- 1Department of Laboratory Medicine, Ruijin Hospital, Shanghai Jiaotong University School of Medicine, Shanghai, China
- 2Department of Obstetrics & Gynecology, Ruijin Hospital, Shanghai Jiaotong University School of Medicine, Shanghai, China
- 3Genecast Biotechnology Co., Ltd., Wuxi, China
Background: Gynecological cancers are the most lethal malignancies among females, most of which are associated with gene mutations. Few studies have compared the differences in the genomic landscape among various types of gynecological cancers. In this study, we evaluated the diversity of mutations in different gynecological cancers.
Methods: A total of 184 patients with gynecological cancer, including ovarian, cervical, fallopian tube, and endometrial cancer, were included. Next-generation sequencing was performed to detect the mutations and tumor mutational burden (TMB). Kyoto Encyclopedia of Genes and Genomes (KEGG) and Gene Ontology (GO) enrichment analyses were also conducted.
Results: We found that 94.57% of patients had at least one mutation, among which single nucleotide variants, insertions and InDels were in the majority. TP53, PIK3CA, PTEN, KRAS, BRCA1, BRCA2, ARID1A, KMT2C, FGFR2, and FGFR3 were the top 10 most frequently mutated genes. Patients with ovarian cancer tended to have higher frequencies of BRCA1 and BRCA2 mutations, and the frequency of germline BRCA1 mutations (18/24, 75.00%) was higher than that of BRCA2 (11/19, 57.89%). A new mutation hotspot in BRCA2 (I770) was firstly discovered among Chinese patients with gynecological cancer. Patients with TP53, PIK3CA, PTEN, and FGFR3 mutations had significantly higher TMB values than those with wild-type genes. A significant cross was discovered between the enriched KEGG pathways of gynecological and breast cancers. GO enrichment revealed that the mutated genes were crucial for the cell cycle, neuronal apoptosis, and DNA repair.
Conclusion: Various gynecological cancer types share similarities and differences both in clinical characterization and genomic mutations. Taken together with the results of TMB and enriched pathways, this study provided useful information on the molecular mechanism underlying gynecological cancers and the development of targeted drugs and precision medicine.
1 Introduction
Ovarian (OC), cervical (CC), and endometrial cancer (EC) are the most common gynecological cancers in the female reproductive system (1, 2). OC is the most lethal gynecological malignancy in developed countries (3), with a 5-year survival rate of ~47% (4). Since ovaries are relatively small and located deep in the pelvic cavity, up to 59% of OCs are only detected at advanced stages, with a low survival rate (5). Epithelial ovarian carcinoma (EOC) accounts for the majority of OCs and can be divided into serous, endometrioid, clear cell, and mucinous carcinoma, amongst others. Serous carcinomas constitute about 75% of EOCs and are further divided into low-grade and high-grade serous carcinomas (LGSC and HGSC) depending on their histological differences (6). CC is the fourth most common cancer among females, affecting approximately 600,000 women annually (7). Although screening programs and human papillomavirus (HPV) vaccines have helped reduce its incidence (8), approximately 310,000 patients with CC die annually (9). CC tends to develop at a younger age (10, 11); however, older patients also often have dismal prognoses (12, 13). EC, which is second only to CC in terms of the incidence of reproductive system cancers, ranks seventh among the most prevalent malignancies among females (14–16). EC can be divided into two types: type I estrogen-dependent EC (EEC) and type II non-estrogen-dependent EC (NEEC) (17). The proportion of patients with EEC is higher, and they are often younger and present with hypertension, diabetes, obesity, and infertility (18). NEEC has higher rates of metastasis and recurrence, poorer prognoses, and is more common among older women (19, 20). Fallopian tube cancer (FTC), which originates in the salpingeal mucosa (21), exhibits clinical behaviors similar to that of OC (22). But mutated fallopian tube epithelial cells were reported to form malignant tumors with a shorter latency and higher penetrance than that of ovarian surface epithelium. Although FTC is a relatively rare gynecological cancer, its incidence increased 4.19-fold from 2001 to 2014 (23).
Cancers are genetic diseases. Gene mutations alter the structure or function of related and encoded proteins, resulting in excessive/persistent stimulation signals for cell growth and transformation. With the development of molecular biology, the use of genetic testing to determine mutations in related tumors has become a topic of interest. Targeted drugs for specific genes and mutations are effective ways to treat cancer. Approximately 10 to 15% of OC are reported to be hereditary, and patients with OC are carriers of germline mutations in BRCA1 and BRCA2 (24). BRCA1 and BRCA2 mutations increase the lifetime risk of peritoneal malignancies and FTC (25, 26). In 2014, olaparib, the first poly ADP-ribose polymerase (PARP) inhibitor, was approved for the treatment of BRCA-mutated OC (27). PPP2R1A and TP53 mutations are dramatically higher in patients with advanced-stage EC (19). PIK3CA, KMT2C, and KMT2D are the most frequently mutated genes in CC (28).
Next-generation sequencing (NGS) is a high-throughput sequencing technology that plays a vital role in cancer research (29). NGS can identify genomic alterations occurring in any region of a target gene, detect one mutated copy among thousands of wild-type copies, and elucidate many types of mutational landscapes of tumors. NGS has become an important aspect of accurate tumor diagnosis and treatment and has a variety of uses, such as tumor-targeted therapy-related driver gene detection, analysis of drug resistance mechanisms, tumor metastasis and prognosis assessment, and molecular diagnostics. In this study, we investigated 184 patients with gynecological malignancies using NGS and created a genomic landscape to show the diversity among different gynecological cancers, providing useful information for future clinical treatment.
2 Materials and methods
2.1 Patients and sampling
A total of 184 patients diagnosed with gynecological cancers at Ruijin Hospital, Shanghai Jiaotong University School of Medicine between January 2020 and June 2022 were enrolled in this study. All included patients gave their informed consent. The study was reviewed and approved by the Ethical Committee of the Ruijin Hospital, Shanghai Jiaotong University School of Medicine. Tissue samples were collected during surgical procedures and were subjected to NGS alongside paired blood samples. Patient information was acquired from medical records. Pathology diagnosis including the tumor site, pathological type, tumor differentiation grade, as well as Federation of International of Gynecologists and Obstetricians (FIGO) grade, were reviewed by two expert pathologists from the pathology department.
2.2 DNA extraction
Imprint cytology was performed to evaluate tumor purity before DNA extraction. Briefly, freshly cut surfaces of tissue specimens were gently pressed to glass slides. Then the slides was stained with hematoxylin and eosin (HE) after fixing with 95% of ethyl alcohol for 5–6 s. If the percentage of tumor cells was higher than 15%, the specimen was considered qualified for subsequent extraction and sequencing. Genomic DNA was extracted from fresh tumor tissue using the TIANamp Genomic DNA Kit (TIANGEN, China). Genomic DNA from peripheral blood lymphocytes (PBL) was extracted using a TGuide S32 Magnetic Blood Genomic DNA Kit (TIANGEN, China). The concentration of DNA was measured using a Qubit dsDNA HS Assay Kit (Thermo Fisher, USA), whereas the DNA quality was assessed using an Agilent 2100 BioAnalyzer (Agilent, USA). All extractions and assays were conducted according to the manufacturers’ instructions supplied in the respective kits used in this study.
2.3 Library preparation and sequencing
Genomic DNA extracted from each tumor or PBL sample was sheared with Covaris LE220 to a length of 200 bp, and fragmented DNA was used to construct a library using the KAPA Hyper Preparation Kit (Kapa Biosystems, USA). Target regions were captured using the HyperCap Target Enrichment Kit (Roche, Switzerland). The customized panel used in the capture process includes 543 genes (30), which are tumor-related major genes, and spans around a 1.67 MB genomic region of the human genome (Supplemental Table 1; Genecast Biotechnology Co., Ltd., Beijing, China). Bioinformatic analyses of these 543 genes were carried out at a College of American Pathologists (CAP)-certified laboratory (Genecast Biotechnology). Hybridization and washing were conducted according to the manufacturer’s protocol. The captured library was sequenced on the instrument of Illumina Novaseq 6000, which produces paired-end reads with the length of each end as 150bp, according to the manufacturer’s protocol.
Clean sequenced reads were mapped to the human reference genome (hg19) using BWA (v0.7.17) (31). VarDict (version 1.5.1) was used to call single nucleotide variant (SNV) mutations (32), whereas compound heterozygous mutations were merged using FreeBayes (version 1.2.0) (33). After annotation using ANNOVAR (2015 Jun17) (34), somatic mutations were selected based on the following standards: (i) located in intergenic/intronic regions; (ii) synonymous SNVs; (iii) allele frequency ≥ 0.002 in Exome Aggregation Consortum (ExAC) and genome aggregation database (gnomAD) (35, 36); (iv) allele frequency <0.05 in the tumor sample/allele frequency <0.01 in the plasma sample; (v) strand bias mutations in the reads; (vi) support reads <5; (vii) depth <30.
2.4 Tumor mutational burden calculation
Primarily, dynamic nonsynonymous mutations in the coding regions were selected for the following analysis of TMB, while driver gene mutations and germline alterations in the Single Nucleotide Polymorphism database (dbSNP) were removed. We filtered SNV mutations in all samples according to the following rules: (i) not splicing or exonic; (ii) depth <100 X/allele frequency <0.05; (iii) allele frequency ≥ 0.002 in the ExAC and gnomAD; and (iv) strand bias mutations in the reads. After quantification of the number of somatic nonsynonymous SNVs, the value was extrapolated to the whole exome using a validated algorithm (37). TMB, measured in mutations per Mb, was then calculated after obtaining absolute mutation counts against the mutation spots of the normal samples using the following formula:
2.5 Gene ontology and Kyoto encyclopedia of genes and genomes pathway enrichment analyses
GO functional enrichment and KEGG pathway analyses were performed using DAVID tools (https://david.ncifcrf.gov/). For GO analysis, contigs were categorized, and their molecular functions, cellular components, and biological processes were statistically analyzed.
2.6 Protein interaction
Search Tool for the Retrieval of Interacting Genes/Proteins (STRING, version 11.0, https://string‐db.org) was used to analyze functional interactions with a confidence of 0.7. “Ovarian cancer,” “cervical cancer,” “fallopian tube cancer,” and “endometrial cancer” were used as keywords in Chilibot (http://www.chilibot.net/) to analyze the interaction between genes and different gynecological cancers, excluding abstract co-occurrence relationships.
2.7 Statistical analysis
Descriptive statistics were presented as the median (interquartile range; IQR) for continuous variables, and as numbers (percentages) for categorical data. All data were analyzed using SPSS version 26.0 (SPSS Inc., Chicago, IL, USA). Mann–Whitney and Kruskal–Wallis nonparametric tests were conducted for comparisons between groups. Pearson’s Chi-square test was used for categorical variables. The Benjamini–Hochberg procedure was conducted to derive significance for enrichment tests. Spearman’s correlation coefficients with a two-tailed p value were determined for correlation analyses; p < 0.05 indicated significance. Data are visualized in graphs produced using GraphPad Prism version 8.0 and R software version 4.0.5.
3 Results
3.1 The clinical features of the analyzed cohort
Among the 184 patients, 140 had OC, 12 had CC, 8 had FTC, and 24 had EC. Clinicopathological characteristics are presented in Table 1. The median age at diagnosis was 60 (50–67) years old.
Patients with CC were younger at diagnosis, especially compared with those with OC and FTC. No significant differences were observed in menopausal status. Patients with OC had larger tumors, found at more advanced stages, while patients with CC and EC were diagnosed at earlier stages (p < 0.05). Metastasis occurred at both at node and organs in all patients with FTC. Patients with OC tended to have personal/family histories of cancer, especially breast cancer. Further analysis was performed among patients with OC according to their pathological types (Supplementary Table 2).
3.2 Gynecological cancers exhibit various genomic landscapes
Patient DNA from tumor tissues and matched peripheral blood were used for NGS. We detected 529 SNVs, 132 insertions and InDels, 36 truncations, 111 gene amplifications, 36 gene deletions, and 17 splice site mutations. Of all our patients, 94.57% (174/184) had at least one mutation (Figure 1). The top 10 most frequently altered genes in patients with gynecological cancer are presented in Figure 2. Patients with OC and FTC had higher frequencies of TP53 mutations, while patients with EC showed more PTEN alterations. Changes in KMT2C and FGFR3 were more frequent among patients with CC than in the other three types.
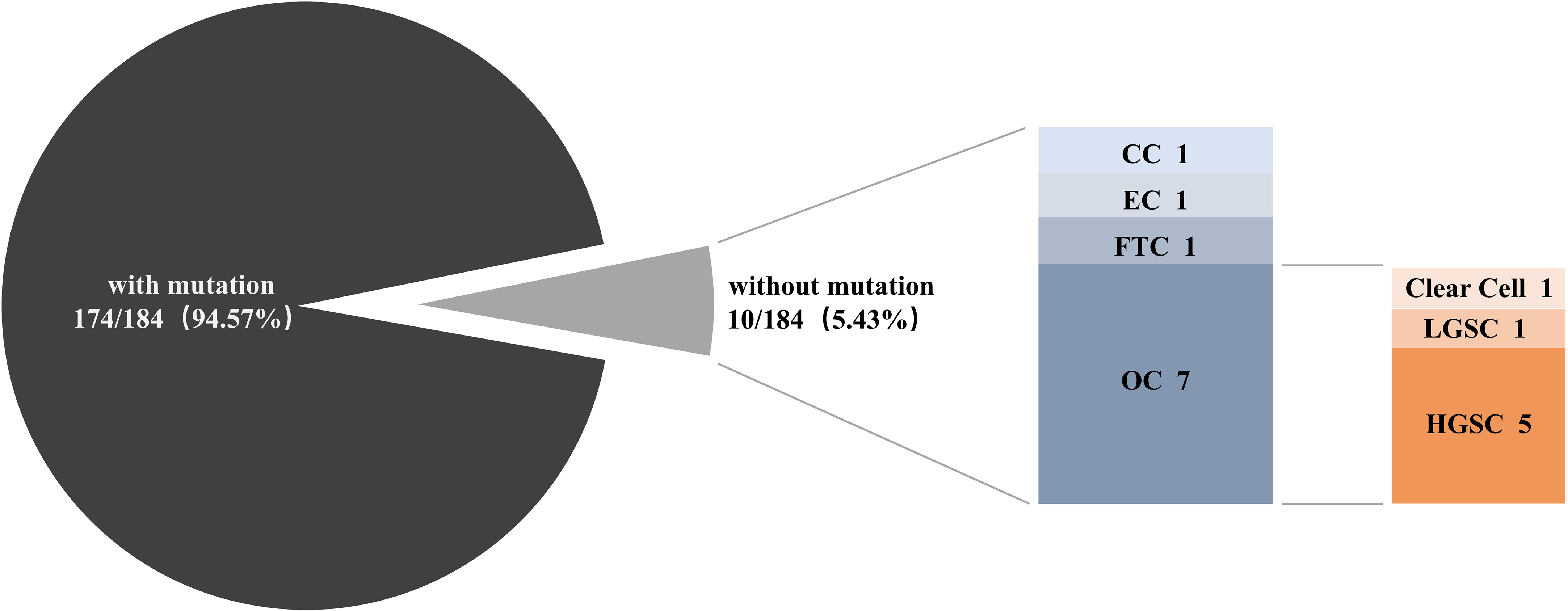
Figure 1 The proportion of patients with and without mutations. Next-generation sequencing was performed among 184 gynecological cancer patients to detect genomic alterations. OC, ovarian cancer; CC, cervical cancer; EC, endometrial cancer; FTC, fallopian tube cancer; LGSC, low-grade serous cancer; HGSC, high-grade serous cancer.
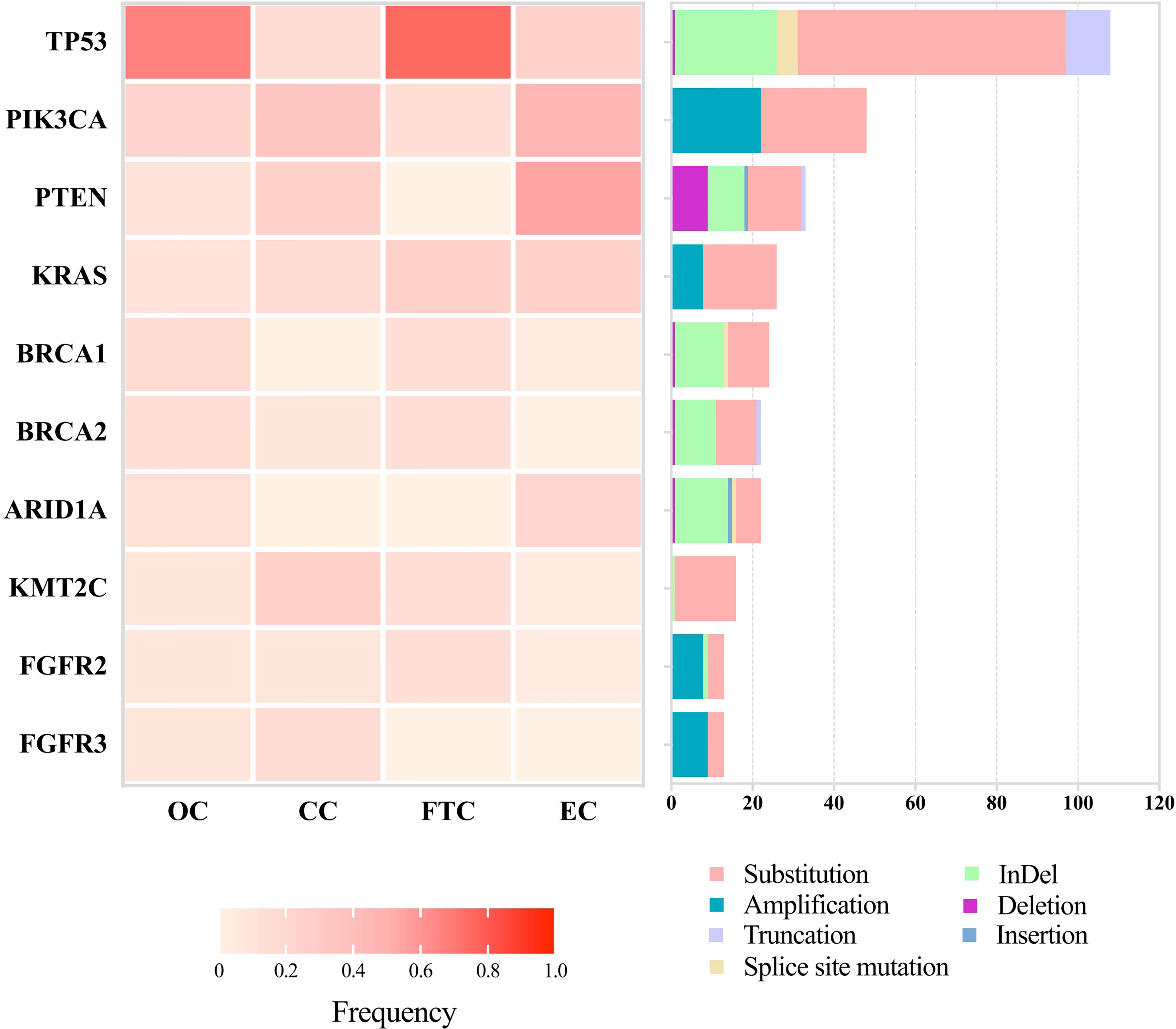
Figure 2 Landscapes of the top 10 most frequently mutated genes among 184 patients with gynecological cancer. Next-generation sequencing was performed to detect mutations. Frequencies of mutated genes are listed on the left, and mutation types are shown on the right, with annotation bars at the bottom. OC, ovarian cancer; CC, cervical cancer; EC, endometrial cancer; FTC, fallopian tube cancer.
Furthermore, different mutation types were uncovered among different genes. TP53 showed obvious alterations in SNVs and InDels. PIK3CA and PTEN revealed higher frequencies of copy number variations. BRCA1 and BRCA2 had similar patterns with slight differences, such as splice site mutations in BRCA1 and insertions in BRCA2. R273 and V173 in TP53, H1047 and E542 in PIK3CA, R183 in PPP2R1A, and G12 in KRAS were hotspots of mutations among these patients (data not shown). The top 10 most frequently altered genes among patients with OC were the same as those in all 184 patients, but in an order with slight changes (Supplementary Figure 1).
3.3 Analysis of BRCA1 and BRCA2 mutations
In total, 24 and 19 mutations were discovered in BRCA1 and BRCA2, respectively, most of which were found in patients with OC (Figure 3). Two patients with OC (HGSC and endometrioid carcinoma, respectively) carried both BRCA1 and BRCA2 mutations simultaneously. No BRCA1 or BRCA2 mutations were found in patients with CC. The proportion of germline mutations was higher than somatic mutations in BRCA1 and BRCA2. Moreover, the frequency of germline BRCA1 mutations (18/24, 75.00%) was higher than that of BRCA2 mutations (11/19, 57.89%). HGSC accounted for the majority of germline mutations in both BRCA1 and BRCA2 in patients with OC (Supplementary Figure 2). BRCA2 mutation c.2307delT p.I770Ffs*2 was the hotspot firstly reported here among Chinese patients with gynecological cancer.
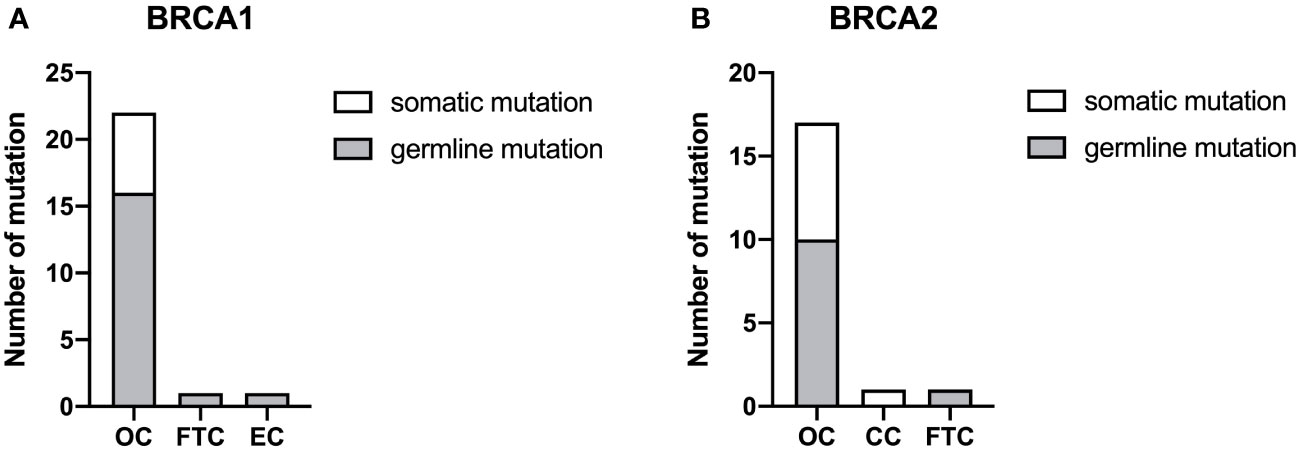
Figure 3 The proportion of BRCA1 (A) and BRCA2 (B) mutations. Somatic and germline mutations were respectively detected by next-generation sequencing among the different types of gynecological cancer. OC, ovarian cancer; CC, cervical cancer; EC, endometrial cancer; FTC, fallopian tube cancer.
3.4 TMB analysis
TMB range in this study spanned 0 to 192.35. To improve the accuracy, the data from one patient with endometrioid OC with statistical outliers (TMB = 192.35) was removed. The median TMB for all remaining patients was 2.94 (1.34–5.17). We ranked the TMB values from the lowest to highest and classified them into low, moderate, and high categories using quantiles ≤ 25%, 25–75%, and ≥75%, respectively. The ratio for TMB-low, TMB-moderate, and TMB-high was 32.79% (60/183), 54.64% (100/183), and 12.57% (23/183), respectively. No difference was observed between the median of the four gynecological cancer types (p = 0.200, Table 2); however, patients with EC tended to have a higher ratio of TMB-high values. Further analysis of TMB among patients with OC is shown in Supplementary Table 3. No correlation was observed between TMB and age, tumor size, menopausal status, metastasis, or FIGO stage (data not shown). Further, we analyzed the association between TMB and the top 10 most frequently changed genes in Figure 2. Compared with the wild-type, significant differences were discovered in the median of TMBs among patients with TP53, PIK3CA, PTEN, and FGFR3 mutations (p < 0.05, Figure 4).
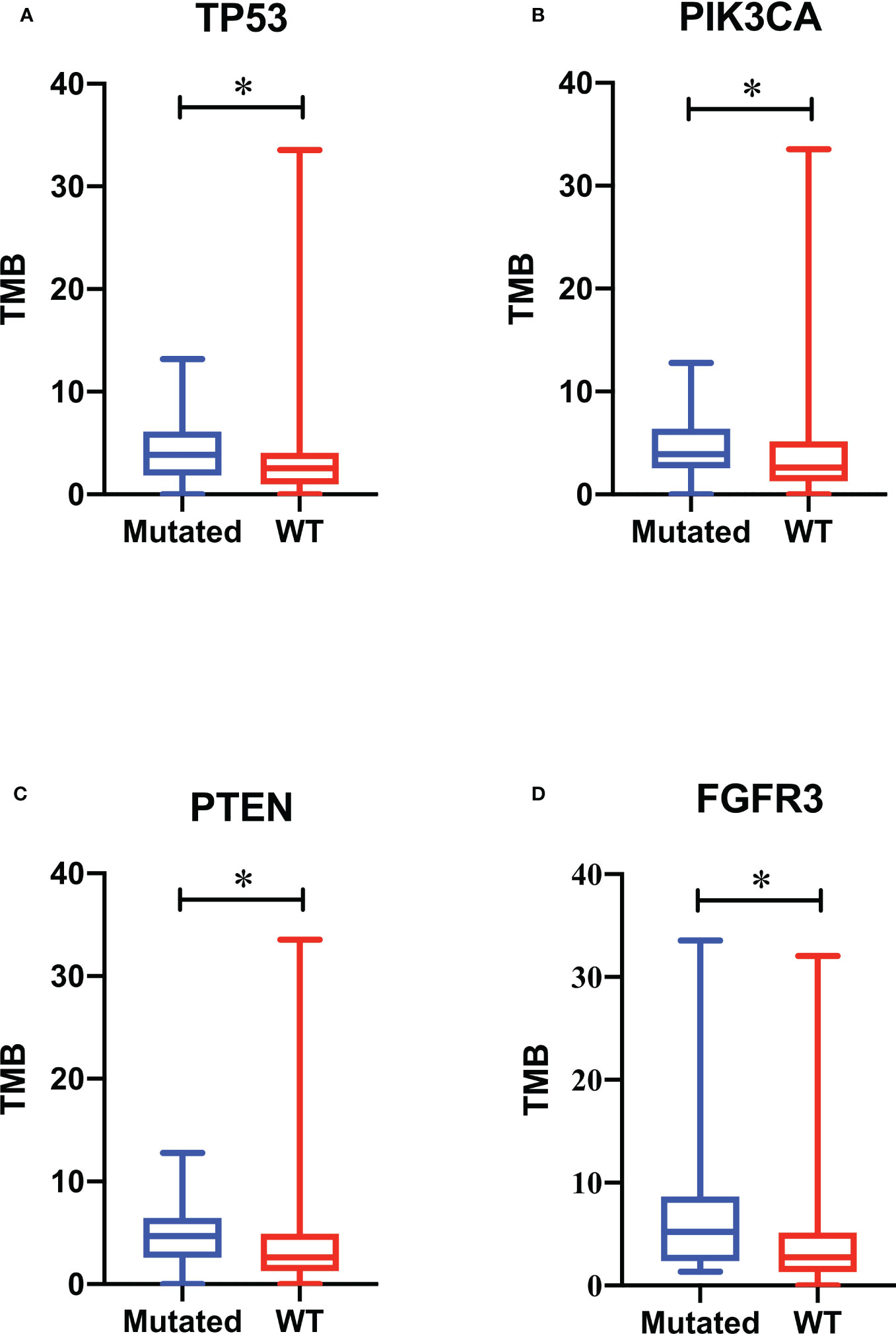
Figure 4 Association of gene mutations with tumor mutational burden (TMB). TMB values of patients with TP53 (A), PIK3CA (B), PTEN (C), and FGFR3 (D) mutations are respectively compared with those of patients with wild-type genes. A box plot was used to show the minimum, maximum, median, and interquartile range of the TMB values. The blue box represents patients with mutations, and the red box represents patients with wild-type genes. * p < 0.05.
3.5 Enrichment analysis and protein interaction
In this cohort, 529 SNVs and 132 insertions and InDels were detected, which accounted for the majority of mutations (661/861, 76.77%). Therefore, we performed an overlap analysis of the SNVs- and insertions and InDels-associated 29 genes. The KEGG and GO analyses of these genes are shown in Figure 5. A significant cross was discovered between the enriched pathways of gynecological and breast cancers (Figure 5A). Enrichment also revealed potential resistance to epidermal growth factor receptor (EGFR) tyrosine kinase inhibitors, endocrine, and platinum drugs. The top five enriched GO terms in biological processes, cellular components, and molecular functions are listed according to their p values (Figure 5B). Results showed that the mutated genes were crucial for neuronal apoptosis and DNA repair, as well as normal cell cycle. Protein interaction analysis identified TP53 as a crucial protein in the network (Figure 6A). SRC, RB1, CREBBP, ARID1A, SMARCA4, BRCA1, and ATM also contributed significantly to the interaction net. Chilibot analysis showed that most of these mutated genes had stimulatory or inhibitory relationships with different gynecological cancers (Figure 6B).
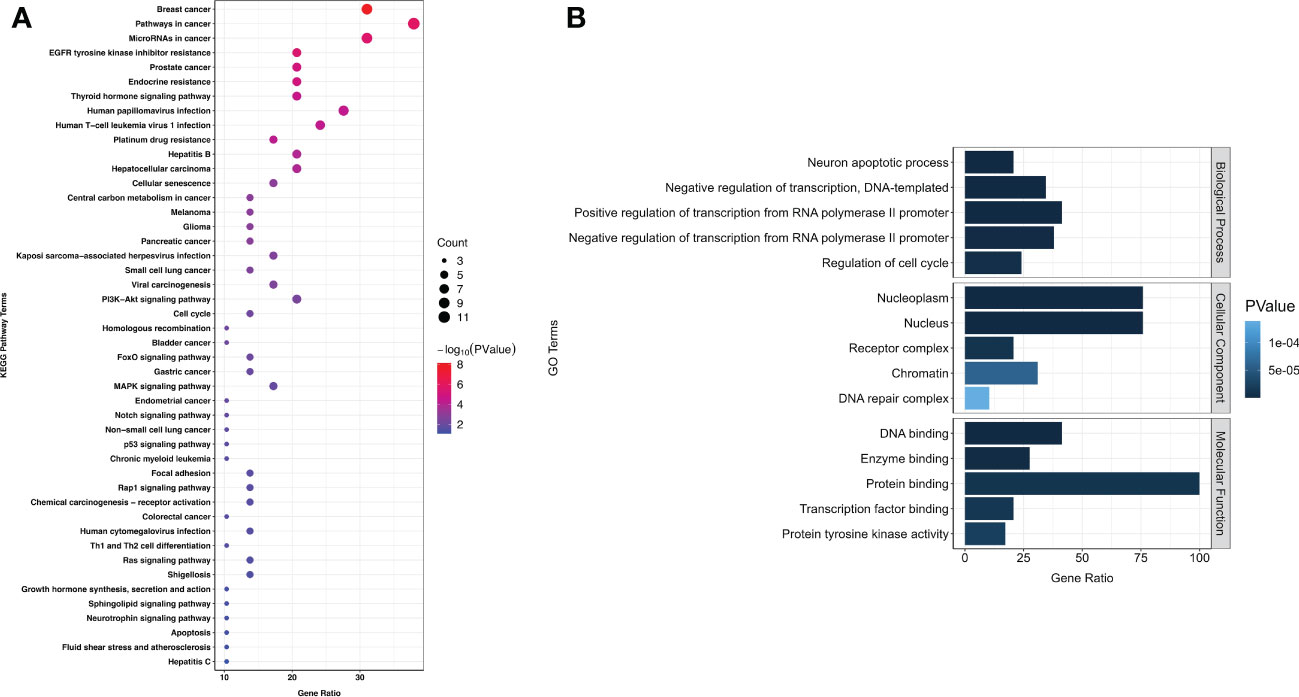
Figure 5 KEGG (A) and GO (B) enrichment among 184 patients with gynecological cancer. Next-generation sequencing was performed to detect mutations. Overlap of SNVs- and insertions and InDels-associated 29 genes was conducted for KEGG and GO enrichment. The size of each dot in KEGG enrichment indicates the number of genes included. The bigger the dot, the more genes are involved in the pathway. The top five GO enrichments are listed according to their p values.
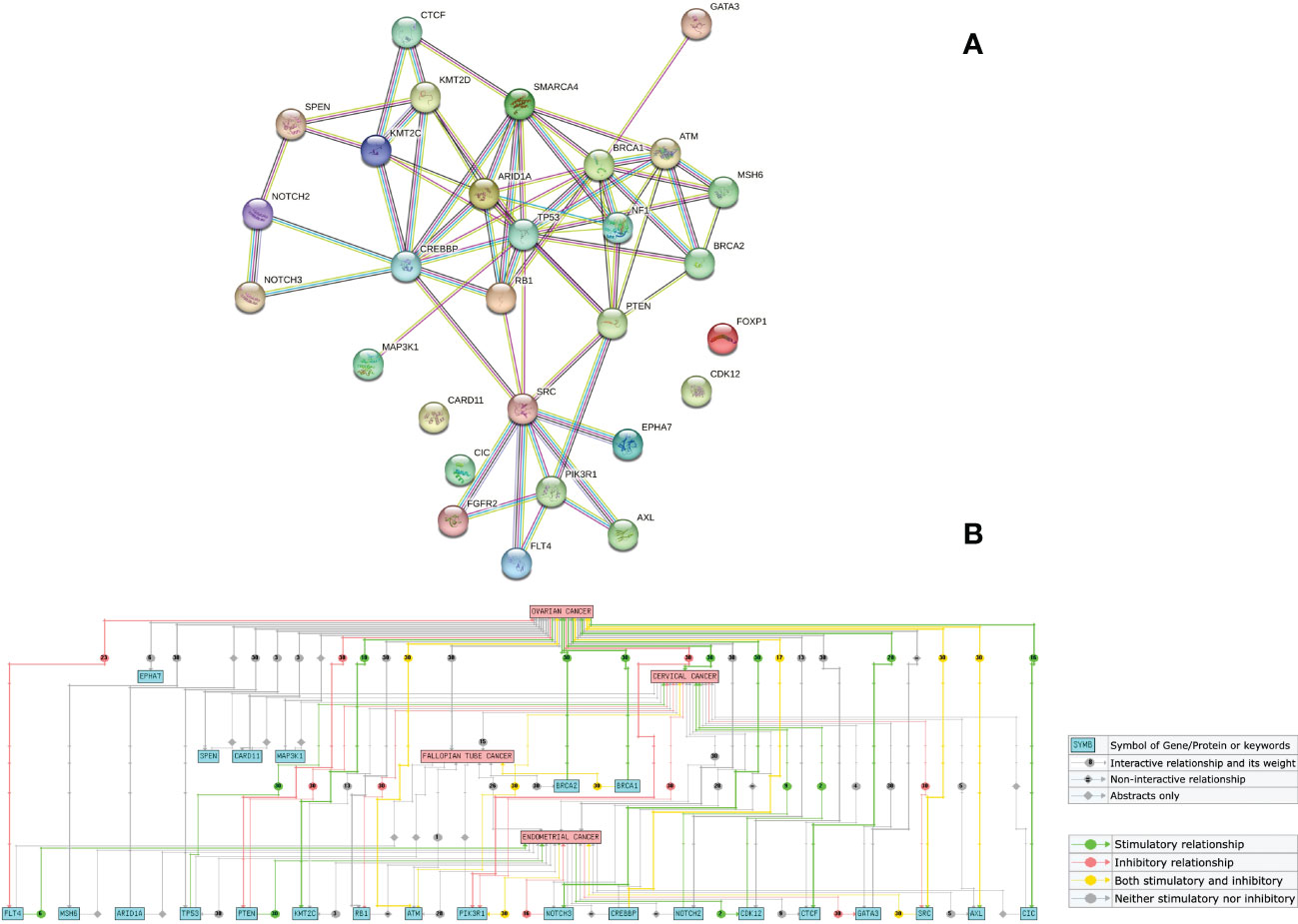
Figure 6 Interaction between mutated genes. Overlap analysis of SNVs- and insertions and InDels-associated 29 genes were performed on STRING (A) and Chilibot (B). A confidence of 0.7 was used to analyze functional interactions on the STRING website. “Ovarian cancer”, “cervical cancer”, “fallopian tube cancer”, and “endometrial cancer” were used as keywords on Chilibot to analyze the interaction between genes and different gynecological cancers, excluding abstract co-occurrence relationships.
4 Discussion
Gynecological cancers are among the most common malignancies with significant morbidity and mortality, primarily classified into five major types according to the organ affected (38, 39). In this retrospective study, we investigated 184 Chinese patients with gynecological cancer using NGS. Patients with OC, CC, EC, and FTC shared similarities but also varied both in clinical characterization and genomic landscape. It is worth noting that our research also has some limitations. Firstly, consistent with their clinical incidence, the numbers of patients with CC, EC, and FTC were limited in this study. Therefore, the relevant statistical results among patients with OC were more informative. Secondly, no overall survival data are provided at this time since the most recent patient enrolled was in June 2022. Therefore, this study may provide preliminary information for the clinical features and mutations of different types of gynecological tumors, and offer new ideas for future clinical treatment and targeted drug development.
It was reported that women with hereditary breast cancer have a 30–50% chance of developing OC (40). All the patients with a family and personal history of cancer in this study were diagnosed with OC, especially those with breast cancer. Our KEGG enrichment results also showed a significant cross between gynecological cancers and breast cancer (Figure 5A). Therefore, for persons with family history, especially with breast cancer history, it is crucial they undergo gynecological tumor gene screening as early as possible.
NGS technology gives us an opportunity to rapidly sequence multiple genes simultaneously and discover relevant mutations to guide treatment, which is beneficial in the field of precision or personalized medicine (41). TP53, the most frequently mutated gene in OC and FTC in our study (Figure 2), was reported in 1979 as the earliest gene to be associated with gynecological cancer (42). Consistently, an analysis from The Cancer Genome Atlas demonstrated that 96% HGSC was characterized by TP53 mutation (43). KMT2C and FGFR3 mutations had higher frequencies among the patients with CC in our study (Figure 2), of which FGFR3-TACC3 fusion was recently reported to be a potential molecular mechanism for inducing small cell cervical carcinoma (44). PTEN overexpression was suggested to promote morular differentiation in EC (45), but our results showed that PTEN deletion also played an important role (Figure 2). SNV was the most common mutation in our study, followed by insertions and InDels. A combination of these mutation-associated genes and the top 10 most frequent mutations will constitute the potential multi-gene panel to screen gynecological cancers.
BRCA1 and BRCA2 mutations are related to the DNA double-strand break repair process, which is also demonstrated in the GO enrichment result (Figure 5B). The process will not proceed normally when these two genes mutate, and the upstream codon will be converted to a stop codon and thus affect the protein formation (40). BRCA gene mutations are also indicators for PARP inhibitor (43, 46) and chemotherapy treatment (47). To the best of our knowledge, the mutation hotspot in BRCA2 (I770) discovered in our study is the first reported among Chinese patients with gynecological cancer (48–50). Patients with BRCA2 mutations have a better prognosis than those with BRCA1 mutations (51).
Comprehensive understanding of factors associated with genomic instability is crucial for improving our knowledge of carcinogenesis. TMB is defined as the total number of somatic coding mutations, base substitutions, and insertion–deletion errors per million bases (52). Recently, researchers have identified the crucial role of TMB in response to immunotherapy and patient prognosis (53, 54). Higher TMB is associated with higher-grade, advanced clinical stage, and immunosuppressive phenotypes (55). According to our results, patients with EC (Table 2) and mucinous carcinoma (Supplementary Table 3) tended to have a higher ratio of TMB-high values. Zhu et al. also documented that the TMB of mucinous tumors in their study was higher than that of HGSC and LGSC (56). However, limited by the sample sizes in our study, more patient data in a larger cohort will be collected to verify this conclusion. A TMB value ≥75% level is usually defined as TMB-high (57), and there were 23 (12.57%) patients with TMB-high in this study. Pre-menopause was found to contribute significantly to higher TMB values among these 23 patients (p < 0.05, data not shown). Genomic alterations are also documented to be associated with TMB. In this study, besides the most frequently altered genes TP53, PKI3CA, and PTEN, patients with FGFR3 mutations also tended to have higher TMB values than those with wild-type genes (Figure 4). Erdafitinib has been approved for patients with urothelial carcinomas with select FGFR3 mutations (58). Therefore, FGFR3 may also become a potential target for patients with gynecological cancers.
5 Conclusions
In summary, our study elucidated the distinct genomic landscapes of various types of gynecological cancers. Taken together with the results of TMB and enriched pathways, this study preliminarily sheds light on the molecular mechanisms of gynecological cancers, and the information gained may contribute to the development of targeted drugs and clinical treatment in precision medicine. Further large-scale and multi-center studies will be performed to validate our findings.
Data availability statement
The original contributions presented in the study are included in the article/Supplementary Material. Further inquiries can be directed to the corresponding authors.
Ethics statement
The studies involving human participants were reviewed and approved by Ethics Committee of the Ruijin Hospital, Shanghai Jiao Tong University School of Medicine. The patients/participants provided their written informed consent to participate in this study.
Author contributions
CJ and LL contributed to study conception and design. HL and WF performed surgeries and enrollment of patients. CJ and YL conducted patient recruitment, data collection and sequencing. ZP and GC performed bioinformatics analysis. CJ and YL drafted the manuscript. WF and LL revised the manuscript. All authors contributed to the article and approved the submitted version.
Funding
The study is supported by Shanghai “Rising Stars of Medical Talents” youth clinical laboratory practitioner program (SHWRS(2020)_87).
Acknowledgments
We would like to thank the patients who gave their consent to present data in this study, as well as the investigators and research staff.
Conflict of interest
ZP is an employee of Genecast Biotechnology Co., Ltd., Wuxi, China.
The remaining authors declare that the research was conducted in the absence of any commercial or financial relationships that could be construed as a potential conflict of interest.
Publisher’s note
All claims expressed in this article are solely those of the authors and do not necessarily represent those of their affiliated organizations, or those of the publisher, the editors and the reviewers. Any product that may be evaluated in this article, or claim that may be made by its manufacturer, is not guaranteed or endorsed by the publisher.
Supplementary material
The Supplementary Material for this article can be found online at: https://www.frontiersin.org/articles/10.3389/fonc.2023.1143876/full#supplementary-material
References
1. Wang N, Yang Y, Jin D, Zhang Z, Shen K, Yang J, et al. PARP inhibitor resistance in breast and gynecological cancer: Resistance mechanisms and combination therapy strategies. Front Pharmacol (2022) 13:967633. doi: 10.3389/fphar.2022.967633
2. Suszynska M, Klonowska K, Jasinska AJ, Kozlowski P. Large-Scale meta-analysis of mutations identified in panels of breast/ovarian cancer-related genes - providing evidence of cancer predisposition genes. Gynecol Oncol (2019) 153(2):452–62. doi: 10.1016/j.ygyno.2019.01.027
3. Caro AA, Deschoemaeker S, Allonsius L, Coosemans A, Laoui D. Dendritic cell vaccines: A promising approach in the fight against ovarian cancer. Cancers (Basel) (2022) 14(16):4037. doi: 10.3390/cancers14164037
4. Chelariu-Raicu A, Coleman RL. Breast cancer (BRCA) gene testing in ovarian cancer. Chin Clin Oncol (2020) 9(5):63. doi: 10.21037/cco-20-4
5. American Cancer Society- Cancer Facts and Figures. (2019). Available at: https://www.cancer.org/research/cancer-facts-statistics/all-cancer-facts-figures/cancer-facts-figures-2019.html.
6. Lalwani N, Prasad SR, Vikram R, Shanbhogue AK, Huettner PC, Fasih N. Histologic, molecular, and cytogenetic features of ovarian cancers: Implications for diagnosis and treatment. Radiographics (2011) 31(3):625–46. doi: 10.1148/rg.313105066
7. Siegel RL, Miller KD, Fuchs HE, Jemal A. Cancer statistics, 2022. CA Cancer J Clin (2022) 72(1):7–33. doi: 10.3322/caac.21708
8. Wen H, Guo QH, Zhou XL, Wu XH, Li J. Genomic profiling of Chinese cervical cancer patients reveals prevalence of DNA damage repair gene alterations and related hypoxia feature. Front Oncol (2022) 11:792003. doi: 10.3389/fonc.2021.792003
9. Maluf FC, Dal Molin GZ, de Melo AC, Paulino E, Racy D, Ferrigno R, et al. Recommendations for the prevention, screening, diagnosis, staging, and management of cervical cancer in areas with limited resources: Report from the international gynecological cancer society consensus meeting. Front Oncol (2022) 12:928560. doi: 10.3389/fonc.2022.928560
10. Siegel RL, Miller KD, Fuchs HE, Jemal A. Cancer statistics, 2021. CA Cancer J Clin (2021) 71(1):7–33. doi: 10.3322/caac.21654
11. Fidler MM, Gupta S, Soerjomataram I, Ferlay J, Steliarova-Foucher E, Bray F. Cancer incidence and mortality among young adults aged 20-39 years worldwide in 2012: a population-based study. Lancet Oncol (2017) 18(12):1579–89. doi: 10.1016/S1470-2045(17)30677-0
12. Cohen PA, Jhingran A, Oaknin A, Denny L. Cervical cancer. Lancet (2019) 393(10167):169–82. doi: 10.1016/S0140-6736(18)32470-X
13. Davies-Oliveira JC, Round T, Crosbie EJ. Cervical screening: The evolving landscape. Br J Gen Pract (2022) 72(721):364–65. doi: 10.3399/bjgp22X720197
14. Li Y, Feng J, Zhao C, Meng L, Shi S, Liu K, et al. A new strategy in molecular typing: the accuracy of an NGS panel for the molecular classification of endometrial cancers. Ann Transl Med (2022) 10(16):870. doi: 10.21037/atm-22-3446
15. Sung H, Ferlay J, Siegel RL, Laversanne M, Soerjomataram I, Jemal A, et al. Global cancer statistics 2020: GLOBOCAN estimates of incidence and mortality worldwide for 36 cancers in 185 countries. CA Cancer J Clin (2021) 71:209–49. doi: 10.3322/caac.21660
16. Kasius JC, Pijnenborg JMA, Lindemann K, Forsse D, van Zwol J, Kristensen GB, et al. Risk stratification of endometrial cancer patients: FIGO stage, biomarkers and molecular classification. Cancers (Basel) (2021) 13:5848. doi: 10.3390/cancers13225848
17. Urick ME, Bell DW. Clinical actionability of molecular targets in endometrial cancer. Nat Rev Cancer (2019) 19:510–21. doi: 10.1038/s41568-019-0177-x
18. Clarfield L, Diamond L, Jacobson M. Risk-reducing options for high-grade serous gynecologic malignancy in BRCA1/2. Curr Oncol (2022) 29(3):2132–40. doi: 10.3390/curroncol29030172
19. Hong JH, Cho HW, Ouh YT, Lee JK, Chun Y, Gim JA. Genomic landscape of advanced endometrial cancer analyzed by targeted next-generation sequencing and the cancer genome atlas (TCGA) dataset. J Gynecol Oncol (2022) 33(3):e29. doi: 10.3802/jgo.2022.33.e29
20. Feng W, Jia N, Jiao H, Chen J, Chen Y, Zhang Y, et al. Circulating tumor DNA as a prognostic marker in high-risk endometrial cancer. J Transl Med (2021) 19(1):51. doi: 10.1186/s12967-021-02722-8
21. Stasenko M, Fillipova O, Tew WP. Fallopian tube carcinoma. J Oncol Pract (2019) 15(7):375–82. doi: 10.1200/JOP.18.00662
22. Maeda M, Hisa T, Matsuzaki S, Ohe S, Nagata S, Lee M, et al. Primary fallopian tube carcinoma presenting with a massive inguinal tumor: A case report and literature review. Medicina (Kaunas) (2022) 58(5):581. doi: 10.3390/medicina58050581
23. Lõhmussaar K, Kopper O, Korving J, Begthel H, Vreuls CPH, van Es JH, et al. Assessing the origin of high-grade serous ovarian cancer using CRISPR-modification of mouse organoids. Nat Commun (2020) 11(1):2660. doi: 10.1038/s41467-020-16432-0
24. Liao CI, Chow S, Chen LM, Kapp DS, Mann A, Chan JK. Trends in the incidence of serous fallopian tube, ovarian, and peritoneal cancer in the US. Gynecol Oncol (2018) 149(2):318–23. doi: 10.1016/j.ygyno.2018.01.030
25. Choi MC, Bae JS, Jung SG, Park H, Joo WD, Song SH, et al. Prevalence of germline BRCA mutations among women with carcinoma of the peritoneum or fallopian tube. J Gynecol Oncol (2018) 29(4):e43. doi: 10.3802/jgo.2018.29.e43
26. Shah S, Cheung A, Kutka M, Sheriff M, Boussios S. Epithelial ovarian cancer: Providing evidence of predisposition genes. Int J Environ Res Public Health (2022) 19(13):8113. doi: 10.3390/ijerph19138113
27. Vicus D, Finch A, Cass I, Rosen B, Murphy J, Fan I, et al. Prevalence of BRCA1 and BRCA2 germ line mutations among women with carcinoma of the fallopian tube. Gynecol Oncol (2010) 118(3):299–302. doi: 10.1016/j.ygyno.2010.05.011
28. Poveda A, Floquet A, Ledermann JA, Asher R, Penson RT, Oza AM, et al. Olaparib tablets as maintenance therapy in patients with platinum-sensitive relapsed ovarian cancer and a BRCA1/2 mutation (SOLO2/ENGOT-Ov21): a final analysis of a double-blind, randomised, placebo-controlled, phase 3 trial. Lancet Oncol (2021) 22(5):620–31. doi: 10.1016/S1470-2045(21)00073-5
29. Liu J, Li Z, Lu T, Pan J, Li L, Song Y, et al. Genomic landscape, immune characteristics and prognostic mutation signature of cervical cancer in China. BMC Med Genomics (2022) 15(1):231. doi: 10.1186/s12920-022-01376-9
30. Imyanitov E, Sokolenko A. Integrative genomic tests in clinical oncology. Int J Mol Sci (2022) 23(21):13129. doi: 10.3390/ijms232113129
31. Jiang T, Jiang L, Dong X, Gu K, Pan Y, Shi Q, et al. Utilization of circulating cell-free DNA profiling to guide first-line chemotherapy in advanced lung squamous cell carcinoma. Theranostics (2021) 11(1):257–67. doi: 10.7150/thno.51243
32. Li H. Aligning sequence reads, clone sequences and assembly contigs with BWA-MEM. arXiv preprint (2013). doi: 10.48550/arXiv.1303.3997
33. Lai Z, Markovets A, Ahdesmaki M, Chapman B, Hofmann O, McEwen R, et al. VarDict: a novel and versatile variant caller for next-generation sequencing in cancer research. Nucleic Acids Res (2016) 44(11):e108. doi: 10.1093/nar/gkw227
34. Garrison E, Marth G. Haplotype-based variant detection from short-read sequencing. arXiv preprint (2012). doi: 10.48550/arXiv.1207.3907
35. Wang K, Li M, Hakonarson H. ANNOVAR: Functional annotation of genetic variants from high-throughput sequencing data. Nucleic Acids Res (2010) 38(16):e164. doi: 10.1093/nar/gkq603
36. Karczewski KJ, Weisburd B, Thomas B, Solomonson M, Ruderfer DM, Kavanagh D, et al. The ExAC browser: Displaying reference data information from over 60 000 exomes. Nucleic Acids Res (2017) 45(D1):D840–5. doi: 10.1093/nar/gkw971
37. Karczewski KJ Francioli L. The genome aggregation database (gnomAD). MacArthur Lab (2017). Available at: https://macarthurlab.org/2017/02/27/the-genome-aggregation-database-gnomad/
38. Chalmers ZR, Connelly CF, Fabrizio D, Gay L, Ali SM, Ennis R, et al. Analysis of 100,000 human cancer genomes reveals the landscape of tumor mutational burden. Genome Med (2017) 9(1):34. doi: 10.1186/s13073-017-0424-2
39. Daoud T, Sardana S, Stanietzky N, Klekers AR, Bhosale P, Morani AC. Recent imaging updates and advances in gynecologic malignancies. Cancers (Basel) (2022) 14(22):5528. doi: 10.3390/cancers14225528
40. Therachiyil L, Anand A, Azmi A, Bhat A, Korashy HM, Uddin S. Role of RAS signaling in ovarian cancer. F1000Res (2022) 11:1253. doi: 10.12688/f1000research.126337.1
41. Xie C, Luo J, He Y, Jiang L, Zhong L, Shi Y. BRCA2 gene mutation in cancer. Med (Baltimore) (2022) 101(45):e31705. doi: 10.1097/MD.0000000000031705
42. Johansen EL, Thusgaard CF, Thomassen M, Boonen SE, Jochumsen KM. Germline pathogenic variants associated with ovarian cancer: A historical overview. Gynecol Oncol Rep (2022) 44:101105. doi: 10.1016/j.gore.2022.101105
43. Kanchi KL, Johnson KJ, Lu C, McLellan MD, Leiserson MD, Wendl MC, et al. Integrated analysis of germline and somatic variants in ovarian cancer. Nat Commun (2014) 5:3156. doi: 10.1038/ncomms4156
44. Cancer Genome Atlas Research Network. Integrated genomic analyses of ovarian carcinoma. Nature (2011) 474(7353):609–15. doi: 10.1038/nature10166
45. Wang X, Jia W, Wang M, Liu J, Zhou X, Liang Z, et al. Human papillomavirus integration perspective in small cell cervical carcinoma. Nat Commun (2022) 13(1):5968. doi: 10.1038/s41467-022-33359-w
46. Yokoi A, Minami M, Hashimura M, Oguri Y, Matsumoto T, Hasegawa Y, et al. PTEN overexpression and nuclear β-catenin stabilization promote morular differentiation through induction of epithelial-mesenchymal transition and cancer stem cell-like properties in endometrial carcinoma. Cell Commun Signal (2022) 20(1):181. doi: 10.1186/s12964-022-00999-w
47. Li N, Liu Q, Tian Y, Wu L. Overview of fuzuloparib in the treatment of ovarian cancer: Background and future perspective. J Gynecol Oncol (2022) 33(6):e86. doi: 10.3802/jgo.2022.33.e86
48. Vencken PMLH, Kriege M, Hoogwerf D, Beugelink S, van der Burg MEL, Hooning MJ, et al. Chemosensitivity and outcome of BRCA1- and BRCA2-associated ovarian cancer patients after first-line chemotherapy compared with sporadic ovarian cancer patients. Ann Oncol (2011) 22(6):1346–52. doi: 10.1093/annonc/mdq628
49. Kwong A, Shin VY, Ma ES, Chan CT, Ford JM, Kurian AW, et al. Screening for founder and recurrent BRCA mutations in Hong Kong and US Chinese populations. Hong Kong Med J (2018) 24 Suppl 3(3):4–6.
50. de Juan Jiménez I, García Casado Z, Palanca Suela S, Esteban Cardeñosa E, López Guerrero JA, Segura Huerta Á, et al. Novel and recurrent BRCA1/BRCA2 mutations in early onset and familial breast and ovarian cancer detected in the program of genetic counseling in cancer of valencian community (eastern Spain). Relationship Family phenotypes Mutat prevalence Fam Cancer (2013) 12(4):767–77. doi: 10.1007/s10689-013-9622-2
51. Zhang Y, Wu H, Yu Z, Li L, Zhang J, Liang X, et al. Germline variants profiling of BRCA1 and BRCA2 in Chinese hakka breast and ovarian cancer patients. BMC Cancer (2022) 22(1):842. doi: 10.1186/s12885-022-09943-0
52. Bolton KL, Chenevix-Trench G, Goh C, Sadetzki S, Ramus SJ, Karlan BY, et al. Association between BRCA1 and BRCA2 mutations and survival in women with invasive epithelial ovarian cancer. JAMA (2012) 307(4):382–90. doi: 10.1001/jama.2012.20
53. Hu-Lieskovan S, Bhaumik S, Dhodapkar K, Grivel JJB, Gupta S, Hanks BA, et al. SITC cancer immunotherapy resource document: A compass in the land of biomarker discovery. J Immunother Cancer (2020) 8(2):e000705. doi: 10.1136/jitc-2020-000705
54. Yarchoan M, Hopkins A, Jaffee EM. Tumor mutational burden and response rate to PD-1 inhibition. N Engl J Med (2017) 377(25):2500–1. doi: 10.1056/NEJMc1713444
55. Samstein RM, Lee CH, Shoushtari AN, Hellmann MD, Shen R, Janjigian YY, et al. Tumor mutational load predicts survival after immunotherapy across multiple cancer types. Nat Genet (2019) 51(2):202–6. doi: 10.1038/s41588-018-0312-8
56. Wang H, Liu J, Yang J, Wang Z, Zhang Z, Peng J, et al. A novel tumor mutational burden-based risk model predicts prognosis and correlates with immune infiltration in ovarian cancer. Front Immunol (2022) 13:943389. doi: 10.3389/fimmu.2022.943389
57. Zhu S, Zhang C, Cao D, Bai J, Yu S, Chen J, et al. Genomic and TCR profiling data reveal the distinct molecular traits in epithelial ovarian cancer histotypes. Oncogene (2022) 41(22):3093–103. doi: 10.1038/s41388-022-02277-y
58. Jiang T, Chen J, Xu X, Cheng Y, Chen G, Pan Y, et al. On-treatment blood TMB as predictors for camrelizumab plus chemotherapy in advanced lung squamous cell carcinoma: Biomarker analysis of a phase III trial. Mol Cancer (2022) 21(1):4. doi: 10.1186/s12943-021-01479-4
Keywords: gynecological cancer, next-generation sequencing, TMB, BRCA1, BRCA2, FGFR3
Citation: Jiang C, Lu Y, Liu H, Cai G, Peng Z, Feng W and Lin L (2023) Clinical characterization and genomic landscape of gynecological cancers among patients attending a Chinese hospital. Front. Oncol. 13:1143876. doi: 10.3389/fonc.2023.1143876
Received: 13 January 2023; Accepted: 20 March 2023;
Published: 30 March 2023.
Edited by:
Umberto Malapelle, University of Naples Federico II, ItalyReviewed by:
Gaia Giannone, Imperial College London, United KingdomIsabel Soto-Cruz, National Autonomous University of Mexico, Mexico
Copyright © 2023 Jiang, Lu, Liu, Cai, Peng, Feng and Lin. This is an open-access article distributed under the terms of the Creative Commons Attribution License (CC BY). The use, distribution or reproduction in other forums is permitted, provided the original author(s) and the copyright owner(s) are credited and that the original publication in this journal is cited, in accordance with accepted academic practice. No use, distribution or reproduction is permitted which does not comply with these terms.
*Correspondence: Weiwei Feng, fww12066@rjh.com.cn; Lin Lin, linlin0415@hotmail.com
†These authors have contributed equally to this work and share first authorship