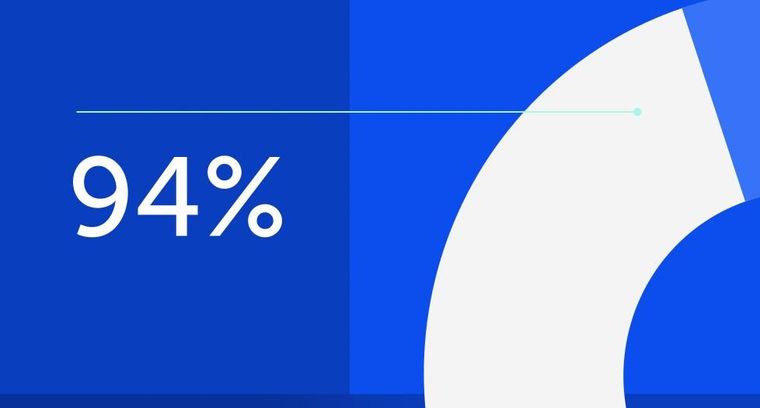
94% of researchers rate our articles as excellent or good
Learn more about the work of our research integrity team to safeguard the quality of each article we publish.
Find out more
ORIGINAL RESEARCH article
Front. Oncol., 05 May 2023
Sec. Thoracic Oncology
Volume 13 - 2023 | https://doi.org/10.3389/fonc.2023.1162181
Background: Small cell lung cancer (SCLC) is an aggressive and almost universally lethal neoplasm. There is no accurate predictive method for its prognosis. Artificial intelligence deep learning may bring new hope.
Methods: By searching the Surveillance, Epidemiology, and End Results database (SEER), 21,093 patients’ clinical data were eventually included. Data were then divided into two groups (train dataset/test dataset). The train dataset (diagnosed in 2010–2014, N = 17,296) was utilized to conduct a deep learning survival model, validated by itself and the test dataset (diagnosed in 2015, N = 3,797) in parallel. According to clinical experience, age, sex, tumor site, T, N, M stage (7th American Joint Committee on Cancer TNM stage), tumor size, surgery, chemotherapy, radiotherapy, and history of malignancy were chosen as predictive clinical features. The C-index was the main indicator to evaluate model performance.
Results: The predictive model had a 0.7181 C-index (95% confidence intervals, CIs, 0.7174–0.7187) in the train dataset and a 0.7208 C-index (95% CIs, 0.7202–0.7215) in the test dataset. These indicated that it had a reliable predictive value on OS for SCLC, so it was then packaged as a Windows software which is free for doctors, researchers, and patients to use.
Conclusion: The interpretable deep learning survival predictive tool for small cell lung cancer developed by this study had a reliable predictive value on their overall survival. More biomarkers may help improve the prognostic predictive performance of small cell lung cancer.
In 2022, around 609,360 cancer deaths were projected to occur in the United States, including approximately 350 deaths per day from lung cancer, the leading cause of cancer death, according to the estimate from the American Cancer Society (1). Moreover, it is estimated that the cancer burden will be projected to double by 2050, of which lung cancer will be at the top of the list (2). Approximately, lung cancer can be classified into two subtypes, small cell lung cancer (SCLC) (accounting for 15% of the total) or non-small cell lung cancer (around 85% of the total). SCLC is an almost universally lethal neoplasm, with rapid growth, aggressive proliferation, and high rate of metastasis (3, 4). Although SCLC is usually sensitive to chemotherapy or radiotherapy, this fast-growing neuroendocrine lung cancer owns a <7% 5-year survival rate only (around 15%–30% in limited-stage and <1% in extensive-stage) (5–8). Frustratingly, there has been little progress in its treatment for nearly 30 years (7).
Predicting SCLC patients’ overall survival (OS) might offer some references to clinicians. The most common reference is the American Joint Committee on Cancer (AJCC) TNM stage. By evaluating patients’ stage, doctors can know their rough survival probabilities. Considering TNM only includes tumor size and lymph node and distant metastases, there are still some controversies in its accuracy. For example, Shuai Shi and colleagues found that the 8th edition AJCC TNM stage for non-small cell lung cancer was not applicable to lung cancer as a second primary malignancy (9). Salomon Tendler and colleagues even mentioned that single metastatic lesions (M1b) had a better prognosis compared with M1c, when validating the 8th TNM stage for SCLC in a retrospective material from Sweden (10). Ziqing Zeng also pointed out that patients owning the same stage and similar treatment might differ in survival (11, 12). Another choice was to use traditional statistical models to achieve predictive tasks. The Cox proportional hazard (CPH) model is a classical statistical method, widely used in clinical practice. The CPH model calculates the linear association between outcome and related factors and can give a patient’s survival probability by accumulating all risk factor scores. However, it can be too simplistic to assume that the association is linear, especially in a complex and realistic clinically scenario (13).
Given the limit from the TNM stage or CPH, clinical researchers turned their attention to deep learning. Deep learning networks usually include several layers, like input layers, hidden layers, and output layers. Each layer contains certain nodes, and nodes between adjacent layers will develop connections weighted by some parameters, which are refreshed by lots of epochs to simulate and study the sophisticated association between inputted variables (like clinical features) and outcome. Due to the above highlights, deep learning can discover the underlying laws between predictive variables and outcome, by learning the complex, non-linear relationships and representation levels, which usually shows more robust performance than statistical methods like CPH or a traditional machine learning algorithm (14, 15). As a subdiscipline of machine learning, deep learning was increasingly valued by clinicians after displaying its advantages especially in handling big data (16–20). In order to handle survival data, Jared L. Katzman suggested a modern Cox proportional hazards model based on the deep learning method, named DeepSurv, to better fit this realistic non-linear application (13). DeepSurv combined the advantages of CPH and deep learning algorithm and can simulate more complex relationships. Yunlang She noted that DeepSurv showed great potential in providing individual prognostic information and treatment recommendations in non-small cell lung cancer, with areas under the receiver operating curve (AUCs) scoring 0.739 and 0.742 in internal and external validations, respectively (21). Homoplastically, Quincy A. Hathaway and colleagues discovered that DeepSurv can leverage simple clinical features alone to accurately predict atherosclerotic cardiovascular disease risks and cardiovascular outcomes (22). John Adeoye and colleagues pointed out that DeepSurv can accurately predict the malignant-transformation-free survival of oral potentially malignant disorders. Therefore, using this deep learning survival algorithm to build a predictive tool for SCLC patients was suitable and needed.
All data used in this study came from the Surveillance, Epidemiology, and End Results database (SEER). It is an authoritative and almost broadest coverage of source for cancer statistics in the United States, managed by the National Cancer Institute (NCI). Many doctors and researchers have used it to obtain more detailed and realistic clinical data and gain more comprehensive understanding of tumors (1, 23, 24). We chose it to search data and conduct models, which might return reliable clinical information and a robustly predictive model.
In this study, we collected SCLC patients’ clinical data from SEER and chose age, sex, tumor site, T, N, M stage, tumor size, surgery, chemotherapy, radiotherapy, and history of malignancy to act as predictive clinical features, then first conducted an interpretable deep learning-based OS predictive model for SCLC. The model showed certain predictive accuracy, so we packaged and uploaded it. This tool is very friendly. Users only need to input the above clinical information and click Predict, and the software will automatically call the pretrained dense neural network to convert the clinical information into the survival curve of that patient. It is free for doctors, researchers, and patients to use.
This study was designed as a retrospective cohort study. We browsed and searched SEER, downloading the 17 Registries database (2000–2019) which covers approximately 26.5% of the US population. By setting Site: Lung and Bronchus and Pathology: ICD-O-3 Small cell carcinoma 8041/3, we acquired the raw data. Considering that the follow-up cutoff date was December 31, 2019 (according to SEER’s official manual), and patients staged by the 8th AJCC TNM stage (mainly used from 2018) without sufficient duration of follow-up, we checked raw data to make sure its 7th AJCC TNM stage information (mainly patients diagnosed in 2010–2015) is intact. With missing values omitted, data with a sample size of 21,093 were eventually included by this study. Based on the diagnosed time, data were then divided into two groups (train dataset/test dataset), and the train dataset (diagnosed in 2010-2014) was utilized to conduct a deep learning survival model, validated by itself and the test dataset (diagnosed in 2015) in parallel (Figure 1).
Figure 1 Flowchart of this research. SEER, the Surveillance, Epidemiology, and End Results database; AJCC, American Joint Committee on Cancer; SCLC, small cell lung cancer. OS, overall survival.
According to clinical experience, age, sex, tumor site, T, N, M stage (7th AJCC TNM stage), tumor size, surgery, chemotherapy, radiotherapy, and history of malignancy were chosen as predictive clinical features. Age, more advanced stage (T, N, M stage), and bigger tumor size were classic risk factors of SCLC, so they were included (25, 26). Meanwhile, tumor sites might also influence clinical treatment (those in the main trachea cannot be easily removed, and tumors near large blood vessels or important organs would not be easily treated with radiotherapy). Therefore, we included tumor site and the most common therapy in SCLC (surgery, chemotherapy, and radiotherapy). Although there is no consensus on the effect of sex on cancer’s prognosis yet, considering the differences in living habits between different genders and the potential influence of hormones, we also included them like in previous studies (27, 28). Malignancy history was a risk factor for most cancers, which might indicate the underlying gene mutations carried by individuals and damage the patient’s health, increasing the occurrence of additional cancers, and thus it served as a prognostic indicator too (29).
Due to SEER record regulation, patients older than 100 years were still recorded as 100-year-olds. Also, survival time of less than 1 month was still documented as 1 month.
The DeepSurv algorithm, a CPH deep neural network and state-of-the-art survival method for modeling interactions between a patient’s covariates and treatment, was taken to complete this survival-predictive model for SCLC (13). This model had two hidden layers (16 and 8 nodes each) and batch normalization layers. Numeric clinical features (age and tumor size) were normalized (subtract the mean and divide by the standard deviation), and the test dataset was normalized too in compliance with the train dataset. The StandardScaler function from Python package sklearn was utilized to achieve the above process, and related parameters were saved in joblib file where we can use its dump function to store parameters and use the load function to call back then standardize data automatically when necessary.
Categorical clinical features (sex, tumor site, T, N, M, stage, surgery, chemotherapy, radiotherapy and history of malignancy) were converted to dummy variables (replace characters with numbers, such as replacing Female as 1). This procedure was accomplished manually. Supplement Table 1 contained the complete numerical codes.
To avoid overfitting, early stopping function were turned on to end training timely, whereas dropout layers were added too to prevent certain nodes from extremely high weights.
The model was conducted only using the train dataset but validated by both the train dataset and test dataset. The C-index was the main indicator to evaluate model performance. The closer the C-index is to 1, the better the model’s performance is, and the closer the C-index is to 0.5, the worse the model’s performance is. A model with a C-index greater than 0.7–0.8 is considered to have a good predictive value, usually. In order to get the C-index and 95% confidence intervals (CIs), we used 1,000 times bootstrap in both datasets.
Given the model’s usability and convenience, we packaged it into a runnable Windows executable files (in an environment of Windows 11, 64-bit version). This deep learning survival predictive tool for SCLC was free for doctors, researchers, and patients to use.
All analysis was completed with R software (https://www.r-project.org/). Non-normally distributed numeric data were analyzed by Wilcoxon test, whereas categorical data were compared by the chi-square test. A two-sided P less than 0.05 was considered statistically significant.
A total of 21,093 patients were eventually included by this study. The train dataset (diagnosed in 2010–2014, N = 17,296) and test dataset (diagnosed in 2015, N = 3,797) had similar clinical features in sex, tumor site, T, M, stage, tumor size, surgery, chemotherapy, radiotherapy, and history of malignancy, but there was a difference in age and N of two datasets (Table 1). Figure 2 shows the presentation of the overall clinical features too, of which categorical clinical features were shown by the (A) train dataset and (B) test dataset and illustrates (C) the age profile of two datasets. The median follow-up time of both datasets was 8 months.
Figure 2 Visualized presentation of the overall categorical clinical features from the (A) train dataset and (B) test dataset. (C) The age profile of above datasets. NOS, not otherwise specified. Stage was evaluated according to the 7th American Joint Committee on Cancer TNM stage.
As we talked, the train dataset was used to conduct the model, which was then validated by both the train dataset and test dataset. After 294 epochs, early stopping function terminated the model training automatically. The training curves are shown in Supplementary Figure 1.
Finally, this model scored 0.7181 in the train dataset and 0.7210 in the test dataset on the C-index. After 1,000 times bootstrap, the predictive model had a 0.7181 C-index (95% CIs, 0.7174–0.7187) in the train dataset and 0.7208 C-index (95% CIs, 0.7202–0.7215) in the test dataset. The above indicated that the model had a reliable predictive value on OS for SCLC patients (Table 2).
We also tested the model’s performance at 1, 3, and 5 years. The model showed 0.8087 AUC, 0.7518 specificity, 0.7123 sensitivity, and 0.7254 accuracy at 1 year in the train dataset, and 0.8175 AUC, 0.7549 specificity, 0.7241 sensitivity, and 0.7341 accuracy at 1 year in the test dataset (Table 3). Meanwhile, the model exhibited 0.8300 AUC, 0.7587 specificity, 0.7417 sensitivity, and 0.7435 accuracy at 3 years in the train dataset and 0. 8228 AUC, 0.7419 specificity, 0.7456 sensitivity, and 0.7452 accuracy at 3 years in the test dataset (Table 3). Moreover, it had 0.8274 AUC, 0.7499 specificity, 0.7456 sensitivity, and 0.7459 accuracy at 5 years in the train dataset and 0.8161 AUC, 0.7247 specificity, 0.7515 sensitivity, and 0.7492 accuracy at 5 years in the test dataset (Table 3). The receiver operating curve also demonstrated that this model had a satisfactory predictive value (Figure 3A).
Figure 3 (A) The receiver operating curve of this model at 1, 3, and 5 years. Interpretable deep learning survival predictive tool for small cell lung cancer: (B) tool interface, (C) screenshot of the results when tool running. SCLC, small cell lung cancer; OS, overall survival.
The detailed hyperparameters of this model are shown in Supplement Figure 2.
Then, we wrapped this deep learning model into Windows executable files. This tool can be installed after launching Supplementary File 1. When inputting an SCLC patient relevant clinical feature, users can click the “Predict” button to start up the deep learning model for calculating (Figure 3B). After a minute, a website will be open in the system default web browser (Figure 3C). This website shows the predictive survival curve, as the x-axis represents months and the y-axis is the predictive OS probability of this patient. This survival curve is interactable, for specific values can be displayed when mouse hovering and be able to zoom in or out. It’s free for doctors, researchers and patients to use.
As a subtype of lung cancer, SCLC arises in peribronchial locations and infiltrates the bronchial submucosa, with many new cases diagnosed every year (30). One to five persons per 10,000 people can be diagnosed with SCLC in the European community, more prevalent in men than women (31–33). As for the treatment of SCLC, some scholars have pessimistically concluded that there has been no virtual progress in the treatment of SCLC for 30 years (34).Considering that only a tiny subset of individuals with SCLC can get surgery, the classical treatment regimen for them is platinum-based chemotherapy (35). Unfortunately, although showing a robust response to initial therapy, almost all SCLC patients recur and are resistant to further therapy, with a median OS of 10 months after first-line chemotherapy (36, 37). Similarly, after receiving therapy with first- or second-generation EGFR tyrosine kinase inhibitors for 9 to 15 months, the majority of SCLC patients inevitably develop acquired resistance through a variety of mechanisms (38–40). Immune checkpoint inhibitors (ICIs) like programmed death-ligand 1 (PD-L1) were exciting new therapeutic agents for SCLC in recent years. In the IMPower133 trial, SCLC patients receiving atezolizumab plus carboplatin and etoposide had 12.3 months of median OS (hazard ratio, HR, 0.76; 95% CIs, 0.60–0.95; P = 0.0154), compared with 10.3 months from the placebo, atezolizumab, and carboplatin group (41). In the CASPIAN trial, durvalumab plus platinum-etoposide patients had a longer OS around 13.0 median months (HR 0.73; 95% CIs 0.59–0.91; P = 0.0047) compared to the platinum-etoposide group (around 10.3 median OS) (42). However, in KEYNOTE-604, pembrolizumab plus etoposide and platinum did not improve SCLC patient OS significantly (43). Meanwhile, it must be mentioned that the expression of PD-L1 was less common in SCLC patients according to reports. The PD-L1 expression level in immune cells was <1% in almost half of the cases (68/137), whereas it was <1% in nearly all samples (129/137) of cancer cells in IMPower133 (41, 44). Therefore, despite the fact that ICIs plus standard chemotherapy might prolong SCLC patients’ OS, there is still a long way to go due to the following: the limited OS improvement, only a small number of beneficial patients, and the lack of predictive biomarkers (45–47). Hence, predicting the OS of SCLC patients can help guide clinical decisions by taking into account the side effects and unfavorable consequences of chemotherapy, targeted therapy, and immunotherapy.
In respect of the management of SCLC patients, a used staging system in the past was the Veterans Administration Lung Study Group (VALSG), grouping SCLC into limited-stage or extensive-stage (48). However, more researchers appealed to use the AJCC TNM stage instead to obtain more benefit in defining optimal treatment strategies these years (10, 35, 49, 50). As we mentioned earlier, there are still some controversies in the TNM staging system’s accuracy for it only includes tumor size, lymph node, and distant metastasis. Previous researchers have tried to develop new models to predict SCLC’s survival. Shidan Wang et al. used age, gender, race, ethnicity, Charlson/Deyo score, TNM stage, treatment type, and laterality to act as predictive variables to develop an SCLC predictive nomogram based on CPH and The National Cancer Database, with a concordance index of 0.722 (51). However, the Charlson/Deyo score is not widely used in some countries, which might limit the application of this model. Hui Pan and colleagues utilized clinical features and CPH to predict SCLC patients’ OS, the model scoring a 0.68 concordance index in the primary cohort and 0.66 in the validation cohort (52). Zeng et al. established a prognostic model for the survival of resected limited-stage SCLC, with a performance 0.722–0.746 in the train cohort and 0.693–0.816 in the validation cohort (53). Simultaneously, some researchers attempted to use biomarkers to predict SCLC’s clinical outcome. Minlin Jiang and colleagues built a FOXP3-based immune risk model to predict I–III stage SCLC’s recurrence, and the model scored 0.656–0.737 AUC in the train cohort and 0.608–0.714 in the validation cohort (54). Xie et al. used peripheral blood markers and CPH to develop a model predicting SCLC’s prognosis with a 0.73 concordance index (55). Shicheng Feng and colleagues identified six novel prognostic gene signatures, which scored 0.825 AUC in SCLC’s prognostic prediction (56). Biomarkers appeared to improve the predictive performance of prognostic models for SCLC, but these materials were not readily available, especially in SCLC for the limited surgical chance.
Deep learning was a novel machine learning algorithm that has been transplanted to the medical field with attention in recent years. It has been demonstrated that it can discriminate high-risk smokers for lung cancer screening computed tomography, Coronavirus disease 2019, fibrotic lung disease, histopathological whole slide images, and so on (57–60). Yunlang She and colleagues have built a deep learning survival model to predict non-small cell lung cancer survival (21). This model had a 0.739 C statistic and was visualized by a user-friendly graphic interface then. However, SCLC still lacks such model to help in clinical decision making. In this study, we pioneered to conduct an interpretable deep learning survival predictive model for SCLC and packaged it into an accessible tool. This model had a reliable predictive performance, with a 0.7181 C-index in the train dataset and 0.7208 in the test dataset.
The median age of SCLC patients in our data was 68 years, similar to previous studies (61–63). Older SCLC patients are thought to have a poorer prognosis, especially older than 60 or 70 years (3, 25, 26). Interestingly, in our tests of this deep learning model, we found that it thought age had limited weight and little influence on OS in most cases. The potential cause might be that we considered age to be a numeric rather than a categorical variable. As a result, an age difference of decades has no significant influence on prognosis. Although it is very common to use age truncation as categorical data in statistical analysis, we still insist on treating it as a continuous variable this time, which is also the most common way of processing in deep learning. Meanwhile, the proportion of male and female patients was approximately equal in our research, as the study by Lin Gao (61), but different from that of Hidefumi Takei or Kosei Doshita (significantly more for men) (63, 64). This difference might be ethnographic between Asians and Americans, but more research was needed to confirm. Patients with SCLC were more often diagnosed with advanced (stages III–IV) in our observation, which was the same with earlier studies (61, 63, 65, 66) but different from the data from Japan between 2004 and 2010 (64). The discrepancies might derive from recent advances in screening tools.
There were still some limitations in this study. This deep learning survival model might need to be validated further by Asian population data. Certain personal history like smoking status, parental cancer diagnosis, or gene-based biomarker might be an implication for patients’ survival but might not be included this time.
To make a long story short, we first built an interpretable deep learning survival predictive tool for SCLC using 21,093 patients’ data. This tool had a reliable predictive value on OS for SCLC with a 0.7181 C-index (95% CIs, 0.7174–0.7187) in the train dataset and a 0.7208 C-index (95% CIs, 0.7202–0.7215) in the test dataset.
An interpretable deep learning survival predictive tool for small cell lung cancer developed by this study had a reliable predictive value on their overall survival. More biomarkers may help improve the prognostic predictive performance of small cell lung cancer.
The original contributions presented in the study are included in the article/Supplementary Material. Further inquiries can be directed to the corresponding authors.
This study has been approved by the Ethics Committee of Tianjin Chest Hospital and individual consent for this retrospective analysis was waived. Written informed consent for participation was not required for this study in accordance with the national legislation and the institutional requirements.
(I) Conception and design: DZ and WJ. (II) administrative support: DZ and YP. (III) provision of study materials or patients: BL, BHL, YP, ZW, MG and BWL. (IV) collection and assembly of data: BL, BHL, YP, ZW, MG and BWL. (V) data analysis and interpretation: DZ and YP. All authors contributed to the article and approved the submitted version.
This work was supported by the Project of Tianjin Chest Hospital (No. 2018XKZ28) and by Tianjin Key Medical Discipline (Specialty) Construction Project to DZ and the Programme of Cultivation Plan of Beijing Municipal Health Administration (No. PX2023059) to YP.
We are grateful for the help of the support team from The Surveillance, Epidemiology, and End Results at the National Cancer Institute.
The authors declare that the research was conducted in the absence of any commercial or financial relationships that could be construed as a potential conflict of interest.
All claims expressed in this article are solely those of the authors and do not necessarily represent those of their affiliated organizations, or those of the publisher, the editors and the reviewers. Any product that may be evaluated in this article, or claim that may be made by its manufacturer, is not guaranteed or endorsed by the publisher.
The Supplementary Material for this article can be found online at: https://www.frontiersin.org/articles/10.3389/fonc.2023.1162181/full#supplementary-material
Supplementary Figure 1 | The training curves of deep learning survival predictive model for SCLC.
Supplementary Figure 2 | The detailed hyper-parameters of this model.
Supplementary File 1 | Installation file of interpretable deep learning survival predictive tool for SCLC. (https://drive.google.com/file/d/1yjdh-mSkqTOEPpsV21t8hd4drjimmY3V/view?usp=share_link).
Supplement File 2 | The raw data of this study.
Supplementary Table 1 | Numerical codes of categorical variables. NOS, not otherwise specified.
SCLC, small cell lung cancer; OS, overall survival; AJCC, American Joint Committee on Cancer; CPH, Cox proportional hazard; AUC, area under the receiver operating curve; SEER, the Surveillance, Epidemiology, and End Results database; NCI, National Cancer Institute; CIs, confidence intervals; ICIs, immune checkpoint inhibitors; PD-L1, programmed death-ligand 1; HR, hazard ratio; VALSG, the Veterans Administration Lung Study Group.
1. Siegel RL, Miller KD, Fuchs HE, Jemal A. Cancer statistics, 2022. CA Cancer J Clin (2022) 72(1):7–33. doi: 10.3322/caac.21708
3. Wang S, Zimmermann S, Parikh K, Mansfield AS, Adjei AA. Current diagnosis and management of small-cell lung cancer. Mayo Clin Proc (2019) 94(8):1599–622. doi: 10.1016/j.mayocp.2019.01.034
4. Travis WD, Brambilla E, Riely GJ. New pathologic classification of lung cancer: relevance for clinical practice and clinical trials. J Clin Oncol (2013) 31(8):992–1001. doi: 10.1200/JCO.2012.46.9270
5. Siegel RL, Miller KD, Jemal A. Cancer statistics, 2020. CA Cancer J Clin (2020) 70(1):7–30. doi: 10.3322/caac.21590
6. Niu X, Chen L, Li Y, Hu Z, He F. Ferroptosis, necroptosis, and pyroptosis in the tumor microenvironment: perspectives for immunotherapy of sclc. Semin Cancer Biol (2022) 86(Pt 3):273–85. doi: 10.1016/j.semcancer.2022.03.009
7. Byers LA, Rudin CM. Small cell lung cancer: where do we go from here? Cancer (2015) 121(5):664–72. doi: 10.1002/cncr.29098
8. Chute JP, Chen T, Feigal E, Simon R, Johnson BE. Twenty years of phase iii trials for patients with extensive-stage small-cell lung cancer: perceptible progress. J Clin Oncol (1999) 17(6):1794–801. doi: 10.1200/JCO.1999.17.6.1794
9. Shi S, Xie H, Yin W, Zhang Y, Peng X, Yu F, et al. The prognostic significance of the 8th edition ajcc tnm staging system for non-Small-Cell lung cancer is not applicable to lung cancer as a second primary malignancy. J Surg Oncol (2020) 121(8):1233–40. doi: 10.1002/jso.25903
10. Tendler S, Grozman V, Lewensohn R, Tsakonas G, Viktorsson K, De Petris L. Validation of the 8th tnm classification for small-cell lung cancer in a retrospective material from Sweden. Lung Cancer (2018) 120:75–81. doi: 10.1016/j.lungcan.2018.03.026
11. Zeng Z, Yang F, Wang Y, Zhao H, Wei F, Zhang P, et al. Significantly different immunoscores in lung adenocarcinoma and squamous cell carcinoma and a proposal for a new immune staging system. Oncoimmunology (2020) 9(1):1828538. doi: 10.1080/2162402X.2020.1828538
12. Mlecnik B, Bindea G, Pages F, Galon J. Tumor immunosurveillance in human cancers. Cancer Metastasis Rev (2011) 30(1):5–12. doi: 10.1007/s10555-011-9270-7
13. Katzman JL, Shaham U, Cloninger A, Bates J, Jiang T, Kluger Y. Deepsurv: personalized treatment recommender system using a cox proportional hazards deep neural network. BMC Med Res Methodol (2018) 18(1):24. doi: 10.1186/s12874-018-0482-1
14. Wainberg M, Merico D, Delong A, Frey BJ. Deep learning in biomedicine. Nat Biotechnol (2018) 36(9):829–38. doi: 10.1038/nbt.4233
15. Ching T, Himmelstein DS, Beaulieu-Jones BK, Kalinin AA, Do BT, Way GP, et al. Opportunities and obstacles for deep learning in biology and medicine. J R Soc Interface (2018) 15(141):20170387. doi: 10.1098/rsif.2017.0387
16. Wang F, Casalino LP, Khullar D. Deep learning in medicine-promise, progress, and challenges. JAMA Intern Med (2019) 179(3):293–4. doi: 10.1001/jamainternmed.2018.7117
17. Jiang Y, Yang M, Wang S, Li X, Sun Y. Emerging role of deep learning-based artificial intelligence in tumor pathology. Cancer Commun (Lond) (2020) 40(4):154–66. doi: 10.1002/cac2.12012
18. Zhang T, Leng J, Liu Y. Deep learning for drug-drug interaction extraction from the literature: a review. Brief Bioinform (2020) 21(5):1609–27. doi: 10.1093/bib/bbz087
19. Ding W, Nakai K, Gong H. Protein design Via deep learning. Brief Bioinform (2022) 23(3):bbac102. doi: 10.1093/bib/bbac102
20. Stahlschmidt SR, Ulfenborg B, Synnergren J. Multimodal deep learning for biomedical data fusion: a review. Brief Bioinform (2022) 23(2):bbab569. doi: 10.1093/bib/bbab569
21. She Y, Jin Z, Wu J, Deng J, Zhang L, Su H, et al. Development and validation of a deep learning model for non-small cell lung cancer survival. JAMA Netw Open (2020) 3(6):e205842. doi: 10.1001/jamanetworkopen.2020.5842
22. Hathaway QA, Yanamala N, Budoff MJ, Sengupta PP, Zeb I. Deep neural survival networks for cardiovascular risk prediction: the multi-ethnic study of atherosclerosis (Mesa). Comput Biol Med (2021) 139:104983. doi: 10.1016/j.compbiomed.2021.104983
23. Doll KM, Rademaker A, Sosa JA. Practical guide to surgical data sets: surveillance, epidemiology, and end results (Seer) database. JAMA Surg (2018) 153(6):588–9. doi: 10.1001/jamasurg.2018.0501
24. Sultan I, Qaddoumi I, Yaser S, Rodriguez-Galindo C, Ferrari A. Comparing adult and pediatric rhabdomyosarcoma in the surveillance, epidemiology and end results program, 1973 to 2005: an analysis of 2,600 patients. J Clin Oncol (2009) 27(20):3391–7. doi: 10.1200/JCO.2008.19.7483
25. Ou SH, Ziogas A, Zell JA. Prognostic factors for survival in extensive stage small cell lung cancer (Ed-sclc): the importance of smoking history, socioeconomic and marital statuses, and ethnicity. J Thorac Oncol (2009) 4(1):37–43. doi: 10.1097/JTO.0b013e31819140fb
26. Zhou M, Fan J, Li Z, Li P, Sun Y, Yang Y, et al. Prognostic impact of tumor mutation burden and the mutation in Kiaa1211 in small cell lung cancer. Respir Res (2019) 20(1):248. doi: 10.1186/s12931-019-1205-9
27. Oberije C, De Ruysscher D, Houben R, van de Heuvel M, Uyterlinde W, Deasy JO, et al. A validated prediction model for overall survival from stage iii non-small cell lung cancer: toward survival prediction for individual patients. Int J Radiat Oncol Biol Phys (2015) 92(4):935–44. doi: 10.1016/j.ijrobp.2015.02.048
28. Siegfried JM. Sex and gender differences in lung cancer and chronic obstructive lung disease. Endocrinology (2022) 163(2):bqab254. doi: 10.1210/endocr/bqab254
29. Greenwald P, Dunn BK. Landmarks in the history of cancer epidemiology. Cancer Res (2009) 69(6):2151–62. doi: 10.1158/0008-5472.CAN-09-0416
30. Meijer JJ, Leonetti A, Airo G, Tiseo M, Rolfo C, Giovannetti E, et al. Small cell lung cancer: novel treatments beyond immunotherapy. Semin Cancer Biol (2022) 86(Pt 2):376–85. doi: 10.1016/j.semcancer.2022.05.004
31. Govindan R, Page N, Morgensztern D, Read W, Tierney R, Vlahiotis A, et al. Changing epidemiology of small-cell lung cancer in the united states over the last 30 years: analysis of the surveillance, epidemiologic, and end results database. J Clin Oncol (2006) 24(28):4539–44. doi: 10.1200/JCO.2005.04.4859
32. Dingemans AC, Fruh M, Ardizzoni A, Besse B, Faivre-Finn C, Hendriks LE, et al. Small-cell lung cancer: esmo clinical practice guidelines for diagnosis, treatment and follow-up(). Ann Oncol (2021) 32(7):839–53. doi: 10.1016/j.annonc.2021.03.207
34. Sabari JK, Lok BH, Laird JH, Poirier JT, Rudin CM. Unravelling the biology of sclc: implications for therapy. Nat Rev Clin Oncol (2017) 14(9):549–61. doi: 10.1038/nrclinonc.2017.71
35. Dingemans AC, Fruh M, Ardizzoni A, Besse B, Faivre-Finn C, Hendriks LE, et al. Small-cell lung cancer: esmo clinical practice guidelines for diagnosis, treatment and follow-up(☆). Ann Oncol (2021) 32(7):839–53. doi: 10.1016/j.annonc.2021.03.207
36. Herzog BH, Devarakonda S, Govindan R. Overcoming chemotherapy resistance in sclc. J Thorac Oncol (2021) 16(12):2002–15. doi: 10.1016/j.jtho.2021.07.018
37. Gazdar AF, Bunn PA, Minna JD. Small-cell lung cancer: what we know, what we need to know and the path forward. Nat Rev Cancer (2017) 17(12):725–37. doi: 10.1038/nrc.2017.87
38. Westover D, Zugazagoitia J, Cho BC, Lovly CM, Paz-Ares L. Mechanisms of acquired resistance to first- and second-generation egfr tyrosine kinase inhibitors. Ann Oncol (2018) 29(suppl_1):i10–i9. doi: 10.1093/annonc/mdx703
39. Mok TS, Wu YL, Ahn MJ, Garassino MC, Kim HR, Ramalingam SS, et al. Osimertinib or platinum-pemetrexed in egfr T790m-positive lung cancer. N Engl J Med (2017) 376(7):629–40. doi: 10.1056/NEJMoa1612674
40. He J, Huang Z, Han L, Gong Y, Xie C. Mechanisms and management of 3rd−Generation Egfr−Tki resistance in advanced Non−Small cell lung cancer (Review). Int J Oncol (2021) 59(5). doi: 10.3892/ijo.2021.5270
41. Liu SV, Reck M, Mansfield AS, Mok T, Scherpereel A, Reinmuth N, et al. Updated overall survival and pd-L1 subgroup analysis of patients with extensive-stage small-cell lung cancer treated with atezolizumab, carboplatin, and etoposide (Impower133). J Clin Oncol (2021) 39(6):619–30. doi: 10.1200/JCO.20.01055
42. Paz-Ares L, Dvorkin M, Chen Y, Reinmuth N, Hotta K, Trukhin D, et al. Durvalumab plus platinum-etoposide versus platinum-etoposide in first-line treatment of extensive-stage small-cell lung cancer (Caspian): a randomised, controlled, open-label, phase 3 trial. Lancet (2019) 394(10212):1929–39. doi: 10.1016/S0140-6736(19)32222-6
43. Rudin CM, Awad MM, Navarro A, Gottfried M, Peters S, Csoszi T, et al. Pembrolizumab or placebo plus etoposide and platinum as first-line therapy for extensive-stage small-cell lung cancer: randomized, double-blind, phase iii keynote-604 study. J Clin Oncol (2020) 38(21):2369–79. doi: 10.1200/JCO.20.00793
44. Longo V, Catino A, Montrone M, Pizzutilo P, Annese T, Pesola F, et al. What are the biomarkers for immunotherapy in sclc? Int J Mol Sci (2021) 22(20):11123. doi: 10.3390/ijms222011123
45. Yang S, Zhang Z, Wang Q. Emerging therapies for small cell lung cancer. J Hematol Oncol (2019) 12(1):47. doi: 10.1186/s13045-019-0736-3
46. Verma V, Sharma G, Singh A. Immunotherapy in extensive small cell lung cancer. Exp Hematol Oncol (2019) 8:5. doi: 10.1186/s40164-019-0129-x
47. Liu B, Song Y, Liu D. Recent development in clinical applications of pd-1 and pd-L1 antibodies for cancer immunotherapy. J Hematol Oncol (2017) 10(1):174. doi: 10.1186/s13045-017-0541-9
48. Davis S, Stanley KE, Yesner R, Kuang DT, Morris JF. Small-cell carcinoma of the lung–survival according to histologic subtype: a veterans administration lung group study. Cancer (1981) 47(7):1863–6. doi: 10.1002/1097-0142(19810401)47:7<1863::aid-cncr2820470724>3.0.co;2-6
49. Rudin CM, Brambilla E, Faivre-Finn C, Sage J. Small-cell lung cancer. Nat Rev Dis Primers (2021) 7(1):3. doi: 10.1038/s41572-020-00235-0
50. Marzano L, Darwich AS, Tendler S, Dan A, Lewensohn R, De Petris L, et al. A novel analytical framework for risk stratification of real-world data using machine learning: a small cell lung cancer study. Clin Transl Sci (2022) 15(10):2437–47. doi: 10.1111/cts.13371
51. Wang S, Yang L, Ci B, Maclean M, Gerber DE, Xiao G, et al. Development and validation of a nomogram prognostic model for sclc patients. J Thorac Oncol (2018) 13(9):1338–48. doi: 10.1016/j.jtho.2018.05.037
52. Pan H, Shi X, Xiao D, He J, Zhang Y, Liang W, et al. Nomogram prediction for the survival of the patients with small cell lung cancer. J Thorac Dis (2017) 9(3):507–18. doi: 10.21037/jtd.2017.03.121
53. Zeng Q, Li J, Tan F, Sun N, Mao Y, Gao Y, et al. Development and validation of a nomogram prognostic model for resected limited-stage small cell lung cancer patients. Ann Surg Oncol (2021) 28(9):4893–904. doi: 10.1245/s10434-020-09552-w
54. Jiang M, Wu C, Zhang L, Sun C, Wang H, Xu Y, et al. Foxp3-based immune risk model for recurrence prediction in small-cell lung cancer at stages I-III. J Immunother Cancer (2021) 9(5):e002339. doi: 10.1136/jitc-2021-002339
55. Xie D, Marks R, Zhang M, Jiang G, Jatoi A, Garces YI, et al. Nomograms predict overall survival for patients with small-cell lung cancer incorporating pretreatment peripheral blood markers. J Thorac Oncol (2015) 10(8):1213–20. doi: 10.1097/JTO.0000000000000585
56. Feng S, Zhang X, Gu X, Zhou M, Chen Y, Wang C. Identification of six novel prognostic gene signatures as potential biomarkers in small cell lung cancer. Comb Chem High Throughput Screen (2022) 26(5):938–949. doi: 10.2174/1386207325666220427121619
57. Lu MT, Raghu VK, Mayrhofer T, Aerts H, Hoffmann U. Deep learning using chest radiographs to identify high-risk smokers for lung cancer screening computed tomography: development and validation of a prediction model. Ann Intern Med (2020) 173(9):704–13. doi: 10.7326/M20-1868
58. Wang S, Zha Y, Li W, Wu Q, Li X, Niu M, et al. A fully automatic deep learning system for covid-19 diagnostic and prognostic analysis. Eur Respir J (2020) 56(2):2000775. doi: 10.1183/13993003.00775-2020
59. Walsh SLF, Mackintosh JA, Calandriello L, Silva M, Sverzellati N, Larici AR, et al. Deep learning-based outcome prediction in progressive fibrotic lung disease using high-resolution computed tomography. Am J Respir Crit Care Med (2022) 206(7):883–91. doi: 10.1164/rccm.202112-2684OC
60. Yang H, Chen L, Cheng Z, Yang M, Wang J, Lin C, et al. Deep learning-based six-type classifier for lung cancer and mimics from histopathological whole slide images: a retrospective study. BMC Med (2021) 19(1):80. doi: 10.1186/s12916-021-01953-2
61. Gao L, Shen L, Wang K, Lu S. Propensity score matched analysis for the role of surgery in stage III small cell lung cancer based on the eighth edition of the tnm classification: a population study of the us seer database and a Chinese hospital. Lung Cancer (2021) 162:54–60. doi: 10.1016/j.lungcan.2021.10.009
62. Tan F, Bi N, Zhang H, Li R, Wang Z, Duan J, et al. External validation of the eighth edition of the tnm classification for lung cancer in small cell lung cancer. Lung Cancer (2022) 170:98–104. doi: 10.1016/j.lungcan.2022.03.011
63. Doshita K, Kenmotsu H, Omori S, Tabuchi Y, Kawabata T, Kodama H, et al. Long-term survival data of patients with limited disease small cell lung cancer: a retrospective analysis. Invest New Drugs (2022) 40(2):411–9. doi: 10.1007/s10637-021-01183-6
64. Takei H, Kondo H, Miyaoka E, Asamura H, Yoshino I, Date H, et al. Surgery for small cell lung cancer: a retrospective analysis of 243 patients from Japanese lung cancer registry in 2004. J Thorac Oncol (2014) 9(8):1140–5. doi: 10.1097/JTO.0000000000000226
65. Kang HS, Lim JU, Yeo CD, Park CK, Lee SH, Kim SJ, et al. Characteristics and clinical outcomes of patients with nonsmoking small cell lung cancer in Korea. BMC Pulm Med (2022) 22(1):200. doi: 10.1186/s12890-022-01989-x
66. Chen Z, Liu X, Shang X, Qi K, Zhang S. The diagnostic value of the combination of carcinoembryonic antigen, squamous cell carcinoma-related antigen, cyfra 21-1, neuron-specific enolase, tissue polypeptide antigen, and progastrin-releasing peptide in small cell lung cancer discrimination. Int J Biol Markers (2021) 36(4):36–44. doi: 10.1177/17246008211049446
Keywords: neural network, deep learning, predictive model, clinical tool, small cell lung cancer
Citation: Zhang D, Lu B, Liang B, Li B, Wang Z, Gu M, Jia W and Pan Y (2023) Interpretable deep learning survival predictive tool for small cell lung cancer. Front. Oncol. 13:1162181. doi: 10.3389/fonc.2023.1162181
Received: 09 February 2023; Accepted: 24 April 2023;
Published: 05 May 2023.
Edited by:
Manash K. Paul, California State University, Los Angeles, United StatesReviewed by:
Gour Sundar Mitra Thakur, Dr B C Roy Engineering College, Durgapur, IndiaCopyright © 2023 Zhang, Lu, Liang, Li, Wang, Gu, Jia and Pan. This is an open-access article distributed under the terms of the Creative Commons Attribution License (CC BY). The use, distribution or reproduction in other forums is permitted, provided the original author(s) and the copyright owner(s) are credited and that the original publication in this journal is cited, in accordance with accepted academic practice. No use, distribution or reproduction is permitted which does not comply with these terms.
*Correspondence: Yuanming Pan, cGV0ZXJmcGFuMjAyMEBtYWlsLmNjbXUuZWR1LmNu; Wei Jia, amlhd2VpbXJAMTI2LmNvbQ==
†These authors have contributed equally to this work and share first authorship
Disclaimer: All claims expressed in this article are solely those of the authors and do not necessarily represent those of their affiliated organizations, or those of the publisher, the editors and the reviewers. Any product that may be evaluated in this article or claim that may be made by its manufacturer is not guaranteed or endorsed by the publisher.
Research integrity at Frontiers
Learn more about the work of our research integrity team to safeguard the quality of each article we publish.