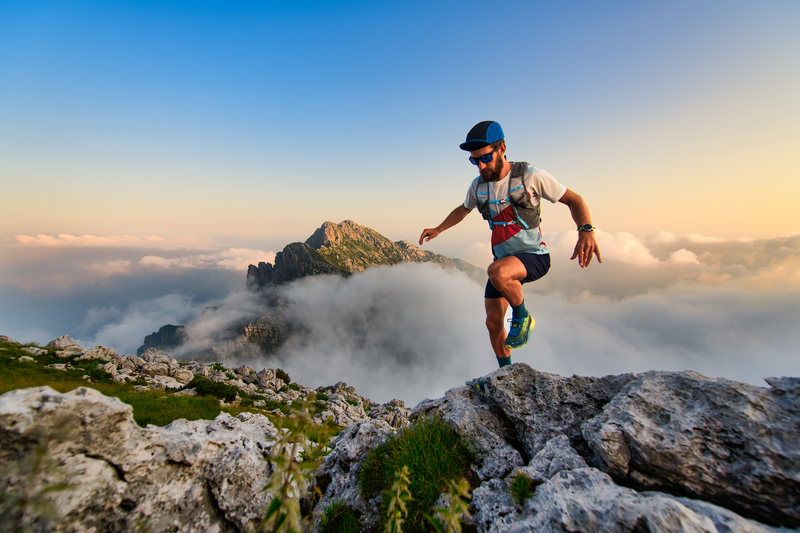
95% of researchers rate our articles as excellent or good
Learn more about the work of our research integrity team to safeguard the quality of each article we publish.
Find out more
ORIGINAL RESEARCH article
Front. Oncol. , 12 October 2023
Sec. Cancer Imaging and Image-directed Interventions
Volume 13 - 2023 | https://doi.org/10.3389/fonc.2023.1162238
This article is part of the Research Topic Editor's Challenge: Abhishek Mahajan - How Can Precision Oncology be Advanced with Validated Imaging-Based Nomograms? View all 8 articles
Purpose: To establish and validate a radiomics nomogram for predicting recurrence of esophageal squamous cell carcinoma (ESCC) after esophagectomy with curative intent.
Materials and methods: The medical records of 155 patients who underwent surgical treatment for pathologically confirmed ESCC were collected. Patients were randomly divided into a training group (n=109) and a validation group (n=46) in a 7:3 ratio. Tumor regions are accurately segmented in computed tomography images of enrolled patients. Radiomic features were then extracted from the segmented tumors. We selected the features by Max-relevance and min-redundancy (mRMR) and least absolute shrinkage and selection operator (LASSO) methods. A radiomics signature was then built by logistic regression analysis. To improve predictive performance, a radiomics nomogram that incorporated the radiomics signature and independent clinical predictors was built. Model performance was evaluated by receiver operating characteristic (ROC) curve, calibration curve, and decision curve analyses (DCA).
Results: We selected the five most relevant radiomics features to construct the radiomics signature. The radiomics model had general discrimination ability with an area under the ROC curve (AUC) of 0.79 in the training set that was verified by an AUC of 0.76 in the validation set. The radiomics nomogram consisted of the radiomics signature, and N stage showed excellent predictive performance in the training and validation sets with AUCs of 0.85 and 0.83, respectively. Furthermore, calibration curves and the DCA analysis demonstrated good fit and clinical utility of the radiomics nomogram.
Conclusion: We successfully established and validated a prediction model that combined radiomics features and N stage, which can be used to predict four-year recurrence risk in patients with ESCC who undergo surgery.
Esophageal cancer is the seventh-most prevalent cancer and has the sixth-highest overall mortality rate among all malignancies (1). Furthermore, esophageal cancer is one of the deadliest and most invasive of all gastrointestinal cancers (2), and approximately half of all esophageal cancer patients experience postoperative recurrence (3). Surgery remains the most effective treatment, especially for early-stage patients. However, recurrence is the primary cause of treatment failure (4). Moreover, recurrence usually occurs within 2 years of the end of treatment (5, 6). Once relapse occurs, patients usually have an unfavorable prognosis, with a reported survival duration of 3–10 months (7). Accurately predicting postoperative recurrence and offering preventive treatment measures is therefore an urgent issue to be addressed in clinical practice. Clinical stage is an important factor that affects prognosis. However, because of the heterogeneity of tumors, patients with the stage of disease have significant variation in prognosis (8). Therefore, early and accurate identification of these patients is beneficial for designing individualized treatments and improving prognosis.
For patients with esophageal squamous cell carcinoma (ESCC), the pre-treatment clinical Tumor-Node-Metastasis (TNM) staging continues to be used widely for predicting prognosis (9). However, the current method has several limitations: the criteria for clinical TNM stage are the same as those for pathological stage, which is based on imaging assessments of lesion size and invasion of peripheral organs; thus, high-dimensional medical imaging data is ignored.
Computed tomography (CT) is a widely used imaging modality that provides a large number of quantitative features and fine anatomical structures that are valuable for confirming the presence of esophageal cancer. CT is the most common non-invasive imaging tool for lesion assessment. In addition, the evaluation of tumor heterogeneity is of great significance for evaluating the degree of malignancy and predicting the prognosis of patients. CT-based imaging informatics has developed rapidly over the last several years and provide valuable information for diagnoses and predictions of prognosis. Driven by the trend of artificial intelligence, “radiomics” was proposed, which involves the rapid extraction of numerous quantitative features from tomographic images via high-throughput computation, and the digital medical images are subsequently converted into mineable multidimensional data (10). This method allows the exploration of pathophysiological information of various diseases using medical images, and the association between images and prognosis can be analyzed (11, 12). Previous studies have reported that radiomics has the potential to predict therapeutic response and prognosis in ESCC patients. Xie et al. suggested that radiomics is superior to volumetric measurements for disease assessment and that it can provide valuable predictions for individualized overall survival (13). Wu et al. combined radiomic features and clinical risk factors to construct a radiomic model and found that it could predict lymph node (LN) metastasis in ESCC patients before surgery (14). Lu et al. indicated that the dual-region radiomics signature is an independent prognostic marker that is better than the single-region signature for predicting ESCC patients’ overall survival (OS); moreover, combining the dual-region radiomics signature and clinicopathological factors could further improve OS prediction (15).
Recently, several clinicopathologic biomarkers have been confirmed as valuable for the prediction of therapeutic response and prognosis in patients with ESCC (16–18). Therefore, we aimed to develop and validate a radiomics nomogram based on a radiomics signature and clinical independent predictors for the prediction of recurrence in ESCC patients who have undergone surgical treatment with curative intent.
The study protocol was approved by the Ethics Committee of the hospital. During the study, ESCC patients who received radical treatment in our hospital from January 2015 to November 2016 were enrolled. Based on the relevant criteria, 155 patients met the requirements and were included in the study. All selected subjects underwent chest-enhanced CT examination before the operation.
The inclusion criteria were as follows (1): postoperatively and pathologically confirmed ESCC; (2) contrast-enhanced CT of the chest performed within one month before surgery; and (3) no distant metastases prior to surgery. The exclusion criteria were as follows: (1) incomplete clinical information; (2) receipt of tumor-related treatment (e.g., chemotherapy or radiotherapy) prior to undergoing CT; (3) poor CT image quality or unrecognizable lesion; and (4) other concurrent malignancies. Patient clinical data, which included sex, age, and TN stage, were obtained from medical records following surgery. Tumor location was determined based on the 8th edition of the AJCC Cancer Staging Manual (19). Follow-up and survival data were collected based on telephonic inquiries. Recurrence was confirmed by histopathological biopsy or clinical follow-up, and included locoregional, distant, or a combination of both. The time of recurrence started from the day of the operation to the discovery of recurrence. Each patient was followed-up for at least 4 years or until the time recurrence occurred. Patients with esophageal cancer were divided into the recurrence group (recurrence occurred in 4 years) and non-recurrence group (recurrence did not occur in 4 years).
In the course of imaging examination, all subjects underwent chest-enhanced CT scanning through multi-detector CT system: Bright Speed, Optima CT 680 Series (GE Medical Systems), Siemens Somatom definition AS 64, and Perspective (Siemens Medical Systems). The scanning parameters set during inspection are as follows: detector configuration 128×0.6 mm; tube voltage, 120–130 kV; tube current, 150–300 mAs; thickness, 5 mm; and pitch, 0.6. According to the obtained image, tumor segmentation and feature extraction were performed.
Pre operative enhanced CT images were collected and saved based on a unified format. Two doctors with extensive experience in imaging examination of digestive system diseases observed the images, and the CT images of each layer were compared and analyzed in detail. Tumor regions of interest (ROIs) were delineated using ITK-SNAP (http://www.itksnap.org); an example is shown in Figure 1. For the tumor ROIs, radiologists reviewed all CT image slices of each patient and segmented the three-dimensional-labeling ROIs covering the whole entire tumor. Observer 1 delineated the lesions of ESCC. The observer 2 re-checked the tumor segmentation area. If the assessment of two radiologists was inconsistent, a thorough negotiation was conducted until consensus was reached.
Figure 1 An example of manual segmentation in ESCC. (A) Localized thick wall of esophageal cancer with enhancement is observed on the arterial phase computed tomography (CT) image; (B) Manual segmentation on the same axial slice is depicted with red label.
Radiomics feature extraction was based on Pyradiomics (https://pyradiomics.readthedocs.io/en/latest/), which is an efficient, open-source platform, through which users can process medical images to extract radiomics features. In this paper, the maximum correlation minimum redundancy (mRMR) and LASSO algorithms were used to select the features, and the rad-score of every ESCC patient was calculated using their coefficients.
For the construction of the radio-clinical model, we first used univariate analysis to analyze the clinical predictors and rad-score, and the strongly associated features were then processed in the multivariate logistic regression analysis. The multivariable logistic regression analysis was used to develop a prediction model by combining the rad-score and clinical predictors (P<0.05). In the training cohorts, for the sake of convenience, the model was converted to the radio-clinical nomogram. The performance of the radio-clinical model was tested by the validation set.
The ROC curve, calibration curve, and Decision curve analysis (DCA) were used to evaluate the prediction of this radio-clinical model. During validation, the performance of this model was evaluated by 10-fold cross validation, and the best model was obtained by comparing the results. The diagnostic value of the clinical model was proved using the validation set. In the evaluation process, the area under the ROC curve (AUC), sensitivity, and specificity-related indicators were calculated first. We also used the Delong test to compare the AUC values in different models. DCA mainly verifies the clinical practicability of the model through net income.
The statistical analyses were carried out using the R software (http://www.Rproject.org). Quantitative data were described as means ± standard deviations, and qualitative data were described as frequencies (percentages). The independent predictive factors in the variables are determined based on multiple regression analysis. Significant difference was based on P<0.05. The “glmnet” package was used in LASSO regression analysis, and the “rms” package was used in multivariate regression analysis. The “pROC” package was used to process the collected data and establish the ROC diagram. “Rmda” package was used for DCA analysis.
Based on the statistical analysis, 93 of the 155 patients with ESCC were in the recurrence group and 62 were in the non-recurrence group. Of the 155 patients included in this study, 93 patients developed disease progression within 4 years. In both the training and validation sets, higher rad-scores were found in the recurrence group than in the non-recurrence group. Additional details are provided in Table 1.
After preprocessing the CT images of each patient, 1781 radiomics features were extracted. At the beginning of processing, redundant and meaningless features were deleted through mRMR, and 30 features were obtained after processing. Then, an optimized feature subset was selected based on LASSO, and the model was established after appropriate processing. When selecting the best radiomics features, LASSO method with 10-fold cross validation is applied to process the results, as shown in Figure 2. Finally, the model contained five radiomics features, which were weighted by coefficients to obtain the rad-score, as shown in Figure 3. The corresponding calculation expression is as follows:
Figure 2 Feature selection with the least absolute shrinkage and selection operator (LASSO) binary logistic regression model. (A, B) The LASSO includes choosing the regular parameter λ, determining the number of the feature. (C) The selected radiomics features (with nonzero coefficients) and their coefficients.
Figure 3 The radscores from class 0 and class 1 on training group and testing group respectively. “0” for no recurrence, “1” for recurrence.
The results of univariate analysis show that T stage, N stage and radiomics signature served as the risk factors of postoperative recurrence in ESCC patients. After multivariate logistic regression analysis, N stage and radiomics signature were identified as independent predictors of postoperative recurrence in ESCC patients (Table 2). Multivariable analysis was performed to develop a prediction model by combining the rad-score and N stage. The radiomics nomogram is illustrated in Figure 4. The formula for the nomoscore is as follows:
Figure 4 The CT-based radiomics nomogram. The radiomics nomogram was built in the training cohort, with the radiomics signature and N stage.
The performance comparison results of the radiomics nomogram are shown in Table 3. According to the results in Figure 5, the prediction ability based on the radiomics features model is limited. The AUC values of this model were 0.79 and 0.76 in the training set and validation set, respectively. If the prediction is only based on the clinical characteristics, the corresponding AUC values are 0.77 and 0.68, respectively. The model combining radiomics features and clinical factors has stronger performance than other relevant models and can effectively predict the recurrence risk in the application process. According to Figure 5, the AUC values of this model for the training set and validation set are 0.85 and 0.83, respectively. The calibration curve of the radiation nomogram also showed good prediction performance (Figure 6). We used DeLong’s test to compare whether the ROC curves are different between nomogram and clinical model. The DeLong’s test showed that the statistical difference between the nomogram and clinical model was significant (P = 0.006 for the training cohort and P =0.019 for the validation cohort). The radiomics nomogram DCA showed a higher overall net benefit than the clinical factors model, demonstrating high clinical utility in predicting postoperative recurrence (Figure 7).
Figure 5 The ROC curves (AUC) of the three models in the training set (A) and the validation set (B).
Figure 7 Decision curve analysis (DCA) of radiomics nomogram in the training set (A) and validation set (B).
Surgery is the most effective strategy for treating early esophageal cancer (20). However, the rate of local recurrence with surgical treatment alone remains high (7). Accurate identification of patients prone to relapse is important for individualized treatment options. CT is a widely accepted clinical imaging modality and occupies an important position in the diagnosis, staging, and response evaluation of esophageal cancer (21). In this study, we used enhanced CT images before surgical treatment to establish a radio-clinical model to predict recurrence in patients with esophageal cancer. CT images are useful in predicting the prognosis of esophageal cancer after surgical treatment, yet poor in revealing potential tumor heterogeneity. Radiomics based on medical images is an emerging method for predicting cancer treatment response and long-term survival. To date, numerous studies have demonstrated the significant value of radiomics in clinical practice. Gillies et al. reported the great potential of radiomics to distinguish between benign and malignant diseases and predict the prognosis of tumor patients (11). Moreover, Ganeshan et al. confirmed that CT texture analysis can evaluate the heterogeneity of esophageal cancers (22).
The radiomics model in our study was established using five radiomics features and achieved a moderate result in predicting recurrence of esophageal cancer after surgery in both the training and validation sets. Two features were wavelet-based features, similar to that reported by few other studies (23–25). Wavelet transform is a new analysis technique developed from the boundedness of the short-time Fourier transform; however, it made up for its deficiencies (e.g., it can provide a change with the frequency of the “time-frequency” window) and is the best solution for analyzing and processing signal time-frequency (26). The “rad-score” integrated multiple radiomics features into a biomarker using multivariate logistic regression models. Our study suggested that the rad-score is an independent predictor of recurrence in ESCC patients after surgical treatment. In contrast to the “N stage,” radiomics features were the dominant factor in our radiomics nomogram (27).
Our radiomics model can predict postoperative recurrence to a certain extent. However, clinical characteristics, such as tumor location, T stage, and N stage, are also important influencing factors of esophageal cancer postoperative recurrence (28). These clinical factors are easily identified during the course of treatment and do not further burden patients. Over the past few years, a growing number of studies have shown that combining radiomics markers with clinical factors improves the accuracy of disease prediction (23, 29). Therefore, we hypothesized that our radiological model could improve predictive performance when combined with clinical factors, which we verified experimentally. Our radiomics signature contained five relevant radiomics features and offered moderate predictive efficacy. The AUC values of the radiomics model in the training and validation sets were 0.79 and 0.76, respectively. When we integrated the independent clinical risk factors with the radiomics signature, the predictive power of the model improved. The AUC values of the radiomics nomogram in the training and validation sets were 0.85 and 0.83, respectively, which showed that the performance of our radiomics nomogram was superior to that of both the radiomics feature model and the clinical factor model.
In terms of clinical factors, we included the postoperative T stage and N stage to avoid the deviation in prediction results caused by inaccurate judgments of TN stage owing to the subjective differences between radiologists. All patients included in this study had esophageal cancer who had undergone surgical resection; hence, none of the patients developed distant metastasis. Therefore, the clinical factors included in this study did not consider the difference in M stage. Furthermore, we found that there was no statistical difference in T stage between the two groups of patients, regardless of relapse. The reason for this might be because the primary tumor lesion was completely removed after radical resection of esophageal cancer, so the degree of invasion of the primary tumor would not be an independent risk factor for recurrence. Lymph node metastasis is used extensively to stratify ESCC patients according to the risk of recurrence. This is important for identifying patients who are likely to benefit from neoadjuvant chemoradiation (30). Previous studies have verified that lymph node metastasis is an independent risk factor for recurrence. In our study, univariate analysis showed that N stage was an independent predictor of recurrence risk; however, there were no significant differences between the recurrence and non-recurrence groups with respect to sex, age, T stage, or tumor location. Therefore, we included N stage in our prediction model.
In the present study, we constructed and validated a radiomics nomogram for the prediction of postoperative 4-year recurrence risk in patients with ESCC who have undergone surgery. The user-friendly nomogram comprising a radiomics signature and N stage demonstrated excellent performance in both cohorts and accurately stratified patients according to postoperative recurrence risk. The nomogram was built using a well-calibrated and well-validated prediction model. Our findings supported our hypothesis in that patients can be successfully stratified using a radiomics nomogram that integrates radio-clinical features by showing good performance in both the training and validation cohorts.
This study has some limitations. First, because long-term prognostic follow-up information was not readily available, the sample size of our study was small. Moreover, it was a single-center study. Thus, it is essential to conduct further large-scale and multi-center studies. Second, because of the retrospective nature of the study, there may be some bias. In future research, a time-divided model should be established. Finally, several previous studies have combined genetic information with radiomics features to predict prognosis; however, we did not include genetic information in this study. Future studies should aim to incorporate genetic information into radio-clinical features.
Our prediction model was established by combining radiomics features with N stage, and it shows great promise and clinical application value for predicting the 4-year recurrence of ESCC following surgery.
Declarations
The data analyzed in this study is subject to the following licenses/restrictions: The original data for this article are not publicly available because of patients information privacy. Requests to access these datasets should be directed to Fangxiao Lu (bGZ4ODA2MTFAMTYzLmNvbQ==).
This retrospective study was approved by the Medical Ethics Committee of Zhejiang Cancer Hospital (No. IRB-2022-54). The Medical Ethics Committee of Zhejiang Cancer Hospital waived the need for informed consent.
YT completed the initial manuscript and designed the whole study; JC collected imaging data and participated in revising the manuscript; JS and KL collected imaging data and participated in tumor segmentation; TL collected patients and recorded the needed information; SD was responsible for data processing and interpretation; KZ participated in the statistics and provided result interpretation and revising the manuscript; JZ and FL revised the manuscript and guaranteed the entire study. All authors contributed to the article and approved the submitted version.
This work was supported by Medical Health Science and Technology Project of Zhejiang Province (2021KY583, 2022KY654, 2022KY655), Key Laboratory of Prevention, Diagnosis and Therapy of Upper Gastrointestinal Cancer of Zhejiang Province (2022E10021), Natural Science Foundation of Zhejiang Province (LSY19H030001).
SD was employed by GE Healthcare.
The remaining authors declare that the research was conducted in the absence of any commercial or financial relationships that could be construed as a potential conflict of interest.
All claims expressed in this article are solely those of the authors and do not necessarily represent those of their affiliated organizations, or those of the publisher, the editors and the reviewers. Any product that may be evaluated in this article, or claim that may be made by its manufacturer, is not guaranteed or endorsed by the publisher.
1. Bray F, Ferlay J, Soerjomataram I, Siegel RL, Torre LA, Jemal A. Global cancer statistics 2018: GLOBOCAN estimates of incidence and mortality worldwide for 36 cancers in 185 countries. CA Cancer J Clin (2018) 68(6):394–424. doi: 10.3322/caac.21492
2. Kumbasar B. Carcinoma of esophagus: radiologic diagnosis and staging. Eur J Radiol (2002) 42(3):170–80. doi: 10.1016/s0720-048x(02)00030-x
3. Miyata H, Yamasaki M, Kurokawa Y, Takiguchi S, Nakajima K, Fujiwara Y, et al. Survival factors in patients with recurrence after curative resection of esophageal squamous cell carcinomas. Ann Surg Oncol (2011) 18(12):3353–61. doi: 10.1245/s10434-011-1747-7
4. Nakagawa S, Kanda T, Kosugi S, Ohashi M, Suzuki T, Hatakeyama K. Recurrence pattern of squamous cell carcinoma of the thoracic esophagus after extended radical esophagectomy with three-field lymphadenectomy. J Am Coll Surg (2004) 198(2):205–11. doi: 10.1016/j.jamcollsurg.2003.10.005
5. Mariette C, Balon JM, Piessen G, Van Seuningen I, Triboulet JP. Pattern of recurrence following complete resection of esophageal carcinoma and factors predictive of recurrent disease. Cancer (2003) 97(7):1616–23. doi: 10.1002/cncr.11228
6. Abate E, DeMeester SR, Zehetner J, Oezcelik A, Ayazi S, Costales J, et al. Recurrence after esophagectomy for adenocarcinoma: defining optimal follow-up intervals and testing. J Am Coll Surg (2010) 210(4):428–35. doi: 10.1016/j.jamcollsurg.2010.01.006
7. Parry K, Visser E, van Rossum PS, Mohammad NH, Ruurda JP, van Hillegersberg R. Prognosis and treatment after diagnosis of recurrent esophageal carcinoma following esophagectomy with curative intent. Ann Surg Oncol (2015) 22(Suppl.3):S1292–300. doi: 10.1245/s10434-015-4840-5
8. Ou J, Wu L, Li R, Wu CQ, Liu J, Chen TW, et al. CT radiomics features to predict lymph node metastasis in advanced esophageal squamous cell carcinoma and to discriminate between regional and non-regional lymph node metastasis: a case control study. Quant Imag Med Surg (2021) 11(2):628–40. doi: 10.21037/qims-20-241
9. Wang WP, He SL, Yang YS, Chen LQ. Strategies of nodal staging of the TNM system for esophageal cancer. Ann Transl Med (2018) 6:77. doi: 10.21037/atm.2017.12.17
10. Lambin P, Leijenaar RTH, Deist TM, Peerlings J, de Jong EEC, van Timmeren J, et al. Radiomics: the bridge between medical imaging and personalized medicine. Nat Rev Clin Oncol (2017) 14(12):749–62. doi: 10.1038/nrclinonc.2017.141
11. Gillies RJ, Kinahan PE, Hricak H. Radiomics: images are more than pictures, they are data. Radiology (2016) 278(2):563–77. doi: 10.1148/radiol.2015151169
12. Xu Y, Lu L, E L-n, Lian W, Yang H, Schwartz LH, et al. Application of radiomics in predicting the Malignancy of pulmonary nodules in different sizes. AJR Am J Roentgenol (2019) 213(6):1213–20. doi: 10.2214/AJR.19.21490
13. Xie CY, Hu YH, Ho JW, Han LJ, Yang H, Wen J, et al. Using genomics feature selection method in radiomics pipeline improves prognostication performance in locally advanced esophageal squamous cell carcinoma-A pilot study. Cancers (Basel) (2021) 13(9):2145. doi: 10.3390/cancers13092145
14. Wu L, Yang X, Cao W, Zhao K, Li W, Ye W, et al. Multiple level CT radiomics features preoperatively predict lymph node metastasis in esophageal cancer: A multicenter retrospective study. Front Oncol (2020) 9:1548. doi: 10.3389/fonc.2019.01548
15. Lu N, Zhang WJ, Dong L, Chen JY, Zhu YL, Zhang SH, et al. Dual-region radiomics signature: Integrating primary tumor and lymph node computed tomography features improves survival prediction in esophageal squamous cell cancer. Compute Methods Programs BioMed (2021) 208:106287. doi: 10.1016/j.cmpb.2021.106287
16. Luo HS, Xu HY, Du ZS, Li XY, Wu SX, Huang HC, et al. Impact of sex on the prognosis of patients with esophageal squamous cell cancer underwent definitive radiotherapy: a propensity score-matched analysis. Radiat Oncol (2019) 14:74. doi: 10.1186/s13014-019-1278-0
17. Yang Z, He B, Zhuang X, Gao X, Wang D, Li M, et al. CT-based radiomic signatures for prediction of pathologic complete response in esophageal squamous cell carcinoma after neoadjuvant chemoradiotherapy. J Radiat Res (2019) 60(4):538–45. doi: 10.1093/jrr/rrz027
18. Hu P, Liu Q, Deng G, Zhang J, Liang N, Xie J, et al. Radiosensitivity nomogram based on circulating neutrophils in thoracic cancer. Future Oncol (2019) 15(7):727–37. doi: 10.2217/fon-2018-0398
19. Rice TW, Patil DT, Blackstone EH. 8th edition AJCC/UICC staging of cancers of the esophagus and esophagogastric junction: application to clinical practice. Ann Cardiothorac Surg (2017) 6:119–30. doi: 10.21037/acs.2017.03.14
20. Harada K, Rogers JE, Iwatsuki M, Yamashita K, Baba H, Ajani JA. Recent advances in treating esophageal cancer. F1000Res (2020) 9:1189. doi: 10.12688/f1000research.22926.1
21. Jayaprakasam VS, Yeh R, Ku GY, Petkovska I, Fuqua JL 3rd, Gollub M, et al. Role of imaging in esophageal cancer management in 2020: update for radiologists. AJR Am J Roentgenol (2020) 215:1072nol. doi: 10.2214/AJR.20.22791
22. Ganeshan B, Skogen K, Pressney I, Coutroubis D, Miles K. Tumor heterogeneity in oesophageal cancer assessed by CT texture analysis: preliminary evidence of an association with tumor metabolism, stage, and survival. Clin Radiol (2012) 67(2):157–64. doi: 10.1016/j.crad.2011.08.012
23. Qiu Q, Duan J, Deng H, Han Z, Gu J, Yue NJ, et al. Development and validation of a radiomics nomogram model for predicting postoperative recurrence in patients with esophageal squamous cell cancer who achieved pCR after neoadjuvant chemoradiotherapy followed by surgery. Front Oncol (2020) 10:1398. doi: 10.3389/fonc.2020.01398
24. Liang W, Yang P, Huang R, Xu L, Wang J, Liu W, et al. A combined nomogram model to preoperatively predict histologic grade in pancreatic neuroendocrine tumors. Clin Cancer Res (2019) 25:584–94. doi: 10.1158/1078-0432.CCR-18-1305
25. Wu S, Zheng J, Li Y, Wu Z, Shi S, Huang M, et al. Development and validation of an MRI-based radiomics signature for the preoperative prediction of lymph node metastasis in bladder cancer. EBioMedicine (2018) 34:76–84. doi: 10.1016/j.ebiom.2018.07.029
26. van Griethuysen JJM, Fedorov A, Parmar C, Hosny A, Aucoin N, Narayan V, et al. Computational radiomics system to decode the radiographic phenotype. Cancer Res (2017) 77(21):e104–7. doi: 10.1158/0008-5472.CAN-17-0339
27. Shen C, Liu Z, Wang Z, Guo J, Zhang H, Wang Y, et al. Building CT radiomics based nomogram for preoperative esophageal cancer patients lymph node metastasis prediction. Transl Oncol (2018) 11(3):815–24. doi: 10.1016/j.tranon.2018.04.005
28. Barbetta A, Sihag S, Nobel T, Hsu M, Tan KS, Bains M, et al. Patterns and risk of recurrence in patients with esophageal cancer with a pathologic complete response after chemoradiotherapy followed by surgery. J Thorac Cardiovasc Surg (2019) 157(3):1249–59.e5. doi: 10.1016/j.jtcvs.2018.09.136
29. Huang K, Lin Y, Yang L, Wang Y, Cai S, Pang L, et al. Alzheimer’s Disease Neuroimaging Initiative. A multipredictor model to predict the conversion of mild cognitive impairment to Alzheimer's disease by using a predictive nomogram. Neuropsychopharmacology (2020) 45(2):358–66. doi: 10.1038/s41386-019-0551-0
Keywords: esophageal squamous cell carcinoma/esophageal cancer, radiomics, tomography, X-Ray Computed, nomogram, recurrence
Citation: Tong Y, Chen J, Sun J, Luo T, Duan S, Li K, Zhou K, Zeng J and Lu F (2023) A radiomics nomogram for predicting postoperative recurrence in esophageal squamous cell carcinoma. Front. Oncol. 13:1162238. doi: 10.3389/fonc.2023.1162238
Received: 09 February 2023; Accepted: 25 September 2023;
Published: 12 October 2023.
Edited by:
Dakai Jin, Alibaba DAMO Academy (U.S.), United StatesReviewed by:
Zhinuan Hong, Fujian Medical University Union Hospital, ChinaCopyright © 2023 Tong, Chen, Sun, Luo, Duan, Li, Zhou, Zeng and Lu. This is an open-access article distributed under the terms of the Creative Commons Attribution License (CC BY). The use, distribution or reproduction in other forums is permitted, provided the original author(s) and the copyright owner(s) are credited and that the original publication in this journal is cited, in accordance with accepted academic practice. No use, distribution or reproduction is permitted which does not comply with these terms.
*Correspondence: Jian Zeng, Njg2NDA3NzBAcXEuY29t; Fangxiao Lu, bHVmeEB6amNjLm9yZy5jbg==
Disclaimer: All claims expressed in this article are solely those of the authors and do not necessarily represent those of their affiliated organizations, or those of the publisher, the editors and the reviewers. Any product that may be evaluated in this article or claim that may be made by its manufacturer is not guaranteed or endorsed by the publisher.
Research integrity at Frontiers
Learn more about the work of our research integrity team to safeguard the quality of each article we publish.