- 1Department of Thoracic Surgery, Affiliated Hospital of Jiangnan University, Wuxi, Jiangsu, China
- 2Department of Thoracic Surgery, First Affiliated Hospital of Soochow University, Suzhou, Jiangsu, China
- 3Wuxi School of Medicine, Jiangnan University, Wuxi, Jiangsu, China
- 4Department of Nutrition, Affiliated Hospital of Jiangnan University, Wuxi, Jiangsu, China
- 5Functional Food Clinical Evaluation Center, Affiliated Hospital of Jiangnan University, Wuxi, Jiangsu, China
- 6Lifestyle-Medicine Strategy to Improve Outcome for Cancer patients (LIOC) Group, Chinese Society of Nutritional Oncology, Beijing, China
- 7Department of Infection Control, Affiliated Hospital of Jiangnan University, Wuxi, Jiangsu, China
- 8School of Bioengineering, Jiangnan University, Wuxi, Jiangsu, China
- 9School of Environment and Civil Engineering, Jiangnan University, Wuxi, Jiangsu, China
- 10Chinese Society of Nutritional Oncology, Beijing, China
Introduction: The occurrence and progression of lung cancer are influenced by pulmonary microbiota, yet the relationship between changes in the pulmonary microbiota and lung cancer remains unclear.
Methods: To investigate the correlation between pulmonary microbiota and the signature of lung lesions, we analyzed the microbial composition at sites adjacent to the stage 1 adenocarcinoma, squamous carcinoma and benign lesion tissues in 49 patients by using 16S ribosomal RNA gene sequencing. We then conducted Linear discriminant analysis, receiver operating characteristic (ROC) curve analysis and PICRUSt prediction based on 16S sequencing results.
Results: Overall, the microbiota composition at sites close to lung lesions showed significant differences between different lesion types. Based on the results of LEfSe analysis, Ralstonia, Acinetobacter and Microbacterium are the dominant genera of lung adenocarcinoma (LUAD), lung squamous carcinoma (LUSC) and benign lesions (BENL), respectively. Furthermore, we determined the diagnostic value of the abundance ratio of Ralstonia to Acinetobacter in adenocarcinoma patients through ROC curve analysis. The PICRUSt analysis revealed 15 remarkably different metabolic pathways in these lesion types. In LUAD patients, the increase of the pathway associated with xenobiotic biodegradation may be due to the continuous proliferation of microbe with degradation ability of xenobiotics, which implied that LUAD patients are often exposed to harmful environment.
Discussion: The abundance of Ralstonia was related to the development of lung cancer. By measuring the abundance of microbiota in diseased tissues, we can distinguish between different types of lesions. The differences in pulmonary microbiota between lesion types are significant in understanding the occurrence and development of lung lesions.
Introduction
Lung cancer has become a malignant tumor with the highest morbidity and mortality (1). In recent decades, the incidence of lung cancer has gradually increased, and the epidemic spectrum has changed. Currently, the incidence rate of adenocarcinoma has rapidly surpassed that of squamous cell carcinoma and other pathological types of lung cancer. The reason for the rapid increase in the incidence rate of lung adenocarcinoma is not clear; smoking remains the primary risk factor for lung cancer (2), while other risk factors include family history of lung cancer, estrogen, lifestyle, exposure to radioactive substances, and chronic inflammation of the lung caused by microorganisms (3–5). Recent studies have found that the steady state of the respiratory tract flora is essential for good health (6). Lee et al. (7) compared the microbial composition of bronchoalveolar fluid between patients with lung cancer and those with benign lesions, and the two genera (Veillonella and Megasphaera) were found to be relatively more abundant in patients with lung cancer. Hosgood et al. (8) observed that the diversity of respiratory tract microbial composition in non-smoking female lung cancer patients was significantly higher than that in the control group, and lung cancer cases had an enrichment of respiratory tract microbiota compared to controls, especially Streptococcus. Yu et al. reported that the microbial composition in squamous cell carcinoma tumor samples was different from that in adenocarcinoma (9). A recent study compared lung microbial composition between patients with lung cancer and healthy controls and reported that a unique lung microbiome is associated with tumor tissue. In squamous cell carcinoma samples with TP53 mutations, a higher abundance of Acidovorax was discovered. In addition, Klebsiella, Comamonas, Acidovorax, Polarmonas, and Rhodoferax genera were more frequently found in small-cell carcinomas, but they were not found in adenocarcinoma (10). However, previous studies investigating the relationship between lung cancer and respiratory tract microorganisms often relied on sample collection from sputum or bronchial washing fluid (BWF), which may be susceptible to contamination with microorganisms from the upper respiratory tract. Furthermore, as the bronchoscope often fails to reach the lesion site, samples may not accurately reflect the composition of microorganisms near the tumor. To better investigate the composition of microorganisms near the lung lesion, we studied the pulmonary microbiome of different pathologic types.
Methods
Participants and general information
A total of 49 inpatients who underwent pulmonary lesion resection in the Affiliated Hospital of Jiangnan University from June 2020 to June 2021 were selected, and the types of patients were benign lesions or stage 1 cancer. All patients provided informed consent and agreed to provide their resected lung tissue for analysis. This study was approved by the Ethics Committee of the Affiliated Hospital of Jiangnan University (Ethics No. 201889) and registered on the Chinese Clinical Trial Register as ChiCTR2000031192. Patients were excluded if they had received antibiotics within one month before the surgery, suffered from a pulmonary infection within one month, or suffered from small cell lung cancer, pulmonary tuberculosis, pulmonary cryptococcosis, or received long-term immunosuppressive drug treatment (11).
Sample collection and processing
Immediately after isolating the lung tissue, para-cancerous tissue or adjacent tissue within 2 cm of the benign lesions, measuring about 1 ∗ 1 cm in size, was collected and placed in dry ice, then transferred to the −80°C deep-frozen refrigerator. After thawing, the lung tissue was cut open and rinsed with sterile normal saline to collect the flushing fluid. According to histological classification, all tissues were divided into three groups: benign lesion (BENL) group, squamous cell carcinoma (LUSC) group, and adenocarcinoma (LUAD) group. Benign lesions include pulmonary bullae and fibromas.
DNA extraction and genomic sequencing
Total DNA was extracted from samples using the E.Z.N.A. ® Soil DNA Kit (Omega Bio-tek, Norcross, GA, U.S.). The quality of the genomic DNA was detected by 1% agarose gel electrophoresis, and the concentration and purity of the genome were determined by NanoDrop2000 (Thermo Fisher Scientific). The V3–V4 region of the 16S rRNA gene was amplified by PCR using primers 338F (5’-ACTCCTACGGGAGGCAGCAG-3’) and 806R (5’-GGACTACHVGGGTWTCTAAT-3’) (12). PCR amplification was performed in triplicate for each sample using a reaction mixture (20 μl), which contains 4 μl of 5×TransStart FastPfu buffer, 10 ng of template DNA, 0.5 μM of each primer, 2 μl of 2.5 mM dNTPs, and 0.4 μl of TransStart FastPfu DNA polymerase. The PCR procedure included an initial denaturation step at 95°C for 2 min, subsequent 29 cycles of temperature gradient at 95°C for 30 s, 55°C for 30 s, and 72°C for 30 s, an extension step at 72°C for 10 min, and finally a preservation step at 4°C in a GeneAmp 9700 thermocycler (ABI, USA). The triplicate amplicons were pooled together and recovered with a 2% (w/v) agarose gel, purified using an AxyPrep DNA Gel Extraction Kit (Axygen Biosciences, Union City, CA, USA), electrophoresed on a 2% (w/v) agarose gel, and the purified amplicon was quantified using a Quantus™ Fluorometer (Promega, USA). Under the same system (the same experimental steps and reagents), each barcore was set up as a blank negative control sample for the PCR process, with a total of 24 CK samples for follow-up experiments.
A composite sequencing library was constructed from the purified amplicon using the NEXTFLEX Rapid DNA-Seq Kit: (1) connector link; (2) magnetic bead screening was used to remove the self-connected fragments of the joint; (3) the library template was enriched by PCR amplification; and (4) the PCR products were recovered by magnetic beads to obtain the final library. The blank control group underwent the same process of risk database construction and sequencing. The libraries were sequenced on an Illumina Miseq sequencing platform (Illumina, San Diego, USA). The original data was uploaded to the NCBI SRA database (serial number: SRP * * *).
Bioinformatics analysis
The quality of original paired-end sequencing sequences was controlled using fastp (13) (https://github.com/OpenGene/fastp, version 0.19.6) and combined using FLASH (14) (http://www.cbcb.umd.edu/software/flash, version 1.2.11). Sequences were then dereplicated and clustered de novo into operational taxonomic units (OTUs) at 97% similarity using UPARSE (15) (http://drive5.com/uparse/, version 7.1). Taxonomic assignment was performed by the RDP classifier (16) (http://rdp.cme.msu.edu/, version 2.11) using the default confidence level of 0.7 against the Silva 16S rRNA database (v138). Then statistical analyses were conducted using the R package decontam (https://github.com/benjjneb/decontam), and the prevalence-based strategy was selected to eliminate the possible pollution annotated in the environmental samples by using the high-frequency species of the negative control samples (17). Finally, the number of sequences in all samples was flattened, and then the community composition of each sample was counted at different species classification levels to minimize the impact of sequencing depth on subsequent alpha and beta diversity data analysis. PICRUSt2 (18) (version 2.2.0) was used for 16S function prediction analysis.
Results
Characteristics of the study participants
A total of 49 patients were divided into three groups based on the histological classification of the lesions: 11 participants in the BENL group, nine participants in the LUSC group, and 29 participants in the LUAD group. The baseline data (gender, age, and smoking index) of participants in all three groups were statistically analyzed. The smoking index was calculated by multiplying the number of cigarettes per day by the number of years of smoking. There was no statistically significant difference in gender distribution, age, or smoking index among the three groups (P >0.05) (Table 1).
α-diversity
According to the Shannon index and the Gini-Simpson index, the α-diversity of the pulmonary microbiota in three lung lesions was analyzed. Statistical analysis revealed a significant difference in α-diversity between the BENL group and the LUAD group, as well as between the LUSC group and the LUAD group, while there was no statistical difference between the BENL group and the LUSC group. Overall, the pulmonary microbiota in the LUAD group exhibited the lowest diversity (Figure 1).
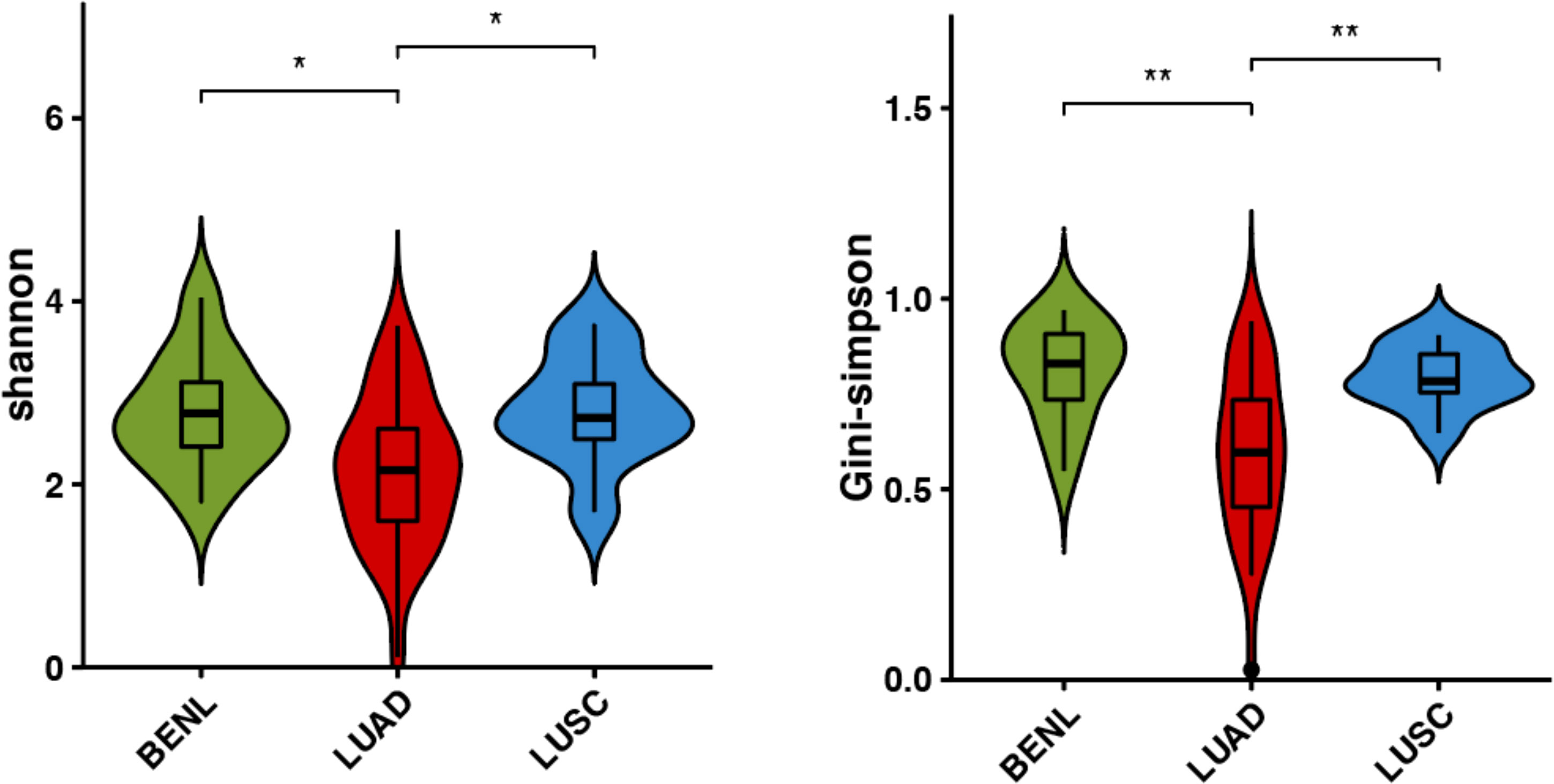
Figure 1 α-diversity in bacterial communities in three classifications of lung lesions. Shannon index and Simpson index were indexes to evaluate α-diversity, and there were differences in the LUAD group, BENL group, and LUSC group. *P<0.05, **P<0.01.
β-diversity
Clusters of pulmonary bacterial genera were identified in hierarchical clustering of BENL, LUSC, and LUAD samples, indicating that pulmonary microbiota in the LUSC group were similar to those in the BENL group, while the LUAD group had an obvious different microbial structure compared with the BENL and LUSC groups (Figure 2A). The β-diversity of pulmonary microbiota based on Principal Coordinates Analysis (PCoA) showed similar differences with hierarchical clustering resulting in structures between three groups (Figure 2B). PERMANOVA analysis suggested that pulmonary microbial structure in the LUAD group showed a significant difference from that in the BENL and LUSC groups (LUAD vs. BENL, P = 0.001; LUAD vs. LUSC, P = 0.002), while no difference in microbiota structure was observed between the BENL and LUSC groups (P = 0.16).
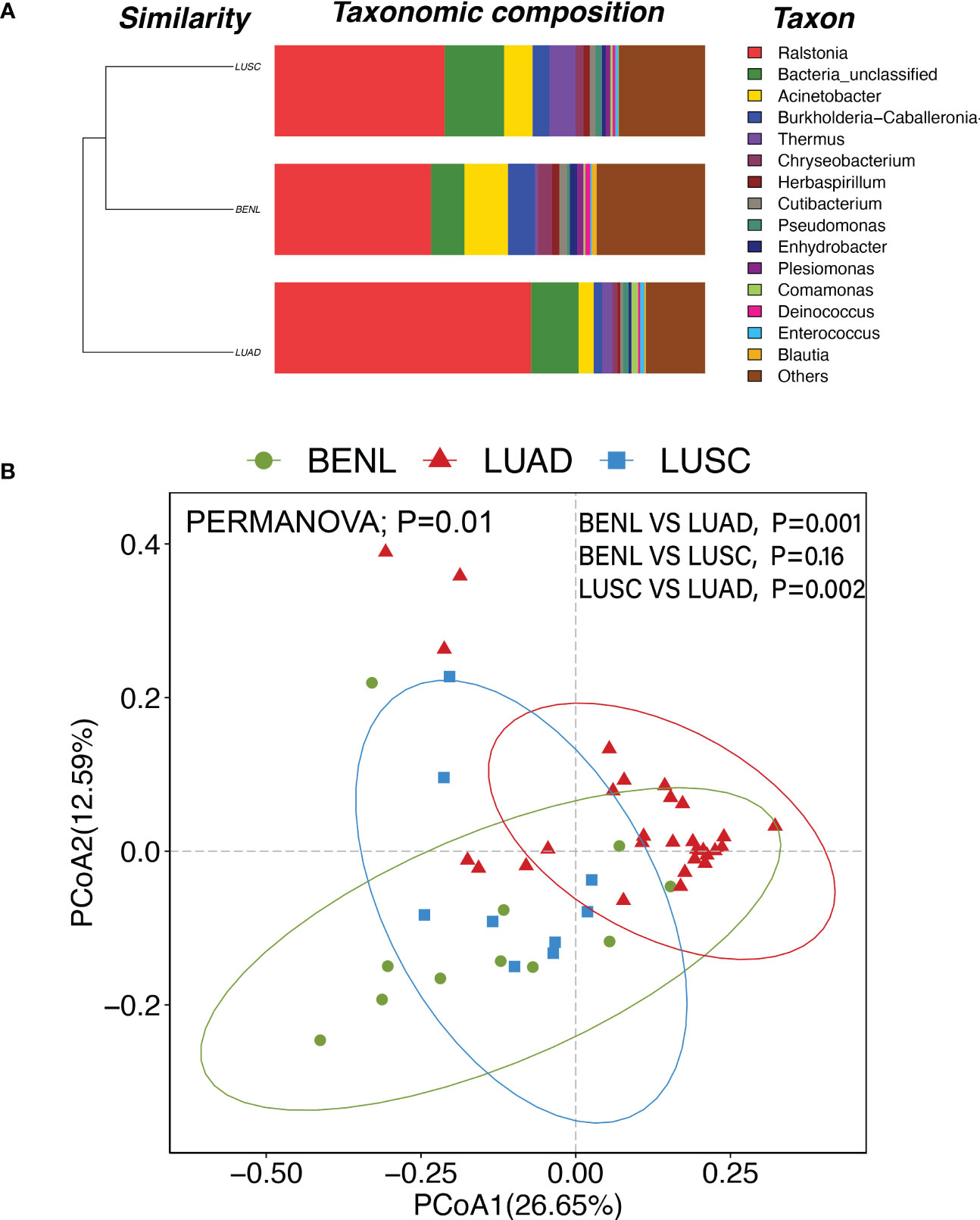
Figure 2 (A) At the genus level, the LUSC group and the BENL group had higher similarity and a closer cluster analysis distance, while the LUAD group was far apart and had poor similarity compared with the LUSC and BENL groups. (B) PCoA diagram and PERMANOVA analysis showed that there were significant differences in pulmonary microbial structures between LUAD and BENL groups (P = 0.001), LUAD and LUSC groups (P = 0.002), but no significant differences between LUSC and BENL groups (P = 0.16).
The representative taxa in each group
The linear discriminant analysis (LDA) effect size (LEfSe) method was used to screen out key OTUs that could discriminate among three groups. Ralstonia, Microbacterium, and Acinetobacter were representative taxa in the LUAD, LUSC, and BENL groups, respectively (Figure 3A). The abundance of Ralstonia in the LUAD group was significantly higher than that in the BENL and LUSC groups. The abundance of Microbacterium in the LUSC group was higher than that in the LUAD and BENL groups. The abundance of Acinetobacter in the BENL group was significantly higher than that in the LUSC and LUAD groups (Figures 3B, C).
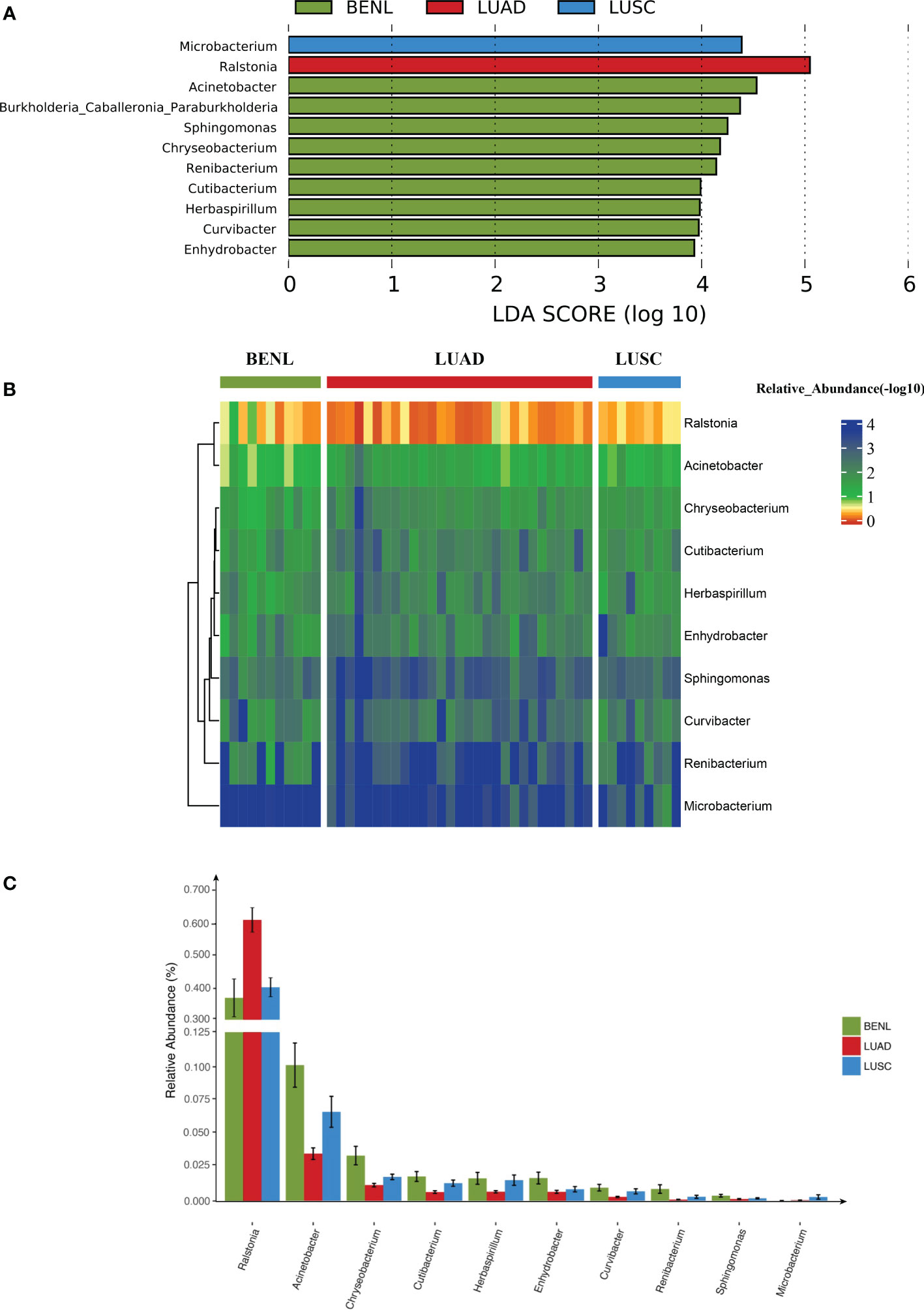
Figure 3 Lung microbiota in lung cancer and relevance. (A) The abundance of Ralstonia in the LUAD group was significantly higher than that of BENL and the LUSC group by statistical analysis of the top three genera. (B) The abundance of Microbacterium in the LUSC group was significantly higher than that in the LUAD and BENL groups. The relative abundance of the displayed microbes was presented in a heat map (−log 10). Different colors corresponded to different abundances; smaller numbers corresponded to higher abundances. (C) The abundance of Acinetobacter in the BENL group was significantly higher than that in the LUSC and LUAD groups.
ROC curve analysis
The receiver operating characteristic (ROC) curve (AUC) between lesion specimens was used to evaluate the prediction accuracy of three genera (Ralstonia, Acinetobacter, and Microbacterium), which were screened out by LEfSe. Ralstonia (AUC = 0.887) had good specificity and sensitivity in distinguishing the LUAD and LUSC groups. Between the LUAD and BENL groups, Acinetobacter (AUC = 0.8871) was the best predicted genus. Acinetobacter (AUC = 0.7517) was the best genus for discriminating LUAD (vs. unLUAD), followed by Ralstonia (AUC = 0.7388). Since the abundance of Ralstonia was higher while that of Acinetobacter was lower in the LUAD group compared with that in the LUSC and BENL groups, we attempted to use the abundance ratio of Ralstonia to Acinetobacter to predict LUAD more efficiently. As expected, this ratio (AUC = 0.8121) can well predict LUAD (Figure 4).
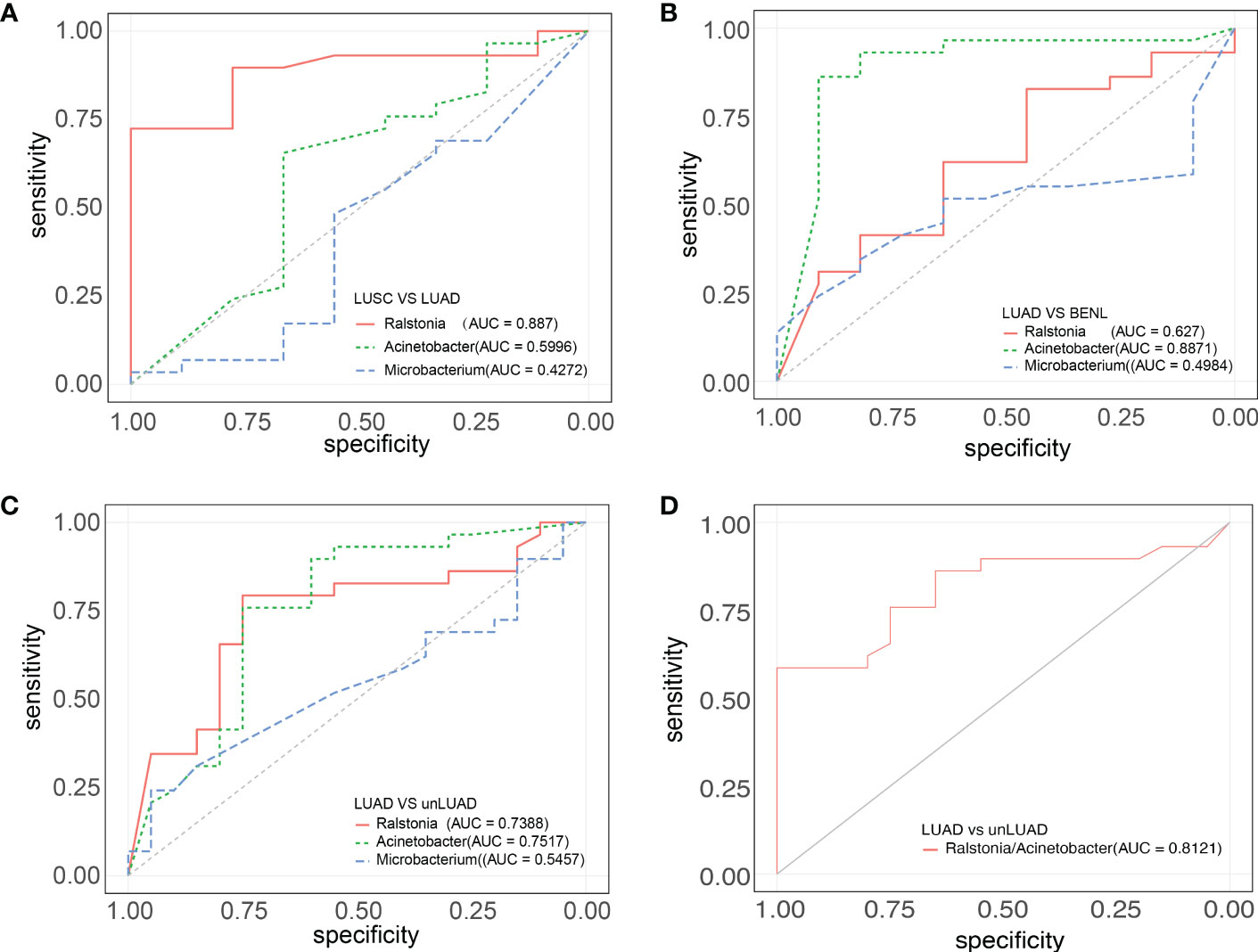
Figure 4 ROC curves of the three groups of highly abundant strains. (A) Ralstonia (AUC = 0.887) had good specificity and sensitivity to distinguish LUAD and LUSC. (B) Acinetobacter (AUC = 0.8871) has good specificity and sensitivity to distinguish between LUAD and BENL. (C) The prediction effects of Ralstonia (AUC = 0.7388) and Acinetobacter (AUC = 0.7517) were good and had certain diagnostic value. (D) The abundance ratio of Ralstonia to Acinetobacter (AUC = 0.8121) can well predict LUAD and unLUAD.
PICRUSt prediction based on 16S sequencing results
In the functional annotation analysis based on 16S rRNA gene data by PICRUSt, we found an enrichment in the representation of pathways associated with carbohydrate, cofactors, and vitamin metabolism in the LUSC group; pathways associated with alanine, aspartate, and glutamate metabolism in the BENL group; and pathways associated with flagellar assembly, xenobiotic biodegradation and metabolism, and oxidative phosphorylation in the LUAD group (Figure 5).
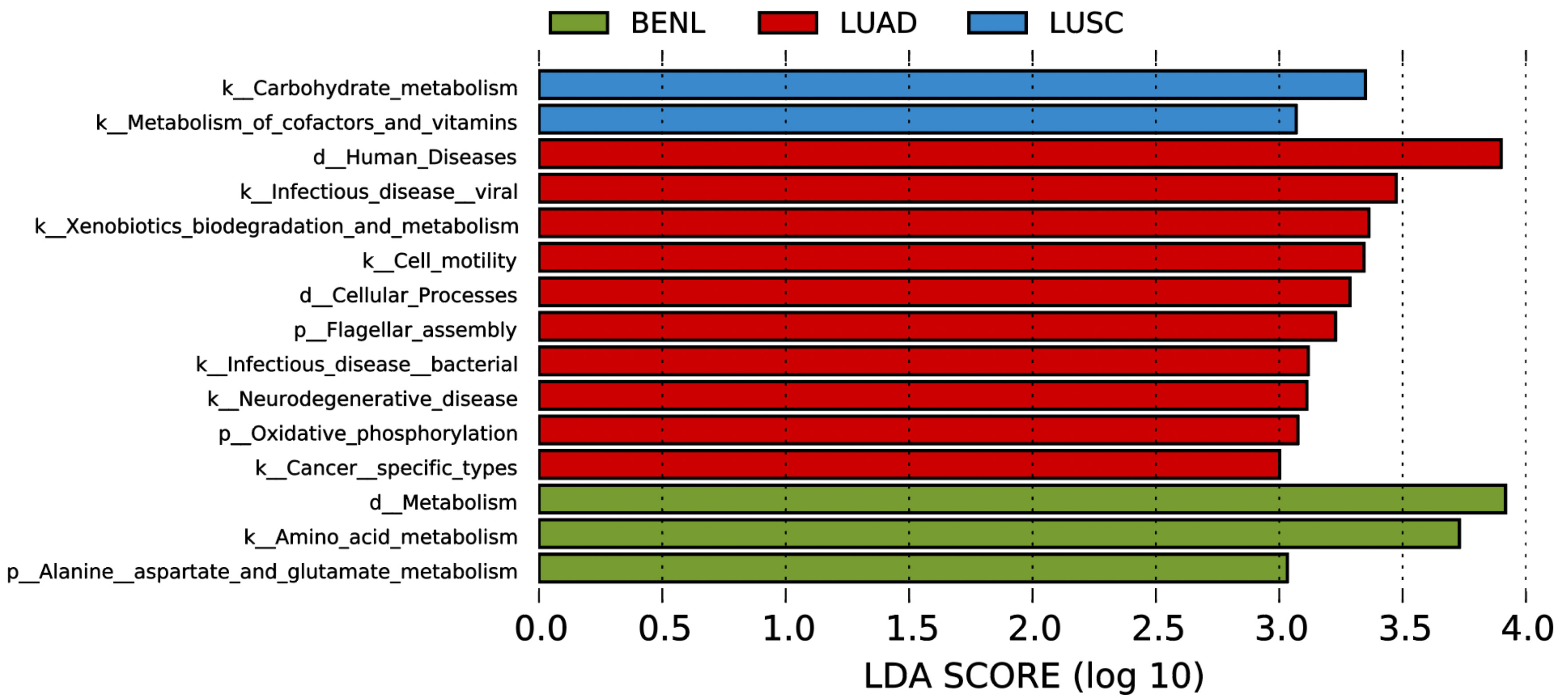
Figure 5 PICRUST-based lung microbiota study in patients with LUSC, LUAD, and BENL. Results are colored by the lesion’s category and sorted in decreasing order of degree of increase within each category. Note that the LDA score (log 10) was >3.0, and the region 3.0–4.0 displayed pathways with more increase.
Discussion
Under normal circumstances, the symbiotic microbial community is beneficial to human health. However, disordered microbial communities result in changes in the local microenvironment and induce the body’s immune response (19), leading to various diseases, the most serious of which are malignant tumors. Previous studies have shown that a variety of malignancies are directly caused by potential gastrointestinal opportunistic microorganisms, such as Helicobacter pylori, which is known to promote the occurrence and progression of gastric cancer (20, 21), and the amount of Fusobacterium nucleatum in colorectal carcinoma has been associated with shorter patient survival (22). Thus, the microbiota plays an important role in the development of colon cancer.
The change in lung microorganisms also has a certain correlation with the occurrence and development of lung cancer. Germ-free rats were found to be less likely to develop lung cancer than infected rats (23). Capnocytophaga, Selenomonas, Veillonella, and Neisseria were found to be significantly altered in the saliva of patients with squamous cell carcinoma and adenocarcinoma (24). However, it is important to note that many of these studies have used samples collected from bronchoalveolar lavage fluid, sputum, or bronchial washing fluid, which may have different microbial compositions compared to samples taken closer to the tumor site. Therefore, collecting samples closer to the tumor site may provide a more accurate microbial composition analysis (25). Bronchoalveolar lavage fluid and bronchial washing fluid can seldom reach the alveoli of peripheral lung cancer, and the specimen would be contaminated by bacteria in the proximal bronchus. In lung cancer tissue, the abundance of bacteria is low, and the tumor tissues we collected are mostly stage 1 cancer with a small volume, which is insufficient for microbiome analysis after pathological examination. Microbiota may be mainly collected from the alveoli adjacent to lesion in our study.
It has been suggested that a lower α-diversity of respiratory microbiota was observed in patients with malignant tumors (26). In this study, the pulmonary microbiota in the LUAD group had the lowest diversity. According to β-diversity and hierarchical cluster analysis, pulmonary microbial structure in the LUAD group showed a significant difference from that in the BENL and LUSC groups, while no significant difference in microbial structure was observed between the BENL and LUSC groups.
LEFSe analysis showed that the abundance of Ralstonia in the LUAD group was significantly higher than in the BENL group and the LUSC group. Microbacterium and Acinetobacter were the dominant bacteria in the LUSC group and the BENL group, respectively. These findings suggest that the composition of the microbiota close to tumor tissues has changed. It has been reported that Ralstonia and Helicobacter were the dominant bacteria before gastric cancer surgery, while Streptococcus and Prevotella were the dominant bacteria after the operation (27). It has also been found that Ralstonia can be used as a prediction model for biliary tract cancer (28). We discovered that the abundance ratio of Ralstonia to Acinetobacter can better predict non-LUAD and LUAD, while Ralstonia could be used to distinguish LUAD from LUAC based on the ROC curve. Therefore, we speculate that Ralstonia may play an important role in the occurrence and development of LUAD. By predicting the metabolic pathways of microbiota, we found that compared with the metabolic pathways associated with LUSC and BENL, some specific pathways associated with lung cancer were discovered to increase in LUAD, such as those associated with bacterial infection, xenobiotic biodegradation, and oxidative phosphorylation.
The increase in the pathway associated with bacterial infection has been observed in many cancer studies. Lung cancer has been linked to inflammatory diseases, and lung-specific carcinogenesis is partly associated with a persistent local inflammatory state (29, 30). Inflammation in the tumor microenvironment has many tumor-promoting effects (31). Neutrophils infiltrating mouse tumors can promote carcinogenesis by supporting tumor-related inflammation in mouse models of lung cancer (32). The increase in the pathway associated with xenobiotic biodegradation may be related to the patient’s exposure to more xenobiotics (33). Lee et al. reported that the abundance of Ralstonia increased after the use of cosmetics, indicating that Ralstonia in facial skin may have the capacity to metabolize cosmetic components (34). Some strains of Ralstonia can degrade aromatic hydrocarbons, trichloroethylene, and quinoline in contaminated environments, and the toxicity of the degraded products is weaker compared with the original substances (35). The increase in the oxidative phosphorylation pathway in the LUAD group is also consistent with the pathological process of lung cancer. Studies have concluded that the increase in oxidative phosphorylation is related to the shorter survival time of lung adenocarcinoma patients (36). Lin et al. found that an actin-binding protein can promote lung cancer metastasis and colonization by enhancing mitochondrial oxidative phosphorylation (37). The application of oxidative phosphorylation inhibitors can slow down the metastasis and invasion of mouse malignant tumor cells (38). Deribe et al. found that oxidative phosphorylation (OXPHOS) was enhanced in smarca4 mutant tumors (39).
It has been proven that Ralstonia, an opportunistic pathogen, can accelerate the progression of cystic fibrosis when immunocompromised, making it a worse prognostic marker (40). Ralstonia is found in both water and soil and can infect a wide range of plants globally (41). For humans, Ralstonia is typically considered a low-pathogenic organism. The potential impact of Ralstonia on LUAD still requires further investigation to elucidate the reason for this bacterium being a dominant member near the lesion of LUAD and its role in degrading xenobiotics.
This study innovatively takes the alveoli tissue adjacent to the lesion as the sample, which can more accurately reflect the changes in the microbial environment adjacent to the tumor than bronchoalveolar lavage fluid. Further research is called upon to provide a new mechanism related to the occurrence and development of lung adenocarcinoma and provide new targets and methods for the prevention and treatment of LUAD. However, this study also has a few limitations: 1. The number of samples is modest: a larger sample size may lead to more accurate results. 2. Because most of the extractive genes in tissue flushing fluid are derived from the host, this study did not perform metagenomic sequencing analysis. However, we believe that the results of this study are still of great significance in understanding the role of pulmonary microbiota in the occurrence and development of lung cancer since these tumor tissues are from stage 1 cancer.
In conclusion, the microbial structure in patients with LUAD was significantly different from that in LUSC and BENL. Ralstonia might have an impact on the development of lung adenocarcinoma. We speculate that the increase in the pathway associated with xenobiotic biodegradation in patients with adenocarcinoma may be related to their exposure to more xenobiotics. Notably, by measuring the abundance of microbiota in pathological tissues, we can predict the types of lesions. As the histopathologic examination of the tissue is the conventional method, the abundance ratio of Ralstonia to Acinetobacter was used in the study of the significance of the change of microbiota in the occurrence and development of lung adenocarcinoma rather than in the prediction of the type of lesion.
Data availability statement
The original contributions presented in the study are included in the article/supplementary material, further inquiries can be directed to the corresponding authors.
Ethics statement
The studies involving human participants were reviewed and approved by Ethics Committee of Affiliated Hospital of Jiangnan University (Ethics No. 201889) and registered on the Chinese Clinical Trial Register as ChiCTR2000031192. The patients/participants provided their written informed consent to participate in this study.
Author contributions
FZ, HC conceived the studies of pulmonary microbiota in lung cancer, designed experiments and wrote the manuscript; JL collected samples and analyzed the data; GW performed 16S rRNA gene sequencing analysis and wrote the manuscript; QW, JY, GW, BG, FC performed statistical analysis; DL, JAY, YX, JS, J.Z helped analyze the data; All authors reviewed, revised and approved the manuscript for submission.
Funding
This work was supported by the National Natural Science Foundation of China (81870544 and 81870594); the Natural Science Foundation of Jiangsu Province (BK20181132, BK20210060, and 1SBK2022023007); the Scientific Research Project of Jiangsu Commission of Health (M2021055); the funding for Leading Talents in Medical and Health Profession in Wuxi Taihu Lake Talent Plan (BK20221204); the Science and Technology Program Project of Jiangsu Market Supervision and Administration (KJ2022028); the Jiangsu Scientific Research Project of Elderly Health (LK2021035); the Jiangsu Scientific Research Project of Women’s and Children’s Health (F201741); the Scientific Research Project of Wuxi Commission of Health (ZZ003 and Q201762); the Scientific Research Project of Wuxi Commission of Health and Family Planning (Q201762); Wuxi Scientific and Technological Development Project (N20192024, N20191001, and Y20212001); and the Translational Medicine Research Program of Wuxi Translational Medicine Center (2020ZHYB08).
Conflict of interest
The authors declare that the research was conducted in the absence of any commercial or financial relationships that could be construed as a potential conflict of interest.
Publisher’s note
All claims expressed in this article are solely those of the authors and do not necessarily represent those of their affiliated organizations, or those of the publisher, the editors and the reviewers. Any product that may be evaluated in this article, or claim that may be made by its manufacturer, is not guaranteed or endorsed by the publisher.
References
1. Raz DJ, Sun V, Kim JY, Williams AC, Koczywas M, Cristea M, et al. Long-term effect of an interdisciplinary supportive care intervention for lung cancer survivors after surgical procedures. Ann Thorac Surg (2016) 101(2):495–502. doi: 10.1016/j.athoracsur.2015.07.031
2. Mattson ME, Pollack ES, Cullen JW. What are the odds that smoking will kill you? Am J Public Health (1987) 77(4):425–31. doi: 10.2105/ajph.77.4.425
3. Alberg AJ, Brock MV, Ford JG, Samet JM, Spivack SD. Epidemiology of lung cancer: diagnosis and management of lung cancer, 3rd Ed: American college of chest physicians evidence-based clinical practice guidelines. Chest (2013) 143(5 Suppl):e1S–e29S. doi: 10.1378/chest.12-2345
4. Nasim F, Sabath BF, Eapen GA. Lung cancer. Med Clin North Am (2019) 103(3):463–73. doi: 10.1016/j.mcna.2018.12.006
5. Stapelfeld C, Dammann C, Maser E. Sex-specificity in lung cancer risk. Int J Cancer (2020) 146(9):2376–82. doi: 10.1002/ijc.32716
6. Doud M, Zeng E, Schneper L, Narasimhan G, Mathee K. Approaches to analyse dynamic microbial communities such as those seen in cystic fibrosis lung. Hum Genomics (2009) 3(3):246–56. doi: 10.1186/1479-7364-3-3-246
7. Lee SH, Sung JY, Yong D, Chun J, Kim SY, Song JH, et al. Characterization of microbiome in bronchoalveolar lavage fluid of patients with lung cancer comparing with benign mass like lesions. Lung Cancer (Amsterdam Netherlands) (2016) 102:89–95. doi: 10.1016/j.lungcan.2016.10.016
8. Hosgood HD 3rd, Sapkota AR, Rothman N, Rohan T, Hu W, Xu J, et al. The potential role of lung microbiota in lung cancer attributed to household coal burning exposures. Environ Mol Mutagen (2014) 55(8):643–51. doi: 10.1002/em.21878
9. Dickson RP, Erb-Downward JR, Huffnagle GB. Towards an ecology of the lung: new conceptual models of pulmonary microbiology and pneumonia pathogenesis. Lancet Respir Med (2014) 2(3):238–46. doi: 10.1016/s2213-2600(14)70028-1
10. Greathouse KL, White JR, Vargas AJ, Bliskovsky VV, Beck JA, von Muhlinen N, et al. Interaction between the microbiome and Tp53 in human lung cancer. Genome Biol (2018) 19(1):123. doi: 10.1186/s13059-018-1501-6
11. Bingula R, Filaire E, Molnar I, Delmas E, Berthon JY, Vasson MP, et al. Characterisation of microbiota in saliva, bronchoalveolar lavage fluid, non-malignant, peritumoural and tumour tissue in non-small cell lung cancer patients: a cross-sectional clinical trial. Respir Res (2020) 21(1):129. doi: 10.1186/s12931-020-01392-2
12. Liu C, Zhao D, Ma W, Guo Y, Wang A, Wang Q, et al. Denitrifying sulfide removal process on high-salinity wastewaters in the presence of halomonas sp. Appl Microbiol Biotechnol (2016) 100(3):1421–6. doi: 10.1007/s00253-015-7039-6
13. Chen S, Zhou Y, Chen Y, Gu J. Fastp: an ultra-fast all-in-One fastq preprocessor. Bioinformatics (2018) 34(17):i884–i90. doi: 10.1093/bioinformatics/bty560
14. Magoc T, Salzberg SL. Flash: fast length adjustment of short reads to improve genome assemblies. Bioinformatics (2011) 27(21):2957–63. doi: 10.1093/bioinformatics/btr507
15. Edgar RC. Uparse: highly accurate otu sequences from microbial amplicon reads. Nat Methods (2013) 10(10):996–8. doi: 10.1038/nmeth.2604
16. Wang Q, Garrity GM, Tiedje JM, Cole JR. Naive Bayesian classifier for rapid assignment of rrna sequences into the new bacterial taxonomy. Appl Environ Microbiol (2007) 73(16):5261–7. doi: 10.1128/AEM.00062-07
17. Davis NM, Proctor DM, Holmes SP, Relman DA, Callahan BJ. Simple statistical identification and removal of contaminant sequences in marker-gene and metagenomics data. Microbiome (2018) 6(1):226. doi: 10.1186/s40168-018-0605-2
18. Douglas GM, Maffei VJ, Zaneveld JR, Yurgel SN, Brown JR, Taylor CM, et al. Picrust2 for prediction of metagenome functions. Nat Biotechnol (2020) 38(6):685–8. doi: 10.1038/s41587-020-0548-6
19. Jungnickel C, Schmidt LH, Bittigkoffer L, Wolf L, Wolf A, Ritzmann F, et al. Il-17c mediates the recruitment of tumor-associated neutrophils and lung tumor growth. Oncogene (2017) 36(29):4182–90. doi: 10.1038/onc.2017.28
20. Helicobacter and Cancer Collaborative Group. Gastric cancer and helicobacter pylori: a combined analysis of 12 case control studies nested within prospective cohorts. Gut (2001) 49(3):347–53. doi: 10.1136/gut.49.3.347
21. Liu L, Li Y, Zhang X, Zhang H. The correlation of the mir-29a/Mmp9 axis with helicobacter pylori infection in gastric cancer. Am J Transl Res (2021) 13(9):10155–62.
22. Mima K, Nishihara R, Qian ZR, Cao Y, Sukawa Y, Nowak JA, et al. Fusobacterium nucleatum in colorectal carcinoma tissue and patient prognosis. Gut (2016) 65(12):1973–80. doi: 10.1136/gutjnl-2015-310101
23. Schreiber H, Nettesheim P, Lijinsky W, Richter C, Walburg H. Induction of lung cancer in germfree, specific-Pathogen-Free, and infected rats by n-nitrosoheptamethyleneimine: enhancement by respiratory infection. JJotNCI (1972) 49(4):1107–14. doi: 10.1093/jnci/49.4.1107
24. Jin J, Gan Y, Liu H, Wang Z, Yuan J, Deng T, et al. Diminishing microbiome richness and distinction in the lower respiratory tract of lung cancer patients: a multiple comparative study design with independent validation. Lung Cancer (2019) 136:129–35. doi: 10.1016/j.lungcan.2019.08.022
25. Huang D, Su X, Yuan M, Zhang S, He J, Deng Q, et al. The characterization of lung microbiome in lung cancer patients with different clinicopathology. Am J Cancer Res (2019) 9(9):2047–63.
26. Peters BA, Hayes RB, Goparaju C, Reid C, Pass HI, Ahn J. The microbiome in lung cancer tissue and recurrence-free survival. Cancer Epidemiol Biomarkers Prev (2019) 28(4):731–40. doi: 10.1158/1055-9965.Epi-18-0966
27. Tseng C, Lin J, Ho H, Lai Z, Wang C, Tang S, et al. Gastric microbiota and predicted gene functions are altered after subtotal gastrectomy in patients with gastric cancer. Sci Rep (2016) 6:20701. doi: 10.1038/srep20701
28. Lee H, Lee H, Min S, Lee W. 16s rdna microbiome composition pattern analysis as a diagnostic biomarker for biliary tract cancer. JWjoso (2020) 18(1):19. doi: 10.1186/s12957-020-1793-3
29. Alberg AJ, Ford JG, Samet JM. Epidemiology of lung cancer: accp evidence-based clinical practice guidelines (2nd edition). Chest (2007) 132(3 Suppl):29s–55s. doi: 10.1378/chest.07-1347
30. Engels EA. Inflammation in the development of lung cancer: epidemiological evidence. Expert Rev Anticancer Ther (2008) 8(4):605–15. doi: 10.1586/14737140.8.4.605
31. Mantovani A, Allavena P, Sica A, Balkwill F. Cancer-related inflammation. Nature (2008) 454(7203):436–44. doi: 10.1038/nature07205
32. Sionov RV, Fridlender ZG, Granot Z. The multifaceted roles neutrophils play in the tumor microenvironment. Cancer Microenviron (2015) 8(3):125–58. doi: 10.1007/s12307-014-0147-5
33. Wang S, Yang Y, Luo D, Wu D, Liu H, Li M, et al. Lung inflammation induced by exposure to bisphenol-a is associated with mtor-mediated autophagy in adolescent mice. Chemosphere (2020) 248:126035. doi: 10.1016/j.chemosphere.2020.126035
34. Lee HJ, Jeong SE, Lee S, Kim S, Han H, Jeon CO. Effects of cosmetics on the skin microbiome of facial cheeks with different hydration levels. MicrobiologyOpen (2018) 7(2):e00557. doi: 10.1002/mbo3.557
35. Ryan MP, Pembroke JT, Adley CC. Ralstonia pickettii in environmental biotechnology: potential and applications. J Appl Microbiol (2007) 103(4):754–64. doi: 10.1111/j.1365-2672.2007.03361.x
36. Cheng C-C, Wooten J, Gibbs ZA, McGlynn K, Mishra P, Whitehurst AW. Sperm-specific Cox6b2 enhances oxidative phosphorylation, proliferation, and survival in human lung adenocarcinoma. eLife (2020) 9. doi: 10.7554/eLife.58108
37. Lin S, Huang C, Gunda V, Sun J, Chellappan SP, Li Z, et al. Fascin controls metastatic colonization and mitochondrial oxidative phosphorylation by remodeling mitochondrial actin filaments. Cell Rep (2019) 28(11):2824–36 e8. doi: 10.1016/j.celrep.2019.08.011
38. Tan JL, Li F, Yeo JZ, Yong KJ, Bassal MA, Ng GH, et al. New high-throughput screening identifies compounds that reduce viability specifically in liver cancer cells that express high levels of Sall4 by inhibiting oxidative phosphorylation. Gastroenterology (2019) 157(6):1615–29 e17. doi: 10.1053/j.gastro.2019.08.022
39. Lissanu Deribe Y, Sun Y, Terranova C, Khan F, Martinez-Ledesma J, Gay J, et al. Mutations in the Swi/Snf complex induce a targetable dependence on oxidative phosphorylation in lung cancer. Nat Med (2018) 24(7):1047–57. doi: 10.1038/s41591-018-0019-5
40. Coman I, Bilodeau L, Lavoie A, Carricart M, Tremblay F, Zlosnik J, et al. Ralstonia mannitolilytica in cystic fibrosis: a new predictor of worse outcomes. Med Case Rep (2017) 20:48–50. doi: 10.1016/j.rmcr.2016.11.014
Keywords: adenocarcinoma, lung cancer, pulmonary microbiota, ralstonia, squamous cell carcinoma
Citation: Li J, Wu G, Yang J, Yan J, Li D, Wang Q, Xia Y, Zhu J, Guo B, Cheng F, Sun J, Cao H and Zhang F (2023) Pulmonary microbiota signatures adjacent to adenocarcinoma, squamous cell carcinoma and benign lesion. Front. Oncol. 13:1163359. doi: 10.3389/fonc.2023.1163359
Received: 10 February 2023; Accepted: 10 May 2023;
Published: 09 June 2023.
Edited by:
Cecilia Ana Suarez, National Scientific and Technical Research Council (CONICET), ArgentinaReviewed by:
Man Liu, Huazhong University of Science and Technology, ChinaXiaodong Zhang, Wenzhou Medical University, China
Copyright © 2023 Li, Wu, Yang, Yan, Li, Wang, Xia, Zhu, Guo, Cheng, Sun, Cao and Zhang. This is an open-access article distributed under the terms of the Creative Commons Attribution License (CC BY). The use, distribution or reproduction in other forums is permitted, provided the original author(s) and the copyright owner(s) are credited and that the original publication in this journal is cited, in accordance with accepted academic practice. No use, distribution or reproduction is permitted which does not comply with these terms.
*Correspondence: Feng Zhang, fengzhang@jiangnan.edu.cn; Hong Cao, HongCao@jiangnan.edu.cn
†These authors have contributed equally to this work and share first authorship
‡These authors have contributed equally to this work
§ORCID: Feng Zhang, orcid.org/0000-0002-1401-9728