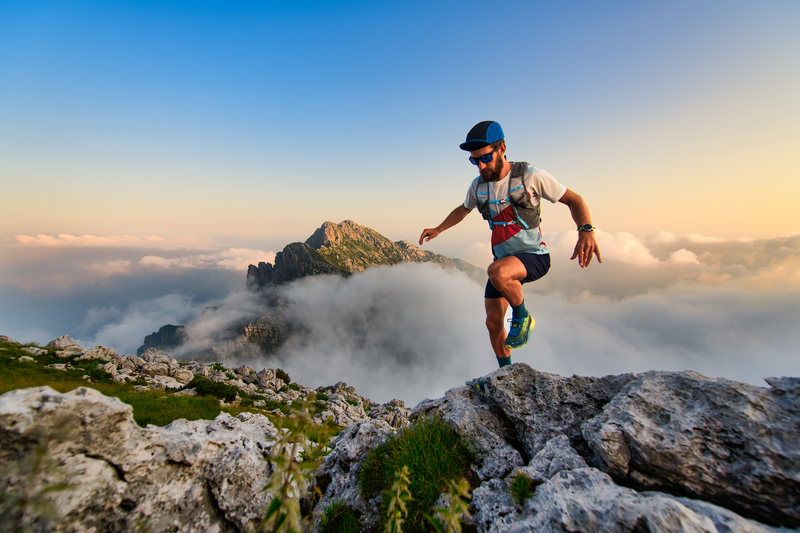
94% of researchers rate our articles as excellent or good
Learn more about the work of our research integrity team to safeguard the quality of each article we publish.
Find out more
ORIGINAL RESEARCH article
Front. Oncol. , 10 November 2023
Sec. Genitourinary Oncology
Volume 13 - 2023 | https://doi.org/10.3389/fonc.2023.1162846
Background: Hypoxia and metabolism are closely correlated with the progression of cancer. We aimed to construct a combined hypoxia- and metabolism-related genes (HMRGs) prognostic signature to predict survival and immunotherapy responses in patients with clear cell renal cell carcinoma (ccRCC).
Methods: The RNA-seq profiles and clinical data of ccRCC were acquired from the TCGA and the ArrayExpress (E-MTAB-1980) databases. Least absolute shrinkage and selection operator (LASSO) and univariate and multivariate Cox regression analyses were applied to establish a prognostic signature. The E-MTAB-1980 cohort was selected for validation. The effectiveness and reliability of the signature were further evaluated by Kaplan–Meier (K-M) survival and time-dependent receiver operating characteristic (ROC) curves. Further analyses, including functional enrichment, ssGSEA algorithm, CIBERSORT algorithm, and expression of immune checkpoints, were explored to investigate immune status and immunotherapy responses.
Results: We constructed a prognostic eight-gene signature with IRF6, TEK, PLCB2, ABCB1, TGFA, COL4A5, PLOD2, and TUBB6. Patients were divided into high-risk and low-risk groups based on the medium-risk score. The K-M analysis revealed that patients in the high-risk group had an apparently poor prognosis compared to those in the low-risk group in the TCGA (p < 0.001) and E-MTAB-1980 (p < 0.005). The area under ROC curve (AUC) of the prognostic signature was 0.8 at 1 year, 0.77 at 3 years, and 0.78 at 5 years in the TCGA, respectively, and was 0.82 at 1 year, 0.74 at 3 years, and 0.75 at 5 years in the E-MTAB-1980, respectively. Independent prognostic analysis confirmed the risk score as a separate prognostic factor in ccRCC patients (p < 0.001). The results of ssGSEA showed not only a high degree of immune cell infiltration but also high scores of immune-related functions in the high-risk group. The CIBERSORT analysis further confirmed that the abundance of immune cells was apparently different between the two risk groups. The risk score was significantly correlated with the expression of cytotoxic T lymphocyte-associated antigen-4 (CTLA4), lymphocyte-activation gene 3 (LAG3), and programmed cell death protein 1 (PD-1).
Conclusion: The HMRGs signature could be used to predict clinical prognosis, evaluate the efficacy of immunotherapy, and guide personalized immunotherapy in ccRCC patients.
Renal cell carcinoma (RCC) is one of the most frequent genitourinary cancers, with an estimated 431,288 newly diagnosed cases and 179,368 deaths occurring in the world in 2020 according to global cancer statistics (1). Clear cell renal cell carcinoma (ccRCC) is the most prevalent subtype among RCCs, representing 75%–85% of all RCC cases (2). Most patients with localized ccRCC can be treated by partial nephrectomy or radical therapy, and overall clinical prognosis is satisfactory. Unfortunately, approximately 30% of patients with localized ccRCC will experience tumor recurrence or metastasis post-operation, which leads to poor prognosis (3). The most common treatment for advanced ccRCC is targeted therapy and immune checkpoint therapy, which significantly improved the prognosis of patients (4–6). However, owing to the high heterogeneity of cancer, some patients are unresponsive or resistant to targeted therapy or immune checkpoint therapy (7, 8), so that the treatment for patients with advanced ccRCC still represents a huge challenge. Thus, in the era of individualized cancer treatment, identifying reliable biomarkers are essential for optimizing risk stratification, providing individualized treatments, and predicting clinical prognosis (9, 10).
Hypoxia, a common and important feature of nearly all solid tumors, is caused by an inadequate oxygen supply due to the tumor uncontrolled growth beyond the existing vasculature (11). The tumor uncontrolled growth beyond the existing vasculature leads to hypoxia occurrence. It is well-known that hypoxia-inducible factors (HIFs) are the master transcription factors for oxygen homeostasis, which could drive the transcription of numerous genes that mediate invasion, metastasis, angiogenesis, epithelial–mesenchymal transition, immune evasion, and therapy resistance (12–14). Moreover, hypoxia mediates a cascade of metabolic reprogramming mostly via HIFs (15, 16).
Tumor metabolism is a hallmark of cancer and plays a significant role in tumor initiation and development. For cancer cells’ characteristic uncontrolled growth and proliferation, cancer cells change metabolic reprogramming to provide increasing energy and nutritional demands (17). Metabolic reprogramming change in TME could lead to immune escape of tumor cells and promote tumor growth (18). Alterations in metabolic reprogramming are closely related to cancer cell proliferation, migration, invasion, angiogenesis, drug resistance, and immune response (19–21). As the main hallmark in TME, both hypoxia and metabolism could influence the cancer cell tumor growth, metastasis and anti-tumor immune response. Hence, it is necessary to construct a robust prognostic model that combine hypoxia- and metabolism-related genes (HMRGs) signature in order to improve risk stratification and predict clinical outcomes for ccRCC.
To evaluate the clinical value of HMRGs in ccRCC, the bioinformatics analysis was performed by constructing an HMRGs prognostic signature in the TCGA and validating it in the E-MTAB-1980 database. Then, the effectiveness and reliability of the risk model were evaluated. In addition, we compared the difference of clinicopathological features, immune cell infiltration, and expression of common immune checkpoints between the high-risk group and low-risk group.
The flowchart of our study is presented in Figure 1.
For training cohort, RNA expression and clinical data of ccRCC cases were downloaded from TCGA (https://genomecancer.ucsc.edu). The E-MTAB-1980 dataset (https://www.ebi.ac.uk/arrayexpress/) was used for the validation cohort. Patients who survived less than 30 days were removed for subsequent analysis.
In this study, 493 hypoxia-related genes and 1,111 metabolism-related genes were acquired from the Molecular Signatures Database (MSigDB; https://www.gsea-msigdb.org/gsea/msigdb/index.jsp) (Supplementary Table 1).
Differentially expressed genes (DEGs) between tumor tissues and normal tissues were analyzed using the package “limma” according to |logFC| > 1 and adjusted p < 0.05. HMRGs were obtained through merging hypoxia-related genes and metabolism-related genes, and deduplication. The differentially expressed HMRGs were identified by intersecting DEGs with HMRGs, and visualizing them by the Venn diagram.
Firstly, a univariate Cox regression analysis was conducted to identify the HMRGs associated with the prognosis in the TCGA. In addition, HMRGs with p < 0.05 were selected into LASSO regression to shrink the scope of prognosis genes’ screening. Subsequently, the candidate prognosis genes identified from LASSO analysis were selected into multivariate Cox regression analysis in order to assess their contribution as prognostic factors. Finally, a prognostic model was established using gene expression level and corresponding regression coefficients. The formula was as follows: risk score = βmRNA1 × ExpressionmRNA1 + βmRNA2 × ExpressionmRNA2 + βmRNA3 × ExpressionmRNA3 + …+ βmRNAn × ExpressionmRNAn.
The patients were classified into the high-risk group and low-risk group based on the medium value of risk score. K-M survival analysis was conducted to compare the overall survival (OS) between the two risk groups. ROC curves were performed to evaluate the prediction efficiency of the risk model.
A predictive nomogram was constructed based on the independent prognostic factors, which included clinical parameters and HMRGs risk score, to predict the OS probability of ccRCC patients. Additionally, the predictive power of the nomogram was evaluated by ROC curves.
To further evaluate the prognostic value of the HMRGs signature prognostic model, stratified analysis was performed in different subgroups based on clinical parameters. Next, the correlation between the risk score and clinicopathological parameters was investigated to better assess the role of the HMRGs signature prognostic model in the ccRCC development.
The package “limma” was conducted to analyze the DEGs between the high-risk group and low-risk group with the criterion set at |logFC| > 1 and adjusted p < 0.05. Then, Gene Ontology (GO) analysis and Kyoto Encyclopedia of Genes and Genomes (KEGG) pathway analysis were conducted by the package “ClusterProfiler” based on DEGs in order to explore the biological function of DEGs.
The ssGSEA algorithm was used to assess the relative abundance of immune cell infiltration between the high-risk group and low-risk group. Then, the CIBERSORT algorithm was applied to explore the infiltration levels of immune cell types. Furthermore, the expression of well-known immune checkpoint genes, including programmed cell death protein 1 (PD-1), programmed cell death protein ligand 1 (PD-L1), cytotoxic T lymphocyte-associated antigen-4 (CTLA4), and lymphocyte-activation gene 3 (LAG3), was compared between the high-risk group and the low-risk group.
Total RNA was extracted from five paired human ccRCC tissues and adjacent non-tumorous tissues using TRIzol Reagent (Invitrogen). The reverse transcription was conducted with PrimeScript™ RT reagent Kit (TaKaRa). Q-PCR was performed using SYBR green Premix Ex Taq II (Takara). GAPDH was selected as an internal control. The sequence of primers is shown in Supplementary Table 2.
Statistical analyses and graphing were conducted using the R software (version R-4.1.2) or GraphPad Prism (version 8.0.2). The Student’s t-test was applied to compare the continuous data between two groups. Correlation coefficients were calculated using Spearman correlation analysis. p-value < 0.05 was considered as statistically significant. p-values were shown as follows: ns, not significant; *p < 0.05; **p < 0.01; ***p < 0.001.
In this study, according to the filtering criteria of the DEGs, 2,276 DEGs were obtained between ccRCC tissues and normal tissues. HMRGs were obtained through merging hypoxia-related genes and metabolism-related genes, and deduplication, and a total of 1,379 HMRGs were included. After intersecting DEGs with HMRGs, a total of 272 differentially expressed HMRGs were included (Figure 2). Then, a univariate Cox regression analysis was performed and a total number of 115 prognosis-related HMRGs were identified (Supplementary Table 3).
Figure 2 The differentially expressed HMRGs between normal and tumor tissue were identified by intersecting DEGs with HMRGs in the TCGA cohort.
The flowchart for the process of constructing the HMRGs prognostic signature is presented in Supplementary Figure 1.
The LASSO regression analysis was performed to screen the key genes (Figures 3A, B). Next, the multivariate Cox regression was conducted to further filter out candidate genes in order to improve the prognostic ability of the model. Finally, eight genes, namely, IRF6, TEK, PLCB2, ABCB1, TGFA, COL4A5, PLOD2, and TUBB6, were selected to construct a prognostic signature. The risk score was calculated as follows: risk score = (−0.158 × the expression level of ABCB1) + (0.378 × the expression level of COL4A5) + (−0.151 × the expression level of IRF6) + (0.319 × the expression level of PLCB2) + (0.136 × the expression level of PLOD2) + (−0.406 × the expression level of TEK) + (−0.163 × the expression level of TGFA) + (0.394 × the expression level of TUBB6).
Figure 3 Construction of the HMRG prognostic signature in the TCGA cohort. (A, B) LASSO Cox regression analysis was performed to screen the key genes. (C) The median value and distribution of the risk score. (D) The distribution of survival status. (E) Expression of eight signature genes. (F) K-M curves for the OS. (G) ROC curve for 1, 3, and 5 years.
The patients were classified as high-risk group and low-risk group based on the median of risk score (Figure 3C). Patients in the high-risk group had a significantly higher mortality rate (Figure 3D). The expression of PLCB2, COL4A5, PLOD2, and TUBB6 was higher in the high-risk group, while the expression of IRF6, TEK, ABCB1, and TGFA were higher in the low-risk group (Figure 3E). Compared to normal tissues, the expression level of PLCB2, TGFA, PLOD2, and TUBB6 was significantly higher in ccRCC tissues, while the expression level of IRF6, TEK, ABCB1, and COL4A5 was significantly lower (Supplementary Figures 2A–H). We performed survival analysis based on the eight genes and found that patients with high expression of identified genes, such as PLCB2, COL4A5, PLOD2, and TUBB6, had a significantly poor survival, while patients with high expression of identified genes, such as IRF6, TEK, ABCB1, and TGFA, had a significantly favorable survival (Supplementary Figures 3A–H). K-M curves indicated that ccRCC samples with a low risk score had a significantly superior prognosis to those with a high risk score (p < 0.001) (Figure 3F). Moreover, the AUC reached 0.8 at 1 year, 0.77 at 3 years, and 0.78 at 5 years (Figure 3G). In general, these results suggested that the HMRGs signature model had a favorable efficacy in predicting the clinical prognosis.
To evaluate the reliability of the HMRGs signature model established from the TCGA cohort, risk scores were measured for the patients from the E-MTAB-1980 cohort with the same formula as the TCGA cohort (Supplementary Figure 4A). Similar to the TCGA cohort, patients in the high-risk group had a higher probability of death than patients in the low-risk group (Supplementary Figure 4B). The expression pattern of the risk model genes was similar to the TCGA cohort (Supplementary Figure 4C). K-M curves indicated that patients in the high-risk group had a significantly shorter OS than that in the low-risk group (p < 0.005) (Supplementary Figure 4D). In addition, the AUC of the HMRGs signature model was 0.82 at 1 year, 0.74 at 3 years, and 0.75 at 5 years (Supplementary Figure 4E), which exhibited a good prediction efficacy.
To determine the independent prediction ability of the HMRGs signature model for clinical prognosis, univariate and multivariate Cox regression analyses were carried out on TCGA and E-MTAB-1980 cohort. In univariate Cox regression analyses, the risk score was a significantly prognostic factor for OS (TCGA cohort: HR =1.090, 95% CI =1.070–1.110, p < 0.001, Figure 4A; E-MTAB-1980 cohort: HR = 1.875, 95% CI= 1.357–2.590, p < 0.001, Supplementary Figure 5A). In the multivariate Cox regression analysis, the risk score remained as an independent prognostic variable for OS (TCGA cohort: HR =1.079, 95% CI = 1.058–1.101, p < 0.001, Figure 4B; E-MTAB-1980 cohort: HR = 1.773, 95% CI = 1.304–2.411, p < 0.001, Supplementary Figure 5B). A nomogram is a practical tool that can predict the likelihood of clinical events. Therefore, a nomogram model was constructed by integrating three variables according to the results of the multivariate Cox regression analyses in order to predict survival probability rates for individuals (Figure 4C). As can be seen in Figures 4D–F, the calibration curve illustrated that a good consistency was presented between the actual survival and nomogram predicted survival at 1, 3, and 5 years.
Figure 4 Development of a nomogram predicting OS in ccRCC. Univariate (A) and multivariate Cox regression (B) were performed in the TCGA cohort. Nomogram integrated age, stage, and risk score (C). Calibration curve of the nomogram for predicting OS at 1, 3 and 5 years (D–F).
The mRNA expression of the eight genes from the HMRGs prognostic signature was detected in five paired human ccRCC tissues and adjacent normal tissues. We found that the expression of IRF6, TEK, ABCB1, and COL4A5 was downregulated in ccRCC tissues compared to that in adjacent normal tissues, and the expression of PLCB2, TGFA, PLOD2, and TUBB6 was upregulated in ccRCC tissues compared to that in adjacent normal tissues (Supplementary Figure 6).
Stratification analysis was conducted in order to further verify the performance of the HMRGs signature model to accurately and independently predict the clinical outcome of patients with ccRCC. Patients from the TCGA cohort were categorized into different subgroups based on age (≤65 vs. >65 years), gender (female vs. male), grade (G1/2 vs. G3/4), (AJCC) T stage (T1–2 vs. T3–4), (AJCC) M stage (M(−) vs. M(+)), and (AJCC) stage (stage I/II vs. stage III/IV). Then, K-M survival analysis was performed. We found that patients in the high-risk group consistently showed significantly poor prognosis in all subgroups, including age ≤65 years, age >65 years, female, male, grade G1/2, grade G3/4, T1–2, T3–4, M(−), M(+), stage I/II, and stage III/IV (Figures 5A–L). These results indicated the universal applicability of the HMRGs signature model for predicting prognostic in patients with ccRCC.
Figure 5 K–M survival analysis between the two risk groups in subgroups stratified by clinical characteristics, including age [ ≤ 65 years vs. >65 years] (A, B), gender [female vs. male] (C, D), tumor grade [G1/G2 vs. G3/G4] (E, F), AJCC T stage [T1/T2 vs. T3/T4] (G, H), AJCC M stage [M(–) vs. M(+)] (I, J), and stages [stage I/stage III vs. stage III/stage IV] (K, L), respectively.
The distribution of risk score values was analyzed after stratification according to clinicopathological features for the purpose of further exploring the correlation between risk score and clinicopathological features. As can be seen in Figures 6A, B, from the TCGA cohort analysis results, it was indicated that patients with worse clinicopathological features, including high grade, advanced (AJCC) T stage, (AJCC) metastasis, and advanced (AJCC) TMN stage, had a significantly higher risk score. In addition, from the E-MTAB-1980 cohort analysis results, it was indicated that patients with advanced (AJCC) TMN stage or lymphatic metastasis were associated with an obviously higher risk score (Supplementary Figures 7A, B). In sum, as the risk score increased, the probability of developing advanced tumor gradually increased, indicating that the HMRGs signature may play an important role in the progression of ccRCC.
Figure 6 Correlation between risk score and clinicopathological parameters in the TCGA cohort (A, B). p-values were shown as: ns, not significant; ***p < 0.001.
To seek the potential biological functions and pathways of risk score-related genes, GO enrichment and KEGG pathway analyses were carried out based on the DEGs between the two risk groups. GO analysis revealed that DEGs mainly focused on several immune response processes, such as complement activation classical pathway, humoral immune responses mediated by circulating immunoglobulin, humoral immune responses, B-cell receptor signaling, and immunoglobulin-mediated immune responses (Figure 7A). Moreover, the KEGG analysis revealed that the PPAR signaling pathway was significantly enriched in those DEGs (Figure 7B).
Figure 7 GO and KEGG analysis in the TCGA cohort. GO enrichment analysis (A). KEGG enrichment analysis (B).
To further investigate the difference of immune status between the two risk groups, ssGSEA algorithm was performed for the purpose of quantifying the enrichment scores of diverse immune cell subpopulations and immune-related functions between the two risk groups. As can be seen in Figures 8A, B, it was found that immune cell abundance, including Treg, TIL,Th2_cells, Th1_cell, Tfh,T_helper_cells, pDCs, macrophages, CD8+_T_cells, and aDCs, was significantly higher in the high-risk group. In addition, immune function scores, including Type_I_IFN_Response, T_cell_costimulation, T_cell_co-inhibition, Parainflammation, Inflammation-promoting, Cytolytic_activity, check-point, CCR, and APC_co_stimulation, were significantly stronger in the high-risk group (Figure 8C). The above results indicated that the immune response is more active in the high-risk group than in the low-risk group. Next, the CIBERSORT algorithm was applied for the purpose of comparing the proportion of 22 types of immune cells between the two risk groups. Correlations of these immune cells were depicted in Figure 8D. As shown in Figure 8E, plasma cells, regulatory T cells, and M0 macrophages were significantly higher in the high-risk group, while CD4 memory resting T cells, resting NK cells, monocytes, M2 macrophages, resting dendritic cells, activated dendritic cells, and resting mast cells were much higher in the low-risk group.
Figure 8 Immune infiltration pattern analysis based on risk characteristics in the TCGA cohort. (A) Relationship heatmap of the risk score and ssGSEA scores. Differential analysis of immune cells (B) and immune function (C) between the high-and low-risk groups based on ssGSEA. (D). Correlation among 22 immune cell types based on the CIBERSORT algorithm. (E). Differences in immune cell levels of the high- and low-risk groups based on the CIBERSORT algorithm. p-values were shown as: ns, not significant; *p < 0.05; **p < 0.01; ***p < 0.001.
Immunotherapy is an important part of treatment for advanced ccRCC (3–5). Therefore, we sought to explore whether there is a relationship between risk scores and immunotherapy response. Common immune molecules, including PD-L1, CTLA4, LAG3, and PD-1 are important markers for personalized immunotherapy. It was clear that the expression of CTLA4, LAG3, and PD-1 was significantly higher in the high-risk group than in the low-risk group (Figures 9A, B). Moreover, the risk score was significantly positively correlated with the expression of CTLA4, LAG3, and PD-1 (Figures 9C–E). In short, patients in the high-risk group would benefit more from immunotherapy. Therefore, the HMRGs signature could predict the response to immunotherapy.
Figure 9 The correlation analysis of risk score and immune checkpoint molecules in the TCGA cohort. (A, B). Heatmap of immune checkpoint molecule expression, including PD-1, CTLA4, LAG3, and PD-L1, based on risk characteristics. (C–E) The correlation between the risk score and the expression of immune checkpoints. p-values were shown as: ns, not significant; ***p < 0.001.
Hypoxia and metabolism are the important characteristics of TME and play a crucial role in the tumor occurrence, progression, angiogenesis, drug resistance, and immune response (12–14, 19–21). Considering the importance of the complex interaction between hypoxia and metabolism, it is appropriate to combine hypoxia and metabolism to construct a prognostic model with better accuracy for patients with ccRCC.
In this study, a signature was constructed based on the HMRGs, and the clinical prognostic value was confirmed. All patients were divided into either high-risk group or low-risk group based on the HMRGs-related risk score. Our results indicated that patients in the high-risk group had a significantly shorter OS time than their counterpart in the low-risk group. To further verify the prognostic value of the HMRGs signature model, ROC and independent prognostic analysis were performed, resulting in the signature model being capable of accurately and independently predicting ccRCC clinical prognoses. In addition, patients were assigned to different subgroups according to clinical pathological characteristic subtypes, especially when the clinicopathological factor typing of age, gender, tumor grade, (AJCC) T stage, (AJCC) M stage, and (AJCC) stage can consistently show significantly poor prognosis in the high-risk group, suggesting the universal applicability of the HMRGs signature model we constructed.
Our signature consisted of eight HMRGs signaling-related genes, namely, IRF6, TEK, PLCB2, ABCB1, TGFA, COL4A5, PLOD2, and TUBB6. IRF6 (interferon regulatory factor 6) belongs to the IRF family of transcription factors (22), which has been identified as a tumor suppressor in a variety of human cancers (23). Xu et al. found that IRF6 expression was downregulated in highly metastatic nasopharyngeal carcinoma. Overexpression of IRF6 suppressed nasopharyngeal carcinoma cell proliferation and growth in vitro (24). A recent study reported that IRF6 expression was downregulated in ccRCC, and overexpression of IRF6 suppressed the proliferation, invasion, and migration of ccRCC cells (25). TEK, also known as TIE-2, was a receptor tyrosine kinase, which plays a key role in vascular regeneration and stabilization (26). Chen et al. found that TEK expression was downregulated in ccRCC and that low TEK expression was associated with the poor survival of ccRCC patients. Knockdown of TEK facilitated proliferation and migration and suppressed apoptosis of ccRCC cells in vitro via promoting AKT phosphorylation (27). PLCB2 belongs to the phospholipase C beta (PLCB) gene family, which has been identified to play an important role in the development of various cancers (28, 29). Bertagnolo et al. demonstrated that PLCB2 was upregulated in breast cancer and abnormal increasing expression of PLCB2 was correlated with a poor clinical prognosis (30). ABCB1, also known as multidrug resistance protein 1 (MDR1), has been reported as a transport protein that functions to protect cells from the damage of xenobiotic and toxic substances, including anticancer drugs (31). Omori et al. found that elevated ABCB1 expression was associated with resistance to etoposide in small cell lung cancer (32). A recent review reported that the inhibition of ABCB1 could restore the drug sensitivity of the cancer cells toward chemotherapeutic drugs (33). TGFA belongs to the epidermal growth factor family, which could bind to EGFR to activate a series of signaling pathways involved in several biological processes, including cell proliferation, migration, differentiation, and energy metabolism (34, 35). COL4A5 (collagen type IV alpha 5 chain) is the important component of glomerular basement membrane, and it is well known that mutation or deficiency of COL4A5 was usually related with hereditary human diseases (36, 37). Recent evidence indicated that COL4A5 played an important role in tumor progression. Wu et al. found that COL4A5 expression was significantly upregulated in luminal breast cancer. Knockdown of COL4A5 significantly suppressed the growth of luminal-type breast cancer cells (38). Xiao et al. reported that COL4A5 facilitated proliferation and angiogenesis in lung cancer (39). PLOD2 belongs to the PLOD family, and it has been confirmed that PLOD2 mediated the formation of stabilized collagen cross-links, which played an important role in extracellular matrix (40). Zhen et al. found that PLOD2 expression was significantly high in osteosarcoma, which was associated with the poor survival of patients with osteosarcoma. Overexpression of PLOD2 facilitated osteosarcoma cell migration, invasion, and angiogenesis (41). Du et al. reported that PLOD2 expression was elevated in non-small-cell lung cancer and positively related to a poor prognosis of patients with non-small-cell lung cancer (42). Kurozumi et al. reported that PLOD2 was significantly upregulated in ccRCC and knockdown of PLOD2 significantly suppressed cell migration and invasion (43). TUBB6 (tubulin beta 6 class V) belongs to the β-tubulin family (44). A recent evidence indicated that high expression of TUBB6 was linked to a poor prognosis of patients with bladder cancer, and knockdown of TUBB6 significantly inhibited cell migration and invasion (45). Nami et al. found that aberrant expression of TUBB6 was involved in the potential mechanisms of taxane resistance in breast cancer (46).
Immune infiltration in the TME is a crucial factor affecting tumor progression and response to immunotherapy. GO analysis revealed differing immune-related pathway activities between high-risk and low-risk groups, including complement activation classical pathway, humoral immune responses mediated by circulating immunoglobulin, humoral immune responses, B-cell receptor signaling, and immunoglobulin mediated immune responses. The ssGSEA algorithm showed differing infiltrating scores of immune-cell and immunity-related pathways between the two groups. The results of the CIBERSORT algorithm indicated that patients in the high-risk group had significantly increased infiltration of plasma cells, regulatory T cells, and M0 macrophages, while patients in the low-risk group had significantly increased infiltration of CD4 memory resting T cells, resting NK cells, monocytes, M2 macrophages, resting dendritic cells, activated dendritic cells, and resting mast cells. Tregs could induce immune tolerance and promote immune escape and cancer metastasis (47, 48). Previous studies have verified that T cells’ regulatory infiltration was related to the poor survival of ccRCC patients (49, 50). Pan et al. reported that increased resting mast cells’ density was linked to a favorable prognosis in ccRCC patients (51). Zhang et al. found that ccRCC patients with low risk had increased abundance of CD4 memory resting T cells, resting NK cells, monocytes, and M2 macrophages (52).
Immunotherapy is transformative in treating advanced ccRCC (4, 6). However, there are still a significant number of patients with no response or resistance to immunotherapy (53). Constructing predictive biomarkers for immunotherapy could assist in screening the appropriate patients to achieve precise treatment. Our study used the HMRGs signature to evaluate the response to immunotherapy between the two risk groups. We found that the expression of CTLA4, LAG3, and PD-1 were significantly higher in the high-risk group, suggesting that patients in the high-risk group could better respond to immunotherapy. Moreover, the risk score was significantly positively correlated with the expression of CTLA4, LAG3, and PD-1. These results consistently show that high-risk patients would benefit more from immunotherapy.
However, several limitations existed in the present study. First, all the conclusions in our study were obtained from bioinformatic analysis, and a prospective multiple clinical trial validation was needed to develop a higher evidence level of findings. Second, further experimental studies are needed to explore the specific function and mechanisms of these genes in relation to the progression of ccRCC.
The HMRGs prognostic signature was established based on the integrated analysis of hypoxia- and metabolism-related genes, which was confirmed to be a reliable predictor for OS in ccRCC. Moreover, this signature was correlated with the expression of common immune checkpoints, which could assist in guiding immunotherapy decisions in order to achieve precise treatment.
The original contributions presented in the study are included in the article/Supplementary material. Further inquiries can be directed to the corresponding author.
LL and XW were responsible for the study design and writing. WX and BG were mainly responsible for data analysis. BF, WC, and LZ were mainly responsible for data collection. LL and XW were responsible for manuscript review and providing constructive comments. All authors contributed to the article and approved the submitted version.
We thank TCGA and ArrayExpress databases for providing data.
The authors declare that the research was conducted in the absence of any commercial or financial relationships that could be construed as a potential conflict of interest.
All claims expressed in this article are solely those of the authors and do not necessarily represent those of their affiliated organizations, or those of the publisher, the editors and the reviewers. Any product that may be evaluated in this article, or claim that may be made by its manufacturer, is not guaranteed or endorsed by the publisher.
The Supplementary Material for this article can be found online at: https://www.frontiersin.org/articles/10.3389/fonc.2023.1162846/full#supplementary-material
Supplementary Figure 1 | The flowchart for the process of constructing the HMRG prognostic signature.
Supplementary Figure 2 | (A–H) Analysis expression of the prognostic signature genes (IRF6, TEK, PLCB2, ABCB1, TGFA, COL4A5, PLOD2, and TUBB6) in ccRCC tissues compared with normal tissues in the TCGA data.
Supplementary Figure 3 | (A–H) The survival plot for the signature genes (IRF6, TEK, PLCB2, ABCB1, TGFA, COL4A5, PLOD2, and TUBB6) in the TCGA data.
Supplementary Figure 4 | Validation of the HMRG prognostic signature in the E-MTAB-1980 cohort. (A) Distribution of patients’ risk score. (B) Survival status. (C) Expression of eight signature genes. (D) K-M curves for the OS. (E) ROC curve for 1, 3, and 5 years.
Supplementary Figure 5 | Univariate (A) and multivariate Cox regression (B) were performed in the E-MTAB-1980 cohort.
Supplementary Figure 6 | Analysis expression of the prognostic signature genes (IRF6, TEK, PLCB2, ABCB1, TGFA, COL4A5, PLOD2, and TUBB6) in five paired human ccRCC tissues and adjacent non-tumorous tissues.
Supplementary Figure 7 | Correlation between risk score and clinicopathological parameters in the E-MTAB-1980 cohort (A, B). p-values were shown as: ns, not significant; *p < 0.05; **p < 0.01.
1. Sung H, Ferlay J, Siegel RL, Laversanne M, Soerjomataram I, Jemal A, et al. Global cancer statistics 2020: GLOBOCAN estimates of incidence and mortality worldwide for 36 cancers in 185 countries. CA Cancer J Clin (2021) 71(3):209–49. doi: 10.3322/caac.21660
2. Moch H, Cubilla AL, Humphrey PA, Reuter VE, Ulbright TM. The 2016 WHO Classification of Tumours of the Urinary System and Male Genital Organs-Part A: Renal, penile, and testicular tumours. Eur Urol. (2016) 70:93–105. doi: 10.1016/j.eururo.2016.02.029
3. Rini BI, Battle D, Figlin RA, George DJ, Hammers H, Hutson T, et al. The society for immunotherapy of cancer consensus statement on immunotherapy for the treatment of advanced renal cell carcinoma (RCC). J Immunother Cancer. (2019) 7(1):354. doi: 10.1186/s40425-019-0813-8
4. Motzer RJ, Powles T, Burotto M, Escudier B, Bourlon MT, Shah AY, et al. Nivolumab plus cabozantinib versus sunitinib in first-line treatment for advanced renal cell carcinoma (CheckMate 9ER): long-term follow-up results from an open-label, randomised, phase 3 trial. Lancet Oncol (2022) 23(7):888–98. doi: 10.1016/S1470-2045(22)00290-X
5. Lalani AA, Heng DYC, Basappa NS, Wood L, Iqbal N, McLeod D, et al. Evolving landscape of first-line combination therapy in advanced renal cancer: a systematic review. Ther Adv Med Oncol (2022) 14:17588359221108685. doi: 10.1177/17588359221108685
6. Bedke J, Albiges L, Capitanio U, Giles RH, Hora M, Lam TB, et al. The 2021 updated european association of urology guidelines on renal cell carcinoma: immune checkpoint inhibitor-based combination therapies for treatment-naive metastatic clear-cell renal cell carcinoma are standard of care. Eur Urol. (2021) 80(4):393–7. doi: 10.1016/j.eururo.2021.04.042
7. Peng Q, Wang L, Zhao D, Lv Y, Wang H, Chen G, et al. Overexpression of FZD1 is associated with a good prognosis and resistance of sunitinib in clear cell renal cell carcinoma. J Cancer. (2019) 10(5):1237–51. doi: 10.7150/jca.28662
8. Roviello G, Corona SP, Nesi G, Mini E. Results from a meta-analysis of immune checkpoint inhibitors in first-line renal cancer patients: does PD-L1 matter? Ther Adv Med Oncol (2019) 11:1758835919861905. doi: 10.1177/1758835919861905
9. Ju L, Shi Y, Liu G. Identification and validation of a ferroptosis-related lncRNA signature to robustly predict the prognosis, immune microenvironment, and immunotherapy efficiency in patients with clear cell renal cell carcinoma. PeerJ (2022) 10:e14506. doi: 10.7717/peerj.14506
10. Zhou P, Liu Z, Hu H, Lu Y, Xiao J, Wang Y, et al. Comprehensive analysis of senescence characteristics defines a novel prognostic signature to guide personalized treatment for clear cell renal cell carcinoma. Front Immunol (2022) 13:901671. doi: 10.3389/fimmu.2022.901671
11. Jing X, Yang F, Shao C, Wei K, Xie M, Shen H, et al. Role of hypoxia in cancer therapy by regulating the tumor microenvironment. Mol Cancer. (2019) 18(1):157. doi: 10.1186/s12943-019-1089-9
12. Wicks EE, Semenza GL. Hypoxia-inducible factors: cancer progression and clinical translation. J Clin Invest (2022) 132(11):e159839. doi: 10.1172/JCI159839
13. Multhoff G, Vaupel P. Hypoxia compromises anti-cancer immune responses. Adv Exp Med Biol (2020) 1232:131–43. doi: 10.1007/978-3-030-34461-0_18
14. Kopecka J, Salaroglio IC, Perez-Ruiz E, Sarmento-Ribeiro AB, Saponara S, De Las Rivas J, et al. Hypoxia as a driver of resistance to immunotherapy. Drug Resist Update (2021) 59:100787. doi: 10.1016/j.drup.2021.100787
15. Infantino V, Santarsiero A, Convertini P, Todisco S, Iacobazzi V. Cancer cell metabolism in hypoxia: role of HIF-1 as key regulator and therapeutic target. Int J Mol Sci (2021) 22(11):5703. doi: 10.3390/ijms22115703
16. Taylor CT, Scholz CC. The effect of HIF on metabolism and immunity. Nat Rev Nephrol (2022) 18(9):573–87. doi: 10.1038/s41581-022-00587-8
17. Schiliro C, Firestein BL. Mechanisms of metabolic reprogramming in cancer cells supporting enhanced growth and proliferation. Cells (2021) 10(5):1056. doi: 10.3390/cells10051056
18. Parks SK, Chiche J, Pouyssegur J. Disrupting proton dynamics and energy metabolism for cancer therapy. Nat Rev Cancer. (2013) 13:611–23. doi: 10.1038/nrc3579
19. Zanotelli MR, Zhang J, Reinhart-King CA. Mechanoresponsive metabolism in cancer cell migration and metastasis. Cell Metab (2021) 33(7):1307–21. doi: 10.1016/j.cmet.2021.04.002
20. Xia L, Oyang L, Lin J, Tan S, Han Y, Wu N, et al. The cancer metabolic reprogramming and immune response. Mol Cancer. (2021) 20(1):28. doi: 10.1186/s12943-021-01316-8
21. McGuirk S, Audet-Delage Y, St-Pierre J. Metabolic fitness and plasticity in cancer progression. Trends Cancer. (2020) 6(1):49–61. doi: 10.1016/j.trecan.2019.11.009
22. Xu L, Huang TJ, Hu H, Wang MY, Shi SM, Yang Q, et al. The developmental transcription factor IRF6 attenuates ABCG2 gene expression and distinctively reverses stemness phenotype in nasopharyngeal carcinoma. Cancer Lett (2017) 431:230–43. doi: 10.1016/j.canlet.2017.10.016
23. Parisi L, Mockenhaupt C, Rihs S, Mansour F, Katsaros C, Degen M. Consistent downregulation of the cleft lip/palate-associated genes IRF6 and GRHL3 in carcinomas. Front Oncol (2022) 12:1023072. doi: 10.3389/fonc.2022.1023072
24. Xu L, Huang TJ, Hu H, Wang MY, Shi SM, Yang Q, et al. The developmental transcription factor IRF6 attenuates ABCG2 gene expression and distinctively reverses stemness phenotype in nasopharyngeal carcinoma. Cancer Lett (2018) 431:230–43. doi: 10.1016/j.canlet.2017.10.016
25. Ma X, Wang X, Dong Q, Pang H, Xu J, Shen J, et al. Inhibition of KIF20A by transcription factor IRF6 affects the progression of renal clear cell carcinoma. Cancer Cell Int (2021) 21(1):246. doi: 10.1186/s12935-021-01879-y
26. Augustin HG, Koh GY, Thurston G, Alitalo K. Control of vascular morphogenesis and homeostasis through the angiopoietin-Tie system. Nat Rev Mol Cell Biol (2009) 10:165–77. doi: 10.1038/nrm2639
27. Chen S, Yu M, Ju L, Wang G, Qian K, Xiao Y, et al. The immune-related biomarker TEK inhibits the development of clear cell renal cell carcinoma (ccRCC) by regulating AKT phosphorylation. Cancer Cell Int (2021) 21(1):119. doi: 10.1186/s12935-021-01830-1
28. Zhang T, Song X, Liao X, Wang X, Zhu G, Yang C, et al. Distinct prognostic values of phospholipase C beta family members for non-small cell lung carcinoma. BioMed Res Int (2019) 2019:4256524. doi: 10.1155/2019/4256524
29. Park MS, Lee YE, Kim HR, Shin JH, Cho HW, Lee JH, et al. Phospholipase C beta 2 protein overexpression is a favorable prognostic indicator in newly diagnosed normal karyotype acute myeloid leukemia. Ann Lab Med (2021) 41(4):409–13. doi: 10.3343/alm.2021.41.4.409
30. Bertagnolo V, Benedus M, Querzol P, Pedriali M, Magri E, Brugnoli F, et al. PLC-beta2 is highly expressed in breast cancer and is associated with a poor outcome: a study on tissue microarrays. Int J Oncol (2006) 28(4):863–72.
31. Kathawala RJ, Gupta P, Ashby CR Jr, Chen ZS. The modulation of ABC transporter-mediated multidrug resistance in cancer: a review of the past decade. Drug Resist Updat. (2015) 18:1–17. doi: 10.1016/j.drup.2014.11.002
32. Omori M, Noro R, Seike M, Matsuda K, Hirao M, Fukuizumi A, et al. Inhibitors of ABCB1 and ABCG2 overcame resistance to topoisomerase inhibitors in small cell lung cancer. Thorac Cancer. (2022) 13(15):2142–51. doi: 10.1111/1759-7714.14527
33. Engle K, Kumar G. Cancer multidrug-resistance reversal by ABCB1 inhibition: A recent update. Eur J Med Chem (2022) 239:114542. doi: 10.1016/j.ejmech.2022.114542
34. Zhang X, Li X, Fu X, Yu M, Qin G, Chen G, et al. Circular RNA TAF4B promotes bladder cancer progression by sponging miR-1298-5p and regulating TGFA expression. Front Oncol (2021) 11:643362. doi: 10.3389/fonc.2021.643362
35. Giricz O, Calvo V, Peterson EA, Abouzeid CM, Kenny PA. Tace-dependent tgfα Shedding drives triple-negative breast cancer cell invasion. Int J Cancer. (2013) 133(11):2587–95. doi: 10.1002/ijc.28295
36. Wang S, Shao Y, Wang Y, Lu J, Shao L. Identification of four novel COL4A5 variants and detection of splicing abnormalities in three chinese X-linked alport syndrome families. Front Genet (2022) 13:847777. doi: 10.3389/fgene.2022.847777
37. Xiu X, Yuan J, Deng X, Xiao J, Xu H, Zeng Z, et al. A novel COL4A5 mutation identified in a Chinese Han family using exome sequencing. BioMed Res Int (2014) 186048. doi: 10.1155/2014/186048
38. Wu Y, Liu X, Zhu Y, Qiao Y, Gao Y, Chen J, et al. Type IV collagen α5 chain promotes luminal breast cancer progression through c-Myc-driven glycolysis. J Mol Cell Biol (2022), 14(10):mjac068. doi: 10.1093/jmcb/mjac068
39. Xiao Q, Jiang Y, Liu Q, Yue J, Liu C, Zhao X, et al. Minor type IV collagen α5 chain promotes cancer progression through discoidin domain receptor-1. PloS Genet (2015) 11(5):e1005249. doi: 10.1371/journal.pgen.1005249
40. Xu Q, Kong N, Zhao Y, Wu Q, Wang X, Xun X, et al. Pan-cancer analyses reveal oncogenic and immunological role of PLOD2. Front Genet (2022) 13:864655. doi:10.3389/fgene.2022.864655
41. Wang Z, Fan G, Zhu H, Yu L, She D, Wei Y, et al. PLOD2 high expression associates with immune infiltration and facilitates cancer progression in osteosarcoma. Front Oncol (2022) 12:980390. doi: 10.3389/fonc.2022.980390
42. Du H, Chen Y, Hou X, Huang Y, Wei X, Yu X, et al. PLOD2 regulated by transcription factor FOXA1 promotes metastasis in NSCLC. Cell Death Dis (2017) 8(10):e3143. doi: 10.1038/cddis.2017.553
43. Kurozumi A, Kato M, Goto Y, Matsushita R, Nishikawa R, Okato A, et al. Regulation of the collagen cross-linking enzymes LOXL2 and PLOD2 by tumor-suppressive microRNA-26a/b in renal cell carcinoma. Int J Oncol (2016) 48(5):1837–46. doi: 10.3892/ijo.2016.3440
44. Janke C, Magiera MM. The tubulin code and its role in controlling microtubule properties and functions. Nat Rev Mol Cell Biol (2020) 21:307–26. doi: 10.1038/s41580-020-0214-3
45. Kim B, Jung M, Moon KC, Han D, Kim K, Kim H, et al. Quantitative proteomics identifies TUBB6 as a biomarker of muscle-invasion and poor prognosis in bladder cancer. Int J Cancer (2023) 152(2):320–30. doi: 10.1002/ijc.34265
46. Nami B, Wang Z. Genetics and expression profile of the tubulin gene superfamily in breast cancer subtypes and its relation to taxane resistance. Cancers (Basel) (2018) 10(8):274. doi: 10.3390/cancers10080274
47. Bauer CA, Kim EY, Marangoni F, Carrizosa E, Claudio NM, Mempel TR. Dynamic treg interactions with intratumoral APCs promote local CTL dysfunction. J Clin Invest (2014) 124(6):2425–40. doi: 10.1172/JCI66375
48. Yang P, Li QJ, Feng Y, Zhang Y, Markowitz GJ, Ning S, et al. TGF-b-miR-34a-CCL22 signaling-induced treg cell recruitment promotes venous metastases of HBV-positive hepatocellular carcinoma. Cancer Cell (2012) 22(3):291–303. doi: 10.1016/j.ccr.2012.07.023
49. Nakayama T, Saito K, Kumagai J, Nakajima Y, Kijima T, Yoshida S, et al. Higher serum c-reactive protein level represents the immunosuppressive tumor microenvironment in patients with clear cell renal cell carcinoma. Clin Genitourin cancer. (2018) 16(6):e1151–e8. doi: 10.1016/j.clgc.2018.07.027
50. Li JF, Chu YW, Wang GM, Zhu TY, Rong RM, Hou J, et al. The prognostic value of peritumoral regulatory T cells and its correlation with intratumoral cyclooxygenase-2 expression in clear cell renal cell carcinoma. BJU Int (2009) 103(3):399–405. doi: 10.1111/j.1464-410X.2008.08151.x
51. Pan Q, Wang L, Chai S, Zhang H, Li B. The immune infiltration in clear cell renal cell carcinoma and their clinical implications: A study based on TCGA and GEO databases. J Cancer. (2020) 11(11):3207–15. doi: 10.7150/jca.37285
52. Zhang Y, Chen X, Fu Q, Wang F, Zhou X, Xiang J, et al. Comprehensive analysis of pyroptosis regulators and tumor immune microenvironment in clear cell renal cell carcinoma. Cancer Cell Int (2021) 21(1):667. doi: 10.1186/s12935-021-02384-y
Keywords: clear cell renal cell carcinoma, hypoxia, metabolism, prognosis, immune
Citation: Wu X, Xie W, Gong B, Fu B, Chen W, Zhou L and Luo L (2023) Development and validation of a combined hypoxia- and metabolism-related prognostic signature to predict clinical prognosis and immunotherapy responses in clear cell renal cell carcinoma. Front. Oncol. 13:1162846. doi: 10.3389/fonc.2023.1162846
Received: 10 February 2023; Accepted: 28 September 2023;
Published: 10 November 2023.
Edited by:
Parthasarathy Seshacharyulu, University of Nebraska Medical Center, United StatesReviewed by:
Gopalakrishnan Natarajan, University of Nebraska Medical Center, United StatesCopyright © 2023 Wu, Xie, Gong, Fu, Chen, Zhou and Luo. This is an open-access article distributed under the terms of the Creative Commons Attribution License (CC BY). The use, distribution or reproduction in other forums is permitted, provided the original author(s) and the copyright owner(s) are credited and that the original publication in this journal is cited, in accordance with accepted academic practice. No use, distribution or reproduction is permitted which does not comply with these terms.
*Correspondence: Lianmin Luo, MzcyOTEyNTI3QHFxLmNvbQ==
†These authors have contributed equally to this work
Disclaimer: All claims expressed in this article are solely those of the authors and do not necessarily represent those of their affiliated organizations, or those of the publisher, the editors and the reviewers. Any product that may be evaluated in this article or claim that may be made by its manufacturer is not guaranteed or endorsed by the publisher.
Research integrity at Frontiers
Learn more about the work of our research integrity team to safeguard the quality of each article we publish.