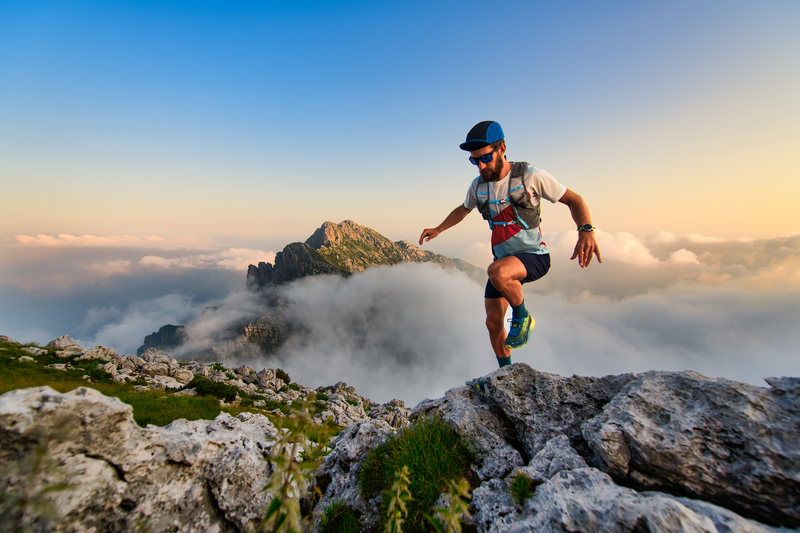
95% of researchers rate our articles as excellent or good
Learn more about the work of our research integrity team to safeguard the quality of each article we publish.
Find out more
SYSTEMATIC REVIEW article
Front. Oncol. , 07 June 2023
Sec. Cancer Imaging and Image-directed Interventions
Volume 13 - 2023 | https://doi.org/10.3389/fonc.2023.1114983
Background/Objective: Early recurrence (ER) affects the long-term survival prognosis of patients with hepatocellular carcinoma (HCC). Many previous studies have utilized CT/MRI-based radiomics to predict ER after radical treatment, achieving high predictive value. However, the diagnostic performance of radiomics for the preoperative identification of ER remains uncertain. Therefore, we aimed to perform a meta-analysis to investigate the predictive performance of radiomics for ER in HCC.
Methods: A systematic literature search was conducted in PubMed, Web of Science (including MEDLINE), EMBASE and the Cochrane Central Register of Controlled Trials to identify studies that utilized radiomics methods to assess ER in HCC. Data were extracted and quality assessed for retrieved studies. Statistical analyses included pooled data, tests for heterogeneity, and publication bias. Meta-regression and subgroup analyses were performed to investigate potential sources of heterogeneity.
Results: The analysis included fifteen studies involving 3,281 patients focusing on preoperative CT/MRI-based radiomics for the prediction of ER in HCC. The combined sensitivity, specificity, and area under the curve (AUC) of the receiver operating characteristic were 75% (95% CI: 65-82), 78% (95% CI: 68-85), and 83% (95% CI: 79-86), respectively. The combined positive likelihood ratio, negative likelihood ratio, diagnostic score, and diagnostic odds ratio were 3.35 (95% CI: 2.41-4.65), 0.33 (95% CI: 0.25-0.43), 2.33 (95% CI: 1.91-2.75), and 10.29 (95% CI: 6.79-15.61), respectively. Substantial heterogeneity was observed among the studies (I²=99%; 95% CI: 99-100). Meta-regression showed imaging equipment contributed to the heterogeneity of specificity in subgroup analysis (P= 0.03).
Conclusion: Preoperative CT/MRI-based radiomics appears to be a promising and non-invasive predictive approach with moderate ER recognition performance.
Hepatocellular carcinoma (HCC) is one of the most common malignant tumors in the world, and the incidence and mortality rate of the disease in the world is increasing year by year (1–3). At present, the situation that HCC patients in China account for nearly half of the world’s total population is not optimistic, which has brought great challenges to the healthcare system and a substantial socioeconomic burden in China (4, 5).
Currently, the radical methods of HCC are still radical resection, liver transplantation, ablation, and other treatments such as vascular intervention have also achieved satisfactory results (6). However, no matter which method is used, some patients still experience poor efficacy and a certain rate of early recurrence (ER) (21.9%-70.2%) after HCC treatment (7, 8).
ER following radical treatment for HCC is defined as intrahepatic or extrahepatic recurrence within one to two years (9, 10). ER is a significant factor affecting HCC prognosis and disease outcome (11). Thus, the identification of sensitive markers associated with ER of HCCs is crucial in facilitating accurate prognostic classification and timely interventions which, in turn, improve overall survival rates. Fortunately, in recent years, radiomics has demonstrated a unique advantage (12). By extracting significant amounts of image information from computed tomography (CT) and magnetic resonance imaging (MRI) with high throughput, it facilitates tumor segmentation, feature extraction, and model establishment. Radiomics enables clinicians to analyze vast image data information in-depth, thereby assisting them in making more accurate diagnoses and predictions.
Many previous studies have utilized CT/MRI-based radiomics to predict ER after radical treatment, achieving high predictive value (7, 9, 10, 13, 14). However, the reported results seem quite variable due to the fact that these above studies differed in the diagnostic performance of the preoperative evaluation of ER because the differences in imaging modalities, research methods, sample size, etc. For these reasons, the diagnostic performance of radiomics for the preoperative identification of ER remains uncertain. Therefore, we performed this meta-analysis to investigate the predictive performance of preoperative CT/MRI-based radiomics for ER in HCC.
This study was conducted strictly according to the Preferred Reporting Items for Systematic reviews and Meta-Analysis of Diagnostic Test Accuracy (PRISMA-DTA) (15).
In this study, the following Medical Subject Headings (MeSH) and their variations were used in PubMed, Web of Science (all databases including MEDLINE), EMBASE and the Cochrane Central Register of Controlled Trials from inception to December 24, 2022: (“hepatocellular carcinoma” OR “carcinoma, hepatocellular” OR “HCC”) AND (“CT”, “computed tomography”) AND (“diagnosis” OR “accuracy” OR “specificity” OR “sensitivity”) AND (“MRI” OR “magnetic resonance imaging”) AND (“Radiomic*”). PubMed’s search strategy was as follows: ((“hepatocellular carcinoma”[Title/Abstract] OR “carcinoma hepatocellular”[Title/Abstract] OR “HCC”[Title/Abstract]) AND (“diagnosis”[Title/Abstract] OR “accuracy”[Title/Abstract] OR “specificity”[Title/Abstract] OR “sensitivity”[Title/Abstract]) AND (“CT”[Title/Abstract] OR “computed tomography”[Title/Abstract]) AND “radiomic*”[Title/Abstract]) OR ((“hepatocellular carcinoma”[Title/Abstract] OR “carcinoma hepatocellular”[Title/Abstract] OR “HCC”[Title/Abstract]) AND (“diagnosis”[Title/Abstract] OR “accuracy”[Title/Abstract] OR “specificity”[Title/Abstract] OR “sensitivity”[Title/Abstract]) AND (“MRI”[Title/Abstract] OR “magnetic resonance imaging”[Title/Abstract]) AND “radiomic*”[Title/Abstract]). There are no language restrictions in the literature search process.
The inclusion criteria were as follows: we included all eligible radiomics articles that used CT or MRI to assess ER (which was defined as the presence of new intrahepatic lesions or metastasis with typical imaging features of HCC (who experienced radical resection, radical ablation, and liver transplantation), or atypical findings with histopathological confirmation within 2 years) in patients with HCC. The exclusion criteria were as follows: (1) the studies did not have enough information to construct a two-by-two contingency table; (2) antitumor therapy was performed preoperatively; (3) academic review, conference abstracts, animal experiments, expert opinions, books, nondiagnostic tests, guidelines, and case report.
Two independent researchers (HT and YX) (radiologists with 6 and 5 years of experience) extracted data from the included studies: first author, publication year, study period, study design (prospective or retrospective), cohort detail, demographic characteristics of the study population (such as age, gender), sample size, comorbid conditions, Tumor information (diameter, number, margin, etc.), true positive (TP), false positive (FP), false negative (FN), and true negative (TN), interval from post-operation to early recurrence, CT/MRI imaging equipment, contrast agent, treatment, MRI sequence, radiomics features extracting software. Inconsistencies between the two investigators are resolved through discussion or consultation with the senior investigator.
We evaluated the methodological quality of the included studies by using the standard Quality Assessment of Diagnostic Accuracy Studies-2 (QUADAS-2) tool (Bristol University, Bristol, UK) (16). We assessed the risk of bias and concerns regarding applicability by the software Review Manager 5.4 (Cochrane Library Software, Oxford, UK; available at https://training.cochrane.org/online-learning/core-software/revman). The four aspects of the evaluation are as follows: patient selection, index test, reference standard, and flow and timing. Risk of bias was classified as ‘yes’, ‘no’ or ‘unclear’ and applicability ‘high’, ‘low’ or ‘unclear’. Furthermore, radiomics quality score (RQS) were used to assess the quality of radiomics studies (17).
In this study, Stata17.0 (Stata Corp LP, College Station, Texas, SA) was used for all statistical calculation. We used a bivariate regression model to calculate the combined sensitivity, specificity, positive likelihood ratio (PLR), negative likelihood ratio (NLR), diagnostic score, and diagnostic odds ratio (DOR) with their corresponding 95% confidence intervals (CI), all of which are shown in forest plots. We plotted the pooled results onto the summary receiver operating characteristic (SROC) curve and determined the area under the receiver operating characteristic curve (AUC) to reflect the diagnostic power of the included studies. In addition, the Stata Midas module was used to detect the magnitude of heterogeneity due to threshold effects. The threshold effect test was obtained by calculating the spearman correlation coefficient of the logarithm of sensitivity to the logarithm of (1-specificity), and if P is less than 0.05, a threshold effect is present. Heterogeneity caused by non-threshold effects was measured using Cochrane’s Q-test and inconsistency index I2, and the difference was considered significant when P <0.05, with I2 ≥ 50% regarded as being indicative of moderate-to-high heterogeneity among studies (18). In addition, we plotted Fagan nomogram through the Midas module, and post-test probabilities were calculated by pre-test probabilities (the ratio of ER-positive cases to all cases in the included studies), PLR, and NLR. Furthermore, publication bias was assessed by using the Deeks asymmetric regression test. Finally, meta-regression and subgroup analysis were used to explore potential sources of heterogeneity. All hypothesis tests were statistically significant with P <0.05 (two-sided).
A total of 520 relevant literature were generated through the systematic search. The process of literature search and study selection is presented in Figure 1. After screening according to the pre-defined inclusion/exclusion criteria, a total of 15 publications were identified for this meta-analysis, consisting of six radiomics studies based on CT and nine radiomics studies based on MRI (7, 9, 10, 13, 14, 19–28). Most of the studies included in the analysis were retrospective, and all of them were published between 2017 and 2022. The analysis included a total of 3,281 patients with hepatocellular carcinoma. The characteristics of these studies are presented in Tables 1, 2.
Figure 2 illustrated the results of the methodological quality assessment of the included studies based on the Quality Assessment of Diagnostic Accuracy Studies-2 (QUADAS-2) scale. Most studies were deemed to have a low to moderate risk of bias, with only mild concerns regarding their applicability. The included articles, as assessed by the Radiomics Quality Score (RQS) presented in Table 3, were determined to be of generally low quality.
Figure 2 Stacked bar charts of the QUADAS-2 scale of methodological quality assessment. (A) Risk of bias and applicability concerns summary: review authors’ judgements about each domain for each included study; (B) Risk of bias and applicability concerns graph: review authors’ judgements about each domain presented as percentages across included studies.
The results of our study indicated high heterogeneity (Q= 211.53; I2 = 99%; 95%CI: 99-100), which required us to use the random-effects model to combine effect sizes. Additionally, the heterogeneity of sensitivity, specificity, PLR, NLR, diagnostic score, and DOR all exceeded 50%, making it necessary to account for this in our analysis. The threshold effect test showed the Spearman correlation coefficient to be 0.364 with P<0.182, implying no threshold effect and lending support to the combination of sensitivity and specificity.
The pooled sensitivity, specificity, and AUC were 75% (95% CI: 65-82), 78% (95% CI: 68-85), and 83% (95% CI: 79-86), respectively (Figures 3, 4). The combined PLR, NLR, diagnostic score, and DOR were 3.35 (95% CI: 2.41-4.65), 0.33 (95% CI: 0.25-0.43), 2.33 (95% CI: 1.91-2.75), and 10.29 (95% CI: 6.79-15.61), respectively (Figures 5, 6). The scatter plot of the likelihood ratios showed that the pooled estimates with 95%CI were located in the lower right quadrant, suggesting that the combined accuracy of CT-based radiomics for diagnosing ER was moderate (Figure 7).
Figure 3 Forest plots show the performance estimates (sensitivity and specificity) of each study based on radiomics for the preoperative prediction of ER in HCC.
Figure 4 Forest plots show the performance estimates (SROC) of each study based on radiomics for the preoperative prediction of ER in HCC.
Figure 5 Forest plots show the performance estimates (PLR and NLR) of each study based on radiomics for the preoperative prediction of ER in HCC.
Figure 6 Forest plots show the performance estimates (diagnostic score, and DOR) of each study based on radiomics for the preoperative prediction of ER in HCC.
Figure 7 Distribution scatter diagram of the likelihood ratio (LR+/LR-) of each study and combined estimated value.
A 39% predicted probability was assessed to simulate a clinical situation, resulting in a posttest probability of 68% for a positive test result, and the negative posttest probability was 17% (Figure 8).
The Deek funnel plot showed a slope coefficient of 0.80, indicating that there was no publication bias in the included studies (Figure 9).
The forest plots indicated high heterogeneity with I2 values > 50% for sensitivity (I²=91%; 95% CI: 87-94, P< 0.001) and specificity (I²=92%; 95% CI: 89-95, P< 0.001). To identify the source of heterogeneity, we performed univariable meta-regression analysis. Table 4 showed the results of univariable meta-regression and subgroup analyses to explore the influence of sample size, imaging equipment, modeling method, radiomics features extracting software. The results showed imaging equipment contributed to the heterogeneity in the specificity analysis (P= 0.03).
In terms of imaging equipment, CT (n=6) had a higher specificity (83%; 95% CI: 72-94) than MRI (n=9) (73%; 95% CI: 62-85) (P= 0.03). However, the sensitivity [(67%; 95CI%: 53-82) vs. (79%; 95CI%: 70-88)] was basically equivalent for both (P= 0.75).
In terms of sample size, modeling method, radiomics features extracting software, the sensitivity (P= 0.25, 0.11, 0.86, respectively) were basically equivalent for both.
To our knowledge, this is the first study to investigate the predictive performance of preoperative CT/MRI-based radiomics for ER in HCC. Our meta-analysis showed moderate pooled sensitivity, specificity, and AUC were 75% (95% CI: 65-82), 78% (95% CI: 68-85), and 83% (95% CI: 79-86), respectively, which demonstrated radiomics has the potential to preoperatively differentiate ER for HCC. The confirmation of this evidence will help ER patients to develop the best postoperative diagnosis and treatment strategy, which plays a critical role in individualized risk stratification and further treatment guidance. For example, if preoperative radiomics highly suggests the possibility of future ER, more frequent follow-up should be taken for this population, the activity of the original tumor lesion should be closely monitored, and corresponding measures can be taken early to intervene, which is of great clinical significance for prolonging the survival of patients and improving long-term prognosis (11).
The likelihood ratio and the probability after the test also provide us with important information about the likelihood that a patient with a positive or negative test actually has ER or not. Our analysis found a PLR of 3.35 indicates that the test is 3.35 times more likely to correctly judge a positive result than to misjudge a positive result, resulting in a 68% post-test probability of a positive test result. Similarly, an NLR value of 0.33 indicates that the test is 0.33 times more likely to misjudge a negative result than to correctly judge a negative result, resulting in a 17% probability of a negative test result. These results further suggest that radiomics has important clinical value in preoperative evaluation of the occurrence of ER in HCC follow-up.
Although the test for heterogeneity showed significant variability among the included studies, the threshold effect test, measured by the Spearman correlation coefficient (0.364, P=0.182), indicated that the heterogeneity was not arise from threshold effects. Therefore, we performed meta-regression and subgroup analyses to try to explore possible sources of heterogeneity. Due to the limited number of included studies (n=15), we performed only univariate rather than multivariate meta-regression analyses. We used five key factors for subgroup analyses. Then, we performed subgroup analysis to compare the diagnostic performance of radiomics based on different imaging equipment. The results showed the sensitivity of CT and MRI was comparable. However, CT (n=6) has higher specificity than MRI (n=9) in predicting ER. Our comprehensive literature search failed to identify any studies that directly compared the performance of CT and MRI in predicting ER. This may be attributed to the fact that the majority of the literature reviewed consisted of diverse MRI sequence compositions with significant variations in sensitivity and specificity depending on the sequence selected (e.g., Zhang et al. used significantly fewer sequences than Chong et al.) (19, 22). However, CT uses radiomics of fixed parameters (enhanced arterial phase combined with venous phase) to predict ER, and imaging parameters are more uniform than MRI. Moreover, MRI image acquisition is more technically complex and sensitive to artifacts and image quality inhomogeneity in the diagnosis of HCC compared to CT. As such, patients with poor breath-holding ability or ascites may experience difficulties during MRI scanning, leading to lower quality images (29). Consequently, this may result in radiomics models failing to provide accurate and relevant imaging biomarker information for predicting ER of HCC. The pooled results which favored CT over MRI are not conclusive. Prospective, large-scale, and multi-center studies are needed to establish the superiority of one imaging method over the other. Although there is significant heterogeneity in sensitivity, we found no source of heterogeneity by regression analysis. We attempted to exclude the study by Wang et al. (10) due to its low sensitivity, but heterogeneity remained at 91.16% (95% CI: 87.56-94.76) (results not listed).
Recently, radiomics has developed rapidly and has been widely used in the study of tumors in different systems, including HCC. In recent years, with the application of the theoretical system and technical framework of radiomics in the study of ER of HCC, the prediction performance has also tended to be stable. However, there are still challenges that need to be addressed before its application in clinical practice. The lack of standardization in imaging equipment and acquisition parameters makes it difficult to uniformly analyze radiomics features, which may affect the practicality and feasibility of the model. In the future, a possible solution is to integrate the features extracted from both CT and MRI imaging methods may create a new breakthrough in radiomics in the prediction of ER of HCC. Establishing a comprehensive HCC image database and enabling data sharing is crucial to train and validate predictive models that simulate the real state and reduce non-biological differences.
The integration of radiomics and multi-omics data may lead to the emergence of multi-omics artificial intelligence, which can play a greater role in personalized medicine via non-invasive and personalized methods for assessing the occurrence, development, and prognosis of HCC. Furthermore, intelligent segmentation of imaging lesions will be a focus of future radiomics research, and potential correlations between radiomics features and biological characteristics can be further clarified through radiogenomics-related index features. However, the complex relationship between radiomics features and biological behavior may be difficult to fully eliminate.
Our meta-analysis had some limitations. Firstly, most studies (n=14) we included were retrospective, and patient selection may introduce some inherent bias. Secondly, all included studies were from China. Therefore, there are differences in disease background due to different regions, countries, ethnicities, etc., and HCC is highly heterogeneous, which may affect the general applicability of the results in clinical practice. Thirdly, not all included studies used uniform pathological biopsy to confirm the diagnosis of ER, and the diagnosis was interspersed with imaging (although only for those with typical radiographic findings), and the interpretation of imaging images may depend on the reader’s experience, which may partly explain differences in sensitivity and specificity between studies. Fourthly, we only searched the foreign language databases, which may introduce publication bias. However, we believe that this bias should be relatively small, as no publication bias was observed in this study. Moreover, the heterogeneity in imaging modality, feature extraction among related studies needs to be carefully considered as radiomics is a platform method rather than a single marker. Finally, although CT/MRI radiomics models are helpful in identifying ER, the modeling methods used may affect the predictions of radiomics analysis. Each study developed a different radiomics model, so the current meta-analysis does not clarify a clear modeling approach to diagnose ER.
In summary, this meta-analysis suggested that preoperative CT/MRI radiomics is a promising noninvasive predictive method with moderate ER recognition performance and is a crucial guide for clinical follow-up planning of postoperative HCC patients. We believe that prospective, large-scale, and multicenter studies utilizing multimodal radiomics methods will improve the predictive power of ER in the future.
The original contributions presented in the study are included in the article/supplementary material. Further inquiries can be directed to the corresponding author.
Study concepts and design: HT, YX, and ZW. Literature research: HT and YX. Data collection: HT and YX. Data analysis: HT and YX. Manuscript writing: HT. Manuscript review: ZW. All authors contributed to the article and approved the submitted version.
The authors declare that the research was conducted in the absence of any commercial or financial relationships that could be construed as a potential conflict of interest.
All claims expressed in this article are solely those of the authors and do not necessarily represent those of their affiliated organizations, or those of the publisher, the editors and the reviewers. Any product that may be evaluated in this article, or claim that may be made by its manufacturer, is not guaranteed or endorsed by the publisher.
1. Bray F, Ferlay J, Soerjomataram I, Siegel RL, Torre LA, Jemal A. Global cancer statistics 2018: GLOBOCAN estimates of incidence and mortality worldwide for 36 cancers in 185 countries. CA Cancer J Clin (2018) 68(6):394–424. doi: 10.3322/caac.21492
2. Sung H, Ferlay J, Siegel RL, Laversanne M, Soerjomataram I, Jemal A, et al. Global cancer statistics 2020: GLOBOCAN estimates of incidence and mortality worldwide for 36 cancers in 185 countries. CA Cancer J Clin (2021) 71(3):209–49. doi: 10.3322/caac.21660
3. McGlynn KA, Petrick JL, El-Serag HB. Epidemiology of hepatocellular carcinoma. Hepatology (2021) 73 Suppl 1(Suppl 1):4–13. doi: 10.1002/hep.31288
4. Chen W, Sun K, Zheng R, Zeng H, Zhang S, Xia C, et al. Cancer incidence and mortality in China, 2014. Chin J Cancer Res (2018) 30(1):1–12. doi: 10.21147/j.issn.1000-9604.2018.01.01
5. Samant H, Amiri HS, Zibari GB. Addressing the worldwide hepatocellular carcinoma: epidemiology, prevention and management. J Gastrointest Oncol (2021) 12(Suppl 2):S361–73. doi: 10.21037/jgo.2020.02.08
6. European Association for the Study of the Liver. Electronic address eee, European association for the study of the l. EASL clinical practice guidelines: management of hepatocellular carcinoma. J Hepatol (2018) 69(1):182–236. doi: 10.1016/j.jhep.2018.03.019
7. Zhao JW, Shu X, Chen XX, Liu JX, Liu MQ, Ye J, et al. Prediction of early recurrence of hepatocellular carcinoma after liver transplantation based on computed tomography radiomics nomogram. Hepatobiliary Pancreat Dis Int (2022) 21(6):543–50. doi: 10.1016/j.hbpd.2022.05.013
8. Shen JX, Zhou Q, Chen ZH, Chen QF, Chen SL, Feng ST, et al. Longitudinal radiomics algorithm of posttreatment computed tomography images for early detecting recurrence of hepatocellular carcinoma after resection or ablation. Transl Oncol (2021) 14(1):100866. doi: 10.1016/j.tranon.2020.100866
9. Wu C, Yu S, Zhang Y, Zhu L, Chen S, Liu Y. CT-based radiomics nomogram improves risk stratification and prediction of early recurrence in hepatocellular carcinoma after partial hepatectomy. Front Oncol (2022) 12:896002. doi: 10.3389/fonc.2022.896002
10. Wang F, Cheng M, Du B, Li LM, Huang WP, Gao JB. Use of radiomics containing an effective peritumoral area to predict early recurrence of solitary hepatocellular carcinoma </=5 cm in diameter. Front Oncol (2022) 12:1032115. doi: 10.3389/fonc.2022.1032115
11. Meniconi RL, Komatsu S, Perdigao F, Boelle PY, Soubrane O, Scatton O. Recurrent hepatocellular carcinoma: a Western strategy that emphasizes the impact of pathologic profile of the first resection. Surgery (2015) 157(3):454–62. doi: 10.1016/j.surg.2014.10.011
12. Ji GW, Zhu FP, Xu Q, Wang K, Wu MY, Tang WW, et al. Radiomic features at contrast-enhanced CT predict recurrence in early stage hepatocellular carcinoma: a multi-institutional study. Radiology (2020) 294(3):568–79. doi: 10.1148/radiol.2020191470
13. Li W, Shen H, Han L, Liu J, Xiao B, Li X, et al. A multiparametric fusion radiomics signature based on contrast-enhanced MRI for predicting early recurrence of hepatocellular carcinoma. J Oncol (2022) 2022:3704987. doi: 10.1155/2022/3704987
14. Gao W, Wang W, Song D, Yang C, Zhu K, Zeng M, et al. A predictive model integrating deep and radiomics features based on gadobenate dimeglumine-enhanced MRI for postoperative early recurrence of hepatocellular carcinoma. Radiol Med (2022) 127(3):259–71. doi: 10.1007/s11547-021-01445-6
15. McInnes MDF, Moher D, Thombs BD, McGrath TA, Bossuyt PM, , The PRISMA-DTA Group, et al. Preferred reporting items for a systematic review and meta-analysis of diagnostic test accuracy studies: the PRISMA-DTA statement. JAMA (2018) 319(4):388–96. doi: 10.1001/jama.2017.19163
16. Whiting PF, Rutjes AW, Westwood ME, Mallett S, Deeks JJ, Reitsma JB, et al. QUADAS-2: a revised tool for the quality assessment of diagnostic accuracy studies. Ann Intern Med (2011) 155(8):529–36. doi: 10.7326/0003-4819-155-8-201110180-00009
17. Lambin P, Leijenaar RTH, Deist TM, Peerlings J, de Jong EEC, van Timmeren J, et al. Radiomics: the bridge between medical imaging and personalized medicine. Nat Rev Clin Oncol (2017) 14(12):749–62. doi: 10.1038/nrclinonc.2017.141
18. Higgins JP, Thompson SG, Deeks JJ, Altman DG. Measuring inconsistency in meta-analyses. BMJ (2003) 327(7414):557–60. doi: 10.1136/bmj.327.7414.557
19. Zhang Z, Jiang H, Chen J, Wei Y, Cao L, Ye Z, et al. Hepatocellular carcinoma: radiomics nomogram on gadoxetic acid-enhanced MR imaging for early postoperative recurrence prediction. Cancer Imaging (2019) 19(1):22. doi: 10.1186/s40644-019-0209-5
20. Zhang L, Cai P, Hou J, Luo M, Li Y, Jiang X. Radiomics model based on gadoxetic acid disodium-enhanced MR imaging to predict hepatocellular carcinoma recurrence after curative ablation. Cancer Manag Res (2021) 13:2785–96. doi: 10.2147/CMAR.S300627
21. Wang L, Ma X, Feng B, Wang S, Liang M, Li D, et al. Multi-sequence MR-based radiomics signature for predicting early recurrence in solitary hepatocellular carcinoma </=5 cm. Front Oncol (2022) 12:899404. doi: 10.3389/fonc.2022.899404
22. Chong H, Gong Y, Pan X, Liu A, Chen L, Yang C, et al. Peritumoral dilation radiomics of gadoxetate disodium-enhanced MRI excellently predicts early recurrence of hepatocellular carcinoma without macrovascular invasion after hepatectomy. J Hepatocell Carcinoma (2021) 8:545–63. doi: 10.2147/JHC.S309570
23. Wang L, Feng B, Wang S, Hu J, Liang M, Li D, et al. Diagnostic value of whole-tumor apparent diffusion coefficient map radiomics analysis in predicting early recurrence of solitary hepatocellular carcinoma </= 5 cm. Abdom Radiol (NY) (2022) 47(9):3290–300. doi: 10.1007/s00261-022-03582-6
24. Ning P, Gao F, Hai J, Wu M, Chen J, Zhu S, et al. Application of CT radiomics in prediction of early recurrence in hepatocellular carcinoma. Abdom Radiol (NY) (2020) 45(1):64–72. doi: 10.1007/s00261-019-02198-7
25. Shan QY, Hu HT, Feng ST, Peng ZP, Chen SL, Zhou Q, et al. CT-based peritumoral radiomics signatures to predict early recurrence in hepatocellular carcinoma after curative tumor resection or ablation. Cancer Imaging (2019) 19(1):11. doi: 10.1186/s40644-019-0197-5
26. Zhou Y, He L, Huang Y, Chen S, Wu P, Ye W, et al. CT-based radiomics signature: a potential biomarker for preoperative prediction of early recurrence in hepatocellular carcinoma. Abdom Radiol (NY) (2017) 42(6):1695–704. doi: 10.1007/s00261-017-1072-0
27. Zhao Y, Wu J, Zhang Q, Hua Z, Qi W, Wang N, et al. Radiomics analysis based on multiparametric MRI for predicting early recurrence in hepatocellular carcinoma after partial hepatectomy. J Magn Reson Imaging (2021) 53(4):1066–79. doi: 10.1002/jmri.27424
28. Ren Y, Bo L, Shen B, Yang J, Xu S, Shen W, et al. Development and validation of a clinical-radiomics model to predict recurrence for patients with hepatocellular carcinoma after curative resection. Med Phys (2023) 50(2):778–90. doi: 10.1002/mp.16061
Keywords: hepatocellular carcinoma, radiomics, early recurrence, prediction, sensitivity, specificity, meta-analysis
Citation: Tian H, Xie Y and Wang Z (2023) Radiomics for preoperative prediction of early recurrence in hepatocellular carcinoma: a meta-analysis. Front. Oncol. 13:1114983. doi: 10.3389/fonc.2023.1114983
Received: 03 December 2022; Accepted: 18 May 2023;
Published: 07 June 2023.
Edited by:
Davide Bellini, Sapienza University of Rome, ItalyReviewed by:
Madjid Soltani, University of Waterloo, CanadaCopyright © 2023 Tian, Xie and Wang. This is an open-access article distributed under the terms of the Creative Commons Attribution License (CC BY). The use, distribution or reproduction in other forums is permitted, provided the original author(s) and the copyright owner(s) are credited and that the original publication in this journal is cited, in accordance with accepted academic practice. No use, distribution or reproduction is permitted which does not comply with these terms.
*Correspondence: Zhiqun Wang, d2FuZ3poaXF1bkAxMjYuY29t
Disclaimer: All claims expressed in this article are solely those of the authors and do not necessarily represent those of their affiliated organizations, or those of the publisher, the editors and the reviewers. Any product that may be evaluated in this article or claim that may be made by its manufacturer is not guaranteed or endorsed by the publisher.
Research integrity at Frontiers
Learn more about the work of our research integrity team to safeguard the quality of each article we publish.