commonly used (33, 48). Some studies have described the use of PET (47) or PET/CT (38) for T-staging. In addition, several studies have attempted to analyze tumor stage from multiple perspectives using multimodal MRI (15, 46, 50). Usually, the use of images is related to the data acquisition method and the type of disease. When predicting tumor stage in hollow organ, under certain conditions, it is better to use higher quality images and more modalities to show the invasion depth.
In these methods, radiomics features are the most utilized, e.g., Xu et al. (15); Liang et al. (17); Li et al. (21); Wu et al. (34); Ma et al. (47); Wang et al. (49); Zhang et al. (50); Wang et al. (46). Other hand-crafted features are introduced in Liu et al. (33, 48); Mu et al. (38). These features are usually selected to find the most effective features by the LASSO method (21, 34). Based on these features, several statistical methods are used to analyze the relationship between features and tumor stage (17, 33, 34, 47, 48. In other studies, various classifiers, such as support vector machines (SVMs) (15, 21, 38, 50), random forests (49), and logistic regression (46), have been chosen to classify these features. The basic workflow of these feature-based radiomics studies for T-staging of hollow organ cancers is almost the same. Because the data sources are inconsistent, it is difficult to judge which methods perform better. However, these methods use only general radiomics features to represent tumors. As stated in Sec. 3, they may ignore the feature representation of the organ wall, which leads to the inability to determine the invasion depth (23).
6 Deep learning-based methods
Recently, with the development of deep learning technologies, this realm has achieved better performance than traditional handcrafted features in various fields. Therefore, radiomics based on deep learning has also received increasing attention.
Studies of EC (29), CRC (52), and BC (53) have used deep learning-based methods for T-staging. This type studies are relatively lacking, since doctors usually use optical endoscopy to screen polyps in hollow organs. Sufficient data are produced in screening, so the existing deep learning work prefers optical images, e.g., Pacal et al. (54); Zhang et al. (55). However, for patients with hollow organ cancer, T-staging is also crucial and directly determines the treatment method. Therefore, research on deep learning-based methods using imaging for T-staging is still needed.
These deep learning works use imaging modalities such as PET (29), CT (52), and T2-weighted imaging (T2WI) (53). Deep learning usually requires a large amount of data for training. However, the data available in these studies are still insufficient (at most, more than 100 cases), so researchers have adopted several techniques that can reduce the risk of overfitting, such as using pretrained models (29), adding additional tasks (52), and using clinical rules (53) to constrain the training of deep networks. Therefore, currently, it may be necessary to construct a large-scale public database of hollow organ cancers for deep learning. This will greatly facilitate the application of deep learning in T-staging.
Several conventional deep networks, such as VGG (29), U-Net (52), and ResNet (53), are introduced in these studies. However, these end-to-end approaches may be too general. Since T-staging requires more attention to the mixed areas of the tumor and hollow organ wall, determining the invasion depth may be a better solution. For instance, in Zhang et al. (53), the authors proposed a two-branch architecture to predict the tumor stage of BC. In one branch, they took the tumor growth volume inside the bladder as a clinical rule to constrain stage prediction. In fact, their work considered the depth of tumor invasion into the bladder wall to some extent. Therefore, adding an additional task of consideration the invasion depth to the current end-to-end network may be more targeted.
In general, deep learning radiomics work is still relatively lacking, mainly because staging is not like segmentation, where multiple slices of images from each case can be used as training samples. However, it can be concluded from studies of other problems in tumors [e.g., Gao et al. (56); Bhatla et al. (57)] that deep learning may be a better tool for T-staging since features learned by deep networks are more representative than handcrafted features. Therefore, considering deep learning methods is a better choice when there is sufficient data. In addition, existing methods [e.g., Liang et al. (17); Mu et al. (38); Zhang et al. (50); Wang et al. (46)] mostly predict tumor stage based on tumor region and fail to consider the key factor in the definition of T-staging for hollow organ cancer, i.e., the depth of tumor invasion. Targeted feature extraction of this factor could more accurately represent tumor stage.
7 Discussion
Hollow organ tumors have garnered considerable attention in research, and this paper focuses on reviewing feature-based and deep learning-based T-staging studies across five representative hollow organ cancers. To provide clarity, we have divided our discussion into separate sections for feature-based radiomics and deep learning-based methods. While these studies have made remarkable progress in realizing automatic T-staging for hollow organ cancers, several limitations persist.
Regarding feature-based radiomics, three segmentation methods have been commonly employed, with manual segmentation being the gold standard but costly and time-consuming. Although semiautomatic methods offer some relief, ensuring their consistency remains a challenge. Furthermore, these methods face difficulty in overall characterization of the hollow organ tumor or wall, as they primarily rely on pixel gradient information. Fully automatic methods show promise in addressing these concerns, but their accuracy still requires improvement due to limited medical image data availability. Additionally, we emphasize the importance of considering hollow organ wall segmentation, as wall tissue invasion serves as a critical reference for downstream T-staging. Unfortunately, most existing research does not prioritize this aspect.
In the context of feature-based T-staging, the current radiomics pipeline favors solid organs over hollow organs, as it disregards the organ wall’s role. The depth of tumor invasion holds significant discriminative information for T-staging in hollow organ cancers, but few studies have quantified this parameter. We propose that subsequent studies should prioritize quantifying tumor invasion depth to explain algorithmic decision-making to physicians. As depicted in Figure 2, tumor invasion depth is determined by the ratio of invasion distance to wall thickness: InvasionDepth = InvasionDistance/WallThickness. Hence, segmenting both the tumor and organ wall is crucial for radiomics approaches in hollow organ cancer T-staging.
Deep learning-based T-staging requires the availability of more public data for improved performance. Moreover, end-to-end approaches may overlook the significance of segmenting the ROI or VOI. To incorporate the advantages of feature-based approaches, it may be more effective to include an additional segmentation task for tumor and organ wall segmentation in deep learning-based methods. By calculating the depth of invasion based on these segmentation results, a more refined T-staging method could be achieved.
8 Conclusions
Hollow organ cancers have always been at the forefront of cancer prevalence and mortality due to their special structures. Radiomics, a noninvasive approach, has become the most effective and convenient way to diagnose these tumors. This paper reviews T-staging related research on two kinds of radiomics methods: feature-based and deep learning-based. To segment hollow organ tumors, the feature-based methods discussed in this review include manual, semiautomatic, and automatic segmentation. In addition, we present the findings of feature-based and deep learning-based T-staging studies for various hollow organ cancers. While acknowledging the progress made by existing studies, it is important to recognize the limitations of feature-based and deep learning-based methods. In future research, it is essential to develop a specialized framework for radiomics in hollow organ cancers, differentiating them from solid organ cancers. This framework should consider the unique growth characteristics of hollow organ tumors. Regardless of the methodology employed, whether feature-based or deep learning-based, quantifying the depth of tumor invasion into the organ wall emerges as a crucial indicator for T-staging.
Author contributions
DH drafted this manuscript. XX helped perform the analysis with constructive discussions. XZ helped revise this manuscript. PD and YF helped to collect relevant literature. HL contributed significantly to manuscript preparation. YL contributed to the main idea of this review. All authors contributed to the article and approved the submitted version.
Funding
This work was supported by the Natural Science Basic Research Program of Shaanxi Province (No. 2023-JC-QN-0704), National Natural Science Foundation of China under grant (No. 81871424, No. 81901698), the Natural Science Foundation of Shaanxi Province (No. 2020JZ-28), and Key Research and development plan of Shaanxi Province (No. 2021SF-192).
Conflict of interest
The authors declare that the research was conducted in the absence of any commercial or financial relationships that could be construed as a potential conflict of interest.
Publisher’s note
All claims expressed in this article are solely those of the authors and do not necessarily represent those of their affiliated organizations, or those of the publisher, the editors and the reviewers. Any product that may be evaluated in this article, or claim that may be made by its manufacturer, is not guaranteed or endorsed by the publisher.
Supplementary material
The Supplementary Material for this article can be found online at: https://www.frontiersin.org/articles/10.3389/fonc.2023.1191519/full#supplementary-material
Abbreviations
GC, Gastric Cancer; CRC, Colorectal Cancer; EC, Esophageal Cancer; CC, Cervical Cancer; BC, Bladder Cancer; DL, Deep Learning; CNN, Convolutional Neural Network; LASSO, Least Absolute Shrinkage and Selection Operator; SVM, Support Vector Machine; ROI, Region of Interest; VOI, Volume of Interest; GLCM, Gray-Level Co-occurrence Matrix; ADC, Apparent Diffusion Coefficient.
References
1. Siegel RL, Miller KD, Fuchs HE, Jemal A. Cancer statistic. CA: Cancer J Clin (2022) 72:7–33. doi: 10.3322/caac.21708
2. Sanli O, Dobruch J, Knowles MA, Burger M, Alemozaffar M, Nielsen ME, et al. Bladder cancer. Nat Rev Dis Primers (2017) 3:1–19. doi: 10.1038/nrdp.2017.22
3. Song Z, Wu Y, Yang J, Yang D, Fang X. Progress in the treatment of advanced gastric cancer. Tumor Biol (2017) 39:1010428317714626. doi: 10.1177/1010428317714626
4. Brown K, Solomon M. Progress and future direction in the management of advanced colorectal cancer. J Br Surg (2018) 105:615–7. doi: 10.1002/bjs.10759
5. Oyervides-Munoz MA, Perez-Maya AA, Rodrıguez-Gutierrez HF, Gomez-Macias GS, FajardoRamırez OR, Trevino V, et al. Understanding the hpv integration and its progression to cervical cancer. Infect Genet Evol (2018) 61:134–44. doi: 10.1016/j.meegid.2018.03.003
6. Sachdeva UM, Shimonosono M, Flashner S, Cruz-Acuna R, Gabre JT, Nakagawa H. Understanding the cellular origin and progression of esophageal cancer using esophageal organoids. Cancer Lett (2021) 509:39–52. doi: 10.1016/j.canlet.2021.03.031
7. Shaukat A, Mongin SJ, Geisser MS, Lederle FA, Bond JH, Mandel JS, et al. Long-term mortality after screening for colorectal cancer. New Engl J Med (2013) 369:1106–14. doi: 10.1056/NEJMoa1300720
8. Hardcastle JD, Chamberlain JO, Robinson MH, Moss SM, Amar SS, Balfour TW, et al. Randomised controlled trial of faecal-occult-blood screening for colorectal cancer. Lancet (1996) 348:1472–7. doi: 10.1016/S0140-6736(96)03386-7
9. Werner S, Krause F, Rolny V, Strobl M, Morgenstern D, Datz C, et al. Evaluation of a 5-marker blood test for colorectal cancer early detection in a colorectal cancer screening settinga 5-marker blood test for crc early detection. Clin Cancer Res (2016) 22:1725–33. doi: 10.1158/1078-0432.CCR-15-1268
10. Hirata M, Tanaka S, Oka S, Kaneko I, Yoshida S, Yoshihara M, et al. Magnifying endoscopy with narrow band imaging for diagnosis of colorectal tumors. Gastrointest Endosc (2007) 65:988–95. doi: 10.1016/j.gie.2006.07.046
11. Badic B, Desseroit MC, Hatt M, Visvikis D. Potential complementary value of noncontrast and contrast enhanced ct radiomics in colorectal cancers. Acad Radiol (2019) 26:469–79. doi: 10.1016/j.acra.2018.06.004
12. Tamaki T, Yoshimuta J, Kawakami M, Raytchev B, Kaneda K, Yoshida S, et al. Computeraided colorectal tumor classification in nbi endoscopy using local features. Med Image Anal (2013) 17:78–100. doi: 10.1016/j.media.2012.08.003
13. Robb RA. Virtual endoscopy: development and evaluation using the visible human datasets. Comput Med Imaging Graphics (2000) 24:133–51. doi: 10.1016/S0895-6111(00)00014-8
14. Gillies RJ, Kinahan PE, Hricak H. Radiomics: Images are more than pictures, they are data. Radiology (2016) 278:563–77. doi: 10.1148/radiol.2015151169
15. Xu X, Zhang X, Tian Q, Wang H, Cui L-B, Li S, et al. Quantitative identification of nonmuscle-invasive and muscle-invasive bladder carcinomas: a multiparametric mri radiomics analysis. J Magn Reson. Imaging (2019) 49:1489–98. doi: 10.1002/jmri.26327
16. Napier KJ, Scheerer M, Misra S. Esophageal cancer: A review of epidemiology, pathogenesis, staging workup and treatment modalities. World J Gastrointest Oncol (2014) 6:112. doi: 10.4251/wjgo.v6.i5.112
17. Liang C, Huang Y, He L, Chen X, Ma Z, Dong D, et al. The development and validation of a ct-based radiomics signature for the preoperative discrimination of stage i-ii and stage iii-iv colorectal cancer. Oncotarget (2016) 7:31401. doi: 10.18632/oncotarget.8919
18. Wang Y, Jin Z-Y. Radiomics approaches in gastric cancer: a frontier in clinical decision making. Chin Med J (2019) 132:1983. doi: 10.1097/CM9.0000000000000360
19. Ai Y, Zhu H, Xie C, Jin X. Radiomics in cervical cancer: Current applications and future potential. Crit Rev Oncol/Hematol (2020) 152:102985. doi: 10.1016/j.critrevonc.2020.102985
20. Lohmann P, Bousabarah K, Hoevels M, Treuer H. Radiomics in radiation oncology–basics, methods, and limitations. Strahlentherapie und Onkologie (2020) 196:848–55. doi: 10.1007/s00066-020-01663-3
21. Li Y, Eresen A, Lu Y, Yang J, Shangguan J, Velichko Y, et al. Radiomics signature for the preoperative assessment of stage in advanced colon cancer. Am J Cancer Res (2019) 9:1429.
22. Amin MB, Greene FL, Edge SB, Compton CC, Gershenwald JE, Brookland RK, et al. The eighth edition ajcc cancer staging manual: Continuing to build a bridge from a population-based to a more “personalized” approach to cancer staging. CA Cancer J Clin (2017) 67:93–9. doi: 10.3322/caac.21388
23. Amin MB, Cancer. AJC, Society. AC. AJCC cancer staging manual. eight edition. Amin MB, Edge SB, Gress DM, Meyer LR, editors. Chicago IL: American Joint Committee on Cancer, Springer (2017).
24. Gradishar WJ, Anderson BO, Abraham J, Aft R, Agnese D, Allison KH, et al. Breast cancer, version 3.2020, nccn clinical practice guidelines in oncology. J Natl Compr Canc Netw (2020) 18:452–78. doi: 10.6004/jnccn.2020.0016
25. Benson AB, D’Angelica MI, Abbott DE, Anaya DA, Anders R, Are C, et al. Hepatobiliary cancers, version 2.2021, nccn clinical practice guidelines in oncology. J Natl Compr Canc Netw (2021) 19:541–65. doi: 10.6004/jnccn.2021.0022
26. Benson AB, Venook AP, Al-Hawary MM, Cederquist L, Chen YJ, Ciombor KK, et al. Rectal cancer, version 2.2018, nccn clinical practice guidelines in oncology. J Natl Compr Canc Netw (2018) 16:874–901. doi: 10.6004/jnccn.2018.0061
27. Flaig TW, Spiess PE, Agarwal N, Bangs R, Boorjian SA, Buyyounouski MK, et al. Bladder cancer, version 3.2020, nccn clinical practice guidelines in oncology. J Natl Compr Canc Netw (2020) 18:329–54. doi: 10.6004/jnccn.2020.0011
28. Benson AB, Venook AP, Al-Hawary MM, Arain MA, Chen YJ, Ciombor KK, et al. Colon cancer, version 2.2021, nccn clinical practice guidelines in oncology. J Natl Compr Canc Netw (2021) 19:329–59. doi: 10.6004/jnccn.2021.0012
29. Ferreira J, Domingues I, Sousa O, Sampaio IL, Santos JA. (2020). Classification of oesophagic early-stage cancers: deep learning versus traditional learning approaches. in: 2020 IEEE 20th International Conference on Bioinformatics and Bioengineering (BIBE), pp. 746–51. IEEE.
30. Wu Q, Wang S, Zhang S, Wang M, Ding Y, Fang J, et al. Development of a deep learning model to identify lymph node metastasis on magnetic resonance imaging in patients with cervical cancer. JAMA Netw Open (2020) 3:e2011625–e2011625. doi: 10.1001/jamanetworkopen.2020.11625
31. Ba-Ssalamah A, Muin D, Schernthaner R, Kulinna-Cosentini C, Bastati N, Stift J, et al. Texture-based classification of different gastric tumors at contrast-enhanced ct. Eur J Radiol (2013) 82:e537–43. doi: 10.1016/j.ejrad.2013.06.024
32. Ahn SJ, Kim JH, Park SJ, Han JK. Prediction of the therapeutic response after folfox and folfiri treatment for patients with liver metastasis from colorectal cancer using computerized ct texture analysis. Eur J Radiol (2016) 85:1867–74. doi: 10.1016/j.ejrad.2016.08.014
33. Liu S, Zheng H, Zhang Y, Chen L, Guan W, Guan Y, et al. Whole-volume apparent diffusion coefficient-based entropy parameters for assessment of gastric cancer aggressiveness. J Magn Reson Imaging (2018) 47:168–75. doi: 10.1002/jmri.25752
34. Wu L, Wang C, Tan X, Cheng Z, Zhao K, Yan L, et al. Radiomics approach for preoperative identification of stages i- ii and iii- iv of esophageal cancer. Chin J Cancer Res (2018) 30:396. doi: 10.21147/j.issn.1000-9604.2018.04.02
35. Ma Z, Fang M, Huang Y, He L, Chen X, Liang C, et al. Ct-based radiomics signature for differentiating borrmann type iv gastric cancer from primary gastric lymphoma. Eur J Radiol (2017) 91:142–7. doi: 10.1016/j.ejrad.2017.04.007
36. Tsujikawa T, Rahman T, Yamamoto M, Yamada S, Tsuyoshi H, Kiyono Y, et al. 18 f-fdg pet radiomics approaches: comparing and clustering features in cervical cancer. Ann Nucl Med (2017) 31:678–85. doi: 10.1007/s12149-017-1199-7
37. Dong X, Xing L, Wu P, Fu Z, Wan H, Li D, et al. Three-dimensional positron emission tomography image texture analysis of esophageal squamous cell carcinoma: relationship between tumor 18f-fluorodeoxyglucose uptake heterogeneity, maximum standardized uptake value, and tumor stage. Nucl Med Commun (2013) 34:40–6. doi: 10.1097/MNM.0b013e32835ae50c
38. Mu W, Chen Z, Liang Y, Shen W, Yang F, Dai R, et al. Staging of cervical cancer based on tumor heterogeneity characterized by texture features on 18f-fdg pet images. Phys Med Biol (2015) 60:5123. doi: 10.1088/0031-9155/60/13/5123
39. Xu X-P, Zhang X, Liu Y, Tian Q, Zhang G-P, Yang Z-Y, et al. (2017). Simultaneous segmentation of multiple regions in 3d bladder mri by efficient convex optimization of coupled surfaces, in: International Conference on Image and Graphics, pp. 528–42. Springer.
40. Shen C, Liu Z, Wang Z, Guo J, Zhang H, Wang Y, et al. Building ct radiomics based nomogram for preoperative esophageal cancer patients lymph node metastasis prediction. Trans Oncol (2018) 11:815–24. doi: 10.1016/j.tranon.2018.04.005
41. Jin D, Guo D, Ho T-Y, Harrison AP, Xiao J, Tseng C-K, et al. (2019). Accurate esophageal gross tumor volume segmentation in pet/ct using two-stream chained 3d deep network fusion, in: International Conference on Medical Image Computing and Computer-Assisted Intervention, pp. 182–91. Springer.
42. Lin Y-C, Lin C-H, Lu H-Y, Chiang H-J, Wang H-K, Huang Y-T, et al. Deep learning for fully automated tumor segmentation and extraction of magnetic resonance radiomics features in cervical cancer. Eur Radiol (2020) 30:1297–305. doi: 10.1007/s00330-019-06467-3
43. Dolz J, Xu X, Rony J, Yuan J, Liu Y, Granger E, et al. Multiregion segmentation of bladder cancer structures in mri with progressive dilated convolutional networks. Med Phys (2018) 45:5482–93. doi: 10.1002/mp.13240
44. Rigaud B, Anderson BM, Zhiqian HY, Gobeli M, Cazoulat G, Sderberg J, et al. Automatic segmentation using deep learning to enable online dose optimization during adaptive radiation therapy of cervical cancer. Int J Radiat Oncol Biol Phys (2021) 109:1096–110. doi: 10.1016/j.ijrobp.2020.10.038
45. Liu Y, Zheng H, Xu X, Zhang X, Du P, Liang J, et al. The invasion depth measurement of bladder cancer using t2-weighted magnetic resonance imaging. Biomed Eng Online (2020) 19:1–13. doi: 10.1186/s12938-020-00834-8
46. Wang H, Xu X, Zhang X, Liu Y, Ouyang L, Du P, et al. Elaboration of a multisequence mri-based radiomics signature for the preoperative prediction of the muscle-invasive status of bladder cancer: a double-center study. Eur Radiol (2020) 30:4816–27. doi: 10.1007/s00330-020-06796-8
47. Ma C, Li D, Yin Y, Cao J. Comparison of characteristics of 18f-fluorodeoxyglucose and 18f-fluorothymidine pet during staging of esophageal squamous cell carcinoma. Nucl Med Commun (2015) 36:1181–6. doi: 10.1097/MNM.0000000000000378
48. Liu S, Zhang Y, Xia J, Chen L, Guan W, Guan Y, et al. Predicting the nodal status in gastric cancers: the role of apparent diffusion coefficient histogram characteristic analysis. Magn Reson Imaging (2017) 42:144–51. doi: 10.1016/j.mri.2017.07.013
49. Wang Y, Liu W, Yu Y, Liu J-J, Jiang L, Xue H-D, et al. Prediction of the depth of tumor invasion in gastric cancer: potential role of ct radiomics. Acad Radiol (2020) 27:1077–84. doi: 10.1016/j.acra.2019.10.020
50. Zhang X, Xu X, Tian Q, Li B, Wu Y, Yang Z, et al. Radiomics assessment of bladder cancer grade using texture features from diffusion-weighted imaging. J Magn Reson Imaging (2017) 46:1281–8. doi: 10.1002/jmri.25669
51. Miller KD, Nogueira L, Mariotto AB, Rowland JH, Yabroff KR, Alfano CM, et al. Cancer treatment and survivorship statistic. CA: Cancer J Clin (2019) 69:363–85. doi: 10.3322/caac.21565
52. Yang H, Guo S, He K, Yang J, Guo Y, Qiu Y, et al. (2021). Computed tomography for preoperative colorectal staging prediction based on deep multi-task learning: Colorectal staging prediction based on deep multi-task learning, in: The Fourth International Symposium on Image Computing and Digital Medicine (Shenyang, China: Association for Computing Machinery), pp. 95–8. doi: 10.1145/3451421.3451442
53. Zhang C, Yue X, Chen Y, Lv Y. (2020). Integrating diagnosis rules into deep neural networks for bladder cancer staging, in: Proceedings of the 29th ACM International Conference on Information & Knowledge Management (Virtual Event, Ireland: Association for Computing Machinery), pp. 2301–4. doi: 10.1145/3340531.3412122
54. Pacal I, Karaboga D, Basturk A, Akay B, Nalbantoglu U. A comprehensive review of deep learning in colon cancer. Comput Biol Med (2020) 126:104003. doi: 10.1016/j.compbiomed.2020.104003
55. Zhang Y-H, Guo L-J, Yuan X-L, Hu B. Artificial intelligence-assisted esophageal cancer management: Now and future. World J Gastroenterol (2020) 26:5256. doi: 10.3748/wjg.v26.i35.5256
56. Gao Y, Zhang Z-D, Li S, Guo Y-T, Wu Q-Y, Liu S-H, et al. Deep neural network-assisted computed tomography diagnosis of metastatic lymph nodes from gastric cancer. Chin Med J (2019) 132:2804. doi: 10.1097/CM9.0000000000000532
Keywords: radiomics, hollow organ cancer, segmentation, T-staging, feature-based methods, deep learning-based methods
Citation: Huang D, Xu X, Du P, Feng Y, Zhang X, Lu H and Liu Y (2023) Radiomics-based T-staging of hollow organ cancers. Front. Oncol. 13:1191519. doi: 10.3389/fonc.2023.1191519
Received: 23 May 2023; Accepted: 11 August 2023;
Published: 30 August 2023.
Edited by:
Rakesh Kapoor, Post Graduate Institute of Medical Education and Research (PGIMER), IndiaReviewed by:
Bilgin Kadri Aribas, Bülent Ecevit University, TürkiyeCopyright © 2023 Huang, Xu, Du, Feng, Zhang, Lu and Liu. This is an open-access article distributed under the terms of the Creative Commons Attribution License (CC BY). The use, distribution or reproduction in other forums is permitted, provided the original author(s) and the copyright owner(s) are credited and that the original publication in this journal is cited, in accordance with accepted academic practice. No use, distribution or reproduction is permitted which does not comply with these terms.
*Correspondence: Hongbing Lu, hblu@fmmu.edu.cn; Yang Liu, yliu@fmmu.edu.cn