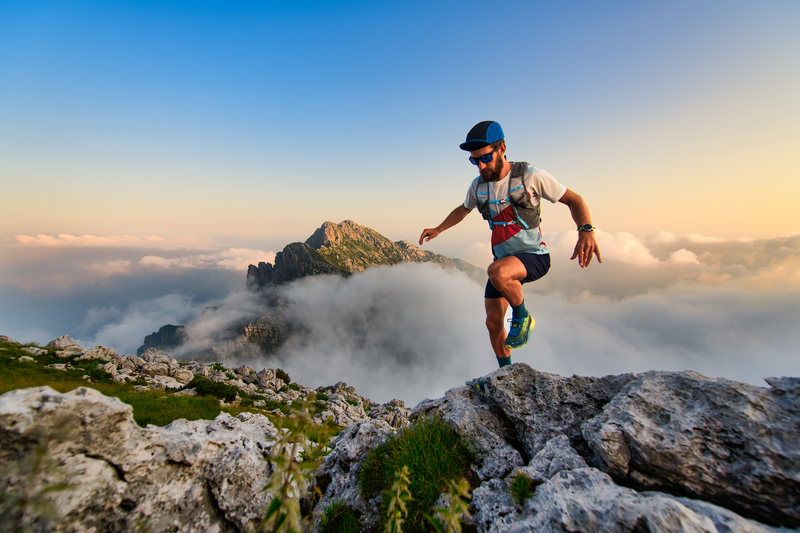
95% of researchers rate our articles as excellent or good
Learn more about the work of our research integrity team to safeguard the quality of each article we publish.
Find out more
MINI REVIEW article
Front. Oncol. , 17 May 2023
Sec. Neuro-Oncology and Neurosurgical Oncology
Volume 13 - 2023 | https://doi.org/10.3389/fonc.2023.1163289
Glioma is one of the most common malignant primary brain tumours in adults, of which, glioblastoma is the most prevalent and malignant entity. Glioma is often diagnosed at a later stage of disease progression, which means it is associated with significant mortality and morbidity. Therefore, there is a need for earlier diagnosis of these tumours, which would require sensitive and specific biomarkers. These biomarkers could better predict glioma onset to improve diagnosis and therapeutic options for patients. While liquid biopsies could provide a cheap and non-invasive test to improve the earlier detection of glioma, there is little known on pre-diagnostic biomarkers which predate disease detection. In this review, we examine the evidence in the literature for pre-diagnostic biomarkers in glioma, including metabolomics and proteomics. We also consider the limitations of these approaches and future research directions of pre-diagnostic biomarkers for glioma.
Glioma is the most common (~75%) group of primary brain tumours in adults with an overall survival of less than 20% over 5 years, and therefore there is a need to better identify biomarkers for prediction and to support early diagnosis (1). Diagnosis can be difficult as many glioma patients present with non-specific symptoms such as headaches (2). Currently the gold standard test for identifying glioma is magnetic resonance imaging (MRI) and computerized tomography (CT) scans, however these tests cause significant burden to the NHS in terms of cost and long wait times (2). Liquid biopsies such as a blood test could be used as a tool to triage patients for further scans (2). Moreover, a liquid biopsy could have utility for disease monitoring and prediction of disease recurrence (3).
Previous literature has identified putative predictive factors which are associated with glioma, some of these factors include atopic disease and diabetes (4–6). A number of nested case-control studies have attempted to define pre-diagnostic biomarkers for glioma; some of which are informed by putative factors and others carry out a hypothesis-free investigation. However, in some instances it can be difficult to be establish whether the pre-diagnostic biomarker truly predates the onset of glioma or if it represents a change seen earlier in the natural history of disease. In this review we consider any pre-diagnostic biomarker, including proteins and metabolites, which is identified before glioma diagnosis.
Proteomics characterise the global protein landscape within liquid biopsies. Protein alterations linked to cancer could provide novel biomarkers for monitoring disease and early detection of cancer (7). Studies measuring protein marker levels in pre-diagnostic glioma patients compared to control individuals could inform pre-diagnostic biomarker discovery (Figure 1). A number of pre-diagnostic protein biomarkers have been suggested to associate with glioma, these are outlined and considered below (Table 1).
Figure 1 Biomarkers investigated in pre-diagnostic blood of glioma patients. Created with BioRender.com.
Table 1 Pre-diagnostic biomarkers associated (p-value < 0.05) with glioma risk at any time point against controls.
Epidermal growth factor receptor (EGFR) is an oncogene implicated in glioma initiation, tumour growth and progression (18, 19). Späth et al. measured pre-diagnostic serum levels of EGFR and human epidermal growth factor receptor 2 (HER2) in glioma patients. EGFR (Odds Ratio (OR)=1.58, 95% confidence interval (95% CI)=1.13-2.22) and HER2 (OR=1.63, 95% CI=1.09-2.44) were found to be elevated in serum samples with an increased risk of glioblastoma development. However only elevated HER2 levels (OR=1.39, 95% CI=1.00-1.93) were found to be associated with increased glioma risk (10). Insulin-like growth factor (IGF-1) has similar downstream signaling pathways to EGF (20). Some studies found no evidence of insulin-like growth factor 1 (IGF-I), insulin-like growth factor binding protein 1 (IGFBP1), IGFBP2, IGFBP-3, or IGF-I/IGFBP3 ratio being associated with glioma risk (9, 12, 21, 22). However, Lönn et al. found conflicting evidence with IGF-I/IGFBP-3 ratio found to be associated with glioma risk, although the sample size was small (9).
Schwartzbaum and colleagues investigated other growth factors from different pathways, with transforming growth factor-beta 2 (TGFB2) found to be inversely related to glioblastoma (OR=0.87, 95% CI=0.76-0.98) in the Janus Serum Bank (JSB) (11). Although, this relationship was not identified in all glioma cases as evidenced in other studies (11, 12). Increased circulating vascular endothelial growth factor (VEGF) was found to be associated with pre-diagnostic glioma (OR=1.46, 95% CI=1.18-1.82) in the JSB, whilst two other studies did not find the same relationship within the Department of Defense Serum Repository (DoDSR) and Northern Sweden and Disease Study cohort (NSHDS) cohorts (8, 12, 13). Increased levels of soluble vascular endothelial growth factor receptor 2 (sVEGFR2) was found to be associated with increased glioma risk (OR=2.44, 95% CI=1.29-4.61) (13). No associations were found between placental growth factor (PLGF), hepatocyte growth factor (HGF), transforming growth factor beta 1 (TGFβ1), fibroblast growth factor 2 (FGF2) and transforming growth factor alpha (TGFα) levels and pre-diagnostic glioma patients (8, 12, 13).
Many epidemiological studies have suggested that allergic and atopic conditions have an inverse relationship with glioma risk (5, 23). Most of these papers examine the association between immunoglobulin E (IgE) antibodies which have a role in type I immediate allergic response (24). Schlehofer et al. investigated atopic status through IgE levels in pre-diagnostic glioma cases’ serum versus controls in the European Prospective Investigation into Cancer and Nutrition. High-grade gliomas were found to have a borderline association with IgE levels, with the greater intensity of the IgE, the lower the OR. Interestingly, only women were found to have an inverse association between allergic sensitization (via IgE levels) and all glioma risk, this association was stronger in high-grade gliomas (25). Schwartzbaum et al. identified the same pronounced association between elevated levels of IgE and glioma risk also observed within women in the JSB. This study also observed an association between testing positive for total IgE and a decreased risk of glioma at least 20 years before diagnosis (26). Conversely, Calboli and colleagues did not find an association between elevated IgE levels and risk of glioma, but there was suggestive evidence of an association in the Nurses’ Health Study, Women’s Health Study (WHS), HPFS, and Physicians’ Health Study (PHS) cohort (27). A receptor for IgE, soluble cluster of differentiation 23 (sCD23), was also investigated but no relationship was found with pre-diagnostic glioma in the JSB and NSHDS cohort (12, 13).
Previous literature has postulated an inverse relationship between immune response derived from a virus. These include a history of chickenpox, shingles, and herpes virus and glioma risk (28–30). Sjöström et al. examined immunoglobulin G (IgG) antibodies for cytomegalovirus, varicella-zoster virus (VZV), adenovirus, and Epstein-Barr virus in the NSHDS, Malmö Diet and Cancer Study (MDCS), and the Diet, Cancer, and Health cohort from Copenhagen. While no overall associations were discovered between IgG levels for the viruses and pre-diagnostic glioma, an inverse relationship was found between VZV IgG levels and pre-diagnostic glioma more than two years before diagnosis, and also an inverse association between positive VZV IgG levels and glioblastoma risk (31). However, Brenner et al. identified no relationship between glioma and prior immune-related conditions (this included any allergy, autoimmune disease or a combination) (8).
Allergy related transcription factors were investigated to identify potential links in pre-diagnostic disease of glioma in the JSB. Elevated beta-Catenin levels in serum were found to be associated with pre-diagnostic glioma (OR=1.86, 95% CI=1.28-2.71) (12). However, no other associations with pre-diagnostic glioma were found in any of the other allergy related transcription factors that were investigated (forkhead box p3 (FOXP3), signal transducer and activator of transcription 3 (STAT3) and STAT6) (11, 12).
Interleukins (ILs) have key roles in the development, progression, and control of cancer (32). Brenner at al. found increased levels of IL15 and IL16 to be inversely associated with glioma risk in pre-diagnostic individuals in the DoDSR (8). However another study did not find this same association in the JSB (12). Schwartzbaum and colleagues found pre-diagnostic glioma to be associated with increased IL4 levels (OR=1.13, 95% CI=0.90-1.43) in the JSB (12). In their previous paper there was only a suggestive relationship between IL4 and risk of glioblastoma, however this was with decreased levels with IL4 less than or equal to 5 years before diagnosis (11). Studies from Schwartzbaum and Wu et al. also investigated the relationship between other ILs and pre-diagnostic glioma cases; however, these were found not to be associated in the JSB and NSHDS (11–13).
Soluble interleukin receptors have also been investigated to identify their relationship with pre-diagnostic glioma. Schwartzbaum and colleagues found an association with reduced soluble interleukin 4 receptor alpha (sIL4RA) (OR=0.92, 95% CI=0.76-1.12) and elevated IL4-sIL4RA (OR=1.37, 95% CI=1.16-1.61) with increased risk of glioma in the JSB (12). This evidence is also suggested in their previous paper using the same cohort with sIL4RA (OR=0.80, 95% CI=0.65-1.00) and IL4-sIL4RA (OR=1.02, 95% CI=1.01-1.04) (11). Elevated levels of sIL2RA (OR=1.48, 95% CI=1.01-2.16) and sIL6R (OR=1.90, 95% CI=1.14-3.17) was suggested to be associated with glioma risk in the NSHDS, but this relationship was not significant after multiple testing and was not consistent in the JSB (12, 13). Decreased levels of sIL10RB (OR=0.69, 95% CI=0.55-0.87) and Leukemia inhibitory factor (LIF) (OR=0.47, 95% CI=0.23-0.94) was associated with glioma 5 or less years before diagnosis (12). SIL13RA2 and IL12p40 were found to have no relationship with glioma risk in two separate cohorts (8, 11, 12).
Chemokines are small, secreted proteins that make up one of the largest families of cytokines, and have critical roles in immune function (33). Schwartzbaum et al. identified the only pre-diagnostic chemokine which was C-C motif chemokine 22 (OR=1.45, 95% CI=1.07-1.96 and associated with glioma risk greater than 15 years before diagnosis) in the JSB (12). The other pre-diagnostic chemokines analysed showed no association with glioma, these included monocyte chemoattractant protein 1, thymus and activation regulated chemokine, these were assessed in the JSB or DoDSR cohorts (8, 12). Similarly, monocyte chemoattractant protein 3, macrophage inflammatory protein 1 alpha and beta, fractalkine and chemokine (C-X-C motif) ligand 13 were assessed in JSB and NSHDS with no association (12, 13).
Interferons (IFNs) are a type of cytokine which have been implicated in cancer progression (34). However, to date no association has been found with pre-diagnostic glioma. Brenner and Schwartzbaum et al. investigated interferons in the DoDSR and JSB. Overall, IFN gamma, IFN beta and IFN-alpha/beta receptors were found to have no relationship with glioma risk (8, 11, 12).
Tumour necrosis factors (TNFs) are key regulators in immune and inflammatory response to cancer (35). Only tumour necrosis factor alpha (TNF-α) and TNF receptors have been explored pre-diagnostic bloods of glioma patients. However, no relationship was identified between TNF-α and glioma risk in the JSB and DoDSR (8, 12, 13). Interestingly, while no relationship was found between TNF-α, Schwartzbaum et al. investigated two soluble receptors, soluble tumour necrosis factor receptor 2 (sTNFR2) was found to have an association with glioma risk (OR=1.72, 95% CI=1.01-2.93) but there was no association with sTNFR1 (13). Two soluble molecules in the tumour necrosis receptor family were also studied, sCD27 and sCD30, but no relationship was found with pre-diagnostic glioma in the JSB or NSHDS cohort (12, 13).
Chronic inflammation is well-established driver of carcinogenesis, it can lead to tumour progression and aid metastasis (36, 37). There are multiple biomarkers which signal systemic inflammation, this is usually due to injury or stress in an individual. These include C-reactive protein (CRP), white blood cell count (WBC) and neutrophil to lymphocyte count (NLR) (38–40). Cote and colleagues published the only study, to our knowledge, looking at biomarkers of inflammation in pre-diagnostic glioma. Data obtained from the UK Biobank cohort suggested a borderline relationship between NLR (hazard ratio=1.54, 95% CI=1.00-2.39, p-trend=0.05) and pre-diagnostic glioma. No other relationship was identified between WBC or CRP and glioma risk (22).
Metabolomics is a tool which measures the broad landscape of metabolites within biological samples such as blood, urine, and saliva, and may be detected using techniques such as mass spectrometry and nuclear magnetic resonance (41). An understanding of the pre-diagnostic metabolic signature in liquid biopsies prior to development of cancer compared to control cases could provide insights to novel candidate pre-diagnostic biomarkers. Several pre-diagnostic metabolite markers have been postulated to associate with glioma, which are summarized and discussed below (Figure 1, Table 1).
Previous studies have suggested an inverse relationship between diabetes and glioma risk (6). Schwartzbaum et al. identified a similar inverse association between pre-diagnostic diabetes and glioma risk in the Apolipoprotein-related Mortality Risk (AMORIS) cohort. The same study identified glucose levels in serum and plasma of pre-diagnostic individuals to have an inverse association with glioma risk within the AMORIS and Metabolic Syndrome and Cancer project (Me-Can) cohorts (17). In contrast, Björkblom and colleagues did not find the same association between glucose levels and pre-diagnostic glioblastoma in the JSB (14).
Deficiencies in vitamin A isoforms (including retinol, retinoic acid and retinal) and vitamin D (most abundant form in the blood is 25-hydroxyvitamin D (25(OH)D)) have been evidenced to increase the risk of cancer in individuals. Whereas vitamin E, which comprises of two groups, tocopherols and tocotrienols each with four isomers (α, β, γ and δ) is considered to have an inverse role in cancer prevention (42). A number of vitamin isoforms (25(OH)D, retinol, α-tocopherol, and γ-tocopherol) were assessed in pre-diagnostic glioma within UK Biobank, Nurses’ Health Study, and Health Professionals Follow-Up Study (HPFS) by Yue et al. However, there was no evidence of an association in 25(OH)D in serum, and α-tocopherol, γ-tocopherol and retinol in plasma and glioma risk (43). However, Björkblom et al. identified an association in increased levels of α-tocopherol (OR=1.7, 95% CI=1.0-3.0) and γ-tocopherol (OR=2.1, 95% CI=1.2-3.8) related to glioblastoma risk in the JSB (14). Alternatively, Huang et al. reported decreased levels of α-tocopherol (OR=0.65, 95% CI=0.44-0.96) associated with glioma risk in the Alpha-Tocopherol, Beta-Carotene Cancer Prevention (ATBC) study.
The amino acid tryptophan is metabolized through the kynurenine pathway in more than 90% of cases and the downstream products of kynurenine pathway have been implicated in carcinogenesis (44). Samanic et al. investigated the levels of tryptophan, kynurenine, and the ratio of kynurenine to tryptophan derived from plasma in pre-diagnostic glioma cases but found no association with circulating plasma in glioma risk within the Nurses’ Health Study and HPFS (45). Similarly, no association was found between tryptophan and glioblastoma risk in the JSB (14).
Björkblom et al. investigated 180 small molecular compounds in pre-diagnostic glioblastoma cases compared to matched control individuals in the JSB. This study found elevated levels of γ-tocopherol (OR=2.1, 95% CI=1.2-3.8), α-tocopherol (OR=1.7, 95% CI=1.0-3.0), 2-keto-L-gluconic acid, erythritol, N-acetyl-L-alanine, xylose and erythronic acid to be associated with glioblastoma risk (14). Jonsson et al. carried out a similar study investigating 142 metabolites in a different cohort of pre-diagnostic glioma patients compared to controls in the NSHDS. This study identified elevated plasma levels of myo-inositol, scyllo-inositol, cysteine, glycine, glyceric acid, aceturic acid, phosphate, proline, 4-hydroxyphenylacetic acid, erythronic acid, erythritol, N-acetylglucosamine, creatinine, uric acid and urea to be associated with glioma risk (16). Interestingly, both studies found elevated levels of erythronic acid (P-value: 0.0043 and 0.0391) and erythritol (P-value: 0.013 and 0.0221) related to an increased glioma risk in pre-diagnostic liquid biopsies within the JSB and NSHDS. Huang et al. performed an analysis on 730 known metabolites in pre-diagnostic glioma patients and controls in the ATBC study. In this study a total of 43 metabolites were found to be associated with overall glioma risk before diagnosis. The strongest associations were found between lower levels of 2-oxoarginine (OR=0.56, 95% CI=0.37-0.85) and cysteine (OR=0.39, 95% CI=0.19-0.77) with overall glioma risk and decreased glycocholenate sulfate (OR=0.39, 95% CI=0.19-0.79) and methyl 4-hydroxybenzoate sulfate (OR=0.47, 95% CI=0.26-0.83) with high-grade glioma risk. The other evidenced associations between metabolites and glioma risk have been noted in Table 1 (15). Notably, in two separate cohorts (ATBC study and NSHDS) cysteine was found to be associated with glioma risk (P-value: 0.0069 and 0.014) (15, 16).
The progression of biomarker analysis through multi-omics technologies has allowed novel protein and metabolite biomarkers to be discovered in liquid biopsies (46). Despite common practice of these techniques in biomarker research, there are still some limitations to the use of metabolomics and proteomics platforms.
Firstly, within metabolomics and proteomics there are multiple platforms and assays to detect biomarkers in liquid biopsies. Depending on the method there are different limitations based on sensitivity, reproducibility, or cost of equipment which need to be considered in biomarker analyses (46, 47).
There are some protein isoforms and metabolites which are present in low abundance, so it can be much harder to detect using current technology. The presence of abnormal/malfunctioning proteins in the body tend to be degraded, which means these proteins are also not measured in proteomic analyses (46).
Metabolites and proteins are dynamic molecules which tend to be difficult to detect due to their complex and changing structures. Because of this, it is unfeasible to accurately measure the entire metabolome and metabolite analyses that are carried out require huge amounts of data (46, 48, 49). Protein and metabolite levels can alter in the body day to day, and throughout the life course depending on many factors including age and lifestyle (50). As this review only found publications using a nested case-control study design, most of the liquid biopsies were taken at a single time point only providing a snapshot of the dynamic molecules at that specific time.
In the current literature, metabolomic and proteomic studies investigating pre-diagnostic biomarkers of glioma have only been carried out in the blood (51). Further analysis of other bodily fluids remains an area for future exploration. Some of the likely candidates for pre-diagnostic biomarker discovery in glioma patients include cerebrospinal fluid (CSF) and urine as these liquid biopsies have previously identified potential biomarkers in glioma (52–54). CSF is more likely to show higher levels of central nervous system (CNS) specific biomarkers due to its proximity to the brain, but to obtain the biopsy this requires an invasive procedure which is not routinely performed (54, 55). In contrast, urine is easily accessible and non-invasive, however it may yield much lower levels of CNS specific biomarkers due to proximity, the blood-brain barrier and glomerular filtration (52, 54, 56, 57). Other bodily fluids which could be considered for biomarker discovery include saliva, stool, and breath (58–60). All of these liquid biopsies could be utilized to discover novel pre-diagnostic biomarkers for glioma or to validate markers that have been suggested in this review.
Additionally, there are many other analytes which can be detected in liquid biopsies and utilized in biomarker discovery. These include microRNA, extracellular vesicles, cell-free RNA, circulating tumour cells, and circulating tumour DNA (61–65). However, the potential of these analytes in the early detection of cancer is unknown (66). Some of these suggested analytes may only be detected in a later stage of glioma progression and therefore may not be suitable as pre-diagnostic markers.
Glioma is a devasting cancer with survival rates less than 20% over 5 years. It is often diagnosed at a late stage of disease progression and usually leads to significant mortality and morbidity. Currently little is known about pre-diagnostic biomarkers which predate glioma detection, but this could improve the earlier detection of glioma. To our knowledge, this review outlines all of the current literature examining studies where biomarkers were assessed pre-diagnosis. Limitations of metabolomics and proteomics in biomarker detection were considered and the future directions for the discovery and validation of pre-diagnostic biomarker for glioma were suggested.
LA was responsible for article conception, article structure, creating figures and tables, and drafting the manuscript. KK was responsible for drafting the article abstract and providing critical revision of the manuscript. PD and CH provided revisions to the manuscript. All authors contributed to the article and approved of the submitted version.
LA and KK is funded by Cancer Research UK [grant number C30758/A29791]. KK is funded by Innovate [grant number 10027624]. This work was supported by Cancer Research UK [grant number C18281/A29019 and C18281/A30905].
The authors declare that the research was conducted in the absence of any commercial or financial relationships that could be construed as a potential conflict of interest.
All claims expressed in this article are solely those of the authors and do not necessarily represent those of their affiliated organizations, or those of the publisher, the editors and the reviewers. Any product that may be evaluated in this article, or claim that may be made by its manufacturer, is not guaranteed or endorsed by the publisher.
1. Ostrom QT, Gittleman H, Liao P, Vecchione-Koval T, Wolinsky Y, Kruchko C, et al. CBTRUS statistical report: primary brain and other central nervous system tumors diagnosed in the united states in 2010–2014. Neuro-oncology (2017) 19(suppl_5):v1–v88. doi: 10.1093/neuonc/nox158
2. Gray E, Butler HJ, Board R, Brennan PM, Chalmers AJ, Dawson T, et al. Health economic evaluation of a serum-based blood test for brain tumour diagnosis: exploration of two clinical scenarios. BMJ Open (2018) 8(5):e017593. doi: 10.1136/bmjopen-2017-017593
3. Mattox AK, Bettegowda C, Zhou S, Papadopoulos N, Kinzler KW, Vogelstein B. Applications of liquid biopsies for cancer. Sci Trans Med (2019) 11(507):eaay1984. doi: 10.1126/scitranslmed.aay1984
4. Howell AE, Zheng J, Haycock PC, McAleenan A, Relton C, Martin RM, et al. Use of mendelian r andomization for I dentifying r isk f actors for b rain T umors. Front Genet (2018) 9:525. doi: 10.3389/fgene.2018.00525
5. Wiemels JL, Wiencke JK, Sison JD, Miike R, McMillan A, Wrensch M. History of allergies among adults with glioma and controls. Int J cancer (2002) 98(4):609–15. doi: 10.1002/ijc.10239
6. Zhao L, Zheng Z, Huang P. Diabetes mellitus and the risk of glioma: a meta-analysis. Oncotarget (2016) 7(4):4483. doi: 10.18632/oncotarget.6605
7. Shruthi BS, Vinodhkumar P. Proteomics: a new perspective for cancer. Adv Biomed Res (2016) 5:67. doi: 10.4103/2277-9175.180636
8. Brenner AV, Inskip PD, Rusiecki J, Rabkin CS, Engels J, Pfeiffer RM. Serially measured pre-diagnostic levels of serum cytokines and risk of brain cancer in active component military personnel. Br J Cancer (2018) 119(7):893–900. doi: 10.1038/s41416-018-0272-x
9. Lönn S, Inskip PD, Pollak MN, Weinstein SJ, Virtamo J, Albanes D. Glioma risk in relation to serum levels of insulin-like growth factors. Cancer Epidemiol Biomarkers Prev (2007) 16(4):844–6. doi: 10.1158/1055-9965.EPI-06-1010
10. Späth F, Andersson U, Dahlin AM, Langseth H, Hovig E, Johannesen TB, et al. Pre-diagnostic serum levels of EGFR and ErbB2 and genetic glioma risk variants: a nested case-control study. Tumor Biol (2016) 37(8):11065–72. doi: 10.1007/s13277-015-4742-y
11. Schwartzbaum J, Seweryn M, Holloman C, Harris R, Handelman SK, Rempala GA, et al. Association between prediagnostic allergy-related serum cytokines and glioma. PloS One (2015) 10(9):e0137503. doi: 10.1371/journal.pone.0137503
12. Schwartzbaum J, Wang M, Root E, Pietrzak M, Rempala GA, Huang R-P, et al. A nested case-control study of 277 prediagnostic serum cytokines and glioma. PloS One (2017) 12(6):e0178705. doi: 10.1371/journal.pone.0178705
13. Wu WYY, Späth F, Wibom C, Björkblom B, Dahlin AM, Melin B. Pre-diagnostic levels of sVEGFR2, sTNFR2, sIL-2Rα and sIL-6R are associated with glioma risk: a nested case–control study of repeated samples. Cancer Med (2022) 11(4):1016–25. doi: 10.1002/cam4.4505
14. Björkblom B, Wibom C, Jonsson P, Mörén L, Andersson U, Johannesen TB, et al. Metabolomic screening of pre-diagnostic serum samples identifies association between α-and γ-tocopherols and glioblastoma risk. Oncotarget (2016) 7(24):37043. doi: 10.18632/oncotarget.9242
15. Huang J, Weinstein SJ, Kitahara CM, Karoly ED, Sampson JN, Albanes D. A prospective study of serum metabolites and glioma risk. Oncotarget (2017) 8(41):70366. doi: 10.18632/oncotarget.19705
16. Jonsson P, Antti H, Späth F, Melin B, Björkblom B. Identification of pre-diagnostic metabolic patterns for glioma using subset analysis of matched repeated time points. Cancers (2020) 12(11):3349. doi: 10.3390/cancers12113349
17. Schwartzbaum J, Edlinger M, Zigmont V, Stattin P, Rempala GA, Nagel G, et al. Associations between prediagnostic blood glucose levels, diabetes, and glioma. Sci Rep (2017) 7(1):1–9. doi: 10.1038/s41598-017-01553-2
18. Zhu H, Acquaviva J, Ramachandran P, Boskovitz A, Woolfenden S, Pfannl R, et al. Oncogenic EGFR signaling cooperates with loss of tumor suppressor gene functions in gliomagenesis. Proc Natl Acad Sci (2009) 106(8):2712–6. doi: 10.1073/pnas.0813314106
19. Saadeh FS, Mahfouz R, Assi HI. EGFR as a clinical marker in glioblastomas and other gliomas. Int J Biol markers. (2018) 33(1):22–32. doi: 10.5301/ijbm.5000301
20. Zielinski R, Przytycki PF, Zheng J, Zhang D, Przytycka TM, Capala J. The crosstalk between EGF, IGF, and insulin cell signaling pathways-computational and experimental analysis. BMC Syst Biol (2009) 3(1):1–10. doi: 10.1186/1752-0509-3-88
21. Rohrmann S, Linseisen J, Becker S, Allen N, Schlehofer B, Overvad K, et al. Concentrations of IGF-I and IGFBP-3 and brain tumor risk in the European prospective investigation into cancer and NutritionIGF-I, IGFBP-3, and brain tumor risk. Cancer epidemiol Biomarkers Prev (2011) 20(10):2174–82. doi: 10.1158/1055-9965.EPI-11-0179
22. Cote DJ, Creed JH, Samanic CM, Gerke TA, Stampfer MJ, Smith-Warner SA, et al. A prospective study of inflammatory biomarkers and growth factors and risk of glioma in the UK biobank. Cancer Epidemiol (2021) 75:102043. doi: 10.1016/j.canep.2021.102043
23. Linos E, Raine T, Alonso A, Michaud D. Atopy and risk of brain tumors: a meta-analysis. J Natl Cancer Instit (2007) 99(20):1544–50. doi: 10.1093/jnci/djm170
24. Shamji MH, Valenta R, Jardetzky T, Verhasselt V, Durham SR, Würtzen PA, et al. The role of allergen-specific IgE, IgG and IgA in allergic disease. Allergy (2021) 76(12):3627–41. doi: 10.1111/all.14908
25. Schlehofer B, Siegmund B, Linseisen J, Schüz J, Rohrmann S, Becker S, et al. Primary brain tumours and specific serum immunoglobulin e: a case–control study nested in the European prospective investigation into cancer and nutrition cohort. Allergy (2011) 66(11):1434–41. doi: 10.1111/j.1398-9995.2011.02670.x
26. Schwartzbaum J, Ding B, Johannesen TB, Osnes LT, Karavodin L, Ahlbom A, et al. Association between prediagnostic IgE levels and risk of glioma. J Natl Cancer Instit (2012) 104(16):1251–9. doi: 10.1093/jnci/djs315
27. Calboli FC, Cox DG, Buring JE, Gaziano JM, Ma J, Stampfer M, et al. Prediagnostic plasma IgE levels and risk of adult glioma in four prospective cohort studies. J Natl Cancer Instit (2011) 103(21):1588–95. doi: 10.1093/jnci/djr361
28. Wrensch M, Weinberg A, Wiencke J, Masters H, Miike R, Barger G, et al. Does prior infection with varicella-zoster virus influence risk of adult glioma? Am J Epidemiol (1997) 145(7):594–7. doi: 10.1093/oxfordjournals.aje.a009155
29. Wrensch M, Weinberg A, Wiencke J, Miike R, Barger G, Kelsey K. Prevalence of antibodies to four herpesviruses among adults with glioma and controls. Am J Epidemiol (2001) 154(2):161–5. doi: 10.1093/aje/154.2.161
30. Wrensch M, Weinberg A, Wiencke J, Miike R, Sison J, Wiemels J, et al. History of chickenpox and shingles and prevalence of antibodies to varicella-zoster virus and three other herpesviruses among adults with glioma and controls. Am J Epidemiol (2005) 161(10):929–38. doi: 10.1093/aje/kwi119
31. Sjöström S, Hjalmars U, Juto P, Wadell G, Hallmans G, Tjönneland A, et al. Human immunoglobulin G levels of viruses and associated glioma risk. Cancer Causes Control (2011) 22(9):1259–66. doi: 10.1007/s10552-011-9799-3
32. Briukhovetska D, Dörr J, Endres S, Libby P, Dinarello CA, Kobold S. Interleukins in cancer: from biology to therapy. Nat Rev Cancer (2021) 21(8):481–99. doi: 10.1038/s41568-021-00363-z
33. Griffith JW, Sokol CL, Luster AD. Chemokines and chemokine receptors: positioning cells for host defense and immunity. Annu Rev Immunol (2014) 32(1):659–702. doi: 10.1146/annurev-immunol-032713-120145
34. Boukhaled GM, Harding S, Brooks DG. Opposing roles of type I interferons in cancer immunity. Annu Rev Pathol: Mech Disease. (2021) 16:167–98. doi: 10.1146/annurev-pathol-031920-093932
35. Balkwill F. Tumour necrosis factor and cancer. Nat Rev cancer (2009) 9(5):361–71. doi: 10.1038/nrc2628
36. Mantovani A, Allavena P, Sica A, Balkwill F. Cancer-related inflammation. nature (2008) 454(7203):436–44. doi: 10.1038/nature07205
37. Colotta F, Allavena P, Sica A, Garlanda C, Mantovani A. Cancer-related inflammation, the seventh hallmark of cancer: links to genetic instability. Carcinogenesis (2009) 30(7):1073–81. doi: 10.1093/carcin/bgp127
38. Kounis NG, Soufras GD, Tsigkas G, Hahalis G. White blood cell counts, leukocyte ratios, and eosinophils as inflammatory markers in patients with coronary artery disease. Clin Appl Thrombosis/Hemostasis. (2015) 21(2):139–43. doi: 10.1177/1076029614531449
39. Stojkovic Lalosevic M, Pavlovic Markovic A, Stankovic S, Stojkovic M, Dimitrijevic I, Radoman Vujacic I, et al. Combined diagnostic efficacy of neutrophil-to-lymphocyte ratio (NLR), platelet-to-lymphocyte ratio (PLR), and mean platelet volume (MPV) as biomarkers of systemic inflammation in the diagnosis of colorectal cancer. Dis Markers (2019) 2019:6036979. doi: 10.1155/2019/6036979
40. Jain S, Gautam V, Naseem S. Acute-phase proteins: as diagnostic tool. J Pharm Bioallied Sci (2011) 3(1):118. doi: 10.4103/0975-7406.76489
41. Schmidt DR, Patel R, Kirsch DG, Lewis CA, Vander Heiden MG, Locasale JW. Metabolomics in cancer research and emerging applications in clinical oncology. CA: Cancer J Clin (2021) 71(4):333–58. doi: 10.3322/caac.21670
42. Albahrani AA, Greaves RF. Fat-soluble vitamins: clinical indications and current challenges for chromatographic measurement. Clin Biochem Rev (2016) 37(1):27.
43. Yue Y, Creed JH, Cote DJ, Stampfer MJ, Wang M, Midttun Ø, et al. Pre-diagnostic circulating concentrations of fat-soluble vitamins and risk of glioma in three cohort studies. Sci Rep (2021) 11(1):1–8. doi: 10.1038/s41598-021-88485-0
44. Agus A, Planchais J, Sokol H. Gut microbiota regulation of tryptophan metabolism in health and disease. Cell Host Microbe (2018) 23(6):716–24. doi: 10.1016/j.chom.2018.05.003
45. Samanic CM, Yue Y, Cote DJ, Stampfer MJ, Wang M, McCann A, et al. A prospective study of pre-diagnostic circulating tryptophan and kynurenine, and the kynurenine/tryptophan ratio and risk of glioma. Cancer Epidemiol (2022) 76:102075. doi: 10.1016/j.canep.2021.102075
46. Hristova VA, Chan DW. Cancer biomarker discovery and translation: proteomics and beyond. Expert Rev proteomics (2019) 16(2):93–103. doi: 10.1080/14789450.2019.1559062
47. Davis VW, Bathe OF, Schiller DE, Slupsky CM, Sawyer MB. Metabolomics and surgical oncology: potential role for small molecule biomarkers. J Surg Oncol (2011) 103(5):451–9. doi: 10.1002/jso.21831
48. Song Z, Wang H, Yin X, Deng P, Jiang W. Application of NMR metabolomics to search for human disease biomarkers in blood. Clin Chem Lab Med (CCLM). (2019) 57(4):417–41. doi: 10.1515/cclm-2018-0380
49. Monteiro M, Carvalho M, Bastos M, Guedes de Pinho P. Metabolomics analysis for biomarker discovery: advances and challenges. Curr med Chem (2013) 20(2):257–71. doi: 10.2174/092986713804806621
50. Enroth S, Enroth SB, Johansson Å, Gyllensten U. Protein profiling reveals consequences of lifestyle choices on predicted biological aging. Sci Rep (2015) 5(1):1–10. doi: 10.1038/srep17282
51. Wu WY-Y, Dahlin AM, Wibom C, Björkblom B, Melin B. Prediagnostic biomarkers for early detection of glioma–using case–control studies from cohorts as study approach. Neuro-Oncol Adv (2022) 4(Supplement_2):ii73–80. doi: 10.1093/noajnl/vdac036
52. Smith ER, Zurakowski D, Saad A, Scott RM, Moses MA. Urinary biomarkers predict brain tumor presence and response to therapy. Clin Cancer Res (2008) 14(8):2378–86. doi: 10.1158/1078-0432.CCR-07-1253
53. Yoshida J, Wakabayashi T, Okamoto S, Kimura S, Washizu K, Kiyosawa K, et al. Tenascin in cerebrospinal fluid is a useful biomarker for the diagnosis of brain tumour. J Neurol Neurosurg Psychiatry (1994) 57(10):1212–5. doi: 10.1136/jnnp.57.10.1212
54. Loo HK, Mathen P, Lee J, Camphausen K. Circulating biomarkers for high-grade glioma. Future Med (2019) 13(3):161–5. doi: 10.2217/bmm-2018-0463
55. Zetterberg H, Smith DH, Blennow K. Biomarkers of mild traumatic brain injury in cerebrospinal fluid and blood. Nat Rev Neurol (2013) 9(4):201–10. doi: 10.1038/nrneurol.2013.9
56. Kadry H, Noorani B, Cucullo L. A blood–brain barrier overview on structure, function, impairment, and biomarkers of integrity. Fluids Barriers CNS. (2020) 17(1):1–24. doi: 10.1186/s12987-020-00230-3
57. Pisitkun T, Johnstone R, Knepper MA. Discovery of urinary biomarkers. Mol Cell Proteomics (2006) 5(10):1760–71. doi: 10.1074/mcp.R600004-MCP200
58. Xiao H, Wong DT. Proteomics and its applications for biomarker discovery in human saliva. Bioinformation (2011) 7:294. doi: 10.6026/97320630005294
59. Guo Q, Song Y, Zhang H, Wu X, Xia P, Dang C. Detection of hypermethylated fibrillin-1 in the stool samples of colorectal cancer patients. Med Oncol (2013) 30(4):1–5. doi: 10.1007/s12032-013-0695-4
60. Filipiak W, Filipiak A, Sponring A, Schmid T, Zelger B, Ager C, et al. Comparative analyses of volatile organic compounds (VOCs) from patients, tumors and transformed cell lines for the validation of lung cancer-derived breath markers. J breath Res (2014) 8(2):027111. doi: 10.1088/1752-7155/8/2/027111
61. Akers JC, Hua W, Li H, Ramakrishnan V, Yang Z, Quan K, et al. A cerebrospinal fluid microRNA signature as biomarker for glioblastoma. Oncotarget (2017) 8(40):68769. doi: 10.18632/oncotarget.18332
62. Zhang H, Yuan F, Qi Y, Liu B, Chen Q. Circulating tumor cells for glioma. Front Oncol (2021) 11:607150. doi: 10.3389/fonc.2021.607150
63. Zhao Z, Zhang C, Li M, Shen Y, Feng S, Liu J, et al. Applications of cerebrospinal fluid circulating tumor DNA in the diagnosis of gliomas. Japanese J Clin Oncol (2020) 50(3):325–32. doi: 10.1093/jjco/hyz156
64. Wang H, Jiang D, Li W, Xiang X, Zhao J, Yu B, et al. Evaluation of serum extracellular vesicles as noninvasive diagnostic markers of glioma. Theranostics (2019) 9(18):5347. doi: 10.7150/thno.33114
65. Ita MI, Wang JH, Toulouse A, Lim C, Fanning N, O’Sullivan M, et al. The utility of plasma circulating cell-free messenger RNA as a biomarker of glioma: a pilot study. Acta Neurochirurgica (2022) 164(3):723–35. doi: 10.1007/s00701-021-05014-8
Keywords: glioblastoma, glioma, liquid biomarkers, pre-diagnostic, early detection
Citation: Andrews LJ, Davies P, Herbert C and Kurian KM (2023) Pre-diagnostic blood biomarkers for adult glioma. Front. Oncol. 13:1163289. doi: 10.3389/fonc.2023.1163289
Received: 10 February 2023; Accepted: 25 April 2023;
Published: 17 May 2023.
Edited by:
Pankaj Pathak, National Institutes of Health (NIH), United StatesReviewed by:
Vikas Sharma, All India Institute of Medical Sciences, IndiaCopyright © 2023 Andrews, Davies, Herbert and Kurian. This is an open-access article distributed under the terms of the Creative Commons Attribution License (CC BY). The use, distribution or reproduction in other forums is permitted, provided the original author(s) and the copyright owner(s) are credited and that the original publication in this journal is cited, in accordance with accepted academic practice. No use, distribution or reproduction is permitted which does not comply with these terms.
*Correspondence: Lily J. Andrews, bGlseS5hbmRyZXdzQGJyaXN0b2wuYWMudWs=
Disclaimer: All claims expressed in this article are solely those of the authors and do not necessarily represent those of their affiliated organizations, or those of the publisher, the editors and the reviewers. Any product that may be evaluated in this article or claim that may be made by its manufacturer is not guaranteed or endorsed by the publisher.
Research integrity at Frontiers
Learn more about the work of our research integrity team to safeguard the quality of each article we publish.