- 1Medical Big Data and Bioinformatics Research Centre, First Affiliated Hospital of Gannan Medical University, Ganzhou, Jiangxi, China
- 2School of Public Health and Health Management, Gannan Medical University, Ganzhou, Jiangxi, China
- 3School of Health and Medicine, Guangzhou Huashang Vocational College, Guangzhou, Guangdong, China
Historically, the chief focus of lymph node metastasis research has been molecular and clinical studies of a few essential pathways and genes. Recent years have seen a rapid accumulation of massive omics and imaging data catalyzed by the rapid development of advanced technologies. This rapid increase in data has driven improvements in the accuracy of diagnosis of lymph node metastasis, and its analysis further demands new methods and the opportunity to provide novel insights for basic research. In fact, the combination of omics data, imaging data, clinical medicine, and diagnostic methods has led to notable advances in our basic understanding and transformation of lymph node metastases in rectal cancer. Higher levels of integration will require a concerted effort among data scientists and clinicians. Herein, we review the current state and future challenges to advance the diagnosis of lymph node metastases in rectal cancer.
1 Introduction
Lymph node metastasis is complex and its progression involves diverse processes in the patient’s body. Consequently, the cancer research community has generated massive omics and imaging data to study the hallmarks of cancer as comprehensively as possible. The fast accumulation of massive omics and imaging data catalyzed by the rapid development of advanced technologies has driven improvements in the accuracy of diagnosis of lymph node metastasis. The accurate detection of lymph node metastasis and staging is essential and well-acknowledged for making appropriate treatment plans and prognostic predictions for these patients (1). However, clinical staging is subjective and influenced by many factors, an important reason for the high rate of missed diagnosis. Therefore, it is necessary to seek more effective detection methods for identifying lymph node metastasis.
The diagnosis of lymph node metastasis plays an essential role in the field of rectal cancer. First, lymph node metastasis is the main mode of metastasis which occurs in 30–40% of patients with rectal cancer, and the local recurrence rate is very high, which is also closely related to their prognoses (2–4). Second, the treatment of rectal cancer depends on the stage (5). The TNM staging system is the basis of treatment formulation and facilitates the assessment of the prognoses of patients with rectal cancer by physicians (6). Moreover, preoperative evaluation of lymph node metastasis can provide critical information for determining the necessity of neoadjuvant therapy and the adequacy of surgical resection (7–9). However, the current clinical accuracy of N-stage remains unsatisfactory (10).
Lymph node metastasis in rectal cancer has immense scope and it is nearly impossible to cover everything in one review. Therefore, herein, we focus on key diagnostic analyses that have led to conceptual advances in our understanding of cancer biology and impacted decision-making for disease treatment. Further, we detail the reviews in pertaining sections to direct interested readers to relevant resources. We acknowledge that our limited selection of topics and examples may omit important work, for which we sincerely apologize.
In this review, studies were systematically searched in PubMed before December, 2022. The search terms used were ((rectal cancer [MeSH Terms]) AND (diagnosis [MeSH Terms]) AND (lymph node metastasis [MeSH Terms])). The “Similar articles” function was used to broaden the search, and all citations were considered for relevance. A manual search of the references of publications was carried out to ensure that no relevant studies were excluded. We begin by describing conventional imaging examination, including magnetic resonance imaging (MRI), endoscopic ultrasonography (EUS), and positron emission tomography/computed tomography (PET/CT) for the diagnosis of N staging of rectal cancer. Different imaging methods vary in the range of their diagnostic accuracy and their benefits and drawbacks. These examination techniques have certain limitations in determining lymph node metastasis in rectal cancer. Presently, artificial intelligence, multidisciplinary team (MDT), biomarkers, and metagenomics methods have simultaneously increased the accuracy of determining lymph node metastasis in rectal cancer. Finally, we discuss current challenges and the future direction of the field. The overall framework of this paper is shown in Figure 1. This review consists solely of a succinct assessment of the development in research on the diagnosis of lymph node metastases in rectal cancer.
2 Traditional imaging examination-based assessment of lymph node metastases in rectal cancer
2.1 MRI
MRI plays a major role in assessing the staging of rectal cancer. It can indicate the precise anatomy of the rectum and mesenteric fascia, and accurately predict the margin of circumferential resection and tumor staging. The guidelines of the European Society of Gastrointestinal and Abdominal Radiology recommend MRI for diagnosing the staging of rectal cancer (11). Its better spatial resolution is advantageous in distinguishing benign from malignant lymph nodes. In the early stage of diagnosis of lymph nodes, their size is used as a diagnostic criterion but the utility of the boundary value of lymph node diameter remains inconclusive. Due to the lack of unified standards, the accuracy of determining the nature of tumor lymph nodes is not ideal, and different studies have the problem of over- or under-staging (12–14). MRI cannot completely determine the status of lymph nodes and the criteria used to determine lymph node metastasis may be different in different institutions (15). The accuracy of the results of MRI to detect lymph node metastasis is shown in Table 1. As shown, the results of different studies vary greatly and are not very accurate overall. Subsequently, the criteria of MRI for the detection of lymph node status should be unified to assist in accurate and individualized treatment of rectal cancer.
2.2 EUS
EUS examination is also a method commonly used for patients with rectal cancer. For the staging of superficial tumors, EUS is preferred (35). As the depth of invasion increases, the penetration of ultrasound to the tumor decreases, thereby decreasing the diagnostic accuracy. A systematic study (36) showed that the sensitivity and specificity of EUS in the diagnosis of lymph node involvement were approximately 0.81 (95%CI, 0.71-0.89) and 0.88 (95%CI, 0.80-0.94), respectively. A meta-analysis (37) for evaluating lymph node metastasis included data from 123 studies that showed that the sensitivity and specificity of EUS were 0.57 and 0.80, respectively. However, its ability to detect lymph nodes is limited by the difficulty in detecting smaller nodes or those closest to the tumor. Tombazzi CR and colleagues (38) have shown that EUS is very accurate for tumor staging of early rectal cancer. However, in fact, as it is difficult to distinguish between inflammatory and metastatic lymph nodes, the judgment of lymph node metastasis by EUS is not accurate, leading to misdiagnosis and possible overtreatment. The scope of EUS is also restricted, which excludes complete exploration of the pelvic cavity or iliac fossa. These limitations weaken its effectiveness in evaluating the staging of lymph nodes. In summary, EUS is not an ideal method for diagnosing lymph node metastasis in rectal cancer.
2.3 PET/CT
PET/CT imaging allows better observation of the health of the entire human body and the study of its anatomical structure and physiological function. It is often used in the clinical detection of early tumors, metastatic lesions, and lymph nodes qualitatively. 18F-FDG PET/CT is the most commonly used PET/CT imaging technique, which is a non-invasive examination method that combines morphological and functional information to diagnose tumors, perform differential diagnosis, assess clinical staging, curative effect monitoring, and determination of radiotherapy plan, bearing great significance (39, 40). 18F-FDG PET/CT imaging is an important imaging tool to evaluate patients’ conditions before surgery (41). This imaging technology can be utilized to assess the N staging of rectal cancer and provide accurate clinical staging and preoperative evaluation (42). A previous study (43) showed that when the cut-off value of the maximum standardized uptake of the lateral pelvic lymph nodes was set to 1.5, it was reasonable in predicting the risk of lateral pelvic lymph node metastasis in patients with rectal cancer. The sensitivity, specificity, positive predictive value, negative predictive value, and false negative value reached 82%, 93%, 58%, 98%, and 18%, respectively. Although the PET/CT technique can provide an accurate diagnosis of lymph node metastases in rectal cancer, it has certain limitations and cannot fully reflect the internal structure of lymph nodes, making accurate diagnoses difficult (44). Further, PET findings of lymph nodes with high FDG uptake levels may indicate that lymph nodes have metastasized, however, this condition is often misidentified as lymph node metastasis due to certain vascular structures (including venous femoral veins or inflammatory lymph nodes) (45). Taken together, future research should focus on improving the display of the internal structure of the lymph nodes, combined with the consequences of PET/CT to improve the accuracy of the diagnosis of lymph node metastasis in rectal cancer.
3 Artificial intelligence to evaluate lymph node metastases in rectal cancer
3.1 Conventional radiomics
The conventional radiomics workflow is typically based on extracting meaningful information from an area being studied. In recent years, radiomics has been used to evaluate several tumors and is increasingly being applied clinically to improve the accuracy of diagnosis, prognosis, and prediction of cancer. The results of the research of Huang YQ et al. (46) showed that the accuracy of detecting lymph node metastasis in rectal cancer could be improved by constructing a radiomic nomogram with radiomic characteristics, CT-reported lymph node status, and clinical risk factors. The distinguishing ability, evidenced by the area under the curve (AUC) was 0.778 (95%CI, 0.769-0.787), indicative of a good practical application value. Similarly, a retrospective study (47) showed that features of radiomics combined with a nomogram comprising a random score of 3, age, and lymph node size showed good discrimination, and the AUC value reached 0.884, suggestive of its high accuracy in the prediction of preoperative lymph node metastasis in patients with rectal cancer. Recently, Chen LD et al. (48) developed a multi-parameter nomogram, which could more accurately predict lymph node metastasis. This model organically integrated CT and S-wave elastic imaging techniques and had a high C-index of 0.857 (95% CI, 0.726–0.989). All the above studies showed good predictive efficacy for metastatic lymph nodes of rectal cancer but the models are different owing to the equipment type and parameters, making it difficult to evaluate an optimal model. The clinical application and promotion of imaging big data are needed to establish unified standards and multi-center data support.
3.2 Deep learning
The application of computer technology to the medical field has been a major breakthrough, allowing clinicians to make more accurate diagnoses and treatment plans. With the application of computer technology in the diagnosis of lymph node metastasis, the incidence of rectal cancer has reduced significantly in China and greatly improved the accuracy of its diagnosis, treatment, evaluation, and prediction (49). Because it is difficult to obtain massive data from medical images sometimes, transfer learning can be used. Transfer learning is a type of deep learning that uses pre-trained models and requires fewer medical images. This method first uses pre-trained weights from similar architecture networks to initialize the network, and then fine-tunes the parameters to suit the target application, with the last fully connected layer usually replaced by neurons of a new class based on the number of classes in the new classification task. Ichimasa K et al. (50) retrospectively analyzed the data of 690 consecutive T1 colorectal cancer patients and established intelligent model Mo1724, wherein 45 clinicopathological factors were analyzed to predict positive or negative lymph node metastases. The results suggested that the sensitivity of the model was 100% (95% CI, 72%–100%), the specificity was 66% (95% CI, 56%–76%), and the accuracy rate was 69% (95%CI, 59%–78%). Compared to the current guidelines, the AI model significantly reduces the number of missed cases of positive lymph node metastases. On this basis, Kudo SE et al. (51) used an artificial neural network to conduct in-depth studies on patients with lymph node metastasis according to their age, sex, tumor size, location, morphology, lymphatic and vascular infiltration, and histological grade. The results showed that in the verification cohort, the AUC value of patients with lymph node metastasis identified by the model was as high as 0.83, while that of patients with lymph node metastasis identified by following the guidelines was only 0.73 (P<0.001). After analysis, limited to patients initially undergoing endoscopic resection, the model showed that the AUC for patients with lymph node metastasis was still as high as 0.84, while the corresponding value with the guidelines was 0.77 (P=0.005). The model can be used to identify the need for additional lymph node dissection after endoscopic resection in patients with T1 colorectal cancer. The development of artificial intelligence technology has a useful guiding role in the diagnosis, treatment, and prognosis of patients with rectal cancer lymph node metastasis. Li J (52) used deep transfer learning to classify the lymph node status of patients with rectal cancer in an attempt to improve the accuracy of N staging. The positive predictive value, negative predictive value, sensitivity, and specificity of this model were 95.2%, 95.3%, 95.3%, and 95.2%, respectively. The AUC and accuracy were 0.994 and 95.7%, respectively. Following deep learning for metastasis evaluation by MRI examination, the lymph node metastasis rate for rectal cancer patients increased significantly, substantially greater than that for the traditional manual examination method. Similarly, a systematic review (53) showed that radiologists and deep learning models had AUCs of 0.688 (0.603–0.772) and 0.917 (0.882–0.952), respectively. The performance of the deep learning model was better than that of the radiologists, and the artificial intelligence model may more accurately predict the lymph node metastasis of rectal cancer. However, existing studies on the application of deep learning in the diagnosis of rectal cancer lymph node metastasis are sparse, and the findings warrant further investigation.
In recent years, with the development of computer technology, the development of medical imaging analysis methods has been considerably promoted. Methods like conventional radiomics and deep learning have gradually been applied to medical imaging analysis. The accuracy of the results of deep learning and radiomics models to detect lymph node metastasis is shown in Table 2.
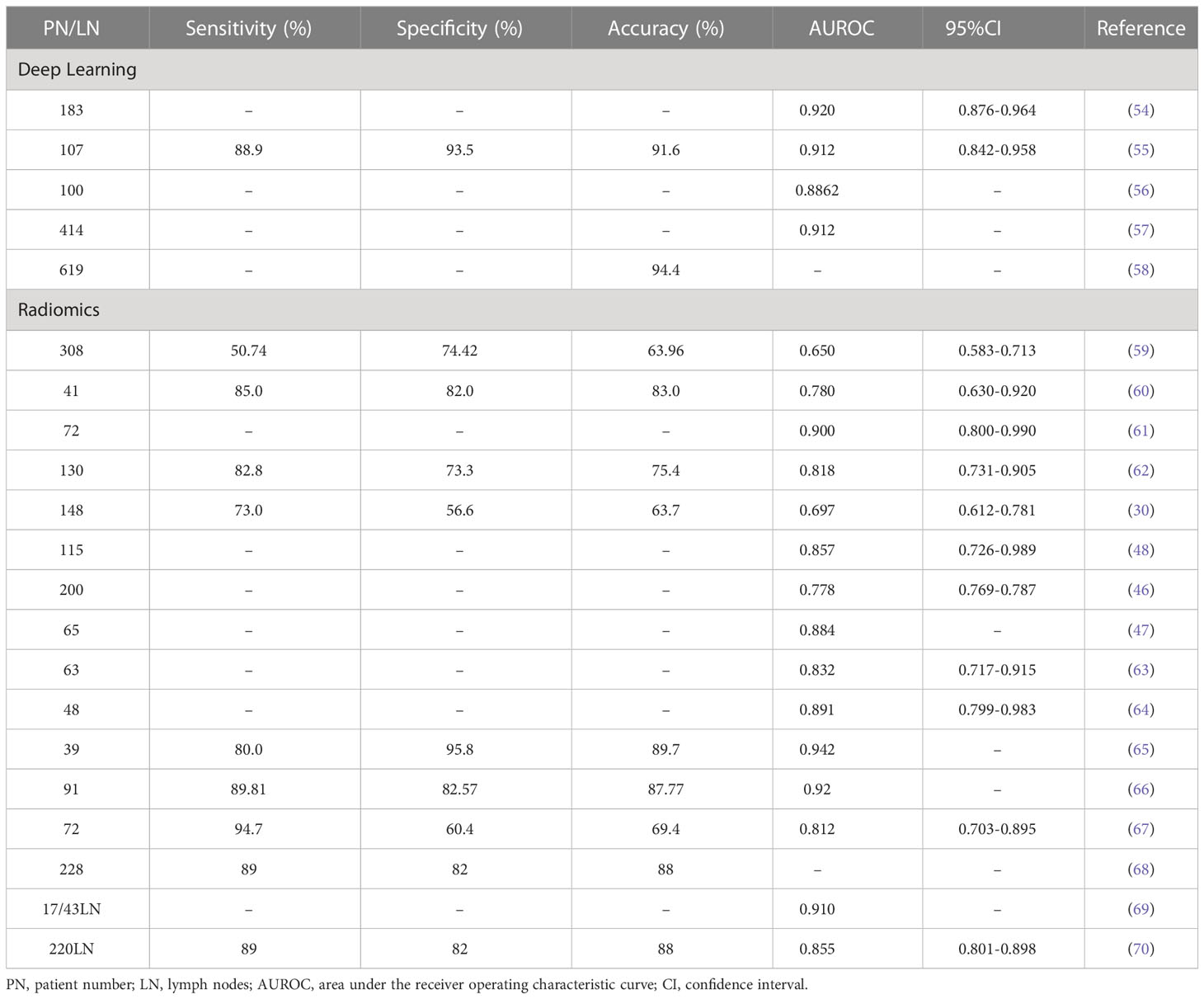
Table 2 Accuracy of results of deep learning and conventional radiomics models to detect lymph node metastasis.
The Table 3 shows the advantages and disadvantages of traditional imaging versus artificial intelligence. Conventional imaging generally relies on human experience and judgement, whereas artificial intelligence uses predictive algorithms to provide rapid results with high accuracy. Conventional imaging has established costs, while the costs for artificial intelligence systems tend to become more cost-effective over time. Artificial intelligence systems surpass conventional imaging by providing standardized results but may lack the personal touch brought by human interaction.
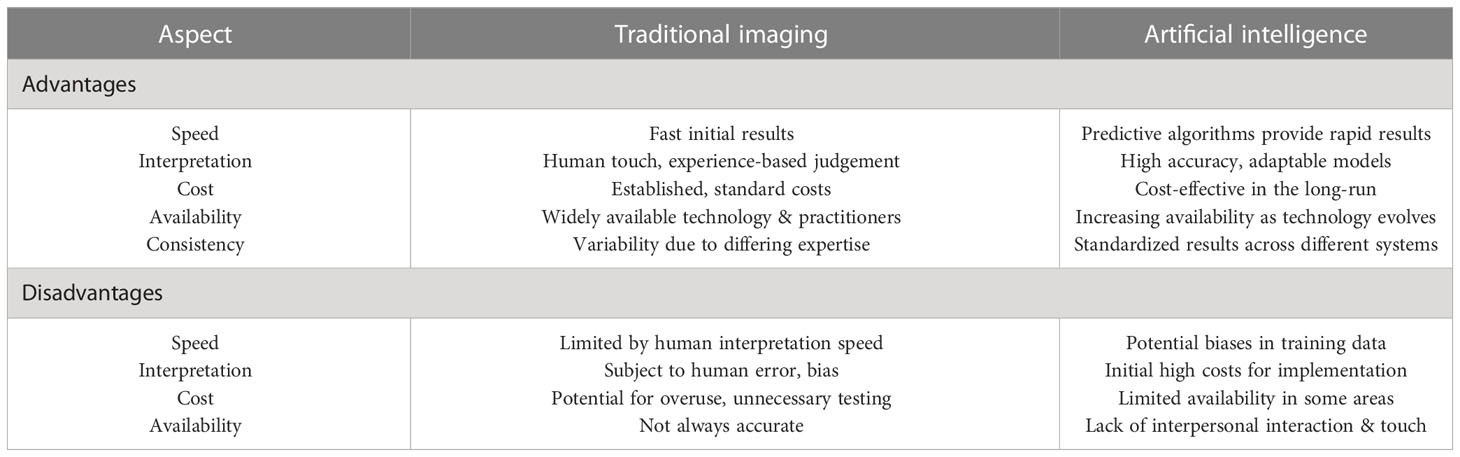
Table 3 Comparing the advantages and disadvantages of traditional imaging and artificial intelligence.
4 Evaluation of lymph node metastasis in rectal cancer by MDT
Preoperative MDT evaluation is linked to the improvement in the long-term survival rate of patients with locally advanced rectal cancer. Owing to the development in assessing preoperative radioactive tumor staging and neoadjuvant therapy, multidisciplinary group discussions have been introduced. A systematic review study (71) showed that the basis of modern treatment strategies is accurate high-resolution imaging to guide neoadjuvant therapy and precision surgery, followed by detailed pathological examinations to determine important prognostic factors for adjuvant chemotherapy. However, these approaches rely on the close cooperation of interrelated disciplines within an MDT and this multidisciplinary forum is becoming the standard for rectal cancer treatment in the United Kingdom, Europe, and the United States. The fundamental components of modern rectal cancer management are evaluated by the MDT to provide colorectal surgeons with the information they need to guide the patient for the best care. Yu L et al. (72) examined the accuracy of MRI evaluation in diagnosing preoperative staging of rectal cancer by an MDT. The results of the study showed that the accuracy of MRI in diagnosing the N stage of patients by an MDT before surgery was significantly higher than that of the non-MDT group (56.2% vs. 42.1%, P=0.021). For patients without lymph node metastasis, the accuracy of MRI by an MDT was higher (61.2% vs. 37.8%, P=0.009). Thus, MDT assessment improves the accuracy of MRI in the preoperative staging diagnosis of rectal cancer. Based on MDT, the clinical stage of patients can be more identified accurately, which is conducive to choosing better treatment strategies.
5 Biomarker-based evaluation of lymph node metastasis in rectal cancer
Biomarkers are molecular models that can be used as a tool for the early detection and personalized treatment of colorectal cancer. These can be classified as diagnostic, prognostic, or predictive. Biomarkers are useful in determining disease progression and recurrence at different stages of the disease, and provide personalized indicators of treatment effects. Quantitative analysis of PVT1 expression in tumors and adjacent normal tissues of 210 patients with colorectal cancer showed that its expression related to tumor differentiation, invasion, high grade, and lymph node spread increased by 51.4% (73). However, not all colorectal cancer cell lines exhibit aggressive behavior which is attributable to the expression of PVT1. The HCT116 colorectal cancer cell line did not show more aggressiveness than the control cell line (74). Gharib et al. studied PVT1 expression as a biomarker of lymph node metastasis but found that when it was used instead as part of a biomarker group including PVT1, HOTTIP, and UCA1, the AUC was higher compared to its expression alone (75). In another study, six EMT-related biomarkers (E-cadherin, N-cadherin, cytoplasmic b-catenin, nuclear b-catenin, Snail, and Twist) and two clinicopathological variables were selected to devise an SVM model, and the sensitivity, specificity and overall accuracy of the model in predicting lymph node metastasis were 68.3%, 81.1%, and 72.3%, respectively (76). Moreover, microRNA−129 (miR-129) is reportedly involved in the metastasis of various malignant tumors, and experimental results show that miR-129 may act as a tumor suppressor in colorectal cancer by inhibiting proliferation, migration, invasion, and EMT of these cells (77). Therefore, future studies can explore the role of miR-129 as a biomarker for the diagnosis and prediction of colorectal cancer. The accuracy of biomarkers to detect lymph node metastasis is shown in Table 4. At present, the molecular differences between rectal cancer and colon cancer are gradually becoming apparent, and future research should strictly distinguish the biomarkers for predicting rectal cancer and colon cancer.
6 Metagenomics evaluation of lymph node metastasis in rectal cancer
In recent years, with the deep exploration of gut microbes, several studies have found that the occurrence and development of colorectal cancer are closely related to the changes in the gut microbiota (83, 84). Advanced metagenomics technologies can detect more potentially pathogenic microbiomes, and metagenome-wide association studies have enabled high-resolution associations between the human microbiome and colorectal cancer (85). The intestinal microbiota can be used as a non-invasive predictive disease biomarker for the occurrence and development of colorectal cancer. Yu (86) performed a metagenome-wide association study on fecal samples from 74 colorectal cancer patients and 54 controls from China and confirmed the association of Fusobacterium nucleatum (Fn) with colorectal cancer in 16 patients and 24 controls from Denmark. Moreover, in the French and Austrian cohorts, four genes were found to distinguish colorectal cancer metagenome from the control group with an AUC of 0.72 and 0.77, respectively, and qPCR testing of two genes, enriched in the early cases, accurately classified colorectal cancer patients in the independent Chinese cohort (AUC=0.84, OR=23). This demonstrates the potential of metagenomics of fecal microbiota as a marker for the diagnosis of early colorectal cancer. In another study, a meta-analysis of 1042 fecal metagenomic samples from seven publicly available studies yielded a better predictor of colorectal cancer based on a functional analysis-based interpretable machine learning approach, which distinguished adenoma samples (87). This approach is promising to prevent colorectal cancer by detecting it in the early stages, making the treatment easier and effective.
Studies on Fn and colorectal cancer show an inseparable relationship with the latter’s occurrence and development, including the research from European molecular biology laboratories on colorectal cancer specimens from eight geographically different regions using fecal shotgun metagenomics meta-analysis. Fn in colorectal cancer metagenomes (false discovery rate (FDR) <1 × 10 ^ (–5)) provides important clues for a better understanding of the relationship between the two (88). Another study, which analyzed 969 stool samples from colorectal cancer patients and healthy controls using metagenomic sequencing analysis, showed that the intestinal tracts of colorectal cancer patients had abundant Fn and the composition of these bacteria was characterized for different data sets, even without model training. It maintained high accuracy (AUC=0.84) (89). Castellin et al. (90) performed metagenomic analysis to compare the genomic DNA of colorectal cancer and normal tissues, and found that the number of Fn in the former group was 415 times higher compared to the surrounding tissues. Therefore, we boldly speculate that the high probability of the occurrence and development of colorectal cancer is related to the enrichment of Fn. More studies and data are needed to support these results, and greater challenges for future in-depth research remain.
Lymph node metastasis and distant metastasis usually occur with the progression of colorectal cancer. Yan et al. (91) have discussed the role of Fn in colorectal cancer metastasis. In a retrospective cohort study, they found that the feces of patients with metastatic colorectal cancer contained a large number of Fn. There was a strong association between the number of bacteria and tumor invasion, lymph node metastasis, and distant metastasis. In another study, Mauro Castellarin (92) performed RNA-sequencing to screen colorectal cancer and match normal tissue samples. The host sequence was subtracted. Fn sequences were significantly overexpressed in tumor samples relative to the controls. qPCR analysis of 99 subjects validated excess Fusobacterium sequences in tumors versus matched normal control tissues (p = 2.5 × 10 ^ (–6)). A significant positive association was found between Fusobacterium and lymph node metastasis. With the development of fecal microbial metagenomic analysis techniques, major breakthroughs have been made in the prediction of lymph node metastasis of colorectal cancer. Although relatively few studies have been conducted, this is a new opportunity to study whether bacteria such as the abundance of Fn can accurately diagnose colorectal cancer, bringing more possibilities for future scientific research.
7 Challenges and future perspectives
While many advances in diagnostic techniques are encouraging and impressive, considerable challenges remain in their application for detecting lymph node metastases in rectal cancer. Accurate diagnosis of lymph node metastasis in rectal cancer requires the use of bioomics technology or imaging to collect data based on computer-based biological analysis, and computational biological analysis needs to be driven by medical data. Therefore, data standardization and model generalization are key factors for the success or failure of the diagnosis of lymph node metastasis in rectal cancer. We discuss these in the following subsections.
7.1 Data standardization
At present, there is a general lack of high-quality data for standardization. The existing data sets have various standards, large systematic deviations, and lack unified understanding of diseases. The lack of a unified and clear standardized description of data leads to interaction barriers between machine learning and actual data, and the machine misunderstands the true meaning of data. Therefore, it is urgent to solve the technical problem of medical data standardization. Establishing a standardized database can improve the quality of medical data, maximize the value of scientific data, assist the construction of models, and apply them to the field of clinical diagnosis of diseases (93).
7.2 Model generalization
The generalization of models is critical to their clinical application (94). Simply put, the generalization of the model is mainly reflected in its reproducibility (95), indicative of the performance of the prediction model for similarly-distributed data. However, the actual situation is that most models have excellent performance in training data but do show stable performance in internal and external independent verification, that is, the model’s generalization is poor. There are many possible reasons but the main one is the small sample size of the data. Therefore, the generalization of the model can be improved by increasing the sample size of the data. Therefore, multi-center research is the future direction in the field. By combining multi-center data, the problem of sample size can be solved, the reproducibility can be improved, and the generalization of the model can be enhanced.
8 Conclusion
Although conventional imaging methods for rectal cancer have certain limitations for judging lymph node metastasis in rectal cancer, medical imaging analysis based on computer technology has achieved unprecedented development in recent years. Moreover, its ability for image recognition and natural language extraction has undergone continuous improvement and is expected to play an increasingly important role in the diagnosis and treatment of rectal cancer. The MDT model combines opinions from multidisciplinary experts to provide more accurate clinical information and guide better clinical decision-making. Biomarkers are useful for determining disease progression and recurrence at various stages of the disease, and provide personalized indicators of treatment efficacy, thereby improving clinical diagnosis accuracy, and providing better and more powerful information for planning treatment. Metagenomic studies of fecal microbes may improve the accuracy of predicting early rectal cancer and, in the future, may predict lymph node metastasis in rectal cancer at the microbe level. In recent years, owing to the rapid development of imaging and omics technologies, researchers have used high-throughput methods and computer techniques to mine a large amount of information from these data. We believe that by integrating artificial intelligence technology and imaging or omics data to build an efficient and generalized disease diagnosis model, accurate diagnosis and evaluation of lymph node metastasis in rectal cancer is possible, along with the provision of better treatment for patients, and ultimately help improve patients’ prognoses and quality of life. With the joint efforts of data scientists and clinicians at home and abroad, the development prospect of diagnosis of lymph node metastasis in rectal cancer has bright prospects.
Author contributions
WP, writing—original draft. YG, project administration, revised, and edited manuscript. HQ and LM, supervision. All authors contributed to the article and approved the submitted version.
Funding
This work was supported by the National Natural Science Foundation of China (NSFC, 82060618), the Key Research and Development Program of Jiangxi Province (20203BBGL73184), Doctoral Fund of First Affiliated Hospital of Gannan Medical University.
Conflict of interest
The authors declare that the research was conducted in the absence of any commercial or financial relationships that could be construed as a potential conflict of interest.
Publisher’s note
All claims expressed in this article are solely those of the authors and do not necessarily represent those of their affiliated organizations, or those of the publisher, the editors and the reviewers. Any product that may be evaluated in this article, or claim that may be made by its manufacturer, is not guaranteed or endorsed by the publisher.
References
1. Benson AB, Venook AP, Al-Hawary MM, Cederquist L, Chen YJ, Ciombor KK, et al. Rectal cancer, version 2.2018, NCCN clinical practice guidelines in oncology. J Natl Compr Canc Netw (2018) 16(7):874–901. doi: 10.6004/jnccn.2018.0061
2. Jin M, Frankel WL. Lymph node metastasis in colorectal cancer. Surg Oncol Clin N Am (2018) 27(2):401–12. doi: 10.1016/j.soc.2017.11.011
3. Nagtegaal ID, Knijn N, Hugen N, Marshall HC, Sugihara K, Tot T, et al. Tumor deposits in colorectal cancer: improving the value of modern staging-a systematic review and meta- analysis. J Clin Oncol (2017) 5(10):1119–27. doi: 10.1200/JCO.2016.68.9091
4. Yagi R, Shimada Y, Kameyama H, Tajima Y, Okamura T, Sakata J, et al. Clinical significance of extramural tumor deposits in the lateral pelvic lymph node area in low rectal cancer: a retrospective study at two institutions. Ann Surg Oncol (2016) 23(Suppl 4):552–8. doi: 10.1245/s10434-016-5379-9
5. Benson AB, Venook AP, Al-Hawary MM, Azad N, Chen YJ, Ciombor KK, et al. Rectal cancer, version 2.2022, NCCN clinical practice guidelines in oncology. J Natl Compr Canc Netw (2022) 20(10):1139–67. doi: 10.6004/jnccn.2022.0051
6. Lugli A, Karamitopoulou E, Zlobec I. Tumour budding: a promising parameter in colorectal cancer. Br J Cancer (2012) 106(11):1713–7. doi: 10.1038/bjc.2012.127
7. Glynne-Jones R, Wyrwicz L, Tiret E, Brown G, Rödel C, Cervantes A, et al. Rectal cancer: ESMO clinical practice guidelines for diagnosis, treatment and follow-up. Ann Oncol (2017) 28(suppl_4):iv22–40. doi: 10.1093/annonc/mdx224
8. Attaallah W, Gunal O, Manukyan M, Ozden G, Yegen C. Prognostic impact of the metastatic lymph node ratio on survival in rectal cancer. Ann Coloproctol (2013) 29(3):100–5. doi: 10.3393/ac.2013.29.3.100
9. Beets GL, Figueiredo NF, Beets-Tan RG. Management of rectal cancer without radical resection. Annu Rev Med (2017) 68:169–82. doi: 10.1146/annurev-med-062915-021419
10. Ishida H, Hatano S, Ishiguro T, Kumamoto K, Ishibashi K, Haga N. Prediction of lateral lymph node metastasis in lower rectal cancer: analysis of paraffin-embedded sections. Jpn J Clin Oncol (2012) 42(6):485–90. doi: 10.1093/jjco/hys041
11. Beets-Tan RGH, Lambregts DMJ, Maas M, Bipat S, Barbaro B, Curvo-Semedo L, et al. Magnetic resonance imaging for clinical management of rectal cancer: updated recommendations from the 2016 European society of gastrointestinal and abdominal radiology (ESGAR) consensus meeting. Eur Radiol (2018) 28(4):1465–75. doi: 10.1007/s00330-017-5026-2
12. Nougaret S, Reinhold C, Mikhael HW, Rouanet P, Bibeau F, Brown G. The use of MR imaging in treatment planning for patients with rectal carcinoma: have you checked the "DISTANCE"? Radiology (2013) 268(2):330–44. doi: 10.1148/radiol
13. Wang FL, Wan DS, Lu ZH, Fang YJ, Li LR, Chen G, et al. [Expression of molecular markers detected by immunohistochemistry and risk of lymph node metastasis in stage T1 and T2 colorecrectal cancers]. Chinese (2013) 35(4):277–81. doi: 10.3760/cma.j.issn
14. Zheng W, Zhang L, Wu YL, Zhang HZ. [The association of metastasis-related indexes of lymph nodes and the prognosis of stage N2b colorectal cancer patients]. Chinese (2018) 40(9):679–83. doi: 10.3760/cma.j.issn
15. Moreno CC, Sullivan PS, Mittal PK. MRI Evaluation of rectal cancer: staging and restaging. Curr Probl Diagn Radiol (2017) 46(3):234–41. doi: 10.1067/j.cpradiol.2016.11.011
16. Xu L, Zhang Z, Qin Q, Zhang C, Sun X. Assessment of T and n staging with MRI(3)T in lower and middle rectal cancer and impact on clinical strategy. J Int Med Res (2020) 48(6):300060520928685. doi: 10.1177/0300060520928685
17. Xu H, Zhao W, Guo W, Cao S, Gao C, Song T, et al. Prediction model combining clinical and MR data for diagnosis of lymph node metastasis in patients with rectal cancer. J Magn Reson Imaging (2021) 53(3):874–83. doi: 10.1002/jmri.27369
18. Tersteeg JJC, Crolla RMPH, Gobardhan PD, Kint PAM, Niers-Stobbe I, Boonman-de Winter L, et al. MRI-Based guidelines for selective neoadjuvant treatment in rectal cancer: does MRI adequately predict the indication for radiotherapy in daily practice in a large teaching hospital. Eur J Cancer Care (Engl) (2020) 29(2):e13190. doi: 10.1111/ecc.13190
19. White R, Ung KA, Mathlum M. Accuracy of magnetic resonance imaging in the pre-operative staging of rectal adenocarcinoma: experience from a regional Australian cancer center. Asia Pac J Clin Oncol (2013) 9(4):318–23. doi: 10.1111/ajco.12033
20. Kim DJ, Kim JH, Ryu YH, Jeon TJ, Yu JS, Chung JJ. Nodal staging of rectal cancer: high-resolution pelvic MRI versus 18F-FDGPET/CT[J]. J Comput Assist Tomogr (2011) 35(5):531–4. doi: 10.1097/RCT.0b013e318225720f
21. Fernández-Esparrach G, Ayuso-Colella JR, Sendino O, Pagés M, Cuatrecasas M, Pellisé M, et al. EUS and magnetic resonance imaging in the staging of rectal cancer: a prospective and comparative study. Gastrointest Endosc (2011) 74(2):347–54. doi: 10.1016/j.gie.2011.03.1257
22. Tatli S, Mortele KJ, Breen EL, Bleday R, Silverman SG. Local staging of rectal cancer using combined pelvic phased-array and endorectal coil MRI. J Magn Reson Imaging (2006) 23(4):534–40. doi: 10.1002/jmri.20533
23. Matsuoka H, Nakamura A, Masaki T, Sugiyama M, Takahara T, Hachiya J, et al. A prospective comparison between multidetector-row computed tomography and magnetic resonance imaging in the preoperative evaluation of rectal carcinoma. Am J Surg (2003) 185(6):556–9. doi: 10.1016/s0002-9610(03)00067-9
24. Kim NK, Kim MJ, Park JK, Park SI, Min JS. Preoperative staging of rectal cancer with MRI: accuracy and clinical usefulness. Ann Surg Oncol (2000) 7(10):732–7. doi: 10.1007/s10434-000-0732-3
25. Algebally AM, Mohey N, Szmigielski W, Yousef RR, Kohla S. The value of high-resolution MRI technique in patients with rectal carcinoma: pre-operative assessment of mesorectal fascia involvement, circumferential resection margin and local staging. Pol J Radiol (2015) 80:115–21. doi: 10.12659/PJR.892583
26. Armbruster M, D'Anastasi M, Holzner V, Kreis ME, Dietrich O, Brandlhuber B, et al. Improved detection of a tumorous involvement of the mesorectal fascia and locoregional lymph nodes in locally advanced rectal cancer using DCE-MRI. Int J Colorectal Dis (2018) 33(7):901–9. doi: 10.1007/s00384-018-3083-x
27. Bogach J, Tsai S, Zbuk K, Wong R, Grubac V, Coates A, et al. Quality of preoperative pelvic computed tomography (CT) and magnetic resonance imaging (MRI) for rectal cancer in a region in Ontario: a retrospective population-based study. J Surg Oncol (2018) 117(5):1038–42. doi: 10.1002/jso.25000
28. Gröne J, Loch FN, Taupitz M, Schmidt C, Kreis ME. Accuracy of various lymph node staging criteria in rectal cancer with magnetic resonance imaging. J Gastrointest Surg (2018) 22(1):146–53. doi: 10.1007/s11605-017-3568-x
29. Xian MF, Zheng X, Xu JB, Li X, Chen LD, Wang W. Prediction of lymph node metastasis in rectal cancer: comparison between shear-wave elastography based ultrasomics and MRI. Diagn Interv Radiol (2021) 27(3):424–31. doi: 10.5152/dir.2021.20031
30. Meng X, Xia W, Xie P, Zhang R, Li W, Wang M, et al. Preoperative radiomic signature based on multiparametric magnetic resonance imaging for noninvasive evaluation of biological characteristics in rectal cancer. Eur Radiol (2019) 29(6):3200–9. doi: 10.1007/s00330-018-5763-x
31. Liu Y, Wan LJ, Zhang HM, Peng WJ, Zou SM, Ouyang H, et al. [MRI associated biomarker analysis for diagnosis of lymph node metastasis in T1-2 stage rectal cancer]. Zhonghua Zhong Liu Za Zhi (2021) 43(2):207–12. doi: 10.3760/cma.j.cn112152-20200429-00391
32. Park JS, Jang YJ, Choi GS, Park SY, Kim HJ, Kang H, et al. Accuracy of preoperative MRI in predicting pathology stage in rectal cancers: node-for-node matched histopathology validation of MRI features. Dis Colon Rectum (2014) 57(1):32–8. doi: 10.1097/DCR.0000000000000004
33. Koh DM, George C, Temple L, Collins DJ, Toomey P, Raja A, et al. Diagnostic accuracy of nodal enhancement pattern of rectal cancer at MRI enhanced with ultrasmall superparamagnetic iron oxide: findings in pathologically matched mesorectal lymph nodes. AJR Am J Roentgenol (2010) 194(6):W505–513. doi: 10.2214/AJR.08.1819
34. Kim CK, Kim SH, Chun HK, Lee WY, Yun SH, Song SY, et al. Preoperative staging of rectal cancer: accuracy of 3-Tesla magnetic resonance imaging. Eur Radiol (2006) 16(5):972–80. doi: 10.1007/s00330-005-0084-2
35. Boot J, Gomez-Munoz F, Beets-Tan RGH. Imaging of rectal cancer. Radiologe (2019) 59(Suppl 1):46–50. doi: 10.1007/s00117-019-0579-5
36. Chan BPH, Patel R, Mbuagbaw L, Thabane L, Yaghoobi M. EUS versus magnetic resonance imaging in staging rectal adenocarcinoma: a diagnostic test accuracy meta-analysis. Gastrointest Endosc (2019) 90(2):196–203.e191. doi: 10.1016/j.gie.2019.04.217
37. Li XT, Sun YS, Tang L, Cao K, Zhang XY. Evaluating local lymph node metastasis with magnetic resonance imaging, endoluminal ultrasound and computed tomography in rectal cancer: a meta-analysis. Colorectal Dis (2015) 17(6):O129–135. doi: 10.1111/codi.12909
38. Tombazzi CR, Loy P, Bondar V, Ruiz JI, Waters B, Tombazzi CR. Accuracy of endoscopic ultrasound in staging of early rectal cancer. Fed Pract (2019) 36(Suppl 5):S26–s29.
39. Cavo M, Terpos E, Nanni C, Moreau P, Lentzsch S, Zweegman S, et al. Role of (18)F-FDG PET/CT in the diagnosis and management of multiple myeloma and other plasma cell disorders: a consensus statement by the international myeloma working group. Lancet Oncol (2017) 18(4):e206–17. doi: 10.1016/S1470-2045(17)30189-4
40. Van Cutsem E, Cervantes A, Adam R, Sobrero A, Van Krieken JH, Aderka D, et al. ESMO consensus guidelines for the management of patients with metastatic colorectal cancer. Ann Oncol (2016) 27(8):1386–422. doi: 10.1093/annonc/mdw235
41. Zou Y, Tong J, Leng H, Jiang J, Pan M, Chen Z. Diagnostic value of using 18F-FDG PET and PET/CT in immunocompetent patients with primary central nervous system lymphoma: a systematic review and meta-analysis. Oncotarget (2017) 8(25):41518–28. doi: 10.18632/oncotarget
42. Lucia F, Visvikis D, Desseroit MC, Miranda O, Malhaire JP, Robin P, et al. Prediction of outcome using pretreatment (18)F-FDG PET/CT and MRI radiomics in locally advanced cervical cancer treated with chemoradiotherapy. Eur J Nucl Med Mol Imaging (2018) 45(5):768–86. doi: 10.1007/s00259-017-3898-7
43. Yukimoto R, Uemura M, Tsuboyama T, Hata T, Fujino S, Ogino T, et al. Efficacy of positron emission tomography in diagnosis of lateral lymph node metastases in patients with rectal cancer: a retrospective study. BMC Cancer (2021) 21(1):520. doi: 10.1186/s12885-021-08278-6
44. Yahya JB, Farrell MJ, Herzig DO, Degnin CR, Chen Y, Holland J, et al. Preferential use of imaging modalities in staging newly diagnosed rectal cancer: a survey of US radiation oncologists. J Gastrointest Oncol (2018) 9(3):435–40. doi: 10.21037/jgo.2018.01.19
45. Tateishi U, Maeda T, Morimoto T, Miyake M, Arai Y, Kim EE. Non-enhanced CT versus contrast-enhanced CT in integrated PET/CT studies for nodal staging of rectal cancer. Eur J Nucl Med Mol Imaging (2007) 34(10):1627–34. doi: 10.1007/s00259-007-0455-9
46. Huang YQ, Liang CH, He L, Tian J, Liang CS, Chen X, et al. Development and validation of a radiomics nomogram for preoperative prediction of lymph node metastasis in colorectal cancer. J Clin Oncol (2016) 34(18):2157–64. doi: 10.1200/JCO.2015.65.9128
47. Li C, Yin J. Radiomics based on T2-weighted imaging and apparent diffusion coefficient images for preoperative evaluation of lymph node metastasis in rectal cancer patients. Front Oncol (2021) 11:671354. doi: 10.3389/fonc.2021.671354
48. Chen LD, Liang JY, Wu H, Wang Z, Li SR, Li W, et al. Multiparametric radiomics improve prediction of lymph node metastasis of rectal cancer compared with conventional radiomics. Life Sci (2018) 208:55–63. doi: 10.1016/j.lfs.2018.07.007
49. Gao Y, Zhang XX, Li S. [Application of artificial intelligence technology in the diagnosis and treatment of colorectal cancer]. Chinese (2020) 23(12):1155–8. doi: 10.3760/cma.j.cn.441530-20200213-00054
50. Ichimasa K, Kudo SE, Mori Y, Misawa M, Matsudaira S, Kouyama Y, et al. Artificial intelligence may help in predicting the need for additional surgery after endoscopic resection of T1 colorectal cancer. Endoscopy (2018) 50(3):230–40. doi: 10.1055/s-0043-122385
51. Kudo SE, Ichimasa K, Villard B, Mori Y, Misawa M, Saito S, et al. Artificial intelligence system to determine risk of T1 colorectal cancer metastasis to lymph node. Gastroenterology (2021) 160(4):1075–1084.e1072. doi: 10.1053/j.gastro.2020.09.027
52. Li J, Zhou Y, Wang P, Zhao H, Wang X, Tang N, et al. Deep transfer learning based on magnetic resonance imaging can improve the diagnosis of lymph node metastasis in patients with rectal cancer. Quant Imaging Med Surg (2021) 11(6):2477–85. doi: 10.21037/qims-20-525
53. Bedrikovetski S, Dudi-Venkata NN, Kroon HM, Seow W, Vather R, Carneiro G, et al. Artificial intelligence for pre-operative lymph node staging in colorectal cancer: a systematic review and meta-analysis. BMC Cancer (2021) 21(1):1058. doi: 10.1186/s12885-021-08773-w
54. Ding L, Liu G, Zhang X, Liu S, Li S, Zhang Z, et al. A deep learning nomogram kit for predicting metastatic lymph nodes in rectal cancer. Cancer Med (2020) 9(23):8809–20. doi: 10.1002/cam4.3490
55. Wang H, Wang H, Song L, Guo Q. (2019). Automatic diagnosis of rectal cancer based on CT images by deep learning method, in: 2019 12th International Congress on Image and Signal Processing, BioMedical Engineering and Informatics (CISP-BMEI), Suzhou, China. pp. 2011–5. doi: 10.1109/CISP-BMEI48845.2019.8965731
56. Zhou YP, Li S, Zhang XX, Zhang ZD, Gao YX, Ding L, et al. [High definition MRI rectal lymph node aided diagnostic system based on deep neural network]. Chinese (2019) 57(2):108–13. doi: 10.3760/cma.j.issn
57. Lu Y, Yu Q, Gao Y, Zhou Y, Liu G, Dong Q, et al. Identification of metastatic lymph nodes in MR imaging with faster region-based convolutional neural networks. Cancer Res (2018) 78(17):5135–43. doi: 10.1158/0008-5472.CAN-18-0494
58. Li J, Wang P, Li Y, Zhou Y, Liu X, Luan K. (2018). Transfer learning of pre- trained inception-V3 model for colorectal cancer lymph node metastasis classification, in: 2018 IEEE International Conference on Mechatronics and Automation (ICMA), Changchun, China. pp. 1650–4. doi: 10.1109/ICMA.2018.8484405
59. Li M, Zhang J, Dan Y, Yao Y, Dai W, Cai G, et al. A clinical-radiomics nomogram for the preoperative prediction of lymph node metastasis in colorectal cancer. J Transl Med (2020) 18(1):46. doi: 10.1186/s12967-020-02215-0
60. Yang YS, Feng F, Qiu YJ, Zheng GH, Ge YQ, Wang YT. High-resolution MRI-based radiomics analysis to predict lymph node metastasis and tumor deposits respectively in rectal cancer. Abdom Radiol (NY) (2021) 46(3):873–84. doi: 10.1007/s00261-020-02733-x
61. Nakanishi R, Akiyoshi T, Toda S, Murakami Y, Taguchi S, Oba K, et al. Radiomics approach outperforms diameter criteria for predicting pathological lateral lymph node metastasis after neoadjuvant (Chemo)Radiotherapy in advanced low rectal cancer. Ann Surg Oncol (2020) 27(11):4273–83. doi: 10.1245/s10434-020-08974-w
62. Zhou X, Yi Y, Liu Z, Zhou Z, Lai B, Sun K, et al. Radiomics-based preoperative prediction of lymph node status following neoadjuvant therapy in locally advanced rectal cancer. Front Oncol (2020) 10:604. doi: 10.3389/fonc.2020.00604
63. Liu X, Yang Q, Zhang C, Sun J, He K, Xie Y, et al. Multiregional-based magnetic resonance imaging radiomics combined with clinical data improves efficacy in predicting lymph node metastasis of rectal cancer. Front Oncol (2020) 10:585767. doi: 10.3389/fonc.2020.585767
64. Su Y, Zhao H, Liu P, Zhang L, Jiao Y, Xu P, et al. A nomogram model based on MRI and radiomic features developed and validated for the evaluation of lymph node metastasis in patients with rectal cancer. Abdom Radiol (NY) (2022) 47(12):4103–14. doi: 10.1007/s00261-022-03672-5
65. Jia H, Jiang X, Zhang K, Shang J, Zhang Y, Fang X, et al. A nomogram of combining IVIM-DWI and MRI radiomics from the primary lesion of rectal adenocarcinoma to assess nonenlarged lymph node metastasis preoperatively. J Magn Reson Imaging (2022) 56(3):658–67. doi: 10.1002/jmri.28068
66. Li J, Zhou Y, Wang X, Zhou M, Chen X, Luan K. An MRI-based multi-objective radiomics model predicts lymph node status in patients with rectal cancer. Abdom Radiol (NY) (2021) 46(5):1816–24. doi: 10.1007/s00261-020-02863-2
67. Zhu H, Zhang X, Li X, Shi Y, Zhu H, Sun Y. Prediction of pathological nodal stage of locally advanced rectal cancer by collective features of multiple lymph nodes in magnetic resonance images before and after neoadjuvant chemoradiotherapy. Chin J Cancer Res (2019) 31(6):984–92. doi: 10.21147/j.issn.1000-9604.2019.06.14
68. Cai H, Cui C, Tian H, Zhang M, Li L. A novel approach to segment and classify regional lymph nodes on computed tomography images. Comput Math Methods Med (2012) 2012:145926. doi: 10.1155/2012/145926
69. Tse DM, Joshi N, Anderson EM, Brady M, Gleeson FV. A computer-aided algorithm to quantitatively predict lymph node status on MRI in rectal cancer. Br J Radiol (2012) 85(1017):1272–8. doi: 10.1259/bjr/13374146
70. Cui C, Cai H, Liu L, Li L, Tian H, Li L. Quantitative analysis and prediction of regional lymph node status in rectal cancer based on computed tomography imaging. Eur Radiol (2011) 21(11):2318–25. doi: 10.1007/s00330-011-2182-7
71. Keller DS, Berho M, Perez RO, Wexner SD, Chand M. The multidisciplinary management of rectal cancer. Nat Rev Gastroenterol Hepatol (2020) 17(7):414–29. doi: 10.1038/s41575-020-0275-y
72. Yu L, Wang L, Tan Y, Hu H, Shen L, Zheng S, et al. Accuracy of magnetic resonance imaging in staging rectal cancer with multidisciplinary team: a single-center experience. J Cancer (2019) 10(26):6594–8. doi: 10.7150/jca.32685
73. Fan H, Zhu JH, Yao XQ. Long non-coding RNA PVT1 as a novel potential biomarker for predicting the prognosis of colorectal cancer. Int J Biol Markers (2018) 33(4):415–22. doi: 10.1177/1724600818777242
74. Chen Z, Cai X, Chang L, Xia Y, Wang L, Hou Y, et al. LINC00152 is a potential biomarker involved in the modulation of biological characteristics of residual colorectal cancer cells following chemoradiotherapy. Oncol Lett (2018) 15(4):4177–84. doi: 10.3892/ol.2018.7833
75. Gharib E, Anaraki F, Baghdar K, Ghavidel P, Sadeghi H, Nasrabadi PN, et al. Investigating the diagnostic performance of HOTTIP, PVT1, and UCA1 long noncoding RNAs as a predictive panel for the screening of colorectal cancer patients with lymph node metastasis. J Cell Biochem (2019) 120(9):14780–90. doi: 10.1002/jcb.28739
76. Fan XJ, Wan XB, Huang Y, Cai HM, Fu XH, Yang ZL, et al. Epithelial-mesenchymal transition biomarkers and support vector machine guided model in preoperatively predicting regional lymph node metastasis for rectal cancer. Br J Cancer (2012) 106(11):1735–41. doi: 10.1038/bjc.2012.82
77. Chen Z, Zhong T, Zhong J, Tang Y, Ling B, Wang L. MicroRNA−129 inhibits colorectal cancer cell proliferation, invasion and epithelial−to−mesenchymal transition by targeting SOX4. Oncol Rep (2021) 45(5):1–11. doi: 10.3892/or.2021.8012
78. Huang CY, Lee KC, Tung SY, Huang WS, Teng CC, Lee KF, et al. 2D-DIGE-MS proteomics approaches for identification of gelsolin and peroxiredoxin 4 with lymph node metastasis in colorectal cancer. Cancers (Basel) (2022) 14(13):3189. doi: 10.3390/cancers14133189
79. Leong KJ, Beggs A, James J, Morton DG, Matthews GM, Bach SP. Biomarker-based treatment selection in early-stage rectal cancer to promote organ preservation. Br J Surg (2014) 101(10):1299–309. doi: 10.1002/bjs.9571
80. Silinsky J, Grimes C, Driscoll T, Green H, Cordova J, Davis NK, et al. CD 133+ and CXCR4+ colon cancer cells as a marker for lymph node metastasis. J Surg Res (2013) 185(1):113–8. doi: 10.1016/j.jss.2013.05.049
81. Huang L, Wang X, Wen C, Yang X, Song M, Chen J, et al. Hsa-miR-19a is associated with lymph metastasis and mediates the TNF-α induced epithelial-to-mesenchymal transition in colorectal cancer. Sci Rep (2015) 5:13350. doi: 10.1038/srep13350
82. Karamitopoulou E, Zlobec I, Patsouris E, Peros G, Lugli A. Loss of e-cadherin independently predicts the lymph node status in colorectal cancer. Pathology (2011) 43(2):133–7. doi: 10.1097/PAT.0b013e3283425b7f
83. Tsilimigras MC, Fodor A, Jobin C. Carcinogenesis and therapeutics: the microbiota perspective. Nat Microbiol (2017) 2:17008. doi: 10.1038/nmicrobiol.2017.8
84. Yachida S, Mizutani S, Shiroma H, Shiba S, Nakajima T, Sakamoto T, et al. Metagenomic and metabolomic analyses reveal distinct stage-specific phenotypes of the gut microbiota in colorectal cancer. Nat Med (2019) 25(6):968–76. doi: 10.1038/s41591-019-0458-7
85. Wang J, Jia H. Metagenome-wide association studies: fine-mining the microbiome. Nat Rev Microbiol (2016) 14(8):508–22. doi: 10.1038/nrmicro.2016.83
86. Yu J, Feng Q, Wong SH, Zhang D, Liang QY, Qin Y, et al. Metagenomic analysis of faecal microbiome as a tool towards targeted non-invasive biomarkers for colorectal cancer. Gut (2017) 66(1):70–8. doi: 10.1136/gutjnl-2015-309800
87. Casimiro-Soriguer CS, Loucera C, Peña-Chilet M, Dopazo J. Towards a metagenomics machine learning interpretable model for understanding the transition from adenoma to colorectal cancer. Sci Rep (2022) 12(1):450. doi: 10.1038/s41598-021-04182-y
88. Wirbel J, Pyl PT, Kartal E, Zych K, Kashani A, Milanese A, et al. Meta-analysis of fecal metagenomes reveals global microbial signatures that are specific for colorectal cancer. Nat Med (2019) 25(4):679–89. doi: 10.1038/s41591-019-0406-6
89. Thomas AM, Manghi P, Asnicar F, Pasolli E, Armanini F, Zolfo M, et al. Metagenomic analysis of colorectal cancer datasets identifies cross-cohort microbial diagnostic signatures and a link with choline degradation. Nat Med (2019) 25(4):667–78. doi: 10.1038/s41591-019-0405-7
90. Repass J, Maherali N, Owen K. Registered report: fusobacterium nucleatum infection is prevalent in human colorectal carcinoma. Elife (2016) 5:299–306. doi: 10.7554/eLife.25801
91. Yan X, Liu L, Li H, Qin H, Sun Z. Clinical significance of fusobacterium nucleatum, epithelial-mesenchymal transition, and cancer stem cell markers in stage III/IV colorectal cancer patients. Onco Targets Ther (2017) 10:5031–46. doi: 10.2147/OTT.S145949
92. Castellarin M, Warren RL, Freeman JD, Dreolini L, Krzywinski M, Strauss J, et al. Fusobacterium nucleatum infection is prevalent in human colorectal carcinoma. Genome Res (2012) 22(2):299–306. doi: 10.1101/gr.126516
93. Vesteghem C, Brøndum RF, Sønderkær M, Sommer M, Schmitz A, Bødker JS, et al. Implementing the FAIR data principles in precision oncology: review of supporting initiatives. Brief Bioinform (2020) 21(3):936–45. doi: 10.1093/bib/bbz044
94. Hosny A, Parmar C, Quackenbush J, Schwartz LH, Aerts HJWL. Artificial intelligence in radiology. Nat Rev Cancer (2018) 18(8):500–10. doi: 10.1038/s41568-018-0016-5
Keywords: rectal cancer, lymph node metastasis, diagnosis, OMICS data, imaging data
Citation: Peng W, Qiao H, Mo L and Guo Y (2023) Progress in the diagnosis of lymph node metastasis in rectal cancer: a review. Front. Oncol. 13:1167289. doi: 10.3389/fonc.2023.1167289
Received: 15 March 2023; Accepted: 28 June 2023;
Published: 13 July 2023.
Edited by:
Hiroki Hashida, Kobe City Medical Center General Hospital, JapanReviewed by:
Jiancong Hu, Sun Yat-sen University, ChinaJunjun She, The First Affiliated Hospital of Xi’an Jiaotong University, China
Copyright © 2023 Peng, Qiao, Mo and Guo. This is an open-access article distributed under the terms of the Creative Commons Attribution License (CC BY). The use, distribution or reproduction in other forums is permitted, provided the original author(s) and the copyright owner(s) are credited and that the original publication in this journal is cited, in accordance with accepted academic practice. No use, distribution or reproduction is permitted which does not comply with these terms.
*Correspondence: You Guo, gy@gmu.edu.cn