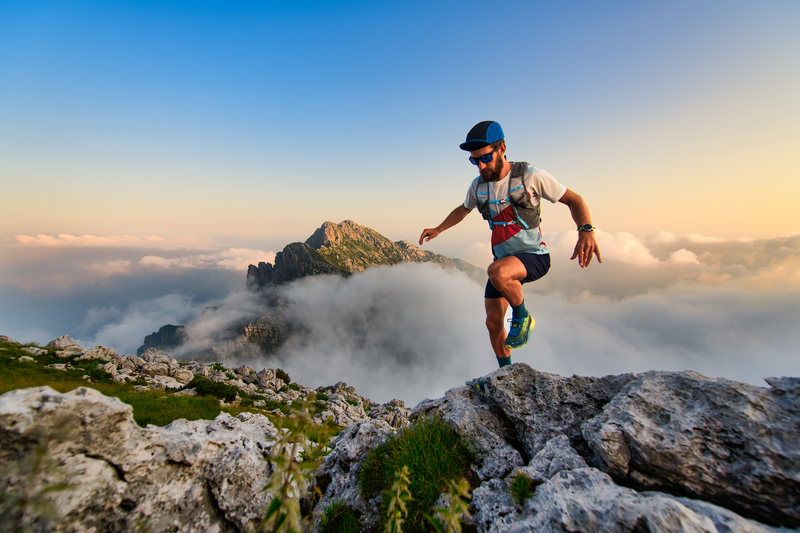
95% of researchers rate our articles as excellent or good
Learn more about the work of our research integrity team to safeguard the quality of each article we publish.
Find out more
METHODS article
Front. Oncol. , 04 April 2023
Sec. Thoracic Oncology
Volume 13 - 2023 | https://doi.org/10.3389/fonc.2023.1146053
This article is part of the Research Topic Real-World Data and Real-World Evidence in Lung Cancer View all 23 articles
Introduction: Population-based datasets are often used to estimate changes in utilization or outcomes of novel therapies. Inclusion or exclusion of unstaged patients may impact on interpretation of these studies.
Methods: A large population-based dataset in Ontario, Canada of non-small cell lung cancer patients was examined to evaluate the characteristics and outcomes of unstaged patients compared to staged patients. Multivariable Poisson regression was used to evaluate differences in patient-level characteristics between groups. Kaplan-Meier estimates of survival and log-rank statistics were utilized.
Results: In our Ontario cohort of 51,152 patients with NSCLC, 11.2% (n=5,707) were unstaged, and there was evidence that stage data was not missing completely at random. Those without assigned stage were more likely than staged patients to be older (RR [95%CI]), (70-79 vs. 20-59: 1.51 [1.38-1.66]; 80+ vs. 20-59: 2.87 [2.62-3.15]), have a higher comorbidity index (Score 1-2 vs 0: 1.19 [1.12-1.27]; 3 vs. 0: 1.49 [1.38-1.60]), and have a lower socioeconomic class (4 vs. 1 (lowest): 0.91 [0.84-0.98]; 5 vs. 1 (lowest): 0.89 [0.83-0.97]). Overall survival of unstaged patients suggested a mixture of early and advanced stage, but with a large proportion that are probably stage IV patients with more rapid death than those with reported stage IV disease.
Conclusion: In this case study, evaluation of stage-specific health care utilization and outcomes for staged patients with stage IV disease at the population level may have a bias as a distinct subset of stage IV patients with rapid death are likely among those without a documented stage in administrative data.
Population-based data are often used to explore stage-based outcomes of large groups of patients, and to describe treatment utilization rates for these groups in routine practice (1). However, many databases may be incomplete with less than 100% capture of variables such as stage (2).
Understanding the impact of missing stage information on studies estimating health care utilization or population-based outcomes in cancer patient data sets may be useful in interpreting various methods of estimating these rates and outcomes. Some databases may be missing stage information due to uniformly incomplete data collection of staged patients, while others may be missing stage information if patients are unstaged for medical reasons such as advanced rapidly progressive disease not amenable to active treatment. The latter condition represents data missing not completely at random, where the variable distribution (in this case stage) is different. Missing data in this case may be informative (3). If the act of being staged is associated with being ‘fit’ enough to receive treatment, then studies examining associated utilization rates or outcomes limited to patients with advanced disease with stage information may produce biased estimates compared to the true population value.
Here we provide a case study exploring patient characteristics and survival of patients stratified according to the presence of stage data. Given the high incidence and mortality of lung cancer, we explored this in a population-based sample of patients with non-small cell lung cancer (NSCLC) in the Canadian province of Ontario.
A population-based cohort of patients from the Ontario Cancer Registry (OCR) diagnosed with NSCLC between January 1, 2007, and December 31, 2016, were included. Ontario has a single-payer universal health care system with a population of over 14 million. We included patients with only one NSCLC diagnosis, with no history of previous chemotherapy, radiation therapy or surgery treatments. Patients were required to have a minimum of 5 years of continuous health insurance coverage prior to diagnosis to provide sufficient look back for comorbidity scoring, to be 20 years of age or older, and have a place of residence in Ontario. This study was approved by the Queen’s University Health Sciences and Affiliated Teaching Hospitals Research Ethics Board.
ICES is an independent, non-profit research institute whose legal status under Ontario’s health information privacy law allows it to collect and analyze health care and demographic data, without consent, for health system evaluation and improvement. These datasets were linked using unique encoded identifiers and analyzed at ICES.
Stage was assigned on available data from Collaborative Stage in OCR and pathological/clinical stage in the Activity Level Reporting (ALR) data. This uses information derived from clinic-reported stage and manual chart review to assign stage based on the most reliable information (e.g. chart review data may be used in priority over cancer centre reported stage). Patient demographic data at the time of diagnosis were obtained from Ministry of Health administrative data. Comorbidity was assigned based on the Elixhauser comorbidity index (a validated algorithm to classify comorbidity using International Classification of Disease codes in administrative data) with a five-year lookback with Canadian Institute for Health Information Discharge Abstract Database (DAD) and Same Day Surgery (SDS) data (4). Diagnostic codes for lymphoma, metastatic cancer and solid tumours without metastasis were not included in the score. Neighbourhood income quintile was utilized as an area-level measure of socioeconomic status. Categorization of place of residence as urban, sub-urban or rural was based on the 2008 Rurality Index for Ontario (5). Chronic diseases (e.g., asthma and congestive heart failure) were identified with ICES-derived datasets based validated algorithms.
Overall survival and cancer-specific survival were measured from the date of diagnosis. Follow-up data were censored at 4 years for overall survival and 2 years for cancer-specific survival. Follow-up was shorter for cancer-specific survival as cause-specific death information from Ontario’s Office of the Registrar General-Death (ORGD) is complete only up to December 31, 2018.
Demographic and general health data were summarized by stage (including unstaged information). Multivariable Poisson regression was used to evaluate the differences in the patient-level characteristics between the unstaged and staged groups. Kaplan-Meier estimates of survival were determined according to stage. Log-rank statistics were utilized. All analyses were performed using the SAS software 9.4 (SAS Institute, Cary NC).
Of 51,152 NSCLC patients, 11.2% (5,707) were unstaged (Table 1). Unstaged patients were significantly more likely to be older (Relative Risk (RR) [95% Confidence Interval (CI)]: 70-79 vs. 20-59, 1.51 [1.38-1.66]; 80+ vs. 20-59, 2.87 [2.62-3.15]), reside in lower income neighbourhoods (RR [95% CI]: 4th vs. 1st quintile, 0.91 [0.84-0.98]; 5th vs. 1st quintile, 0.89 [0.83-0.97]) and rural areas (RR [95% CI]: urban vs. rural, 0.58 [0.54-0.61]; sub-urban vs. rural, 0.71 [0.66-0.77]), and have a higher comorbidity index (RR [95% CI]: 1-2 vs. 0, 1.19 [1.12-1.27]; 3+ vs. 0, 1.49 [1.38-1.60]) (Table 2). The occurrence of missing stage also changed over time, becoming increasingly less likely during the study period (RR year of diagnosis, per 1-year increase [95% CI]: 0.92 [0.91-0.92]). Among the unstaged group, 89.4% (5,102) died within 4 years from diagnosis. Earlier stage patients at diagnosis (stage I/II/III) comprised ~32.8% of deaths.
Table 1 Demographic and general health characteristics for non-small cell lung cancer (NSCLC) patients in 2007-2016.
Table 2 Comparison of demographic and general health characteristics according to stage information for non-small cell lung cancer (NSCLC) patients in 2007-20161.
Survival curves are shown in Figures 1A, B. For stage III and IV patients, the one-year overall survival (OS) are 47.3% and 20.2% (Figure 1A), while the one-year cancer-specific survival (CSS) are 51.8% and 22.8%, respectively (Figure 1B). Noticeable in the Kaplan Meier curves is the different shape of the curve for unstaged patients, with a steeper initial drop than stage IV patients, but with a similar one-year survival to stage IV patients (one-year OS: 21.6% vs. 20.2%) and a higher survival in the tail of the curve (four-year OS: 10.6% vs. 3.9%).
Figure 1 Kaplan-Meier survival curves according to stage information for non-small cell lung cancer (NSCLC) patients in 2007-2016 (A) Overall survival. Data is censored 4 years from diagnosis. (B) Cancer-specific survival. Data is censored 2 years from diagnosis.
In this case study of a large population-based cohort of NSCLC patients, stage data is not missing completely at random. Evaluation of stage-specific health care utilization and outcomes for staged patients, particularly those with stage IV disease, at the population level may thus have a bias as a distinct subset of stage IV patients with rapid death are likely among those without a documented stage in administrative data.
Healthcare utilization differences between staged and unstaged groups was not evaluated in our study. However, it is known that costs (and therefore utilization) vary by lung cancer stage in Canada. A recent study found that unstaged patients with lung cancer had higher costs than stage I and II patients with lung cancer, likely due to the high costs of end-of-life care (6). Treatment receipt for both staged and unstaged groups is delivered based on accepted provincial, national, and international guidelines. These guidelines are based on important prognostic factors not fully available in our cohort, but both groups (staged and unstaged) would have access to fully reimbursed standard of care treatment options. The unstaged group accounted for approximately 11.2% of cases and approximately 12.1% of deaths. These patients have higher comorbidity, rurality, and age than staged patients. It is well known that variation in care and service delivery exists in a single-payer public universal health care system and are associated with patient-level characteristics (7, 8).
Missing stage was also more likely to occur earlier in the study period. Based on the shape of survival curves, we hypothesize that the group without stage data likely represents at least two populations; a rapidly dying advanced cancer cohort dying too quickly to be formally staged or treated in a cancer centre, as well as an earlier stage cohort with better survival with omitted staging due to technical, rather than clinical, reasons. This potential mixture of early and advanced cases argues against simply combining unstaged patients with stage IV patients in studies of stage IV management and outcome.
In population-based studies on palliative systemic therapy utilization in Ontario and possibly other jurisdictions, using a metric of the number of patients who received such therapy divided by all stage IV patients can overestimate utilization (9, 10). This is because the ‘denominator’ of database-recorded stage IV lung cancer may be lower than the ‘true’ number of stage IV patients in the population as a component of patients with true stage IV disease may be missing stage information. In certain populations, like the aged (80+), the bias may be significantly higher, as 40.2% of the unstaged patients were 80+, representing 20.1% of the lung cancers diagnosed in that group.
Cancer stage determination in Ontario is captured by the OCR who receive pathological and clinical (stage assigned by the managing physician) reporting from regional cancer centers across Ontario (11). This process often relies on OCR registrar staff to incorporate and assess clinical, pathological and post-therapy stage information. Other Canadian provincial cancer registries as well as large American cancer registries (National Cancer Database (NCDB) and Surveillance, Epidemiology, and End Results Program (SEER)) have collected stage information following similar processes to Ontario, using trained tumor registrars to abstract specified data elements from patient records in accordance with registry data standards (12).
Our study supports previous findings from other high-income countries of improved (decreasing) rates of missing stage data over time, likely due to improvements in coding standards and cancer registry quality (12, 13). However, as much as stage data capture is improving, it will never be entirely complete due to clinical (e.g., physician failing to assign a category) and data-registry (e.g., miscoded fields) level factors. In the NCDB, high levels of missing data were found for NSCLC and other major cancer sites that also appear not to be missing completely at random. (12). The SEER is also faced with similar challenges with regards to missing data (14). It is highly likely that the COVID-19 pandemic has compromised and continues to affect cancer stage recording and capture, as it has already impacted recent studies (15). Therefore, we expect the trend of decreasing missing cancer data to reverse, and further emphasize the importance of understanding the implications and nature of missing data.
While large databases and staging are helpful in determining real world utilization of palliative systemic therapy and real-world outcomes, there are factors that may bias data collection and interpretation and may lead to over- or under-estimation of treatment utilization. Using only staged patients with stage IV disease to determine palliative systemic treatment utilization in NSCLC may lead to different estimates of utilization in comparison to other methods, such as the ‘lookback’ method from death – which will miss those who have not died, but includes those who receive palliative therapy for unresectable or recurrent disease. Another approach is to look forward from the time of first palliative therapy, which will miss those who receive no palliative therapy, but may include those who had earlier stage disease and subsequently recurred, and those with incurable locally advanced disease (e.g., some stage IIIB). Each of these methods of estimating palliative systemic therapy utilization may lead to different estimates and should be seen as complimentary in determining the real ‘real world’ utilization.
In this case study, there was evidence that stage data was not missing completely at random. Evaluation of stage-specific health care utilization and outcomes for staged patients with stage IV disease at the population level may have a bias as a distinct subset of stage IV patients with rapid death are likely among those without a documented stage in administrative data.
The dataset from this study is held securely in coded form at ICES. While legal data sharing agreements between ICES and data providers (e.g., healthcare organizations and government) prohibit ICES from making the dataset publicly available, access may be granted to those who meet pre-specified criteria for confidential access, available at www.ices.on.ca/DAS (email: ZGFzQGljZXMub24uY2E=). The full dataset creation plan and underlying analytic code are available from the authors upon request, understanding that the computer programs may rely upon coding templates or macros that are unique to ICES and are therefore either inaccessible or may require modification.
AR: conceptualization, formal analysis, methodology, writing original draft, writing, review and editing. TH: funding acquisition, investigation, writing review and editing. PN: writing, review and editing, data curation, formal analysis, methodology. CG: funding acquisition, writing review and editing. MJ: writing, review and editing, interpretation. All authors contributed to the article and approved the submitted version.
This study was supported by ICES, which is funded by an annual grant from the Ontario Ministry of Health (MOH) and the Ministry of Long-Term Care (MLTC). TH holds a research chair provided by the Ontario Institute for Cancer Research through funding provided by the Government of Ontario (#IA-035). Parts of this material are based on data and information compiled and provided by: MOH, MLTC, CIHI, CCO, ORG and Statistics Canada. The analyses, conclusions, opinions and statements expressed herein are solely those of the authors and do not reflect those of the funding or data sources; no endorsement is intended or should be inferred. Parts of this material are based on data and/or information compiled and provided by CIHI. However, the analyses, conclusions, opinions and statements expressed in the material are those of the author(s), and not necessarily those of CIHI. Parts of this material are based on data and information provided by Cancer Care Ontario (CCO). The opinions, results, view, and conclusions reported in this paper are those of the authors and do not necessarily reflect those of CCO. No endorsement by CCO is intended or should be inferred. Parts of this report are based on Ontario Registrar General (ORG) information on deaths, the original source of which is Service Ontario. The views expressed therein are those of the author and do not necessarily reflect those of ORG or the Ministry of Government Services.
AR reports speaker fees from Astra-Zeneca, Merck and BMS, outside the submitted work.
The remaining authors declare that the research was conducted in the absence of any commercial or financial relationships that could be construed as a potential conflict of interest.
All claims expressed in this article are solely those of the authors and do not necessarily represent those of their affiliated organizations, or those of the publisher, the editors and the reviewers. Any product that may be evaluated in this article, or claim that may be made by its manufacturer, is not guaranteed or endorsed by the publisher.
1. Shah M, Parmar A. Socioeconomic disparity trends in diagnostic imaging, treatments, and survival for non-small cell lung cancer 2007-2016. Cancer Med (2020) 9(10):3407–16. doi: 10.1002/cam4.2978
2. Jairam V, Park HS. Strengths and limitations of large databases in lung cancer radiation oncology research. Transl Lung Cancer Res (2019) 8(Suppl 2):S172–s183. doi: 10.21037/tlcr.2019.05.06
3. Bhaskaran K, Smeeth L. What is the difference between missing completely at random and missing at random? Int J Epidemiol (2014) 43(4):1336–9. doi: 10.1093/ije/dyu080
4. Moore BJ, White S, Washington R, Coenen N, Elixhauser A. Identifying increased risk of readmission and in-hospital mortality using hospital administrative data: The AHRQ elixhauser comorbidity index. Med Care (2017) 55(7):698–705. doi: 10.1097/MLR.0000000000000735
5. Kralj B. Measuring rurality–RIO2008 BASIC: Methodology and results (2008). Available at: http://www.eriestclairlhin.on.ca/Page.aspx?id=11606 (Accessed 11, 2022).
6. Mittmann N, Liu N, Cheng SY, Seung SJ, Saxena FE, Look Hong NJ, et al. Health system costs for cancer medications and radiation treatment in Ontario for the 4 most common cancers: a retrospective cohort study. Can Med Assoc Open Access J (2020) 8(1):E191–8. doi: 10.9778/cmajo.20190114
7. Forrest LF, Adams J, Wareham H, Rubin G, White M. Socioeconomic inequalities in lung cancer treatment: Systematic review and meta-analysis. PloS Med (2013) 10(2):e1001376. doi: 10.1371/journal.pmed.1001376
8. Lofters AK, Gatov E, Lu H, Baxter NN, Guilcher SJT, Kopp A, et al. Lung cancer inequalities in stage of diagnosis in Ontario, Canada. Curr Oncol (2021) 28(3):1946–56. doi: 10.3390/curroncol28030181
9. Sacher AG, Le LW, Lau A, Earle CC, Leighl NB. Real-world chemotherapy treatment patterns in metastatic non-small cell lung cancer: Are patients undertreated? Cancer (2015) 121(15):2562–9. doi: 10.1002/cncr.29386
10. Kehl KL, Hassett MJ, Schrag D. Patterns of care for older patients with stage IV non-small cell lung cancer in the immunotherapy era. Cancer Med (2020) 9(6):2019–29. doi: 10.1002/cam4.2854
11. Ontario Cancer registry data surveillance and cancer registry. Ontario: Ontario Health Cancer Care Ontario (2020).
12. Yang DX, Khera R, Miccio JA, Jairam V, Chang E, Yu JB, et al. Prevalence of missing data in the national cancer database and association with overall survival. JAMA Network Open (2021) 4(3):e211793–e211793. doi: 10.1001/jamanetworkopen.2021.1793
13. Piñeros M, Parkin DM, Ward K, Chokunonga E, Ervik M, Farrugia H, et al. Essential TNM: A registry tool to reduce gaps in cancer staging information. Lancet Oncol (2019) 20(2):e103–11. doi: 10.1016/S1470-2045(18)30897-0
14. Kim HM, Goodman M, Kim BI, Ward KC. Frequency and determinants of missing data in clinical and prognostic variables recently added to SEER. J Registry Manag (2011) 38(3):120–31.
Keywords: missing data, non-small cell lung cancer, administrative data, population-based, cancer stage
Citation: Robinson AG, Nguyen P, Goldie CL, Jalink M and Hanna TP (2023) Is cancer stage data missing completely at random? A report from a large population-based cohort of non-small cell lung cancer. Front. Oncol. 13:1146053. doi: 10.3389/fonc.2023.1146053
Received: 16 January 2023; Accepted: 22 February 2023;
Published: 04 April 2023.
Edited by:
Henry Soo-Min Park, Yale University, United StatesReviewed by:
Janaki Deepak, University of Maryland, United StatesCopyright © 2023 Robinson, Nguyen, Goldie, Jalink and Hanna. This is an open-access article distributed under the terms of the Creative Commons Attribution License (CC BY). The use, distribution or reproduction in other forums is permitted, provided the original author(s) and the copyright owner(s) are credited and that the original publication in this journal is cited, in accordance with accepted academic practice. No use, distribution or reproduction is permitted which does not comply with these terms.
*Correspondence: Andrew G. Robinson, YW5kcmV3LnJvYmluc29uQGtpbmdzdG9uaHNjLmNh
Disclaimer: All claims expressed in this article are solely those of the authors and do not necessarily represent those of their affiliated organizations, or those of the publisher, the editors and the reviewers. Any product that may be evaluated in this article or claim that may be made by its manufacturer is not guaranteed or endorsed by the publisher.
Research integrity at Frontiers
Learn more about the work of our research integrity team to safeguard the quality of each article we publish.