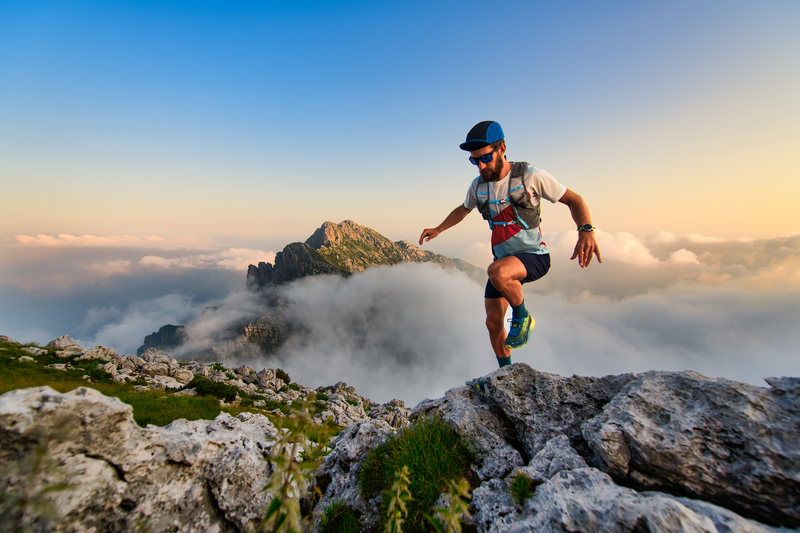
95% of researchers rate our articles as excellent or good
Learn more about the work of our research integrity team to safeguard the quality of each article we publish.
Find out more
ORIGINAL RESEARCH article
Front. Oncol. , 05 July 2023
Sec. Gastrointestinal Cancers: Hepato Pancreatic Biliary Cancers
Volume 13 - 2023 | https://doi.org/10.3389/fonc.2023.1116129
Purpose: This study aimed to explore the clinical value of non-invasive preoperative Edmondson-Steiner grade of hepatocellular carcinoma (HCC) using contrast-enhanced ultrasound (CEUS).
Methods: 212 cases of HCCs were retrospectively included, including 83 cases of high-grade HCCs and 129 cases of low-grade HCCs. Three representative CEUS images were selected from the arterial phase, portal vein phase, and delayed phase and stored in a 3-dimensional array. ITK-SNAP was used to segment the tumor lesions manually. The Radiomics method was conducted to extract high-dimensional features on these contrast-enhanced ultrasound images. Then the independent sample T-test and the Least Absolute Shrinkage and Selection Operator (LASSO) were employed to reduce the feature dimensions. The optimized features were modeled by a classifier based on ensemble learning, and the Edmondson Steiner grading was predicted in an independent testing set using this model.
Results: A total of 1338 features were extracted from the 3D images. After the dimension reduction, 10 features were finally selected to establish the model. In the independent testing set, the integrated model performed best, with an AUC of 0.931.
Conclusion: This study proposed an Edmondson-Steiner grading method for HCC with CEUS. The method has good classification performance on independent testing sets, which can provide quantitative analysis support for clinical decision-making.
Hepatocellular carcinoma (HCC) is the sixth most common cancer in the world and the second most common cause of cancer-related death (1), and the incidence rate is increasing year by year (2). For early HCC patients, surgical resection is still an effective treatment (3). However, HCC is prone to relapse and metastasis, and the prognosis is poor (4, 5). Some studies have shown that recurrence is closely related to pathological manifestation (6). Several studies have shown that Edmondson-Steiner grade is an essential preoperative predictor of HCC (7, 8). For low-grade HCC with isolated lesions ≤ 2cm, the 3-year and 5-year relapse-free survival rates were 64% and 50%, respectively, while the 3-year and 5-year relapse-free survival rates for high-grade HCC were 39% and 29%, respectively (9, 10). According to the research, the recurrence rate of high-grade HCC is higher than that of low-grade HCC (11, 12). Moreover, compared with high-grade HCC, low-grade HCC has a higher surgical cure rate (13, 14) and higher short-term and long-term survival rates. Therefore, accurate prediction of the Edmondson-Steiner grade of HCC is highly significant for clinical decision-making, treatment plan optimization, and prognosis prediction (15–18).
Postoperative histopathological examination of resected tumor specimens is the gold standard for diagnosis and pathological grading of HCC (19). A needle biopsy can provide preliminary pathological grading before the operation. However, the risk of complications and misdiagnosis must always be considered (20). At present, some studies have shown that imaging examination may have the potential to reflect pathological grading (21, 22). MRI combined with liver-specific contrast agents, and diffusion-weighted imaging (DWI) has been proven to help evaluate the histological grading of HCC (23–25). Wu et al. showed that the radiomics characteristics of non-enhanced MRI and clinical factors helped predict the HCC grade before operation (26). The area under the ROC curve (AUC) of the best model for HCC grading prediction was 0.80. Jiseon et al. believed that CT texture analysis can provide texture features significantly related to higher tumor grade (27). Seitz et al. believe that CEUS and MRI have equal value for the differentiation and regulation of newly discovered liver tumors in clinical practice. Contrast-enhanced ultrasound (CEUS) and MRI are reliable for differentiating benign and malignant lesions and diagnosing hepatic hemangiomas and FNH. Characterization of metastasis and hepatocellular carcinoma is also very reliable (28). Compared with CT and MRI, CEUS provides dynamic perfusion images with fewer application restrictions (29). Liu et al. report that CEUS can give helpful information for the differential diagnosis of HCC, indicating its use in clinical practice (30). Wang et al. showed that the combination of the radiomics model of gray scale ultrasound and contrast-enhanced ultrasound with clinical information has potential clinical value for tumor grading (31). However, the quality of ultrasonic imaging depends significantly on the operator. Most of the previous studies are based on subjective feature recognition, which largely depends on the experience of doctors. These features may reveal pathological features that the naked eye can recognize (32).
Radiomics has been increasingly applied to the field of medical image analysis. It extracts massive amounts of information from radiological images for more in-depth mining, prediction, and analysis to help doctors diagnose more accurately. This study aimed to explore the value of sonographic features in predicting the Edmondson-Steiner grade of HCC before surgery.
The Shengjing Hospital of China Medical University Ethics Committee has approved this study. This study retrospectively collected the clinical information and ultrasonic images of 404 patients with primary HCC from April 2014 to March 2023. Among those, 212 cases were included in the first dataset, including 83 cases with high grades and 129 with low grades. An additional 51 cases, including 29 of low-grade and 22 of high-grade HCC, were used as an external independent testing set for evaluation. The inclusion criteria were as follows: (1) pathology confirmed HCC. (2) CEUS was performed one month before the operation, and the ultrasonic image data were complete. (3) No history of anti-tumor therapy such as liver transplantation (LT), microwave ablation (MWA), radiofrequency ablation (RFA), transcatheter arterial chemoembolization (TACE), etc. (4) The ultrasound image meets the analysis requirements (clear without artifacts and the target lesion is evident on the image). (5) No history of other malignant tumors. The exclusion criteria were: (1) liver cancer surgery and radiofrequency ablation before the operation; (2) Ultrasound images have severe artifacts, and tumors can hardly be observed with the naked eye.
We collected the preoperative laboratory examination information, including primary personal data (gender, age, hepatitis history), laboratory test results (alpha-fetoprotein (AFP), alanine aminotransferase (ALT), aspartate transaminase (AST), total bilirubin (TB), and prothrombin time (PT)).
The differentiation grade of HCC reported by clinical pathology is based on the Edmondson-Steiner grade. In this study, low grades correspond to Edmondson grades I, I-II, and II, and high grades correspond to Edmondson grades III, III-IV, and IV. To summarize, 83 high-grade HCCs and 129 cases of low-level HCCs were included. Specific exclusion criteria is shown in Figure 1.
Figure 1 Inclusion and exclusion of patients. H stands for high-grade HCC, and L stand for low-grade HCC.
The patients were divided into training and testing cohorts randomly. There were 118 (79.7%) male patients and 30 (20.3%) female patients in the training cohort, while 51 (79.6%) male patients and 13 (20.4%) female patients were in the testing cohort. The median ages of patients in the training and testing cohorts were 58 years (range 29-79 years) and 57 years (range 28-74 years), respectively. In the training data set, the number of patients with low-grade HCC was 90 (60.8%), and 58 (39.2%) patients with high-grade HCC. In the testing data set, there were 39 (60.9%) cases with low-grade HCC and 25 (39.1%) cases with high-grade HCC. There was no significant difference between the training data set and the test data set in clinical pathological characteristics (p>0.05).
All CEUS examinations were performed by doctors with more than eight years of CEUS experience in liver cancer. All patients fasted for more than 8 hours before the examination. First, the whole liver was scanned with gray-scale ultrasound. The maximum diameter, echo signal, blood flow signal, shape, boundary, and lesion edge were evaluated and recorded. Store at least one original ultrasound image showing lesions and the same image containing measurement parameters in DICOM format. Then, the patient was given 2.4ml (3ml at most) of SonoVue (Bracco, Milan, Italy) via the anterior elbow vein, and then 5ml of 0.9% standard saline solution was added. The phase of contrast-enhanced ultrasound is divided into arterial phase, portal static phase, and delayed phase, which are 0-30s, 31-120s, and>120s after injection, respectively.
The static and real-time ultrasound images obtained in this study are retrieved from the picture archiving and communication system (PACS) and stored in DCM format. Three representative CEUS images were selected from the arterial phase (AP), portal vein phase (PVP), and delayed phase (DP) in which the image is clear shown and artifact-free. These images were stored in a 3-dimensional array. The main steps of this method can be described in Figure 2.
Figure 2 The research process includes image acquisition and segmentation, feature extraction and selection, model construction, and evaluation.
We loaded the 3D image array into ITK-SNAP (v3.8.0, http://www.itk-snap.org) (33) for manual segmentation. To ensure the segmentation accuracy, a doctor with more than eight years of abdominal ultrasound experience and another doctor with ten years of abdominal ultrasound experience jointly complete the final confirmation.
This study used the open-source software package Pyradiomic (https://www.radiomics.io/index.html) (34) for feature extraction and selection. We chose the original image, Local Binary Pattern (LBP) 3D, and the Wavelet for the filter classes. The feature classes extracted here include First Order Statistics, Shape-based (3D), Gray Level Co-occurrence (GLCM), Gray Level Run Length Matrix (GLRLM), Gray Level Size Zone Matrix (GLSZM), Gray Level Dependence Matrix (GLDM), Neighboring Gray Tone Difference Matrix (NGTDM). The independent sample t-test was used first for feature selection. Then, the Least Absolute Shrinkage and Selection Operator (LASSO) was used.
212 patients were randomly divided into the training cohort (n=148) and the testing cohort (n=64). Based on the selected features, five single prediction models, namely linear regression (LR), random forest (RF), K-nearest neighbor (KNN), naive Bayes (NB), and Xgboost (XGB), were constructed by using a five-fold cross-validation method in the training set. Then, a support vector machine (SVM) was used as the meta-classifier to build the integrated prediction model.
This study used an independent testing set to evaluate the model’s performance. Sensitivity, specificity, accuracy, and AUC were used to evaluate the model’s performance.
Experienced doctors conducted the ROI segmentation. Figure 3 shows a segmentation example of a patient in different image phases.
Figure 3 An example of ROI segmentation from a 63-year-old male patient with low-grade HCC, (A) arterial phase (AP), (B) portal vein phase (PVP), and (C) delayed phase (DP).
A total of 1338 features were extracted from the 3D CEUS images, and the number of selected features was 987 by the independent sample t-test. Then, after the selection by LASSO, ten features from seven categories were chosen finally: First Order Statistics, Shape-based (3D), GLCM, GLRLM, GLSZM, GLDM, and NGTDM. See Figure 4.
Figure 4 The selected feature weight. From left to right: original_glrlm_ShortRunLowGrayLevelEmphasis, LBP-3D-m1_firstorder_Kurtosis, LBP-3D-m2_glszm_GrayLevelNonUniformityNormalized, LBP-3D-k_glcm_Imc2, LBP-3D-k_glcm_Idn, log-sigma-1-0-mm-3D_glcm_Imc2, log-sigma-2-0-mm-3D_glcm_Idn, log-sigma-3-0-mm-3D_glszm_SmallAreaEmphasis, wavelet-LHH_glcm_Correlation, wavelet-LLL_glcm_Idn.
Based on the selected features, five single prediction models, LR, RF, KNN, NB, and XGB, were constructed by using a five-fold cross-validation method in the training set. Then, SVM was used as the meta-classifier to build the integrated prediction model. Table 1 shows the performance index in the training set, and Table 2 shows the performance in the testing set of all the models. The corresponding ROCs can be found in Figure 5.
Figure 5 The ROCs. (A) The average performance of different models after five-fold cross-validation in the training set. (B) Models performance in the testing set.
Although significant progress has been made in treating HCC in recent years, the postoperative recurrence rate is still high, leading to poor clinical prognosis (35). Pathological grading is one of the critical factors in determining the postoperative recurrence of HCC. Compared with low-grade, high-grade HCC has a higher recurrence rate and shorter survival period, requiring expanded surgical margins and more frequent postoperative follow-up (36). Accurate preoperative prediction of pathological grading can provide a theoretical basis for individualized treatment decisions. However, the evaluation of HCC grading in clinical practice can only rely on pathological analysis of tumor tissue obtained after liver resection or puncture, which limits its value in guiding treatment decision-making before surgery.
Imaging examination is essential for diagnosing and preoperatively evaluating HCC. The commonly used contrast-enhanced imaging techniques in clinical practice include contrast-enhanced CT (CECT), contrast-enhanced MRI (CEMRI), hepatobiliary-specific MRI, and contrast-enhanced ultrasound (CEUS). Among the above four image types, CEUS differs from CECT, CEMRI, and hepatobiliary-specific MRI. The contrast agent of CEUS is a pure-blood pool contrast agent, which can accurately display the vascular architecture within the tumor. In addition, CEUS belongs to three-dimensional temporal imaging, which can reflect real-time changes in blood flow perfusion of tumor tissue (37). Previous studies have shown that factors such as tumor diameter, boundary, echo, clearance time, clearance degree, and LI-RADS classification of CEUS are related to the pathological grading of HCC, but their predictive ability is still weak (38–40).
Radiomics has been applied to the diagnosis of HCC and postoperative efficacy evaluation, and other aspects by analyzing existing images of patients without additional examinations or increasing the economic burden on patients (41, 42). This study used CEUS to predict the Edmondson-Steiner grading of HCC before operation. First, we extracted the Radiomics features from the enhanced three phases CEUS images and reduced the dimension of the features using independent sample t-test and LASSO. Next, five individual classifiers were trained using the five-fold cross-validation, and then the classifiers were integrated and obtained a prediction model with good performance.
The experimental results show that the prediction performance of the stacking ensemble model is much better than that of the individual classifier prediction model. For example, from Table 2, we can find that the accuracy and AUC of the ensemble model can reach 0.891 and 0.931, respectively.
The results of the additional testing set show that the performance of KNN compared to all other models, as shown in Figure 6 and Table 3, has an accuracy and AUC of 0.901 and 0.940. The ensemble model also showed a good performance with an AUC of 0.937.
Radiomics is a new field that aims to explore the potential relationship between medical images and tumor cell phenotypic characteristics in a non-invasive manner. According to previous studies, radiomics plays an essential role in tumor grading. Table 4 lists recent research on the Edmondson-Steiner grading of HCC.
This study innovatively selected three representative phases of images from CEUS as the objects for feature extraction and analysis. By using ensemble learning methods, the classification performance has been significantly improved compared to existing studies. See Table 4.
Compared with enhanced CT and MRI, CEUS is real-time dynamic imaging, which has the advantages of low cost, safety, portability, and non-radiation, incomparable to other imaging technologies. The CEUS-based diagnosis is promising and can be used more widely in clinical practice.
However, our research has some limitations. First, we only classified HCC patients into two types: high-grade and low-grade. In the future study, the sample size will be further expanded, and the value of Radiomics for multi-category classification of HCC in CEUS will be further explored. Secondly, most of the enrolled patients had a history of viral hepatitis. The robustness of the proposed method needs to be verified in heterogeneous liver diseases with other high-risk factors of HCC. Third, we selected three phases of CEUS images by experienced doctors, which may have errors caused by subjective factors. Fourth, the ultrasound images came from a variety of different devices. The difference in ultrasonic parameters between patients could impact the experimental results. Fifth, our data comes from one center. In the future, we will take multi-center research to improve our experiments’ generalization performance.
This study proposed an automated Edmondson-Steiner grading method for HCC with CEUS. Based on three-dimensional dynamically enhanced ultrasound images, we used Radiomics methods to extract image bio-markers, made effective feature selection, and then used the integrated classifier to build a prediction model. The experimental results show that the method proposed in this study has good classification performance on independent testing sets, which can provide quantitative analysis support for clinical decision-making.
The raw data supporting the conclusions of this article will be made available by the authors, without undue reservation.
The studies involving human participants were reviewed and approved by the Shengjing Hospital of China Medical University Ethics Committee. The patients/participants provided their written informed consent to participate in this study.
YW presented clinical problems, completed data collection, drawing segmentation labels, and establishing and evaluating models. DY and FL completed image data processing, model building and optimization. HS and XP helped applying the Radiomics and machine learning method. YH confirms the accuracy of segmentation labels and guides clinical related issues. HL and ST are responsible for the overall design and guidance. All authors contributed to the article and approved the submitted version.
This work was funded by Liaoning Science and Technology Plan of the People’s Livelihood, Joint Plan Project (2021JH2/10300114).
The authors declare that the research was conducted in the absence of any commercial or financial relationships that could be construed as a potential conflict of interest.
All claims expressed in this article are solely those of the authors and do not necessarily represent those of their affiliated organizations, or those of the publisher, the editors and the reviewers. Any product that may be evaluated in this article, or claim that may be made by its manufacturer, is not guaranteed or endorsed by the publisher.
1. Njei B, Rotman Y, Ditah I, Lim JK. Emerging trends in hepatocellular carcinoma incidence and mortality. Hepatology (2015) 61(1):191–9. doi: 10.1002/hep.27388
2. Li X, Han X, Li L, Su C, Sun J, Zhan C. Dynamic contrast-enhanced ultrasonography with sonazoid for diagnosis of microvascular invasion in hepatocellular carcinoma. Ultrasound Med Biol (2022) 48(3):575–81. doi: 10.1016/j.ultrasmedbio.2021.11.005
3. Bruix J, Reig M, Sherman M. Evidence-based diagnosis, staging, and treatment of patients with hepatocellular carcinoma. Gastroenterology (2016) 150(4):835–53. doi: 10.1053/j.gastro.2015.12.041
4. Jiang HY, Chen J, Xia CC, Cao LK, Duan T, Song B. Noninvasive imaging of hepatocellular carcinoma: from diagnosis to prognosis. World J Gastroenterol (2018) 24(22):2348. doi: 10.3748/wjg.v24.i22.2348
5. Yang G, Yao Y, Wu D, Guo H, Zhou S, Sun D, et al. Upregulated mH2A1 serves as an unfavorable prognostic indicator and promotes the progress of hepatocellular carcinoma (HCC). Life Sci (2020) 263:118576. doi: 10.1016/j.lfs.2020.118576
6. Zhou L, Rui JA, Wang SB, Chen S. G., Qu Q. LCSGJ-T classification, 6th or 5th edition TNM staging did not independently predict the long-term prognosis of HBV-related hepatocellular carcinoma after radical hepatectomy. J Surg Res (2010) 159(1):538–44. doi: 10.1016/j.jss.2008.09.004
7. Zhou L, Rui JA, Ye DX, Wang SB, Chen SG, Qu Q. Edmondson-Steiner grading increases the predictive efficiency of TNM staging for long-term survival of patients with hepatocellular carcinoma after curative resection. World J Surg (2008) 32(8):1748–56. doi: 10.1007/s00268-008-9615-8
8. Zhou L, Rui JA, Wang SB, Chen SG, Qu Q, Chi TY, et al. Factors predictive for long-term survival of male patients with hepatocellular carcinoma after curative resection. J Surg Oncol (2007) 95(4):298–303. doi: 10.1002/jso.20678
9. Gebauer F, Tachezy M, Vashist YK, Marx AH, Yekebas E, Izbicki JR, et al. Resection margin clearance in pancreatic cancer after implementation of the Leeds pathology protocol (LEEPP): clinically relevant or just academic? World J Surg (2015) 39(2):493–9. doi: 10.1007/s00268-014-2808-4
10. Ren S, Qi Q, Liu S, Duan S, Mao B, Chang Z, et al. Preoperative prediction of pathological grading of hepatocellular carcinoma using machine learning-based ultrasomics: a multicenter study. Eur J Radiol (2021) 143:109891. doi: 10.1016/j.ejrad.2021.109891
11. Fu J, Li WZ, McGrath NA, Lai CW, Brar G, Xiang YQ, et al. Immune checkpoint inhibitor associated hepatotoxicity in primary liver cancer versus other cancers: a systematic review and meta-analysis. Front Oncol (2021) 11:650292. doi: 10.3389/fonc.2021.650292
12. Calderaro J, Ziol M, Paradis V, Zucman-Rossi J. Molecular and histological correlations in liver cancer. J Hepatol (2019) 71(3):616–30. doi: 10.1016/j.jhep.2019.06.001
13. Ameli S, Shaghaghi M, Aliyari Ghasabeh M, Pandey P, Hazhirkarzar B, Ghadimi M, et al. Role of baseline volumetric functional MRI in predicting histopathologic grade and patients’ survival in hepatocellular carcinoma. Eur Radiol (2020) 30(7):3748–58. doi: 10.1007/s00330-020-06742-8
14. Li X, Zhang K, Shi Y, Wang F, Meng X. Correlations between the minimum and mean apparent diffusion coefficient values of hepatocellular carcinoma and tumor grade. J Magnetic Resonance Imaging (2016) 44(6):1442–7. doi: 10.1002/jmri.25323
15. Wang GZ, Guo LF, Gao GH, Li Y, Wang XZ, Yuan ZG. Magnetic resonance diffusion kurtosis imaging versus diffusion-weighted imaging in evaluating the pathological grade of hepatocellular carcinoma. Cancer Manage Res (2020) 12:5147. doi: 10.2147/CMAR.S254371
16. Mao B, Zhang L, Ning P, Ding F, Wu F, Lu G, et al. Preoperative prediction for pathological grade of hepatocellular carcinoma via machine learning–based radiomics. Eur Radiol (2020) 30(12):6924–32. doi: 10.1007/s00330-020-07056-5
17. Xuan Z, Wu N, Li C, Liu Y. Application of contrast-enhanced ultrasound in the pathological grading and prognosis prediction of hepatocellular carcinoma. Trans Cancer Res (2021) 10(9):4106. doi: 10.21037/tcr-21-1264
18. Wu M, Tan H, Gao F, Hai J, Ning P, Chen J, et al. Predicting the grade of hepatocellular carcinoma based on non-contrast-enhanced MRI radiomics signature. Eur Radiol (2019) 29(6):2802–11. doi: 10.1007/s00330-018-5787-2
19. Russo FP, Imondi A, Lynch EN, Farinati F. When and how should we perform a biopsy for HCC in patients with liver cirrhosis in 2018? a review. Digestive liver Dis (2018) 50(7):640–6. doi: 10.1016/j.dld.2018.03.014
20. Di Tommaso L, Spadaccini M, Donadon M, Personeni N, Elamin A, Aghemo A, et al. Role of liver biopsy in hepatocellular carcinoma. World J Gastroenterol (2019) 25(40):6041. doi: 10.3748/wjg.v25.i40.6041
21. Ayuso C, Rimola J, Vilana R, Burrel M, Darnell A, García-Criado Á, et al. Diagnosis and staging of hepatocellular carcinoma (HCC): current guidelines. Eur J Radiol (2018) 101:72–81. doi: 10.1016/j.ejrad.2018.01.025
22. Ayuso C, Rimola J, García-Criado Á. Imaging of HCC. Abdominal Imaging (2012) 37(2):215–30. doi: 10.1007/s00261-011-9794-x
23. Nasu K, Kuroki Y, Tsukamoto T, Nakajima H, Mori K, Minami M, et al. Diffusion-weighted imaging of surgically resected hepatocellular carcinoma: imaging characteristics and relationship among signal intensity, apparent diffusion coefficient, and histopathologic grade. Am J Roentgenol (2009) 193(2):438–44. doi: 10.2214/AJR.08.1424
24. Nishie A, Tajima T, Asayama Y, Ishigami K, Kakihara D, Nakayama T, et al. Diagnostic performance of apparent diffusion coefficient for predicting histological grade of hepatocellular carcinoma. Eur J Radiol (2011) 80(2):e29–33. doi: 10.1016/j.ejrad.2010.06.019
25. Kogita S, Imai Y, Okada M, Kim T, Onishi H, Takamura M, et al. Gd-EOB-DTPA-enhanced magnetic resonance images of hepatocellular carcinoma: correlation with histological grading and portal blood flow. Eur Radiol (2010) 20(10):2405–13. doi: 10.1007/s00330-010-1812-9
26. Wu M, Tan H, Gao F, Hai J, Ning P, Chen J, et al. Predicting the grade of hepatocellular carcinoma based on non-contrast-enhanced MRI radiomics signature. Eur Radiol (2019) 29(6):2802–11. doi: 10.1007/s00330-018-5787-2
27. Oh J, Lee JM, Park J, Joo I, Yoon J. H, Lee D. H, et al. Hepatocellular carcinoma: texture analysis of preoperative computed tomography images can provide markers of tumor grade and disease-free survival. Korean J Radiol (2019) 20(4):569–79. doi: 10.3348/kjr.2018.0501
28. Seitz K, Bernatik T, Strobel D, Blank W, Friedrich-Rust M, Strunk H, et al. Contrast-enhanced ultrasound (CEUS) for the characterization of focal liver lesions in clinical practice (DEGUM multicenter trial): CEUS vs. MRI–a prospective comparison in 269 patients. Ultraschall der Medizin-European J Ultrasound (2010) 31(05):492–9. doi: 10.1055/s-0029-1245591
29. Hu HT, Wang W, Chen LD, Ruan SM, Chen SL, Li X, et al. Artificial intelligence assists identifying malignant versus benign liver lesions using contrast-enhanced ultrasound. J Gastroenterol Hepatol (2021) 36(10):2875–83. doi: 10.1111/jgh.15522
30. Liu YH, Fan ZH, Yin SS, Yan K, Sun LQ, Jiang BB, et al. Diagnostic value of color parametric imaging and contrast-enhanced ultrasound in the differentiation of hepatocellular adenoma and well-differentiated hepatocellular carcinoma. J Clin Ultrasound (2022) 50(2):216–21. doi: 10.1002/jcu.23138
31. Wang W, Wu SS, Zhang JC, Xian MF, Huang H, Li W, et al. Preoperative pathological grading of hepatocellular carcinoma using ultrasomics of contrast-enhanced ultrasound. Acad Radiol (2021) 28(8):1094–101. doi: 10.1016/j.acra.2020.05.033
32. Kumar V, Gu Y, Basu S, Berglund A, Eschrich SA, Schabath MB, et al. Radiomics: the process and the challenges. Magnetic resonance Imaging (2012) 30(9):1234–48. doi: 10.1016/j.mri.2012.06.010
33. Yushkevich PA, Gao Y, Gerig G. (2016)., in: ITK-SNAP: An interactive tool for semi-automatic segmentation of multi-modality biomedical images[C]//2016 38th annual international conference of the IEEE engineering in medicine and biology society (EMBC), Orlando, FL, USA. pp. 3342–5. doi: 10.1109/EMBC.2016.7591443
34. Van Griethuysen JJM, Fedorov A, Parmar C, Hosny A, Aucoin N, Narayan V, et al. Computational radiomics system to decode the radiographic phenotype. Cancer Res (2017) 77(21):e104–7. doi: 10.1158/0008-5472.CAN-17-0339
35. Marrero JA, Kulik LM, Sirlin CB, Zhu AX, Finn RS, Abecassis MM, et al. Diagnosis, s taging, and m anagement of h epatocellular c arcinoma: 2018 p ractice G uidance by the a merican a ssociation for the s tudy of l iver d iseases. Hepatology (2018) 68(2):723–50. doi: 10.1002/hep.29913
36. Kim SU, Jung KS, Lee S, Park JY, Kim DY, Ahn SH, et al. Histological subclassification of cirrhosis can predict recurrence after curative resection of hepatocellular carcinoma. Liver Int (2014) 34(7):1008–17. doi: 10.1111/liv.12475
37. Dietrich CF, Nolsøe CP, Barr RG, Berzigotti A, Burns PN, Cantisani V, et al. Guidelines and good clinical practice recommendations for contrast enhanced ultrasound (CEUS) in the liver–update 2020–WFUMB in cooperation with EFSUMB, AFSUMB, AIUM, and FLAUS[J]. Ultraschall der Medizin-European J Ultrasound (2020) 41(05):562–85. doi: 10.1055/a-1177-0530
38. Sugimoto K, Shiraishi J, Tanaka H, Tsuchiya K, Aso K, Kobayashi Y, et al. Computer-aided diagnosis for estimating the malignancy grade of hepatocellular carcinoma using contrast-enhanced ultrasound: an ROC observer study. Liver Int (2016) 36(7):1026–32. doi: 10.1111/liv.13043
39. Cheng MQ, Hu HT, Huang H, Pan JM, Xian MF, Huang Y, et al. Pathological considerations of CEUS LI-RADS: correlation with fibrosis stage and tumour histological grade. Eur Radiol (2021) 31:5680–8. doi: 10.1007/s00330-020-07660-5
40. Tada T, Kumada T, Toyoda H, Ito T, Sone Y, Kaneoka Y, et al. Utility of contrast-enhanced ultrasonography with perflubutane for determining histologic grade in hepatocellular carcinoma. Ultrasound Med Biol (2015) 41(12):3070–8. doi: 10.1016/j.ultrasmedbio.2015.07.023
41. Oestmann PM, Wang CJ, Savic LJ, Hamm CA, Stark S, Schobert I, et al. Deep learning-assisted differentiation of pathologically proven atypical and typical hepatocellular carcinoma (HCC) versus non-HCC on contrast-enhanced MRI of the liver. Eur Radiol (2021) 31(7):4981–90. doi: 10.1007/s00330-020-07559-1
42. Ma QP, He X, Li K, Wang J. F, Zeng Q. J, Xu EJ, et al. Dynamic contrast-enhanced ultrasound radiomics for hepatocellular carcinoma recurrence prediction after thermal ablation. Mol Imaging Biol (2021) 23:572–85. doi: 10.1007/s11307-021-01578-0
43. Wei J, Ji Q, Gao Y, Yang X, Guo D, Gu D, et al. A multi-scale, multi-region and attention mechanism-based deep learning framework for prediction of grading in hepatocellular carcinoma. Med Phys (2022) 50(4):2290–302. doi: 10.1002/mp.16127
Keywords: hepatocellular carcinoma, Edmondson-Steiner grade, contrast-enhanced ultrasound, radiomics, ensemble learning
Citation: Wang Y, Yuan D, Sun H, Pan X, Lu F, Li H, Huang Y and Tang S (2023) Non-invasive preoperative prediction of Edmondson-Steiner grade of hepatocellular carcinoma based on contrast-enhanced ultrasound using ensemble learning. Front. Oncol. 13:1116129. doi: 10.3389/fonc.2023.1116129
Received: 05 December 2022; Accepted: 15 May 2023;
Published: 05 July 2023.
Edited by:
John Gibbs, Hackensack Meridian Health, United StatesCopyright © 2023 Wang, Yuan, Sun, Pan, Lu, Li, Huang and Tang. This is an open-access article distributed under the terms of the Creative Commons Attribution License (CC BY). The use, distribution or reproduction in other forums is permitted, provided the original author(s) and the copyright owner(s) are credited and that the original publication in this journal is cited, in accordance with accepted academic practice. No use, distribution or reproduction is permitted which does not comply with these terms.
*Correspondence: Hong Li, bGlob25nQGJtaWUubmV1LmVkdS5jbg==; Shaoshan Tang, dGFuZ3NzQHNqLWhvc3BpdGFsLm9yZw==
Disclaimer: All claims expressed in this article are solely those of the authors and do not necessarily represent those of their affiliated organizations, or those of the publisher, the editors and the reviewers. Any product that may be evaluated in this article or claim that may be made by its manufacturer is not guaranteed or endorsed by the publisher.
Research integrity at Frontiers
Learn more about the work of our research integrity team to safeguard the quality of each article we publish.