- 1Department of Oncology, Xiangya Hospital, Central South University, Changsha, Hunan, China
- 2Department of Internal Medicine, Mbeya Zonal Referral Hospital and Mbeya College of Health and Allied Sciences, University of Dar-es-salaam, Mbeya, Tanzania
- 3Garissa Cancer Center, Garissa County Referral Hospital, Garissa, Kenya
- 4Department of Oncology, The First People's Hospital of Loudi, Loudi, Hunan, China
Background: The role of platelet–lymphocyte ratio (PLR) and neutrophil–lymphocyte ratio (NLR) as independent prognostic markers in different tumors is well established. However, there is a limited review of the potential of NLR and PLR as predictors of treatment outcomes from immune checkpoint inhibitors (ICIs).
Objective: To establish a correlation between NLR and PLR and the potential of clinical benefit from ICIs.
Methods: The literature search was performed for studies that reported the association between NLR, PLR, and treatment outcomes among cancer patients treated with ICIs. The outcomes of interest were objective response rate (ORR), disease control rate (DCR), and progressive disease (PD). ORR was the summation of patients who achieved complete response and partial response. DCR included patients who achieved stable disease. PD was the proportion of patients who progressed, relapsed, or discontinued the treatment. Statistical analysis was performed using the STATA 12.0 package. Heterogeneity was determined by the I2 value. Quality assessment was performed using the Newcastle–Ottawa Scale. Egger’s test was used to establish publication bias and sensitivity analysis.
Results: A total of 40 papers that met the inclusion criteria were included in the systematic review. However, only 17 studies were used in the meta-analysis to determine the correlation between NLR, PLR, and treatment response. We found that treatment with ICIs and monitoring of outcomes and adverse events using PLR and NLR parameters have been studied in different tumors. Our analysis showed that low NLR correlated with higher ORR (OR = 0.62 (95% CI 0.47–0.81, p = 0.001) and higher DCR (OR = 0.23, 95% CI 0.14–0.36, p < 0.001). Higher NLR predicted a higher probability of PD (OR = 3.12, 95% CI 1.44, 6.77, p = 0.004). Similarly, low PLR correlated with higher ORR (OR = 0.69, 95% CI 0.5, 0.95, p = 0.025). Generally, patients with low NLR and PLR were more likely to achieve clinical benefit and better response (p-value < 0.001). Meanwhile, patients with high ratios were more likely to progress (p-value < 0.005), although there was significant heterogeneity among studies. There was no significant publication bias observed.
Conclusion: The study showed that high NLR and PLR either at baseline or during treatment is associated with poorer treatment outcome. Therefore, these ratios can be utilized in clinical practice with other markers to determine treatment efficacy from immunotherapy.
1 Introduction
Chronic inflammation is one of the enabling characteristics in the acquisition of hallmarks of cancer, together with genomic instability (1). The inflammatory process is driven by key inflammatory cells, namely, lymphocytes, neutrophils, monocytes, and platelets (2). Interaction of these cells in the tumor microenvironment (TME) and the peripheral circulation not only facilitates the propagation and survival of cancer cells but also provides them with the ability to evade the immune system, induce angiogenesis, and metastasize to other sites (2).
Immunotherapy is one of the pillars of cancer treatment in combination with surgery, chemotherapy, radiotherapy, and the expanding targeted therapy. These drugs function by blocking immune checkpoints, which are programmed death-1 (PD-1) and its ligand (PDL-1), cytotoxic T-lymphocyte antigen 4 (CTLA-4), and lymphocyte-activation gene 3 (LAG-3), resulting in upregulation of T-cell activation, preventing tumor evasion and increasing CD8 T-cell response toward cancer cells (3).
Indication of immune checkpoint inhibitors (ICIs) is expanding rapidly from advanced disease settings to neo-adjuvant and adjuvant use in early disease (4–6) with the potential of complete treatment response and durable disease control in some patients (3). Currently, ICIs are indicated for multiple cancers with non-small cell lung cancer (NSCLC) and melanoma deriving the greatest benefit to gastrointestinal, genitourinary, and breast cancers and lymphomas just to mention a few (3).
The mechanism of action of immunotherapy depends on the inflammatory cells and tumor immunogenicity (3). Hence, in a state of lymphopenia (7), thrombocytosis (8, 9), and neutrophilia (10) either at baseline or during the course of treatment as is the case in most patients with advanced disease and poor performance status, it is less likely to achieve durable clinical response. In addition, tumors that can generate significant immune responses like melanoma and squamous cell carcinomas show dramatic responses in comparison to cold tumors like gliomas and pancreatic cancer (3).
The currently approved biomarkers to predict response to immunotherapy are PDL-1 levels, microsatellite instability status (MSI), and tumor mutation burden (TMB). However, these have been shown to be applicable in a small proportion of patients (2, 11). Although they have revolutionized the use of ICIs in cancer treatment, the fact that they are tissue-based makes them susceptible to tumor heterogeneity (12). In addition, they cannot distinguish between patients who will respond to therapy against those who will not (12).
Interaction between neutrophils, platelets, and lymphocytes reflects the balance between protumoral inflammation and anti-tumor activity (3). In some studies, the neutrophil–lymphocyte ratio (NLR) and platelet–lymphocyte ratio (PLR) was associated with a better response than PDL-1 levels (11, 13). Therefore, there is a need to develop a prognostic and predictive model that incorporates other potential biomarkers to be able to determine those who are more likely to benefit from treatment (14, 15).
A recent meta-analysis looked at the association of dynamic changes in NLR with survival outcomes and treatment response (16). The study concluded that lower baseline NLR and a downward trend of NLR during and post-treatment with immunotherapy were associated with longer survival and better tumor response (16). However, very few studies that were included reported on treatment response and disease control. Another meta-analysis on renal cell carcinoma (RCC) also showed high NLR correlated with worse survival outcomes (17).
Notably, a significant number of studies have focused on the role of NLR and PLR as prognostic factors, but very few have focused on treatment response with immunotherapy (18–21). Therefore, this study will focus on the role of NLR and PLR as predictive markers of response to immunotherapy.
2 Methods
2.1 Search strategy
The systematic review and meta-analysis were conducted in accordance with Preferred Reporting Items for Systematic Reviews and Meta-analyses (PRISMA) guidelines. A comprehensive literature search was conducted from PubMed, Embase, Web of Science, and Cochrane Library from 2015 to 2022. The search terms employed were “neutrophil-to-lymphocyte ratio” AND “immune checkpoint inhibitors” and “platelet-to-lymphocyte ratio” AND “immune checkpoint inhibitors” (Table S1).
The outcomes of interest were objective response rate (ORR), disease control rate (DCR), and progressive disease (PD) as defined by Response Evaluation Criteria in Solid Tumors (RECIST) 1.1. ORR was the summation of patients who achieved complete response and partial response. DCR included patients who achieved stable disease. PD was the proportion of patients who progressed, relapsed, or discontinued the treatment. The correlation was made according to cutoff values of NLR and PLR established at baseline and during the course of treatment as determined by the authors.
2.2 Inclusion and exclusion criteria
Studies that met the following criteria were included in the study:
□ studies published from 2015 to 2022;
□ studies that enrolled patients with solid tumors who received any of the ICIs;
□ studies reporting clinical response (ORR, DCR, and PD) and prognostic value of inflammatory markers and ICIs; and
□ prospective studies, retrospective studies, exploratory studies, and randomized controlled trials (RCTs).
2.2.1 Exclusion criteria
□ Studies that did not document or analyze the association or prognostic value of inflammatory markers and ICIs;
□ non-English studies;
□ abstracts, reviews, meta-analyses, case reports, editorials, letters to the editor, and commentaries; and
□ animal studies.
2.3 Data extraction
The following information was extracted:
□ name of the first author,
□ year of publication,
□ type of cancer,
□ number of patients,
□ type of study design,
□ inflammatory markers investigated, and
□ numerical data for NLR, PLR, ORR, DCR, and PD from frequency tables.
2.4 Quality assessment
The Newcastle–Ottawa Scale (NOS) was used to assess the quality of included studies. Any study with a minimum of two stars was considered suitable to be included in the review and meta-analysis. However, the most important criterion was the availability of quality extractable data from an individual study (Table S2).
2.5 Statistical analysis
Authoritative statistical software (Stata 12.0: StataCorporation) was used to perform the meta-analysis. The OR and 95% CI values were applied to estimate the prognostic value of NLR and PLR for patients treated with ICIs. Individual OR and 95% CI values were combined to an overall OR and 95% CI. An OR < 1 indicated a better treatment outcome. The Higgins I2 statistic was applied to detect the heterogeneity between studies; p ≤ 0.1 and I2 > 50% indicated a substantial heterogeneity between studies, and random-effects models were adopted. Egger’s test and visual inspection of a funnel plot were carried out to evaluate the possibility of publication bias. Egger’s test result was the primary indicator, and a symmetry funnel plot with a p-value ≥0.05 was considered an insignificant publication bias.
3 Results
3.1 Literature screening results
The literature search identified 1,062 studies from the database and registers. Out of those, 711 were removed as duplicates, 158 records were removed because they were not eligible, 80 reports could not be retrieved, and 73 reports either had missing information or were not related to the study. The final review and meta-analysis included 40 studies and 17 studies, respectively (Figure 1). The characteristics of studies, data extracted, and patient characteristics involved in the studies are represented in Tables 1 and 2. Important findings from the studies are summarized in Supplementary Table 3.
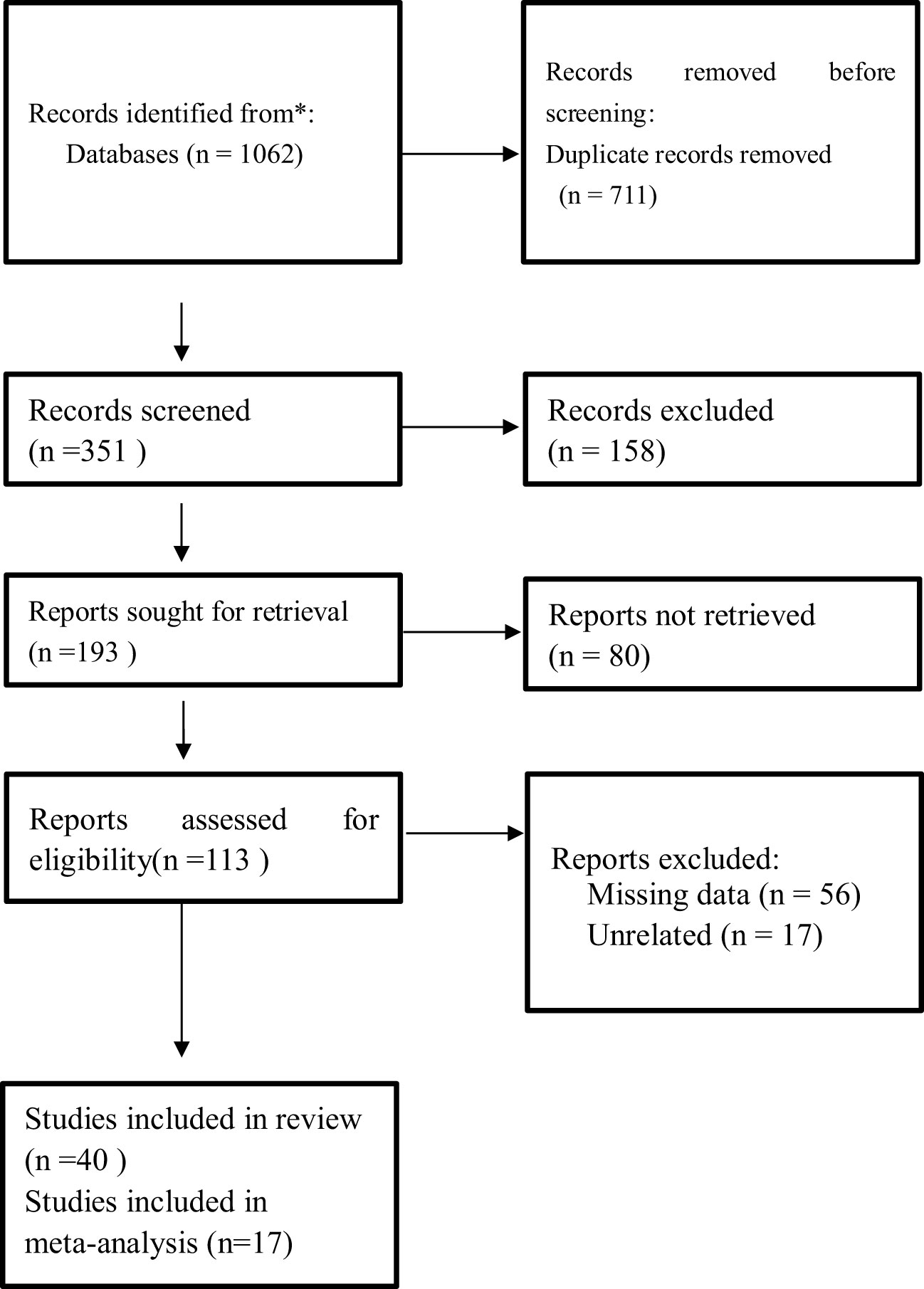
Figure 1 PRISMA flow diagram of study selection for inclusion in the systematic review and meta-analysis. PRISMA, Preferred Reporting Items for Systematic Reviews and Meta-analyses.
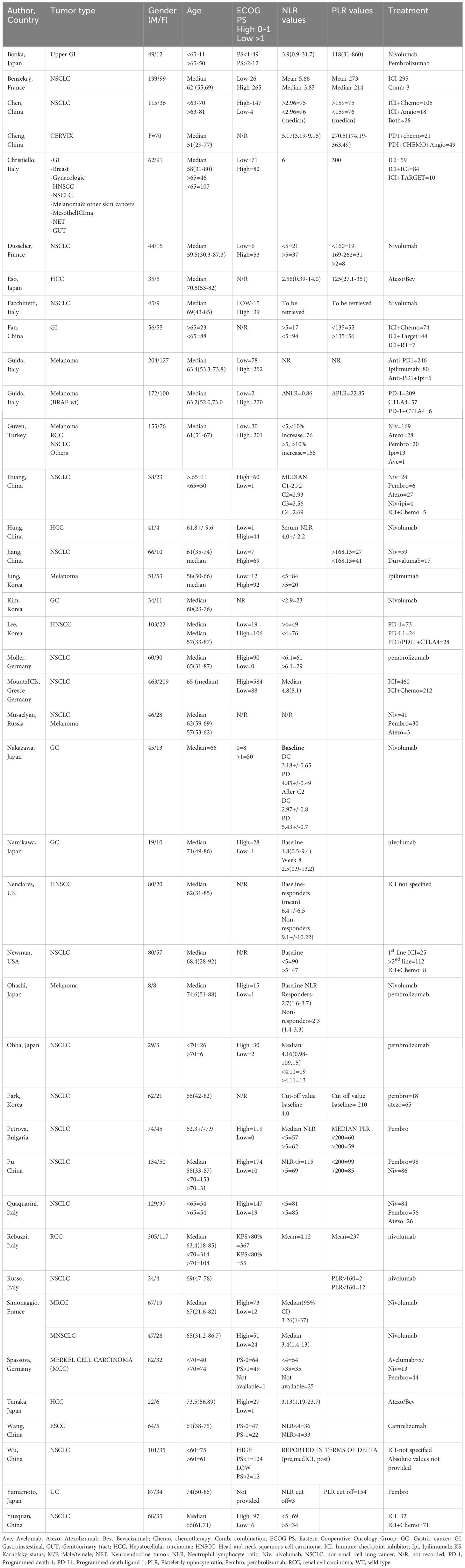
Table 2 Characteristics of patients involved in the studies that related NLR, PLR and treatment response.
3.2 Research characteristics
A total of 40 studies were included in this systematic review, but only 17 studies (13, 22–37) qualified for meta-analysis. Regionwise, almost half of the included studies came from Asian countries (Japan, China, and Korea). The sample size in the included 40 studies ranged from 16 patients to 672 patients. Almost all studies were retrospective in nature except for one study that used a prospective study design (23) and received moderately high scores in the Newcastle–Ottawa Scale quality assessment. A total of 36 studies looked at distinct cancer types, and three studies looked at two or more types of tumors. Out of the 36 studies that looked at specific cancer types, NSCLC was the most frequently studied tumor. Fifteen studies focused only on ICI as a single agent, while the remaining studies included patients who received immunotherapy in combination with other cancer treatment modalities. Apart from reporting NLR and PLR, other blood cell counts included derived neutrophil–lymphocyte ratio (dNLR), absolute lymphocyte count (ALC), absolute neutrophil count (ANC), absolute platelet count (APC), absolute eosinophil count (AEC), and leukocyte count and its differentials. Receiver operating characteristic (ROC) curves were used in nine studies (25, 33, 36, 38–43) to determine the optimal cutoff value for NLR and PLR.
3.2.1 Non-small cell lung cancer
Up to 50% (18 studies) of the included studies (10, 11, 13, 22–28, 38, 39, 44–49) reported the association of NLR and PLR in non-small cell lung cancer patients treated with ICIs. The sample size ranged from 45 to 672 patients. The average number of patients was 142 patients. In total, there were 2,563 patients. One study only reported the ORR, five studies reported on DCR, four studies reported on ORR and DCR, and seven studies reported DCR and PD. None of the included studies had all the three components. Two studies compared numerical percentages between patients who responded to treatment (DCR) and those who progressed (PD). One study reported the correlation of ratios with overall short-term efficacy.
The studies were further divided on the basis of the presence or absence of a significant relationship between the ratios and end points. The subdivision produced a total of 51 reports. Out of those, five reports were on ORR, 27 reports were on DCR, and 19 reports were on PD.
3.2.1.1 Objective response rate
Three out of five reports showed a statistically significant relationship between the ratios and ORR. In one of the studies, ORR was higher in PLR-low patients compared to PLR-high patients (46.15% vs. 8.3%, p < 0.0004). In another study, those who had a decrease in NLR 12 weeks post-treatment were more likely to derive clinical benefit than those with increasing NLR (OR = 3.304, 95% CI 1.560–7.001, p = 0.002).
3.2.1.2 Disease control rate
Out of 29 reports, 16 reports showed a significant correlation between NLR, PLR, and DCR, while nine studies reported a lack of relationship. Most of the reports with significant association also noted a higher DCR among patients with low ratios either at baseline or after a few cycles of treatment.
3.2.1.3 Progressive disease
Ten reports showed a statistically significant correlation between the ratios and PD. Generally, patients with higher NLR and PLR tend to progress earlier and have a higher rate of PD as compared to those with low ratios.
3.2.2 Gastrointestinal cancer
Six studies (29–32, 50, 51) (17 reports) reported the correlation between ratios and study end points. Out of five reports for ORR, only one study showed a significant relationship with NLR at baseline compared with other times (NLR L vs. H Baseline 36.1% vs. 9.1%, p = 0.018; at V1 34.4% vs. 15.6%, p = 0.083; variation (baseline-V1) < 20% vs. >20%; 31.6% vs. 22.2%, p = 0.430).
Among nine reports that looked at DCR, five of them showed a positive correlation, while four reports lacked a statistically significant relationship. Those with low PLR and NLR had higher DCR than those with high ratios (PLR L vs. H) = 36.7% vs. 9.7%, p = 0.012; NLR (L vs. H) = 33.3% vs. 12.9%, p = 0.058).
Likewise, patients with higher ratios had higher rates of progressive disease than those with low ratios. In a retrospective study of 58 patients (50), mean NLR was significantly higher in the PD group at both baseline and post-treatment (Pre-rx, 318 vs. 4.85, p = 0.045; Post-rx, 2.97 vs. 5.43, p = 0.025). In another study (51), NLR showed a statistically significant relationship at week 4 post-treatment compared to other times (p = 0.044).
3.2.3 Melanoma
A study by Ohashi et al. with a small sample size of 16 patients reported no significant relation between NLR and ORR (52).
Another retrospective study (53) that looked at DCR reported a significant correlation between NLR and DCR despite the NRAS mutation status (OR = 0.88, 95% CI 0.77–1.00, p = 0.005). Also, a change in NLR and PLR correlated with lower response (ΔNLR with OR = 2.779, p < 0.001, ΔPLR OR = 2.022, p < 0.009) (40).
3.2.4 Hepatocellular carcinoma
Out of the three studies (33, 41, 54) (10 reports), three reports on DCR and three reports on PD showed a significant relationship. Only one study reported a lack of correlation between the ratios and ORR.
3.2.5 Urothelial carcinoma (renal [RCC] and bladder [UC])
Rebuzzi et al. (34) reported mean values of NLR and PLR among patients who achieved clinical response and those with progressive disease at baseline and after four doses of treatment (longitudinal variation). The mean value of NLR and PLR at baseline and after four doses of treatment was lower as compared with the group with progressive disease (NLR, 3.18 vs. 4.12, p = 0.012; PLR, 184 vs. 237, p = 0.003).
In the study by Simonaggio et al. (55), the NLR-low group had greater DCR in any NLR decrease at week 6 as compared to the NLR-high group (81% vs. 40%, p = 0.0007).
Likewise, in the study by Yamamoto et al. (42), NLR had a statistically significant association with ORR (p = 0.016), while PLR had a marginal significance (p = 0.0536).
3.2.6 Head and neck cancers
A study by Lee et al. (56) showed that those patients with high NLR were associated with poor response (OR = 0.3, 95% CI 0.11–0.84, p = 0.022). Similar findings were reported by Nenclares et al. (43) where NLR was significantly lower in responders (DCR) compared to non-responders (p < 0.001).
3.2.7 Across solid tumors
In both pan-solid cancer retrospective studies (35, 57), those with low ratios had a greater response rate as compared to those with high ratios. In a study by Guven et al., those patients with high NLR and greater than 10% NLR increase had the lowest ORR.
3.2.8 Other tumors
One study on cervical cancer (36) reported a significant relationship between NLR and ORR [NLR (L vs. H) 78.26% vs. 53.19%, OR = 0.316, 95% CI 0.1–0.991, p = 0.048], while there was no relationship with PLR [PLR (L vs. H) 70% vs. 58%, OR = 0.592, 95% CI 0.195–1.794, p = 0.354]. However, despite the lack of significance, ORR was higher in patients with low ratios than those with high ratios.
A study on advanced Merkel cell carcinoma by Spassova et al. (37) noted that the NLR-low group had more patients with disease control (ORR) than the NLR-high group (49% vs. 37%), while there was no difference in the group with disease progression.
3.3 Meta-analysis
As described in the Methods, a meta-analysis was conducted under two subgroups NLR and PLR for the treatment efficacy end-point ORR, DCR, and PD. Each study that focused on these inflammatory markers was assessed independently. Forest plots were used to represent the pooled results (Figures 2A–F).
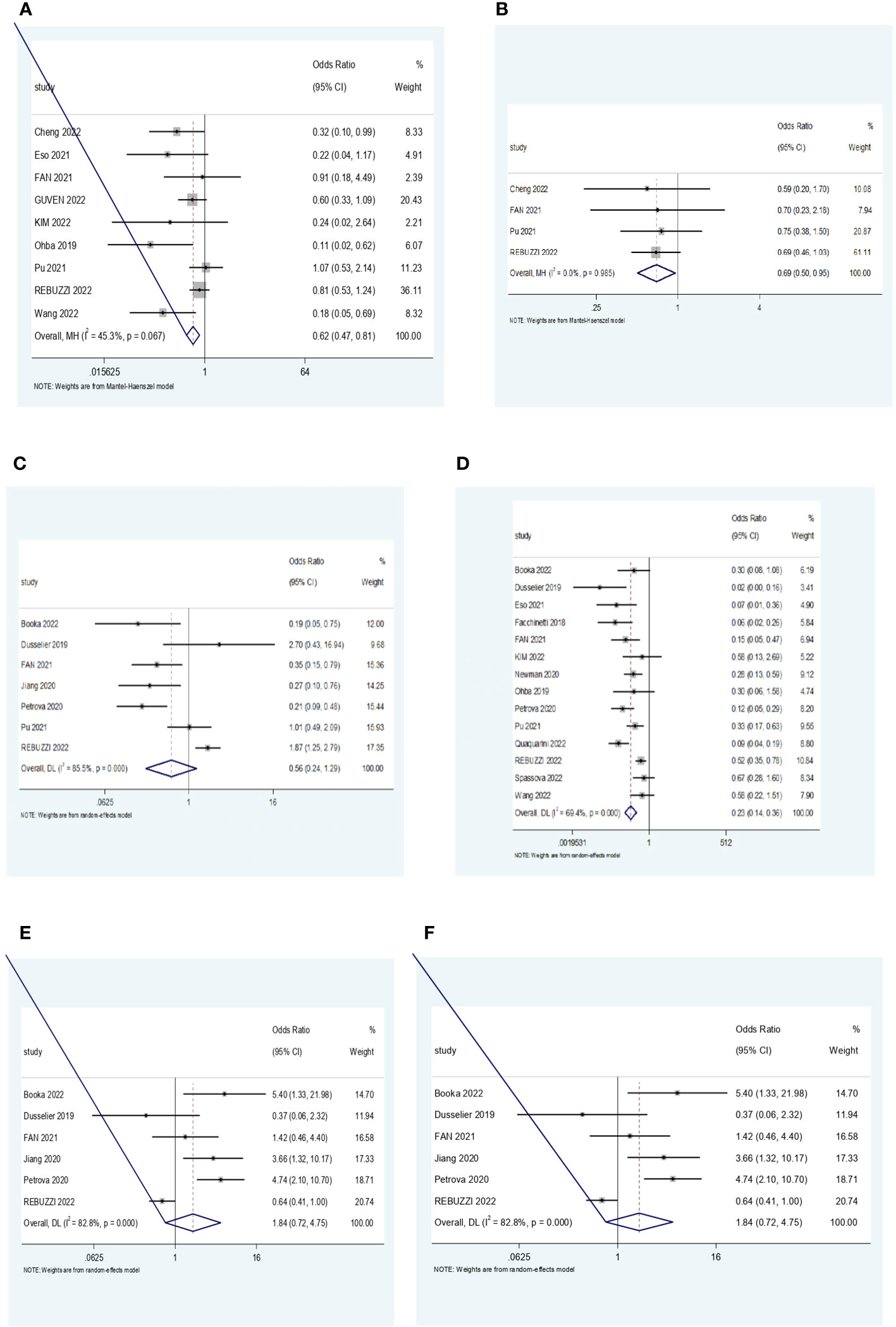
Figure 2 (A) Forest plot for the association NLR and ORR. (B) Forest plot for the association between PLR and ORR. (C) Forest plot for the association between NLR and DCR. (D) Forest plot for the association between PLR and DCR. (E) Forest plot for the association between NLR and PD. (F) Forest plot for the association between PLR and PD. NLR, neutrophil–lymphocyte ratio; ORR, objective response rate; PLR, platelet–lymphocyte ratio; DCR, disease control rate; PD, progressive disease.
3.3.1 Meta-analysis for ORR, DCR, and PD in NLR subgroup
A total of nine studies looked at the impact of NLR on ORR. All studies showed a positive correlation between a low NLR and a higher ORR. Out of the nine studies, five of them showed statistical significance (p < 0.05). The pooled effect estimate (OR) was found to be statistically significant at a value of 0.62 (95% CI 0.47–0.81, p = 0.001). Assessment of heterogeneity suggests that there is low heterogeneity between the studies included in the subgroup meta-analysis (I2 = 45.3%, p = 0.067).
Analysis of DCR included 14 studies, whereby 10 of them showed a statistically significant correlation in favor of low NLR, suggesting that patients with low ratios were more likely to have a better treatment response. The pooled effect estimate (OR) was found to be statistically significant at a value of 0.23 (95% CI 0.14–0.36, p < 0.001). Assessment of heterogeneity suggests that there is high heterogeneity between the studies included in the subgroup meta-analysis (I2 = 69.4%, p = 0.000).
Ten studies reported the correlation of NLR with progressive disease. Eight studies demonstrated that higher NLR was associated with a higher probability of disease progression. One study had contrasting results whereby low NLR was associated with PD. The overall estimate was statistically significant at a value of 3.12 (95% CI 1.44, 6.77, p = 0.004). Assessment of heterogeneity showed substantial heterogeneity among the included studies (I2 = 84.8%, p = 0.000).
3.3.2 Meta-analysis of ORR, DCR, and PD in PLR subgroup
Four studies were pooled to determine the impact of PLR levels on the ORR. All four studies showed a positive correlation between low PLR levels and ORR, but only one study was statistically significant. The pooled effect (OR) was found to be statistically significant at a value of 0.69 (95% CI 0.5, 0.95, p = 0.025). There was no heterogeneity among the studies (I2 = 0.0%, p = 0.985).
In the analysis of DCR, four studies showed a statistically significant positive correlation between low PLR and DCR, suggesting a better treatment response in PLR-low patients. Three studies favored high PLR, but only one of them was statistically significant. The overall estimate (OR) was 0.56 (95% CI 0.24, 1.29, p = 0.172), although it was not statistically significant. Assessment of heterogeneity showed high heterogeneity between the included studies (I2 = 85.5%, p = 0.000).
Six studies were analyzed for the relationship between PLR levels and PD. Four studies reported a positive correlation between high PLR and a higher probability of progressive disease. Meanwhile, two studies were contradicting, suggesting that low PLR levels were associated with the likelihood of disease progression. Out of the six studies, only two studies did not show a statistically significant correlation. The overall estimate was not statistically significant at a value of 1.84 (95% CI 0.72, 4.75, p = 0.205). There was substantial heterogeneity among the studies (I2 = 82.8%, p = 0.000).
3.3.3 Publication bias
Egger’s test and funnel plots were used to assess publication bias (Figures 3A–F). All funnel plots had a symmetrical distribution of studies. However the findings from Egger’s test showed that there was publication bias for studies that reported the association between ORR, DCR and NLR. After performing the trim and fill method, there was no significant change in the results.
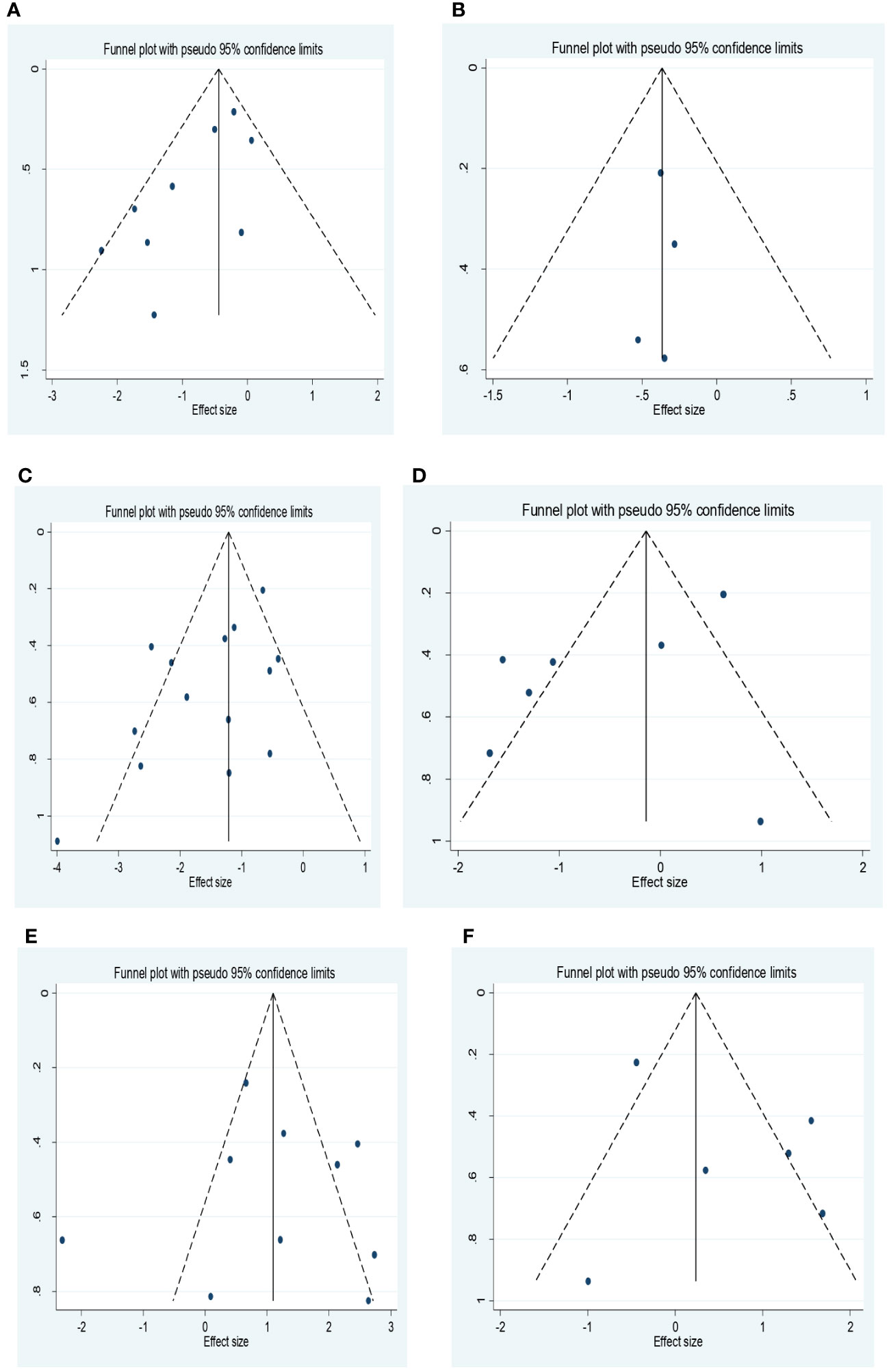
Figure 3 (A) Funnel plot for the association between NLR and ORR. (B) Funnel plot for the association between PLR and ORR. (C) Funnel plot for the association between NLR and DCR. (D) Funnel plot for the association between PLR and DCR. (E) Funnel plot for the association between NLR and PD. (F) Forest plot for the association between PLR and PD. NLR, neutrophil–lymphocyte ratio; ORR, objective response rate; PLR, platelet–lymphocyte ratio; DCR, disease control rate; PD, progressive disease.
4 Discussion
Our review and meta-analysis looked at the correlation between NLR and PLR and treatment response in patients treated with ICIs across different tumors. Generally, patients who had low ratios at baseline or decreasing trend during the course of treatment according to cutoff values pre-determined by authors based on previous studies or derived from the area under the curve (AUC) had a better treatment outcome and were more likely to obtain clinical benefit than those with higher values. Also, they had a lower rate of disease progression compared to the high-ratio group.
The findings of this study correspond with previous studies that looked at the correlation between inflammatory markers and treatment efficacy. A meta-analysis by Guo et al. studying the dynamics of NLR during ICI treatment also showed that patients with a significant upward trend of NLR did not respond to immunotherapy, while those with a downward trend were associated with better clinical and treatment outcomes (16). Similarly, Zhang et al. observed a significant correlation between NLR and ORR (p = 0.003) and a lack of significance between NLR and DCR (p = 0.111) in a meta-analysis involving patients with gastric cancer treated with immunotherapy (58).
There are limited studies on the effect of PLR and response to immunotherapy compared to NLR. Most of the literature focuses on PLR as a prognostic indicator (33, 34, 40, 41, 52–54). One of the studies included in this meta-analysis investigated blood markers before treatment that could be used as predictors of best clinical response (13). With the use of chi-square analysis, the PLR-H (<168.13) group had an inferior stable disease/partial response (SD/PR) rate than the PLR-L (<168.13). However, there were no significant differences in the best clinical response between PD-L1-positive and PD-L1-negative patients. Therefore, the study concluded that PLR could be a better predictive marker to differentiate the best response of ICIs than PD-L1 expression. Likewise in the study by Spassova et al. (37) and Musaelyan et al. (11), there was a lack of a statistically significant relationship between PDL-1 levels and clinical response. A study by Diem et al. also showed that elevated pre-treatment NLR and PLR were independently associated with poorer survival and lower response rates in lung cancer patients treated with nivolumab.
It is well-established that NLR is an independent prognostic factor in different cancers (59). The mechanism behind this observation is that some cancers express chemokines that drive the proliferation of tumor cells. Also, these chemokines drive the influx of myeloid-derived suppressor cells (MDSCs). Examples of those chemokines include CXCL5 and CXCL8, which interact with receptor CXCR2 and CXCR1 expressed on neutrophils. This influx inhibits the tumor-suppressor activity of tumor-infiltrating lymphocytes (TILs) and cytotoxic CD8+ T cells. Additionally, they promote angiogenesis and metastatic potential of cancer cells (59). A study by Kargl et al. in NSCLC patients treated with immunotherapy demonstrated that cells of myeloid origin contributed to treatment failure (60).
Tumor-associated neutrophils (TANs) present in the TME, and neutrophils present in the blood or the bone marrow are linked with resistance to immunotherapy through adaptive immune cell polarization and suppression, tumor neoangiogenesis, immune evasion and exclusion, and tumor intrinsic characteristics. TAN-rich tumors display lower macrophage and TIL infiltration, making them resistant to ICIs. In gastric cancer patients, a sub-population of neutrophils was identified in the peripheral circulation that suppresses CD8+ cell activity. Arginase-1 (ARG1)-expressing human granulocytic cells downregulate T-cell proliferation and cytokine secretion. ARG1+ neutrophils increase with tumor stage in treatment-naive patients and negatively correlate with the number of CD8+ cytotoxic T-cell lymphocytes (61).
Platelet activation is stimulated by pro-inflammatory cytokines and participates in the recruitment of neutrophils (62). They play a fundamental role in systemic and local responses against cancer. They sequester tumor molecules, including RNA and protein transcripts, altering their RNA profiles. After their interaction with the TME, they are called tumor-educated platelets. They transport material from the TME to sites closer to the tumor, creating a favorable environment for the development of metastases. They contain a rich repertoire of RNA varieties, providing biomolecules for diagnosis and prognostic, predictive, or follow-up biomarkers (62).
The prognostic and predictive roles of NLR and PLR cut across most cancer types and in all forms of cancer treatment, not only in immunotherapy. However, the lack of standard cutoff values makes them difficult to apply in clinical practice. Also, baseline values are affected by underlying pre-clinical state, co-morbid systemic conditions, and other confounders.
The findings of this study have shown how heterogeneous the utilization of NLR and PLR as prognostic and predictive factors is. The study has shown that these ratios are predictive but not in all cancers. For example, the study by Wu et al. reported a lack of correlation between inflammatory markers and immune response (63). Moreover, in the same cancer type, one factor could be predictive while the other is not, which indicates that these markers cannot be used as a single entity; rather, they are more functional when combined with other markers in a predictive or prognostic model (64).
Examples of existing models and indexes that are multivariable include neutrophil–platelet score (NPS) (65), which is a systemic inflammation score based on the number of neutrophils and platelets. When tested in NSCLC patients, NPS predicted OS and DCR in pre-treated advanced NSCLC patients who received treatment with nivolumab or pembrolizumab (65). A study by Zhao et al. showed three models, namely, lung immune prognostic index (LIPI) based on pre-treatment blood levels of derived-NLR and lactate dehydrogenase (LDH), EPSILoN (ECOG-PS, smoking, liver metastases, LDH, and NLR), and modified LIPI were predictive and prognostic in immunotherapy (64).
Another study in melanoma patients built a multivariable predictive model for response and survival. A combination of performance status, number of liver and lung metastatic sites, serum LDH, blood NLR, type of treatment (monotherapy vs. combination), and line of treatment was predictive of ORR (14). Another is the Gustave Roussy Immune Score (GRIm-S), which is a composite of neutrophil–lymphocyte ratio (>6 = 1), albumin (<35 = 1), and LDH (>ULN = 1) established as a prognostic score and may aid in the selection of patients for phase 1 trials of immune checkpoint inhibitors (66). Additionally, the Pan-immune inflammation value (PIV), also called the aggregate index of systemic inflammation (AISI), which combines neutrophils, monocytes, platelets, and lymphocytes, is another useful prognostic index (67, 68).
Other prognostic models and indexes utilized in overall cancer treatment include systemic immune-inflammatory index (SII), which combines platelets and NLR (20); advanced lung cancer inflammatory index (ALI), which combines body mass index and the ratio of albumin to NLR (22, 69); and the immune metabolic prognostic index, which is an association of NLR, dNLR, lymphocyte–monocyte ratio (LMR), PLR, and SII (70, 71). In genitourinary tumors, there is a FAN score in urothelial carcinoma that relates to Fibrosis-4-index, albumin–bilirubin ratio, and NLR (72). The International Metastatic RCC Database Consortium (IMDC) predictive score combines hemoglobin levels, serum calcium levels, Karnofsky performance status, time to treatment, and number of platelets and neutrophils (73). The risk blood biomarker (RBB) accounts for the total leukocyte count and ratio of neutrophils, monocytes, and lymphocytes (74). More so, the Glasgow prognostic score (GPS-m) relates to C-reactive protein (CRP), albumin, and NLR (42, 75).
Prospective studies on inflammatory cells that constitute the TME and affect treatment response continue to report other cellular markers apart from neutrophils and platelets. One study reports that more infiltration of cytotoxic CD8+ TCLs present in the intratumoral area was associated with better disease control (37). The study by Musaelyan et al. suggested that other markers of T-cell activation like IL-18 and β2-microglobulin could be used to evaluate and monitor treatment response (11). Another study used artificial intelligence-powered analysis of TILs to generate immunophenotypes, which was shown to correlate with treatment response (76). A combined model of FOXP3+ TCLs and other clinical covariates including NLR was a better predictor of response to immunotherapy in urothelial carcinoma patients (77).
Apart from inflammatory cells, the use of gene expression like circulating tumor DNA (ctDNA) kinetics (78), single-nucleotide variants (SNVs) of PD-1 and PDL-1 (79), gene expression signatures (80), and tumor burden determined from FDG-PET derived metabolic tumor volume (MTV) (81) provide additional biomarkers that predict benefit from ICIs. Pioneer trial (NCT 03493581), which is a comprehensive biomarker analysis for treatment efficacy of ICI with chemotherapy in NSCLC patients, has identified up to 15 biomarker signatures associated with efficacy and progression-free survival (PFS) (82).
This study aimed to highlight the association between inflammatory markers NLR and PLR with disease control, objective response, and disease progression for patients treated with immunotherapy. The study has highlighted that at any point in time before, during, or after treatment, both low and high ratios of NLR and PLR correlate with treatment outcomes regardless of cutoff points, something that was not reported in previous meta-analyses.
Despite the highlighted correlation, the findings are limited by the fact that almost all the included studies were retrospective in nature with a risk of information bias and publication bias. The grouping of patients according to treatment response was not homogeneous. Some studies in the systematic review were not included in the meta-analysis due to the heterogeneous nature of reported data, particularly patients with SD and those with PD. Patients with stable disease were counted with those who progressed as non-responders, while in other studies, they were counted as part of disease control. Also, there was variation in reporting of the ratios, as some studies reported the means and medians, while others just the numerical data or percentages.
Our study was heavily skewed toward NSCLC and melanoma patients, which is attributed to the fact that these were the first tumor sites to obtain Food and Drug Administration (FDA) approval to use ICIs in comparison to other sites. In addition, most patients involved in the studies were treated with pembrolizumab (anti-PD1), nivolumab (anti-PD1) and ipilimumab (anti-CTLA4), and atezolizumab (anti-PDL-1) with limited studies in other agents, hence making it challenging to generalize our findings.
In addition, most of the included studies did not report on the association between NLR and PLR and treatment response in patients treated with immunotherapy according to racial background. Therefore, determining the correlation according to racial background was not possible.
There is a paucity of literature that reported the association of NLR, PLR, and racial background in cancer patients treated with immune checkpoint inhibitors, while some studies that performed sub-group analysis according to country of origin reported contradicting results (58, 83, 84). However, sub-group analysis was not performed in this particular systematic review.
Despite the contradiction, it is evident that with effective treatment, a drop in NLR and PLR correlates with better treatment outcomes and improved survival.
5 Conclusion and recommendations
It is clear that the state of inflammation plays a significant role in treatment response to cancer treatment overall. Inflammatory cells serve as adjunct markers to the FDA-approved biomarkers. The fact that in some studies there was a lack of correlation between PDL-1 levels and treatment response calls for additional markers to augment the predictive and prognostic roles of PDL-1 levels, MSI status, and TMB.
These markers tend to be affected by other underlying co-morbid conditions and the overall state of the body, which compromises their prognostic and predictive functions. Therefore, there is a need to develop a comprehensive clinical model that is reflective of real-world settings and the models to be tested in clinical trials for validation before being incorporated into clinical practice.
Data availability statement
The original contributions presented in the study are included in the article/Supplementary Material. Further inquiries can be directed to the corresponding author.
Author contributions
(I) Conception and design: TR, HS, SZ, and WQ. (II) Collection and assembly of data: XZ, YP, and CC. (III) Data analysis and interpretation: OA and CC. (IV) Manuscript writing: all authors. (V) Final approval of manuscript: all authors.
Funding
This study was supported by grants from the National Natural Science Foundation of China (Nos. 82173342, 81874073, and 81974384), Reform and Development Fund for Colleges and Universities of Hunan Province (No. 2050205), and Nature Science Foundation of Hunan Province (Nos. 2021JJ31092 and 2021JJ31048).
Acknowledgments
The authors wish to acknowledge Fan Jing Li for his assistance in data analysis and Alphonce Nyalali for proofreading the manuscript.
Conflict of interest
The authors declare that the research was conducted in the absence of any commercial or financial relationships that could be construed as a potential conflict of interest.
Publisher’s note
All claims expressed in this article are solely those of the authors and do not necessarily represent those of their affiliated organizations, or those of the publisher, the editors and the reviewers. Any product that may be evaluated in this article, or claim that may be made by its manufacturer, is not guaranteed or endorsed by the publisher.
Supplementary material
The Supplementary Material for this article can be found online at: https://www.frontiersin.org/articles/10.3389/fonc.2023.1181248/full#supplementary-material
References
1. Pecorino L. Molecular biology of cancer : mechanisms, targets, and therapeutics. Oxford [u.a.]: Oxford Univ. Press (2016).
2. Ravindranathan D, Master VA, Bilen MA. Inflammatory markers in cancer immunotherapy. Biology (2021) 10(4):325. doi: 10.3390/biology10040325
3. Tan S, Day D, Nicholls SJ, Segelov E. Immune checkpoint inhibitor therapy in oncology: current uses and future directions: JACC: cardioOncology state-of-the-art review. JACC Cardio Oncol (2022) 4(5):579–97. doi: 10.1016/j.jaccao.2022.09.004
4. Schmid P, Rugo HS, Adams S, Schneeweiss A, Barrios CH, Iwata H, et al. Atezolizumab plus nab-paclitaxel as first-line treatment for unresectable, locally advanced or metastatic triple-negative breast cancer (IMpassion130): updated efficacy results from a randomised, double-blind, placebo-controlled, phase 3 trial. Lancet Oncol (2020) 21(1):44–59. doi: 10.1016/S1470-2045(19)30689-8
5. Chan JJ, Tan TJY, Dent RA. Integrating immunotherapy in the (neo)adjuvant setting of early breast cancer. Curr Opin Oncol (2020) 32(6):575–84. doi: 10.1097/CCO.0000000000000675
6. Fasano M, Corte CMD, Liello RD, Viscardi G, Sparano F, Iacovino ML, et al. Immunotherapy for head and neck cancer: Present and future. Crit Rev Oncol/Hematol (2022) 174:103679. doi: 10.1016/j.critrevonc.2022.103679
7. Ménétrier-Caux C, Ray-Coquard I, Blay JY, Caux C. Lymphopenia in Cancer Patients and its Effects on Response to Immunotherapy: an opportunity for combination with Cytokines? J Immunother Cancer (2019) 7(1):85. doi: 10.1186/s40425-019-0549-5
8. Hinterleitner C, Strähle J, Malenke E, Hinterleitner M, Henning M, Seehawer M, et al. Platelet PD-L1 reflects collective intratumoral PD-L1 expression and predicts immunotherapy response in non-small cell lung cancer. Nat Commun (2021) 12(1):7005. doi: 10.1038/s41467-021-27303-7
9. Riesenberg BP, Li M, Spakowicz D, Hoyd R, Beane J, Yang Y, et al. Platelets impact the responsiveness of immune checkpoint blockade therapy in solid tumors. J Clin Oncol (2020) 38(15_suppl):e15023. doi: 10.1200/JCO.2020.38.15_suppl.e15023
10. Russo A, FranChina T, Ricciardi GRR, Battaglia A, Scimone A, Berenato R, et al. Baseline neutrophilia, derived neutrophil-to-lymphocyte ratio (dNLR), platelet-to-lymphocyte ratio (PLR), and outcome in non small cell lung cancer (NSCLC) treated with Nivolumab or Docetaxel. J Cell Physiol (2018) 233(10):6337–43. doi: 10.1002/jcp.26609
11. Musaelyan AA, Lapin SV, Urtenova MA, Odintsova SV, Chistyakov IV, Ulitin AM, et al. Inflammatory and autoimmune predictive markers of response to anti-PD-1/PD-L1 therapy in NSCLC and melanoma. Exp Ther Med (2022) 24(3):557. doi: 10.3892/etm.2022.11495
12. Wang Y, Tong Z, Zhang W, Zhang W, Buzdin A, Mu X, et al. FDA-approved and emerging next generation predictive biomarkers for immune checkpoint inhibitors in cancer patients. Front Oncol (2021) 11:683419. doi: 10.3389/fonc.2021.683419
13. Jiang M, Peng W, Pu X, Chen B, Li J, Xu F, et al. Peripheral blood biomarkers associated with outcome in non-small cell lung cancer patients treated with nivolumab and durvalumab monotherapy. Front Oncol (2020) 10:913. doi: 10.3389/fonc.2020.00913
14. Silva I, Ahmed T, McQuade JL, Nebhan CA, Park JJ, Versluis JM, et al. Clinical models to define response and survival with anti–PD-1 antibodies alone or combined with ipilimumab in metastatic melanoma. J Clin Oncol (2022) 40(10):1068–80. doi: 10.1200/JCO.21.01701
15. Li Y, Pan Y, Lin X, Hou J, Hu Z, Xu L, et al. Development and validation of a prognostic score for hepatocellular carcinoma patients in immune checkpoint inhibitors therapies: the hepatocellular carcinoma modified gustave roussy immune score. Front Pharmacol (2021) 12:819985. doi: 10.3389/fphar.2021.819985
16. Guo Y, Xiang D, Wan J, Yang L, Zheng C. Focus on the dynamics of neutrophil-to-lymphocyte ratio in cancer patients treated with immune checkpoint inhibitors: A meta-analysis and systematic review. Cancers (2022) 14(21):5297. doi: 10.3390/cancers14215297
17. Chen X, Meng F, Jiang R. Neutrophil-to-lymphocyte ratio as a prognostic biomarker for patients with metastatic renal cell carcinoma treated with immune checkpoint inhibitors: A systematic review and meta-analysis. Front Oncol. (2021) 11:746976. doi: 10.3389/fonc.2021.746976
18. Ksienski D, Wai ES, Alex D, Croteau NS, Freeman AT, Chan A, et al. Prognostic significance of the neutrophil-to-lymphocyte ratio and platelet-to-lymphocyte ratio for advanced non-small cell lung cancer patients with high PD-L1 tumor expression receiving pembrolizumab. Trans Lung Cancer Res (2021) 10(1):355–67. doi: 10.21037/tlcr-20-541
19. Liu D, Czigany Z, Heij LR, Bouwense SAW, van Dam R, Lang SA, et al. The value of platelet-to-lymphocyte ratio as a prognostic marker in cholangiocarcinoma: A systematic review and meta-analysis. Cancers (2022) 14(2):438. doi: 10.3390/cancers14020438
20. Liu J, Li S, Zhang S, Liu Y, Ma L, Zhu J, et al. Systemic immune-inflammation index, neutrophil-to-lymphocyte ratio, platelet-to-lymphocyte ratio can predict clinical outcomes in patients with metastatic non-small-cell lung cancer treated with nivolumab. J Clin Lab Analysis (2019) 33(8):e22964. doi: 10.1002/jcla.22964
21. Sun W, Zhang L, Luo M, Hu G, Mei Q, Liu D, et al. Pretreatment hematologic markers as prognostic factors in patients with nasopharyngeal carcinoma: Neutrophil-lymphocyte ratio and platelet-lymphocyte ratio. Head Neck (2016) 38 Suppl 1:E1332–40. doi: 10.1002/hed.24224
22. Dusselier M, Deluche E, Delacourt N, Ballouhey J, Egenod T, Melloni B, et al. Neutrophil-to-lymphocyte ratio evolution is an independent predictor of early progression of second-line nivolumab-treated patients with advanced non-small-cell lung cancers. PloS One (2019) 14(7):e0219060. doi: 10.1371/journal.pone.0219060
23. Facchinetti F, Veneziani M, Buti S, Gelsomino F, Squadrilli A, Bordi P, et al. Clinical and hematologic parameters address the outcomes of non-small-cell lung cancer patients treated with nivolumab. Immunotherapy (2018) 10(8):681–94. doi: 10.2217/imt-2017-0175
24. Newman J, Preeshagul I, Kohn N, Devoe C, Seetharamu N. Simple parameters to solve a complex issue: Predicting response to checkpoint inhibitor therapy in lung cancer. Lung Cancer Manage (2021) 10(2). doi: 10.2217/lmt-2020-0024
25. Ohba T, Takamori S, Toyozawa R, Nosaki K, Umeyama Y, Haratake N, et al. Prognostic impact of the Controlling Nutritional Status score in patients with non-small cell lung cancer treated with pembrolizumab. J Thorac Dis (2019) 11(9):3757–68. doi: 10.21037/jtd.2019.09.29
26. Petrova MP, Eneva MI, Arabadjiev JI, Conev NV, Dimitrova EG, Koynov KD, et al. Neutrophil to lymphocyte ratio as a potential predictive marker for treatment with pembrolizumab as a second line treatment in patients with non-small cell lung cancer. Biosci Trends (2020) 14(1):48–55. doi: 10.5582/bst.2019.01279
27. Pu D, Xu Q, Zhou LY, Zhou YW, Liu JY, Ma XL. Inflammation-nutritional markers of peripheral blood could predict survival in advanced non-small-cell lung cancer patients treated with PD-1 inhibitors. Thorac Cancer (2021) 12(21):2914–23. doi: 10.1111/1759-7714.14152
28. Quaquarini E, Sottotetti F, Agustoni F, Pozzi E, Malovini A, Teragni CM, et al. Clinical and biological variables influencing outcome in patients with advanced non-small cell lung cancer (NSCLC) treated with anti-PD-1/PD-L1 antibodies: A prospective multicentre study. J Pers Med (2022) 12(5):679. doi: 10.3390/jpm12050679
29. Booka E, Kikuchi H, Haneda R, Soneda W, Kawata S, Murakami T, et al. Neutrophil-to-lymphocyte ratio to predict the efficacy of immune checkpoint inhibitor in upper gastrointestinal cancer. Anticancer Res (2022) 42(6):2977–87. doi: 10.21873/anticanres.15781
30. Fan X, Wang D, Zhang W, Liu J, Liu C, Li Q, et al. Inflammatory markers predict survival in patients with advanced gastric and colorectal cancers receiving anti–PD-1 therapy. Front Cell Dev Biol. (2021) 9:638312. doi: 10.3389/fcell.2021.638312
31. Kim JH, Ryu MH, Park YS, Ma J, Lee SY, Kim D, et al. Predictive biomarkers for the efficacy of nivolumab as ≥ 3(rd)-line therapy in patients with advanced gastric cancer: a subset analysis of ATTRACTION-2 phase III trial. BMC Cancer (2022) 22(1):378. doi: 10.1186/s12885-022-09488-2
32. Wang L, Zhu Y, Zhang B, Wang X, Mo H, Jiao Y, et al. Prognostic and predictive impact of neutrophil-to-lymphocyte ratio and HLA-I genotyping in advanced esophageal squamous cell carcinoma patients receiving immune checkpoint inhibitor monotherapy. Thorac Cancer (2022) 13(11):1631–41. doi: 10.1111/1759-7714.14431
33. Eso Y, Takeda H, Taura K, Takai A, Takahashi K, Seno H. Pretreatment neutrophil-to-lymphocyte ratio as a predictive marker of response to atezolizumab plus bevacizumab for hepatocellular carcinoma. Curr Oncol (2021) 28(5):4157–66. doi: 10.3390/curroncol28050352
34. Rebuzzi SE, Signori A, Stellato M, Santini D, Maruzzo M, De Giorgi U, et al. The prognostic value of baseline and early variations of peripheral blood inflammatory ratios and their cellular components in patients with metastatic renal cell carcinoma treated with nivolumab: The Δ-Meet-URO analysis. Front Oncol. (2022) 12:955501. doi: 10.3389/fonc.2022.955501
35. Guven DC, Sahin TK, Erul E, Cakir IY, Ucgul E, Yildirim HC, et al. The association between early changes in neutrophil-lymphocyte ratio and survival in patients treated with immunotherapy. J Clin Med (2022) 11(15):4523. doi: 10.3390/jcm11154523
36. Cheng M, Li G, Liu Z, Yang Q, Jiang Y. Pretreatment neutrophil-to-lymphocyte ratio and lactate dehydrogenase predict the prognosis of metastatic cervical cancer treated with combination immunotherapy. J Oncol (2022) 2022:1828473. doi: 10.1155/2022/1828473
37. Spassova I, Ugurel S, Kubat L, Zimmer L, Terheyden P, Mohr A, et al. Clinical and molecular characteristics associated with response to therapeutic PD-1/PD-L1 inhibition in advanced Merkel cell carcinoma. J Immunother Cancer (2022) 10(1):e003198. doi: 10.1136/jitc-2021-003198
38. Möller M, Turzer S, Ganchev G, Wienke A, Schütte W, Seliger B, et al. Blood immune cell biomarkers in lung cancer patients undergoing treatment with a combination of chemotherapy and immune checkpoint blockade. Cancers (Basel) (2022) 14(15):3690. doi: 10.3390/cancers14153690
39. Park CK, Oh HJ, Kim MS, Koh BG, Cho HJ, Kim YC, et al. Comprehensive analysis of blood-based biomarkers for predicting immunotherapy benefits in patients with advanced non-small cell lung cancer. Trans Lung Cancer Res (2021) 10(5):2103–17. doi: 10.21037/tlcr-21-100
40. Guida M, Bartolomeo N, Quaresmini D, Quaglino P, Madonna G. Basal and one-month differed neutrophil, lymphocyte and platelet values and their ratios strongly predict the efficacy of checkpoint inhibitors immunotherapy in patients with advanced BRAF wild-type melanoma. J Transl Med (2022) 20(1):159. doi: 10.1186/s12967-022-03359-x
41. Hung HC, Lee JC, Wang YC, Cheng CH, Wu TH, Lee CF, et al. Response prediction in immune checkpoint inhibitor immunotherapy for advanced hepatocellular carcinoma. Cancers (2021) 13(7). doi: 10.3390/cancers13071607
42. Yamamoto Y, Yatsuda J, Shimokawa M, Fuji N, Aoki A, Sakano S, et al. Prognostic value of pre-treatment risk stratification and post-treatment neutrophil/lymphocyte ratio change for pembrolizumab in patients with advanced urothelial carcinoma. Int J Clin Oncol (2021) 26(1):169–77. doi: 10.1007/s10147-020-01784-w
43. Nenclares P, Gunn L, Soliman H, Bover M, Trinh A, Leslie I, et al. On-treatment immune prognostic score for patients with relapsed and/or metastatic head and neck squamous cell carcinoma treated with immunotherapy. J ImmunoTher Cancer (2021) 9(6). doi: 10.1136/jitc-2021-002718
44. Benzekry S, Grangeon M, Karlsen M, Alexa M, Bicalho-Frazeto I, Chaleat S, et al. Machine learning for prediction of immunotherapy efficacy in non-small cell lung cancer from simple clinical and biological data. Cancers (2021) 13(24). doi: 10.3390/cancers13246210
45. Liu X, Shi Y, Zhang D, Zhou Q, Liu J, Chen M, et al. Risk factors for immune-related adverse events: what have we learned and what lies ahead? biomark Res (2021) 9(1):79. doi: 10.1186/s40364-021-00314-8
46. Huang L, Li L, Zhou Y, Yang Z, Wang M, Gao Y, et al. Clinical characteristics correlate with outcomes of immunotherapy in advanced non-small cell lung cancer. J Cancer (2020) 11(24):7137–45. doi: 10.7150/jca.49213
47. Khunger M, Patil PD, Khunger A, Li M, Hu B, Rakshit S, et al. Post-treatment changes in hematological parameters predict response to nivolumab monotherapy in non-small cell lung cancer patients. PloS One (2018) 13(10):e0197743. doi: 10.1371/journal.pone.0197743
48. Mountzios G, Samantas E, Senghas K, Zervas E, Krisam J, Samitas K, et al. Association of the advanced lung cancer inflammation index (ALI) with immune checkpoint inhibitor efficacy in patients with advanced non-small-cell lung cancer. ESMO Open (2021) 6(5). doi: 10.1016/j.esmoop.2021.100254
49. Wu M, Liu J, Wu S, Liu J, Wu H, Yu J, et al. Systemic immune activation and responses of irradiation to different metastatic sites combined with immunotherapy in advanced non-small cell lung cancer. Front Immunol (2021) 12. doi: 10.3389/fimmu.2021.803247
50. Nakazawa N, Sohda M, Ubukata Y, Kuriyama K, Kimura A, Kogure N, et al. Changes in the gustave roussy immune score as a powerful prognostic marker of the therapeutic sensitivity of nivolumab in advanced gastric cancer: A multicenter, retrospective study. Ann Surg Oncol (2022) 29(12):7400–6. doi: 10.1245/s10434-022-12226-4
51. Namikawa T, Yokota K, Tanioka N, Fukudome I, Iwabu J, Munekage M, et al. Systemic inflammatory response and nutritional biomarkers as predictors of nivolumab efficacy for gastric cancer. Surg Today (2020) 50(11):1486–95. doi: 10.1007/s00595-020-02048-w
52. Ohashi H, Takeuchi S, Miyagaki T, Kadono T. Increase of lymphocytes and eosinophils, and decrease of neutrophils at an early stage of anti-PD-1 antibody treatment is a favorable sign for advanced Malignant melanoma. Drug Discoveries Ther (2020) 14(3):117–21. doi: 10.5582/ddt.2020.03043
53. Guida M, Bartolomeo N, Quaglino P, Madonna G, Pigozzo J, Di Giacomo AM, et al. No impact of NRAS mutation on features of primary and metastatic melanoma or on outcomes of checkpoint inhibitor immunotherapy: An italian melanoma intergroup (IMI) study. Cancers (2021) 13(3):1–15. doi: 10.3390/cancers13030475
54. Tanaka T, Takata K, Yokoyama K, Fukuda H, Yamauchi R, Fukunaga A, et al. Pretreatment modified albumin–bilirubin grade is an important predictive factor associated with the therapeutic response and the continuation of atezolizumab plus bevacizumab combination therapy for patients with unresectable hepatocellular carcinoma. Curr Oncol (2022) 29(7):4799–810. doi: 10.3390/curroncol29070381
55. Simonaggio A, Elaidi R, Fournier L, Fabre E, Ferrari V, Borchiellini D, et al. Variation in neutrophil to lymphocyte ratio (NLR) as predictor of outcomes in metastatic renal cell carcinoma (mRCC) and non-small cell lung cancer (mNSCLC) patients treated with nivolumab. Cancer Immunol Immunother CII (2020) 69(12):2513–22. doi: 10.1007/s00262-020-02637-1
56. Lee YG, Chang H, Keam B, Chun SH, Park J, Park KU, et al. Outcomes and biomarkers of immune checkpoint inhibitor therapy in patients with refractory head and neck squamous cell carcinoma: Kcsg hn18-12. Cancer Res Treat (2021) 53(3):671–7. doi: 10.4143/CRT.2020.824
57. Criscitiello C, Marra A, Morganti S, Zagami P, Viale G, Esposito A, et al. Pretreatment blood parameters predict efficacy from immunotherapy agents in early phase clinical trials. Oncol (2020) 25(11):e1732–e42. doi: 10.1634/theoncologist.2020-0518
58. Zhang S, Qiu C, Yu H, Xu Y, Xu X. Prognostic value of neutrophil to lymphocyte ratio in gastric cancer patients receiving immune checkpoint inhibitors: a systematic review and meta-analysis. Front Oncol (2023) 13:1070019. doi: 10.3389/fonc.2023.1070019
59. Rapoport BL, Steel HC, Theron AJ, Smit T, Anderson R. Role of the neutrophil in the pathogenesis of advanced cancer and impaired responsiveness to therapy. Mol (Basel Switzerland) (2020) 25(7). doi: 10.3390/molecules25071618
60. Kargl J, Zhu X, Zhang H, Yang GHY, Friesen TJ, Shipley M, et al. Neutrophil content predicts lymphocyte depletion and anti-PD1 treatment failure in NSCLC. JCI Insight (2019) 4(24). doi: 10.1172/jci.insight.130850
61. Faget J, Peters S, Quantin X, Meylan E, Bonnefoy N. Neutrophils in the era of immune checkpoint blockade. J Immunother Cancer (2021) 9(7). doi: 10.1136/jitc-2020-002242
62. Honrubia-Peris B, Garde-Noguera J, García-Sánchez J, Piera-Molons N, Llombart-Cussac A, Fernández-Murga ML. Soluble biomarkers with prognostic and predictive value in advanced non-small cell lung cancer treated with immunotherapy. Cancers (2021) 13(17). doi: 10.3390/cancers13174280
63. Wu M, Liu J, Wu S, Liu J, Wu H, Yu J, et al. Systemic immune activation and responses of irradiation to different metastatic sites combined with immunotherapy in advanced non-small cell lung cancer. Front Immunol (2021) 12:803247. doi: 10.3389/fimmu.2021.803247
64. Zhao Q, Li B, Xu Y, Wang S, Zou B, Yu J, et al. Three models that predict the efficacy of immunotherapy in Chinese patients with advanced non-small cell lung cancer. Cancer Med (2021) 10(18):6291–303. doi: 10.1002/cam4.4171
65. Mielgo Rubio X, Sereno Moyano M, Chara LE, López-Castro R, Rubio-Martínez J, Velastegui A, et al. Neutrophil-platelet score (NPS), a predictive systemic inflammation score for PD-1 immune checkpoint inhibitors (ICI) in pretreated advanced non-small cell lung cancer (NSCLC) patients. Ann Oncol (2018) 29:viii510. doi: 10.1093/annonc/mdy292.035
66. Ma LX, Wang Y, Espin-Garcia O, Allen MJ, Jang GH, Zhang A, et al. Systemic inflammatory prognostic scores in advanced pancreatic adenocarcinoma. Br J Cancer (2023) 128(10):1916-21. doi: 10.1038/s41416-023-02214-0
67. Ginesu GC, Paliogiannis P, Feo CF, Cossu ML, Scanu AM, Fancellu A, et al. Inflammatory indexes as predictive biomarkers of postoperative complications in oncological thoracic surgery. Curr Oncol (Toronto Ont) (2022) 29(5):3425–32. doi: 10.3390/curroncol29050276
68. Şahin AB, Cubukcu E, Ocak B, Deligonul A, Oyucu Orhan S, Tolunay S, et al. Low pan-immune-inflammation-value predicts better chemotherapy response and survival in breast cancer patients treated with neoadjuvant chemotherapy. Sci Rep (2021) 11(1):14662. doi: 10.1038/s41598-021-94184-7
69. Shiroyama T, Suzuki H, Tamiya M, Tamiya A, Tanaka A, Okamoto N, et al. Pretreatment advanced lung cancer inflammation index (ALI) for predicting early progression in nivolumab-treated patients with advanced non-small cell lung cancer. Cancer Med (2018) 7(1):13–20. doi: 10.1002/cam4.1234
70. Bauckneht M, Genova C, Rossi G, Rijavec E, Dal Bello MG, Ferrarazzo G, et al. The role of the immune metabolic prognostic index in patients with non-small cell lung cancer (NSCLC) in radiological progression during treatment with nivolumab. Cancers (2021) 13(13). doi: 10.3390/cancers13133117
71. Castello A, Toschi L, Rossi S, Mazziotti E, Lopci E. The immune-metabolic-prognostic index and clinical outcomes in patients with non-small cell lung carcinoma under checkpoint inhibitors. J Cancer Res Clin Oncol (2020) 146(5):1235–43. doi: 10.1007/s00432-020-03150-9
72. Kawashima A, Yamamoto Y, Sato M, Nakata W, Kakuta Y, Ishizuya Y, et al. FAN score comprising fibrosis-4 index, albumin–bilirubin score and neutrophil–lymphocyte ratio is a prognostic marker of urothelial carcinoma patients treated with pembrolizumab. Sci Rep (2021) 11(1):21199. doi: 10.1038/s41598-021-00509-x
73. Zheng W, Zhu W, Yu S, Li K, Ding Y, Wu Q, et al. Development and validation of a nomogram to predict overall survival for patients with metastatic renal cell carcinoma. BMC Cancer (2020) 20(1):1066. doi: 10.1186/s12885-020-07586-7
74. Sánchez-Gastaldo A, Muñoz-Fuentes MA, Molina-Pinelo S, Alonso-García M, Boyero L, Bernabé-Caro R. Correlation of peripheral blood biomarkers with clinical outcomes in NSCLC patients with high PD-L1 expression treated with pembrolizumab. Trans Lung Cancer Res (2021) 10(6):2509–22. doi: 10.21037/tlcr-21-156
75. Namikawa K, Takahashi A, Mori T, Tsutsumida A, Suzuki S, Motoi N, et al. Nivolumab for patients with metastatic uveal melanoma previously untreated with ipilimumab: a single-institution retrospective study. Melanoma Res (2020) 30(1):76–84. doi: 10.1097/cmr.0000000000000617
76. Park S, Ock C-Y, Kim H, Pereira S, Park S, Ma M, et al. Artificial intelligence–powered spatial analysis of tumor-infiltrating lymphocytes as complementary biomarker for immune checkpoint inhibition in non–small-cell lung cancer. J Clin Oncol (2022) 40(17):1916–28. doi: 10.1200/JCO.21.02010
77. Adib E, Zarif TE, Freeman D, Labban M, Curran C, Sharma B, et al. FOXP3+ T-cell infiltration is associated with improved outcomes in metastatic urothelial carcinoma (mUC) treated with immune-checkpoint inhibitors (ICI). J Clin Oncol (2022) 40(6_suppl):549–. doi: 10.1200/JCO.2022.40.6_suppl.549
78. Sanz Garcia E, Laliotis G, Avery L, Spreafico A, Hansen AR, Eng L, et al. 9P Early circulating tumor DNA (ctDNA) kinetics and gene expression analysis to predict treatment outcomes with anti-PD-1 therapy. Immuno-Oncol Technol (2022) 16:100114. doi: 10.1016/j.iotech.2022.100114
79. Boutros A, Carosio R, Campanella D, Spagnolo F, Banelli B, Morabito A, et al. 24P The predictive and prognostic role of single nucleotide gene variants in PD-1 and PD-L1 in patients with advanced melanoma treated with PD-1 inhibitors. Immuno-Oncol Technol (2022) 16:100129. doi: 10.1016/j.iotech.2022.100129
80. Tarhini AA, Coleman SS, El Naqa I, Sukrithan V, Aakrosh R, McCarter M, et al. 18P Systematic evaluation of published predictive gene expression signatures in pan-cancer patient cohorts treated with immune checkpoint inhibitors in a real-world setting. Immuno-Oncol Technol (2022) 16:100123. doi: 10.1016/j.iotech.2022.100123
81. Dall'Olio FG, Garcia CA, Zrafi WS, Fortunati E, Bettayeb A, Zalcman G, et al. 4P FDG PET derived metabolic tumor volume (MTV) and its transcriptomic correlates as biomarker to predict efficacy of immune checkpoint inhibitors (ICB) alone or in combination with chemotherapy in advanced NSCLC: A multicentric study. Immuno-Oncol Technol (2022) 16:100109. doi: 10.1016/j.iotech.2022.100109
82. Barlesi F, Greillier L, Monville F, Audigier Valette C, Martinez S, Cloarec N, et al. 3MO Comprehensive biomarkers (BMS) analysis to predict efficacy of PD1/L1 immune checkpoint inhibitors (ICIs) in combination with chemotherapy: A subgroup analysis of the precision immuno-oncology for advanced non-small cell lung cancer (pioneer) trial. Immuno-Oncol Technol (2022) 16:100108. doi: 10.1016/j.iotech.2022.100108
83. Li Y, Zhang Z, Hu Y, Yan X, Song Q, Wang G, et al. Pretreatment neutrophil-to-lymphocyte ratio (NLR) may predict the outcomes of advanced non-small-cell lung cancer (NSCLC) patients treated with immune checkpoint inhibitors (ICIs). Front Oncol (2020) 10:654. doi: 10.3389/fonc.2020.00654
Keywords: neutrophil-lymphocyte ratio, platelet-lymphocyte ratio, immune checkpoint inhibitors, predictive, biomarkers, response
Citation: Rugambwa TK, Abdihamid O, Zhang X, Peng Y, Cai C, Shen H, Zeng S and Qiu W (2023) Neutrophil–lymphocyte ratio and platelet–lymphocyte ratio as potential predictive markers of treatment response in cancer patients treated with immune checkpoint inhibitors: a systematic review and meta-analysis. Front. Oncol. 13:1181248. doi: 10.3389/fonc.2023.1181248
Received: 07 March 2023; Accepted: 26 September 2023;
Published: 26 October 2023.
Edited by:
Takaji Matsutani, Repertoire Genesis, Inc., JapanReviewed by:
Xiaobin Shang, Tianjin Medical University Cancer Institute and Hospital, ChinaLei Yang, Harbin Medical University, China
Hashem Obaid Alsaab, Taif University, Saudi Arabia
Copyright © 2023 Rugambwa, Abdihamid, Zhang, Peng, Cai, Shen, Zeng and Qiu. This is an open-access article distributed under the terms of the Creative Commons Attribution License (CC BY). The use, distribution or reproduction in other forums is permitted, provided the original author(s) and the copyright owner(s) are credited and that the original publication in this journal is cited, in accordance with accepted academic practice. No use, distribution or reproduction is permitted which does not comply with these terms.
*Correspondence: Wei Qiu, 15973881888@163.com