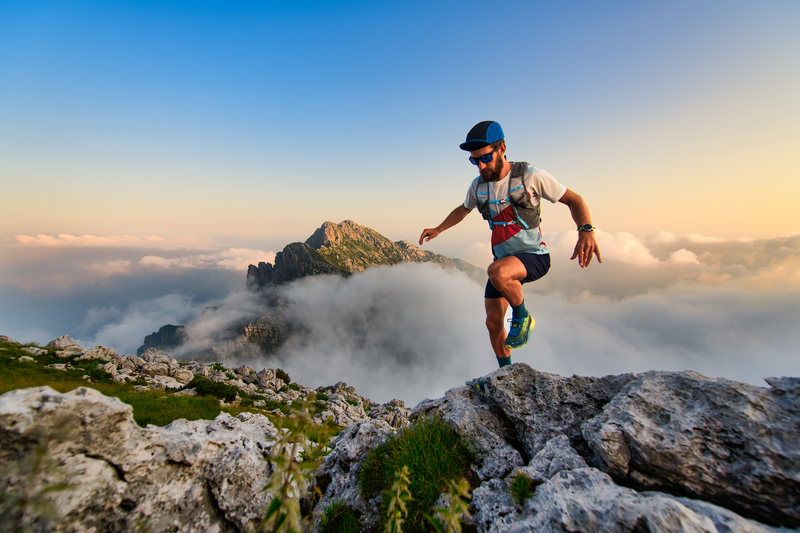
95% of researchers rate our articles as excellent or good
Learn more about the work of our research integrity team to safeguard the quality of each article we publish.
Find out more
ORIGINAL RESEARCH article
Front. Oncol. , 26 May 2023
Sec. Gastrointestinal Cancers: Hepato Pancreatic Biliary Cancers
Volume 13 - 2023 | https://doi.org/10.3389/fonc.2023.1169102
This article is part of the Research Topic Transarterial Chemoembolization for Hepatocellular Carcinoma Patients View all 13 articles
Background: Postoperative adjuvant transarterial chemoembolization (PA-TACE) has been increasing widely used to improve the prognosis of hepatocellular carcinoma (HCC) patients. However, clinical outcomes vary from patient to patient, which calls for individualized prognostic prediction and early management.
Methods: A total of 274 HCC patients who underwent PA-TACE were enrolled in this study. The prediction performance of five machine learning models was compared and the prognostic variables of postoperative outcomes were identified.
Results: Compared with other machine learning models, the risk prediction model based on ensemble learning strategies, including Boosting, Bagging, and Stacking algorithms, presented better prediction performance for overall mortality and HCC recurrence. Moreover, the results showed that the Stacking algorithm had relatively low time consumption, good discriminative ability, and the best prediction performance. In addition, according to time-dependent ROC analysis, the ensemble learning strategies were found to perform well in predicting both OS and RFS for the patients. Our study also found that BCLC Stage, hsCRP/ALB and frequency of PA-TACE were relatively important variables in both overall mortality and recurrence, while MVI contributed more to the recurrence of the patients.
Conclusion: Among the five machine learning models, the ensemble learning strategies, especially the Stacking algorithm, could better predict the prognosis of HCC patients following PA-TACE. Machine learning models could also help clinicians identify the important prognostic factors that are clinically useful in individualized patient monitoring and management.
Liver cancer is the sixth most prevalent malignancy and the third leading cause of cancer-related death worldwide (1, 2). By 2025, the estimated incidence of liver cancer may exceed 1 million (3). Hepatocellular carcinoma (HCC) is the most common primary liver cancer, accounting for about 75%-85% (4). Although curative hepatectomy is still recommended as the main curative treatment for HCC patients with adequate liver function (5, 6), postoperative prognosis of HCC patients is jeopardized by a high recurrence rate (7). Therefore, several postoperative adjuvant therapies have been developed to reduce the risk of recurrence and improve overall survival (8–10). Transarterial chemoembolization (TACE), which has long been one of the first-line treatments for unresectable HCC (11), is now most widely used as an adjuvant therapy after curative resection for HCC with many recurrence risk factors (12–14). Substantial studies have also shown that postoperative adjuvant TACE (PA-TACE) is beneficial for HCC patients with more tumor numbers, larger tumor size, and microvascular invasion (15–18), especially for those with portal vein tumor thrombus (19). However, few studies have established effective and practical prediction models for prognosis of HCC patients underwent PA-TACE and achieved satisfactory prediction efficacy. Therefore, novel prediction models are needed to facilitate clinical decision making in early management and further improve patient outcomes.
Machine learning, a type of computer science that makes empirical predictions from multi-dimensional datasets, is increasingly being applied to modern medical research, including HCC (20–23). In the prognostic prediction of HCC, it has shown superior advantages in image recognition and feature selection compared with traditional methods, thereby improving the accuracy of the model prediction and subsequent results (21, 22, 24). The rise of artificial intelligence (AI) technology has also brought many new machine learning strategies to predict patient prognosis. In recent researches, several ensemble learning strategies, including Boosting and Bagging algorithm have been developed for HCC and achieved encouraging results (25–27). Different from other machine learning methods that typically apply one model or one algorithm to a specific task, ensemble learning performs greater flexibility in model selection. Specific training strategies could be set for complex clinical datasets to improve the performance of the ensemble learning strategy.
In summary, this study aimed to utilize and compare different machine learning algorithms to establish a better prediction model for survival and recurrence of HCC patients underwent PA-TACE. Five machine learning models, including three novel ensemble learning models and two other models, were selected to provide intelligent postoperative monitoring and management for the patients. We also explored the variable importance and verified important prognostic indicators of postoperative outcomes.
The database was retrospectively derived from HCC patients who received curative resection and PA-TACE at Sichuan Provincial People’s Hospital between May 2018 and May 2022. The inclusion criteria were included as following: (1) pathological confirmation of HCC; (2) no preoperative therapy for primary HCC; (3) R0 surgical resection of tumor with curative intent; (4) TACE as the only adjuvant treatment; (5) received the first adjuvant TACE within 2 months after resection. Patients who (1) having history of non-HCC malignancies or concurrent with other malignancies; (2) diagnosed with HCC relapse, or distant metastasis; (3) died within 30 days after surgery or lost to follow-up were excluded from this study. The flow chart of the study design can be found in Figure 1.
Figure 1 The flowchart of patients enrolled in this study. HCC, hepatocellular carcinoma; PA-TACE, postoperative adjuvant transarterial chemoembolization; ML, machine learning; DL, deep learning.
The study was approved by the Human Ethics Committee of Sichuan Academy of Medical Sciences and Sichuan Provincial People’s Hospital, and written informed consent was obtained from all participants. All procedures were performed in accordance with the ethical guidelines of the Helsinki Declaration.
The clinicopathological characteristics collected from the database included laboratory tests, tumor characteristics, inflammatory-based prognostic indices, and clinical stages. All laboratory tests were collected within 1 week before the operation, including serum indicators, liver and coagulation functions, and hepatitis B virus markers. The tumor characteristics included differentiation, cirrhosis, the number of tumors, the diameter of the largest nodule, microvascular invasion and so on. Microvascular invasion (MVI) is defined as the presence of HCC microemboli in blood vessels lined by endothelial cells under histological microscope (28). Nowadays, various inflammatory-based prognostic biomarkers are widely used to predict the prognosis of cancer patient. Our study included neutrophil lymphocyte ratio (NLR), platelet lymphocyte ratio (PLR), systemic immune-inflammation index (SII), systemic inflammation response index (SIRI), high sensitivity C-reactive protein (hsCRP)/albumin (ALB), and prognostic nutritional index (PNI). NLR and PLR were calculated as neutrophil/lymphocyte counts and platelet/lymphocyte counts, respectively (29). SII and SIRI were defined as platelet × neutrophil/lymphocyte counts and monocytes × neutrophil/lymphocyte counts, respectively (29, 30). The calculation formula of PNI was as follow: albumin level (g/L) + 5× total lymphocyte count (109/L) (31). The clinical stages included Child-Pugh grade, and Barcelona Clinic Liver Cancer (BCLC) stage.
After the surgery, the follow-up was conducted every 3 months in the first year, and then every 6 months thereafter if there was no recurrence or metastasis. The primary outcome was overall survival (OS), which was defined as the time interval from the surgery to death, or the end of the follow-up (July 2022), whichever came first. And the secondary outcome was recurrence-free survival (RFS), which was defined as the time interval from the surgery to death, recurrence, metastasis, or the end of the follow-up (July 2022), whichever came first.
Continuous variables were expressed as the medians and interquartile ranges (Q1–Q3), and categorical variables were expressed as frequency (%). Time-dependent ROC curves were used to detect the prognostic performance of the Boosting, Bagging, and Stacking model for OS and RFS, respectively. Survival curves were plotted using the Kaplan-Meier method and the differences were compared by log rank test. The learning rate represents the step size of the model iteration, and the number of estimators means the number of base learners (base models). Two-sided p< 0.05 was considered statistically significant. All statistical analyses were performed using Python version v3.8.10 and GraphPad Prism version 9.2.0.
As a commonly used supervised classification algorithm, KNN (K Nearest Neighbors) has a simple structure and good performance. According to different weight calculation of the neighbor node, there are 2 kinds of model, namely KNN (uniform) and KNN (distance).
If the weight is uniform, the value assigned to a point is calculated according to the simple majority vote of the nearest neighbors. However, in some cases, it is better to weight the neighbors so that the closer neighbors can make more contributions to the fit. Therefore, the second calculation method allocates weights proportional to the reciprocal of the distance from the query point.
Boosting is a commonly used ensemble learning strategy. To get better knowledge about how boosting works and why this strategy is useful for clinical data, we used 3 kinds of boosting algorithm in the present study.
XGBoost (Optimized Parallel Tree Boosting) support CART (Classification and Regression Trees) and linear classifier at the same time with high flexibility. The XGBoost with linear classifier can be considered as Logistic regression or linear regression with L1 and L2 regularization. Regularization, which contains the number of leaf node on the tree and L2 regularization of the weight of the leaf node, is be used to balance the model complexity and avoid overfitting. Compared with some deep learning methods, XGBoost has simpler structure and greater interpretability.
Compared with XGBoost, LightGBM gets a breakthrough in memory consumption and calculation speed to some extent. Within histogram algorithm, we change the traversal over samples to traversal on histogram and get performance improvement. At the same time, to speed up further, we present Gradient-based One-Side Sampling (GOSS) to filter out those samples with small gradient. Considering the high calculating speed, the model can be applied to real-time operation (e.g., large-scale medical real-time data analysis).
CatBoost perform roughly between XGBoost and LightGBM, but in view of no parameters adjustment and ordered boosting application to avoid prediction offset, this model wins in simplicity and efficiency.
Bagging, including ExtraTrees (Gini, Entr) and RandomForest (Gini, Entr), is also a very commonly used ensemble learning strategy.
As a set of many decision trees, RandomForest perform well on multivariate data due to the composite structure of processing discrete data and continuous data concurrently. There is no need to reduce dimension and choose any other feature selection tools. Also, RandomForest can measure the impact of different attributes, which can help understand multiple indicators of the patient. According to different nodes split type, we construct two kinds of model with Gini coefficient and entropy.
ExtraTrees (Extremely Randomized Trees) enable each base decision tree to use the same original dataset. Overall, ExtraTrees is quite similar to RandomForest except for higher variance and lower bias. Sometimes, ExtraTrees perform better in terms of regularization, so we chose to use this model for comparison in the medical tasks.
For models, we consider combining some base learners, including Boosting or Bagging, to perform well. For datasets, we also set a specific training strategy to improve performance of the ensemble model.
Stacking aims at building a new model from several base models through feature transformation on training and testing sets. As shown in Figure 2, M represents a base model. We divide training set into N pieces and choose 1 piece for validation, while the other N-1 pieces are used to train the base model. After training, we get test and valid results. Then, we take an average of the N test results and get the test set feature transformation. We concatenate N valid results and get the valid set feature transformation.
Figure 2 The stacking training process for a single model, including dataset segmentation and result integration.
For N base models, we proposed the method in Figure 3. In Figure 3, we concatenate N pairs of train-test and original train-test to get a new dataset and assign it to be the input of the N+1 models. Different from the normal stacking strategy with linear regression(model*) setting on high stacking layer to avoid overfitting, we still set base models on high stacking layer, which can help get better performance. Then we calculate suitable weight combination and get the prediction result from the N+1 models.
At first, we did not adopt any deep learning models because of the risk of overfitting and weak interpretability. But AutoGluon offers a different network, which applies different layers for categorical and numerical data.
For multivariate data, individual embedding layers enable the network to learn about each category feature individually before mixed variables to be used as input. In the next step, the embeddings of the category features are concatenated with the number features into a large vector. The vector will be fed into a 3-layer feedforward network and directly connected to the output predictions via a linear skip-connection. In AutoGluon, we adopted 2 neural network model based on PyTorch and Fastai v1 respectively.
Accuracy, a good and intuitive evaluation index, is commonly used to evaluate the predictive performance of the machine learning models. Generally, higher accuracy means better prediction efficiency. And it can be written as:
(TP: True Positive, TN: True Negative, FP: False Positive, FN: False Negative)
Importance can be used to measure the degree to which different indicators contribute to the model. For example, if we delete one indicator and use the remaining indicators to train a model, the model prediction performance will decline. The degree of the decline, which is the magnitude of the decline in accuracy, is the value of the importance. Therefore, the higher the importance, the more important the indicator will be.
In the present study, different models were constructed using the database and randomly divided into training and validation sets at a ratio of 8:2. We also used K-fold cross-validation to validate the predicative performance of the machine learning model. Because K-fold cross-validation is easy to implement and had skill estimation with lower bias than other methods, it is often used to compare and select models for a given predictive modeling problem. The K-fold cross-validation was performed as follows: Divide the original dataset into K groups (the K value is 10 in our model); Select one group as the test dataset and the remaining groups as the train dataset; Fit a model with the train dataset and test the model on the test dataset; Retain the evaluation score (we use accuracy, precision and recall, and accuracy is the main indicator); Repeat the group selection until each group is tested. In summary, K-fold cross-validation averages K rounds of fitness in the prediction to derive the most accurate estimate of the model prediction performance.
A total of 274 participants who underwent PA-TACE were recruited in this study, of which 142(51.8%) patients received once PA-TACE treatment. In the prediction model, twenty-eight predictors including clinicopathological characteristics, inflammatory-based prognostic biomarkers and clinical stages were analyzed. The baseline characteristics of all the participants are shown in Table 1. At the end of follow-up, 137 (50.0%) patients presented HCC recurrence, and 56 (20.4%) patients died. The median follow-up time of the study was 20 (IQR: 9–30) months.
The machine learning models included in our study were normal ML (KNN), Boosting (XGBoost; CatBoost; LightGBM), Bagging (Extra Trees; Random Forest), Stacking, and simple DL (DeepNN; Fastai). The discriminatory performance of the five models in overall mortality and HCC recurrence were assessed with the accuracy. Among the five models, the ensemble learning strategies, including Boosting, Bagging and Stacking, presented better predictive performance in terms of prognostic risk for HCC patients following PA-TACE (Tables 2, 3). Specially, the accuracy of the Stacking model in predicting overall mortality and HCC recurrence (test-accuracy: 0.8909, valid-accuracy: 0.9318; Table 2; test-accuracy: 0.7636, valid-accuracy: 0.8182; Table 3) was at the highest level in both training sets and validation sets. Moreover, the fitting time, prediction time and gain of five machine learning models were also compared, indicating that the Stacking model had relatively low time consumption and the best prediction performance (Tables 2, 3).
In time-dependent ROC analysis, the Boosting, Bagging, and Stacking model were found to perform well in predicting OS (1-year: 0.878, 0.871, 0.907; 2-year: 0.910, 0.919, 0.941; 3-year: 0.946, 0.930, 0.953; Figures 4A–C) and RFS (1-year: 0.784, 0.809, 0.812; 2-year: 0.845, 0.849, 0.847; 3-year: 0.789, 0.822, 0.834; Figures 4D–F) for HCC patients received PA-TACE. Moreover, patients were categorized into low- and high- risk groups based on the median risk score of the Stacking model. The low-risk group had significantly better overall survival and recurrence-free survival than the high-risk group (P<0.001; Supplemental Figures 1A, B). Therefore, KM curves indicated good discriminative ability of the Stacking model.
Figure 4 One-, two-, and three-year time-dependent ROC curves for overall survival (A–C) and recurrence-free survival (D–F) of the Boosting, Bagging and Stacking models.
We also established the Stacking model to examine the variable importance of recurrence and overall mortality of the HCC patients after PA-TACE. The specific prediction performance of each predictor was measured using importance. The variables with the top 10 importance and P values are also shown in Table 4.
Table 4 Variable importance of features included in the Stacking model to predict recurrence and overall mortality of the HCC patients after PA-TACE.
For overall mortality, the importance of BCLC Stage was 0.029341, substantially higher than scores of other variables, such as AFP (0.021429), ALB (0.014286), and frequency of PA-TACE (0.013106). For HCC recurrence, hsCRP/ALB (0.049635) was the most important variable, followed by MVI (0.029197), NLR (0.023358), and Tumor diameter (0.022628). Furthermore, BCLC Stage, hsCRP/ALB and frequency of PA-TACE were found to be relatively important variables in both overall mortality and recurrence, while MVI contributed more to the recurrence of the patients.
According to different cutoff values, continuous variables were converted into binary variables. Univariate Cox regression analyses were used to determine independent prognostic factors for OS and RFS. The results were generally consistent with the above results obtained from the Stacking model (Supplemental Table 1).
To the best of our knowledge, our study was the first to utilize and compare different machine learning algorithms to analyze the RFS and OS outcomes of HCC patients following PA-TACE. Among the five machine learning models, the risk prediction model based on ensemble learning strategies, including Boosting, Bagging, and Stacking algorithms, presented better prediction performance for overall mortality and HCC recurrence. Specially, the fitting time, prediction time and gain of five machine learning models were also compared, indicating that the Stacking model had relatively low time consumption and the best prediction performance. In addition, according to time-dependent ROC analysis, the ensemble learning strategies were found to perform well in predicting both OS and RFS for the patients. In the present study, we also identified the important prognostic factors for postoperative outcomes. We found that BCLC Stage, hsCRP/ALB and frequency of PA-TACE were relatively important variables in both overall mortality and recurrence, while MVI contributed more to the recurrence of the patients.
Nowadays, increasing scoring systems have been developed to evaluate the prognosis of HCC and stratify patients. Most scoring systems have mainly selected significant clinical predictive indices through multivariate analysis, and constructed conventional Cox proportional risk models based on limited risk factors (32–34). However, in clinical studies, various risk factors often have nonlinear effects on recurrence-free survival, especially when they are used in cancer research (35–37). Therefore, the previous traditional models may fail to show the goodness of fitting or make accurate predictions. Machine learning could train algorithms to detect and recognize complex patterns and adapt to more complex nonlinear relationships, thus it might be superior than the traditional models in medical research (25). In our study, machine learning algorithms, including normal machine learning, Boosting, Bagging, Stacking, and simple deep learning were used and compared on RFS and OS outcomes of HCC patients received PA-TACE. The results showed that the Stacking algorithm, an ensemble learning strategy, presented relatively low time consumption, good discriminative ability, and the best prediction performance for the clinical outcomes. Therefore, this ensemble learning model, based on routine peripheral blood cell measurements and clinical characteristics, provides an easily accessible, effective, and intelligent approach for predicting OS and RFS in HCC patients received PA-TACE. Admittedly, the clinical practicability of this model needs further investigation.
In the present study, we also focused on the adaptability of different ensemble learning strategies to clinical data, so that other researchers could better apply specific ensemble learning models to specific clinical data through our comparative experiments. Considering the limited data size and complex data combinations of multiple attributes and dimensions used to predict the prognosis of HCC patients receiving PA-TACE, we therefore developed ensemble learning models including Boosting, Bagging, and Stacking algorithms, rather than sophisticated deep learning strategies. These models do not have excessive overfitting, nor do they have a large number of hyperparameter to learn, which ensures a certain degree of generalization of the model. Moreover, K-fold cross-validation could be used to average K rounds of fitness in the prediction to derive the most accurate estimate of the model prediction performance. In conclusion, we believe that the ensemble learning model developed in our study could also be used to predict the prognosis in subsequent small sample clinical data with multiple attributes and dimensions.
The majority of liver cancers occur in cirrhotic livers with chronic inflammation, which creates a pro-inflammatory environment that promotes tumor formation and development (38–40). The importance of host inflammatory responses indicates the role of inflammatory indices in predicting clinical outcomes of the cancer patients (41). Therefore, apart from the laboratory tests and tumor characteristics, several inflammatory-based prognostic indices (NLR, PLR, SII, SIRI, hsCRP/ALB and PNI) were also included in this study. According to the feature importance analysis based on the Stacking model, interesting outcomes were obtained using these variables. Specifically, BCLC Stage, hsCRP/ALB and frequency of PA-TACE were relatively important variables in predicting both overall mortality and recurrence, while MVI contributed more to the recurrence of the patients. These findings are supported by the following studies.
Firstly, treatment allocation and prognostic stratification based on BCLC staging system, which is closely related to the prognosis of HCC, are currently the most widely used guidelines in clinical practice (42). For HCC patients, those in the early stages of BCLC could obtain good survival and low recurrence prognosis after curative resection (42, 43). In the present study, the predictive performance of the BCLC stage ranked first and fifth in overall mortality and recurrence of patients undergoing PA-TACE, which is in line with the results of previous studies.
Secondly, preoperative hsCRP is considered to be the most sensitive protein synthesized by the liver to detect systemic inflammation and could reflect the burden or development of HCC tumor cells (44, 45). In addition, preoperative albumin is an effective factor to reflect the nutritional status and liver function of the patients, and it is also a decisive factor of tumor cell immune response (46, 47). Studies have also shown that hsCRP/ALB and hsCRP/LYM have a powerful prognostic value for recurrence outcomes in HCC (48, 49). Therefore, for patients receiving PA-TACE, hsCRP/ALB might be a better prognostic indicator than other features, especially in predicting HCC recurrence.
Thirdly, the basic principle of postoperative TACE is to remove tumor cells that may have been shed from the resected tumor mass during hepatectomy and to eliminate small intrahepatic metastases that may not have been detected before or during the operation (50). Therefore, several studies have proved that postoperative adjuvant TACE could improve the prognosis of HCC patients (12, 13, 50). Specifically, the frequency of PA-TACE is also associated with a reduced HCC recurrence rate, improving the long-term prognosis of patients (12). Furthermore, tumor dissemination and spread through microvessels might be one of the reasons for advanced tumor, tumor progression, and poor prognosis (51). Consistent with our results, MVI was also one of the unique parameters in many prognostic models for surgically resected HCC, including Early Recurrence After Surgery for Liver Tumor (ERASL), Singapore Liver Cancer Recurrence (SLICER) and Surgery-Specific Cancer of the Liver Italian Program (SS-CLIP) models (52–54).
This study also has several limitations. First, our model is primarily based on patients from one Chinese center with a limited sample size. It is necessary to validate our findings in further international, multicenter, large-scale studies. Second, the follow-up period is relatively short, long-term outcomes from prospective studies are critical to further extend the performance of our model.
In conclusion, we have utilized and compared models based on different machine learning algorithms and found that the ensemble learning models could better predict the risk of mortality and recurrence in individual HCC patients following PA-TACE. Specially, the Stacking algorithm presents relatively low time consumption, good discriminative ability, and the best predictive performance for clinical outcomes. Machine learning models could also help clinicians identify the important prognostic factors that are clinically useful in individualized patient monitoring and management.
The raw data supporting the conclusions of this article will be made available by the authors, without undue reservation.
The studies involving human participants were reviewed and approved by theHuman Ethics Committee of Sichuan Academy of Medical Sciences and Sichuan Provincial People’s Hospital. All procedures were performed in accordance with the ethical guidelines of the Helsinki Declaration. The participants provided their written informed consent to participate in this study.
YL, ZW and YP were responsible for study conception and design, data acquisition, data analysis and drafting and revision of the manuscript. XH, JS were responsible for study conception and design, data analysis and drafting and revision of the manuscript. ZD, CL, YQ, YY and YS were responsible for data analysis and drafting and revision of the manuscript. All authors contributed to the article and approved the submitted version.
This study was supported by Sichuan Province Science and Technology Support Program (No. 2018HH0062), and Sichuan Province Science and Technology Support Program (No. 2021YFH0187).
The authors declare that the research was conducted in the absence of any commercial or financial relationships that could be construed as a potential conflict of interest.
All claims expressed in this article are solely those of the authors and do not necessarily represent those of their affiliated organizations, or those of the publisher, the editors and the reviewers. Any product that may be evaluated in this article, or claim that may be made by its manufacturer, is not guaranteed or endorsed by the publisher.
The Supplementary Material for this article can be found online at: https://www.frontiersin.org/articles/10.3389/fonc.2023.1169102/full#supplementary-material
1. Sung H, Ferlay J, Siegel RL, Laversanne M, Soerjomataram I, Jemal A, et al. Global cancer statistics 2020: GLOBOCAN estimates of incidence and mortality worldwide for 36 cancers in 185 countries. CA Cancer J Clin (2021) 71:209–49. doi: 10.3322/caac.21660
2. Villanueva A. Hepatocellular carcinoma. N Engl J Med (2019) 380:1450–62. doi: 10.1056/NEJMra1713263
3. Llovet JM, Kelley RK, Villanueva A, Singal AG, Pikarsky E, Roayaie S, et al. Hepatocellular carcinoma. Nat Rev Dis Primers (2021) 7:6. doi: 10.1038/s41572-020-00240-3
4. Torimura T, Iwamoto H. Treatment and the prognosis of hepatocellular carcinoma in Asia. Liver Int (2022) 42:2042–54. doi: 10.1111/liv.15130
5. Gomaa AI, Waked I. Recent advances in multidisciplinary management of hepatocellular carcinoma. World J Hepatol (2015) 7:673–87. doi: 10.4254/wjh.v7.i4.673
6. Bruix J, Llovet JM. Prognostic prediction and treatment strategy in hepatocellular carcinoma. Hepatology (2002) 35:519–24. doi: 10.1053/jhep.2002.32089
7. Bruix J, Gores GJ, Mazzaferro V. Hepatocellular carcinoma: clinical frontiers and perspectives. Gut (2014) 63:844–55. doi: 10.1136/gutjnl-2013-306627
8. Zuo CH, Xia M, Liu JS, Qiu XX, Lei X, Xu RC, et al. Transcatheter arterial chemoembolization combined with interferon-α is safe and effective for patients with hepatocellular carcinoma after curative resection. Asian Pac J Cancer Prev (2015) 16:245–51. doi: 10.7314/APJCP.2015.16.1.245
9. Fukumoto T, Tominaga M, Kido M, Takebe A, Tanaka M, Kuramitsu K, et al. Long-term outcomes and prognostic factors with reductive hepatectomy and sequential percutaneous isolated hepatic perfusion for multiple bilobar hepatocellular carcinoma. Ann Surg Oncol (2014) 21:971–8. doi: 10.1245/s10434-013-3305-y
10. Chen K, Xia Y, Wang H, Xiao F, Xiang G, Shen F. Adjuvant iodine-125 brachytherapy for hepatocellular carcinoma after complete hepatectomy: a randomized controlled trial. PloS One (2013) 8:e57397. doi: 10.1371/journal.pone.0057397
11. Bruix J, Sherman M. Management of hepatocellular carcinoma. Hepatology (2005) 42:1208–36. doi: 10.1002/hep.20933
12. Feng AL, Zhu JK, Yang Y, Wang YD, Liu FY, Zhu M, et al. Repeated postoperative adjuvant TACE after curative hepatectomy improves outcomes of patients with HCC. Minim Invasive Ther Allied Technol (2021) 30:163–8. doi: 10.1080/13645706.2019.1707689
13. Zhong JH, Li LQ. Postoperative adjuvant transarterial chemoembolization for participants with hepatocellular carcinoma: a meta-analysis. Hepatol Res (2010) 40:943–53. doi: 10.1111/j.1872-034X.2010.00710.x
14. Gao Q, Qiu SJ, Fan J, Zhou J, Wang XY, Xiao YS, et al. Intratumoral balance of regulatory and cytotoxic T cells is associated with prognosis of hepatocellular carcinoma after resection. J Clin Oncol (2007) 25:2586–93. doi: 10.1200/JCO.2006.09.4565
15. Dong ZR, Zhang PF, Wang CH, Zhang C, Cai JB, Shi GM, et al. Postoperative adjuvant transcatheter arterial chemoembolization for resectable multiple hepatocellular carcinoma beyond the Milan criteria: a retrospective analysis. Am J Cancer Res (2015) 5:450–7.
16. Peng BG, He Q, Li JP, Zhou F. Adjuvant transcatheter arterial chemoembolization improves efficacy of hepatectomy for patients with hepatocellular carcinoma and portal vein tumor thrombus. Am J Surg (2009) 198:313–8. doi: 10.1016/j.amjsurg.2008.09.026
17. Zhong C, Guo RP, Li JQ, Shi M, Wei W, Chen MS, et al. A randomized controlled trial of hepatectomy with adjuvant transcatheter arterial chemoembolization versus hepatectomy alone for stage III a hepatocellular carcinoma. J Cancer Res Clin Oncol (2009) 135:1437–45. doi: 10.1007/s00432-009-0588-2
18. Yang J, Liang H, Hu K, Xiong Z, Cao M, Zhong Z, et al. The effects of several postoperative adjuvant therapies for hepatocellular carcinoma patients with microvascular invasion after curative resection: a systematic review and meta-analysis. Cancer Cell Int (2021) 21:92. doi: 10.1186/s12935-021-01790-6
19. Xiang X, Lau WY, Wu ZY, Zhao C, Ma YL, Xiang BD, et al. Transarterial chemoembolization versus best supportive care for patients with hepatocellular carcinoma with portal vein tumor thrombus: a multicenter study. Eur J Surg Oncol (2019) 45:1460–7. doi: 10.1016/j.ejso.2019.03.042
20. Camacho DM, Collins KM, Powers RK, Costello JC, Collins JJ. Next-generation machine learning for biological networks. Cell (2018) 173:1581–92. doi: 10.1016/j.cell.2018.05.015
21. Calderaro J, Seraphin TP, Luedde T, Simon TG. Artificial intelligence for the prevention and clinical management of hepatocellular carcinoma. J Hepatol (2022) 76:1348–61. doi: 10.1016/j.jhep.2022.01.014
22. Shi JY, Wang X, Ding GY, Dong Z, Han J, Guan Z, et al. Exploring prognostic indicators in the pathological images of hepatocellular carcinoma based on deep learning. Gut (2021) 70:951–61. doi: 10.1136/gutjnl-2020-320930
23. Chi H, Zhao S, Yang J, Gao X, Peng G, Zhang J, et al. T-Cell exhaustion signatures characterize the immune landscape and predict HCC prognosis via integrating single-cell RNA-seq and bulk RNA-sequencing. Front Immunol (2023) 14:1137025. doi: 10.3389/fimmu.2023.1137025
24. Singal AG, Mukherjee A, Elmunzer BJ, Higgins PD, Lok AS, Zhu J, et al. Machine learning algorithms outperform conventional regression models in predicting development of hepatocellular carcinoma. Am J Gastroenterol (2013) 108:1723–30. doi: 10.1038/ajg.2013.332
25. Huang Y, Chen H, Zeng Y, Liu Z, Ma H, Liu J. Development and validation of a machine learning prognostic model for hepatocellular carcinoma recurrence after surgical resection. Front Oncol (2020) 10:593741. doi: 10.3389/fonc.2020.593741
26. Wang L, Wu M, Zhu C, Li R, Bao S, Yang S, et al. Ensemble learning based on efficient features combination can predict the outcome of recurrence-free survival in patients with hepatocellular carcinoma within three years after surgery. Front Oncol (2022) 12:1019009. doi: 10.3389/fonc.2022.1019009
27. An H, Bhatia I, Cao F, Huang Z, Xie C. CT texture analysis in predicting treatment response and survival in patients with hepatocellular carcinoma treated with transarterial chemoembolization using random forest models. BMC Cancer (2023) 23:201. doi: 10.1186/s12885-023-10620-z
28. Roayaie S, Blume IN, Thung SN, Guido M, Fiel MI, Hiotis S, et al. A system of classifying microvascular invasion to predict outcome after resection in patients with hepatocellular carcinoma. Gastroenterology (2009) 137:850–5. doi: 10.1053/j.gastro.2009.06.003
29. Lin WF, Zhong MF, Zhang YR, Wang H, Zhao HT, Cheng BB, et al. Prognostic role of platelet-to-Lymphocyte ratio in hepatocellular carcinoma with different BCLC stages: a systematic review and meta-analysis. Gastroenterol Res Pract (2018) 2018:5670949. doi: 10.1155/2018/5670949
30. Hu B, Yang XR, Xu Y, Sun YF, Sun C, Guo W, et al. Systemic immune-inflammation index predicts prognosis of patients after curative resection for hepatocellular carcinoma. Clin Cancer Res (2014) 20:6212–22. doi: 10.1158/1078-0432.CCR-14-0442
31. Fan X, Chen G, Li Y, Shi Z, He L, Zhou D, et al. The preoperative prognostic nutritional index in hepatocellular carcinoma after curative hepatectomy: a retrospective cohort study and meta-analysis. J Invest Surg (2021) 34:826–33. doi: 10.1080/08941939.2019.1698679
32. Kamarajah SK, Frankel TL, Sonnenday C, Cho CS, Nathan H. Critical evaluation of the American joint commission on cancer (AJCC) 8th edition staging system for patients with hepatocellular carcinoma (HCC): a surveillance, epidemiology, end results (SEER) analysis. J Surg Oncol (2018) 117:644–50. doi: 10.1002/jso.24908
33. Cho HJ, Kim B, Lee JD, Kang DR, Kim JK, Lee JH, et al. Development of risk prediction model for hepatocellular carcinoma progression of indeterminate nodules in hepatitis b virus-related cirrhotic liver. Am J Gastroenterol (2017) 112:460–70. doi: 10.1038/ajg.2016.480
34. Sasaki K, Firl DJ, Hashimoto K, Fujiki M, Diago-Uso T, Quintini C, et al. Development and validation of the HALT-HCC score to predict mortality in liver transplant recipients with hepatocellular carcinoma: a retrospective cohort analysis. Lancet Gastroenterol Hepatol (2017) 2:595–603. doi: 10.1016/S2468-1253(17)30106-1
35. Katzman JL, Shaham U, Cloninger A, Bates J, Jiang T, Kluger Y. DeepSurv: personalized treatment recommender system using a cox proportional hazards deep neural network. BMC Med Res Methodol (2018) 18:24. doi: 10.1186/s12874-018-0482-1
36. Van Calster B, Wynants L. Machine learning in medicine. N Engl J Med (2019) 380:2588. doi: 10.1056/NEJMc1906060
37. Austin PC, Pencinca MJ, Steyerberg EW. Predictive accuracy of novel risk factors and markers: a simulation study of the sensitivity of different performance measures for the cox proportional hazards regression model. Stat Methods Med Res (2017) 26:1053–77. doi: 10.1177/0962280214567141
39. Roth GS, Decaens T. Liver immunotolerance and hepatocellular carcinoma: patho-physiological mechanisms and therapeutic perspectives. Eur J Cancer (2017) 87:101–12. doi: 10.1016/j.ejca.2017.10.010
40. O'Rourke JM, Sagar VM, Shah T, Shetty S. Carcinogenesis on the background of liver fibrosis: implications for the management of hepatocellular cancer. World J Gastroenterol (2018) 24:4436–47. doi: 10.3748/wjg.v24.i39.4436
41. Qu Z, Lu YJ, Feng JW, Chen YX, Shi LQ, Chen J, et al. Preoperative prognostic nutritional index and neutrophil-to-Lymphocyte ratio predict survival outcomes of patients with hepatocellular carcinoma after curative resection. Front Oncol (2021) 11:823054. doi: 10.3389/fonc.2021.823054
42. Heimbach JK, Kulik LM, Finn RS, Sirlin CB, Abecassis MM, Roberts LR, et al. AASLD guidelines for the treatment of hepatocellular carcinoma. Hepatology (2018) 67:358–80. doi: 10.1002/hep.29086
43. Forner A, Reig M, Bruix J. Hepatocellular carcinoma. Lancet (2018) 391:1301–14. doi: 10.1016/S0140-6736(18)30010-2
44. Ko YJ, Kwon YM, Kim KH, Choi HC, Chun SH, Yoon HJ, et al. High-sensitivity c-reactive protein levels and cancer mortality. Cancer Epidemiol Biomarkers Prev (2012) 21:2076–86. doi: 10.1158/1055-9965.EPI-12-0611
45. Crusz SM, Balkwill FR. Inflammation and cancer: advances and new agents. Nat Rev Clin Oncol (2015) 12:584–96. doi: 10.1038/nrclinonc.2015.105
46. Wang D, Hu X, Xiao L, Long G, Yao L, Wang Z, et al. Prognostic nutritional index and systemic immune-inflammation index predict the prognosis of patients with HCC. J Gastrointest Surg (2021) 25:421–7. doi: 10.1007/s11605-019-04492-7
47. Lu J, Xu BB, Zheng ZF, Xie JW, Wang JB, Lin JX, et al. CRP/prealbumin, a novel inflammatory index for predicting recurrence after radical resection in gastric cancer patients: post hoc analysis of a randomized phase III trial. Gastric Cancer (2019) 22:536–45. doi: 10.1007/s10120-018-0892-0
48. Liao M, Chen P, Liao Y, Li J, Yao W, Sun T, et al. Preoperative high-sensitivity c-reactive protein to lymphocyte ratio index plays a vital role in the prognosis of hepatocellular carcinoma after surgical resection. Onco Targets Ther (2018) 11:5591–600. doi: 10.2147/OTT.S167857
49. Oh TK, Choi YR, Cho JY, Yoon YS, Han HS, Park IS, et al. The high-sensitivity c-reactive Protein/Albumin ratio predicts long-term oncologic outcomes after curative resection for hepatocellular carcinoma. J Clin Med (2018) 7(6):139. doi: 10.3390/jcm7060139
50. Jiang JH, Guo Z, Lu HF, Wang XB, Yang HJ, Yang FQ, et al. Adjuvant transarterial chemoembolization after curative resection of hepatocellular carcinoma: propensity score analysis. World J Gastroenterol (2015) 21:4627–34. doi: 10.3748/wjg.v21.i15.4627
51. Lim KC, Chow PK, Allen JC, Chia GS, Lim M, Cheow PC, et al. Microvascular invasion is a better predictor of tumor recurrence and overall survival following surgical resection for hepatocellular carcinoma compared to the Milan criteria. Ann Surg (2011) 254:108–13. doi: 10.1097/SLA.0b013e31821ad884
52. Beumer BR, Takagi K, Vervoort B, Buettner S, Umeda Y, Yagi T, et al. Prediction of early recurrence after surgery for liver tumor (ERASL): an international validation of the ERASL risk models. Ann Surg Oncol (2021) 28:8211–20. doi: 10.1245/s10434-021-10235-3
53. Ang SF, Ng ES, Li H, Ong YH, Choo SP, Ngeow J, et al. The Singapore liver cancer recurrence (SLICER) score for relapse prediction in patients with surgically resected hepatocellular carcinoma. PloS One (2015) 10:e0118658. doi: 10.1371/journal.pone.0118658
Keywords: machine learning, hepatocellular carcinoma, postoperative adjuvant TACE, recurrence, prognosis
Citation: Liang Y, Wang Z, Peng Y, Dai Z, Lai C, Qiu Y, Yao Y, Shi Y, Shang J and Huang X (2023) Development of ensemble learning models for prognosis of hepatocellular carcinoma patients underwent postoperative adjuvant transarterial chemoembolization. Front. Oncol. 13:1169102. doi: 10.3389/fonc.2023.1169102
Received: 18 February 2023; Accepted: 09 May 2023;
Published: 26 May 2023.
Edited by:
Tommaso Maria Manzia, University of Rome Tor Vergata, ItalyReviewed by:
Xiaofeng Wu, Nanjing Medical University, ChinaCopyright © 2023 Liang, Wang, Peng, Dai, Lai, Qiu, Yao, Shi, Shang and Huang. This is an open-access article distributed under the terms of the Creative Commons Attribution License (CC BY). The use, distribution or reproduction in other forums is permitted, provided the original author(s) and the copyright owner(s) are credited and that the original publication in this journal is cited, in accordance with accepted academic practice. No use, distribution or reproduction is permitted which does not comply with these terms.
*Correspondence: Xiaolun Huang, aHVhbmd4aWFvbHVuQG1lZC51ZXN0Yy5lZHUuY24=; Jin Shang, OTM1NTE2MTY1QHFxLmNvbQ==
†These authors have contributed equally to this work
Disclaimer: All claims expressed in this article are solely those of the authors and do not necessarily represent those of their affiliated organizations, or those of the publisher, the editors and the reviewers. Any product that may be evaluated in this article or claim that may be made by its manufacturer is not guaranteed or endorsed by the publisher.
Research integrity at Frontiers
Learn more about the work of our research integrity team to safeguard the quality of each article we publish.