- Department of Radiation Oncology, The Affiliated Huaian No.1 People’s Hospital of Nanjing Medical University, Huaian, Jiangsu, China
Purpose: This study aims to develop and validate a prediction model for non-operative, epidermal growth factor receptor (EGFR)-positive, locally advanced elderly esophageal cancer (LAEEC).
Methods: A total of 80 EGFR-positive LAEEC patients were included in the study. All patients underwent radiotherapy, while 41 cases received icotinib concurrent systemic therapy. A nomogram was established using univariable and multivariable Cox analyses. The model’s efficacy was assessed through area under curve (AUC) values, receiver operating characteristic (ROC) curves at different time points, time-dependent AUC (tAUC), calibration curves, and clinical decision curves. Bootstrap resampling and out-of-bag (OOB) cross-validation methods were employed to verify the model’s robustness. Subgroup survival analysis was also conducted.
Results: Univariable and multivariable Cox analyses revealed that icotinib, stage, and ECOG score were independent prognostic factors for LAEEC patients. The AUCs of model-based prediction scoring (PS) for 1-, 2-, and 3-year overall survival (OS) were 0.852, 0.827, and 0.792, respectively. Calibration curves demonstrated that the predicted mortality was consistent with the actual mortality. The time-dependent AUC of the model exceeded 0.75, and the internal cross-validation calibration curves showed good agreement between predicted and actual mortality. Clinical decision curves indicated that the model had a substantial net clinical benefit within a threshold probability range of 0.2 to 0.8. Model-based risk stratification analysis demonstrated the model’s excellent ability to distinguish survival risk. Further subgroup analyses showed that icotinib significantly improved survival in patients with stage III and ECOG score of 1 (HR 0.122, P<0.001).
Conclusions: Our nomogram model effectively predicts the overall survival of LAEEC patients, and the benefits of icotinib were found in the clinical stage III population with good ECOG scores.
1 Introduction
Currently, concurrent chemoradiotherapy (CCRT) is the preferred treatment for unresectable locally advanced elderly esophageal carcinoma (LAEEC) (1–3). However, elderly patients often struggle to tolerate CCRT due to their poor performance status. Studies have demonstrated that only about one-third of elderly patients can complete concurrent chemoradiotherapy, which fails to improve their survival compared to radiotherapy alone, resulting in a median overall survival (OS) of less than 2 years (4–8). Thus, there is a pressing need to explore new therapeutic strategies for elderly patients with unresectable esophageal cancer.
Targeted therapy has emerged as a low-toxicity, high-efficiency antitumor systemic therapy (9–12). Epidermal growth factor receptor (EGFR) tyrosine kinase inhibitors (TKIs) represent the most common targeted drugs and play a crucial role in treating non-small cell lung cancer with EGFR gene mutations (10, 13–15). Research has shown that several EGFR-TKIs, including gefitinib and icotinib, exhibit a favorable safety profile in elderly patients, with significantly fewer adverse effects than cytotoxic drugs (16–20). Among these, icotinib has been reported to have lower adverse effects than gefitinib and better suitability for elderly patients or those with poor performance scores (21). Concurrently, EGFR overexpression has been observed in approximately 30-70% of esophageal cancers (22), and EGFR-TKIs have been demonstrated to disrupt cell proliferation and enhance the radiosensitivity of cancer cells (23, 24). This suggests that EGFR-TKIs could be a promising treatment option for EGFR-positive esophageal cancer.
Previous studies have shown that many EGFR TKIs, including erlotinib (23, 25, 26), gefitinib (27), cetuximab (15, 16) and icotinib (28) are effective and safe for patients with esophageal squamous cell carcinoma (ESCC). However, most of these studies focused on the combination of EGFR-TKIs with chemotherapy in metastatic esophageal cancer patients, with limited research on LAEEC. Therefore, we designed this study to investigate the efficacy of icotinib and establish a prediction model of icotinib for EGFR-positive LAEEC population, aiming to provide valuable insights into potential treatment strategies for this population.
2 Methods
2.1 Patients
A total of 80 LAEEC patients who received primary treatment at our hospital between 2014 and 2018 were enrolled in this study. All patients underwent radical radiation therapy with a dose of 60Gy/30F (2), and 41 of them received icotinib. Inclusion criteria were as follows: 1) pathologically diagnosed esophageal squamous carcinoma with immunohistochemical (IHC) confirmed overexpression of EGFR, 2) age >= 70 years, 3) limited stage (stage II-III, AJCC 7th) (29), 4) intolerance to surgery or refusal of surgery, and 5) complete treatment and have complete clinical pathology data. The flow chart is displayed in Figure 1.
This study was approved by the review committee of the Affiliated Huaian No.1 People’s Hospital of Nanjing Medical University. Informed consent was waived as it was a retrospective study.
2.2 Follow-up
Overall survival was defined as the time from initial treatment to the end of follow-up or death. All patients were followed up until 2022-08-01, with a median follow-up duration of 1.9 years.
2.3 Treatment procedures
All patients received radical radiation therapy (RT) using 6Mv X-rays through 3D conformal radiation therapy or intensity-modulated radiation therapy. Gross tumor volume (GTV) comprised the primary tumor and any metastatic regional lymph nodes. Clinical target volume (CTV) included total tumor volume, 3-5 cm longitudinal extensions along the esophagus, 0.5-1 cm horizontally extension, and regional lymph nodes. PGTV and PTV were defined as 0.5-1 cm outward expansion of GTV and CTV, receiving a dose of 60 Gy/30F/6W and 40-50Gy/20-25F/4-5W, respectively. Icotinib was administered at 125 mg, orally, three times daily, concurrently with RT.
2.4 Statistical analysis
Statistical analyses were conducted using R 4.2.1 (30) (R Foundation for Statistical Computing, Vienna, Austria). Age and tumor length were grouped by optimal cut-off point calculated by the “survminer” package (31). In this study, a total of 60 patients were confirmed dead. As OS was the positive event, according to the principle of 10 events per variable (10 EPV), the number of variables included in the final model should not exceed six. Categorical variables were expressed as numbers (percentages), and continuous data were expressed as mean ± standard deviation (SD). Differences between groups were compared using the chi-square test or Fisher’s exact test for categorical variables and the ANOVA test for continuous variables. Univariable and multivariable Cox analyses were employed to select the prognostic factors and develop a predictive model. Bidirectional forward and backward stepwise regression was utilized to optimize the model. A nomogram and a prognostic score (PS) were established based on the optimized model. The time-dependent ROC curve, calibration curve and decision curve were plotted by the “timeROC”, “riskRegression”, and “dcurves” packages (32–34) to evaluate the model’s performance. The “riskRegression” package (33) was employed to calculate the 1000-time average AUC, plot the time-dependent AUC curve and the calibration curve to verify the model’s robustness via bootstrap resampling and the internal cross-validation method outside the bag (OOB). The “survminer” package (31) was used to select the cut-off values, divide the patients into high-risk and low-risk groups, and compare the survival differences between the two groups with log-rank test. Finally, The survival benefit of icotinib in various subgroup populations was analyzed with log-rank test to identify the beneficiary population of icotinib. Univariable analysis was considered statistically significant at p < 0.1, and other analyses were considered statistically significant at p < 0.05.
3 Results
3.1 Patient characteristics
A total of 80 patients were included in this study, and their baseline characteristics are presented in Table 1. Nine factors were considered, including icotinib treatment, gender, age (≤78 years vs. >78 years), tumor length (<5 cm vs. ≥5 cm), tumor location (upper vs. middle vs. lower), clinical stage (II vs. III, AJCC 7th), EGFR expression ((+, Low) vs. (++~+++, High)) (Figure S1)), weight loss of more than 5% within 6 months, and ECOG score. Patients were divided into two groups based on icotinib treatment. No significant differences in clinical characteristics were observed between the two groups, except for EGFR expression.
3.2 Univariable and multivariable analysis
Univariable and multivariable analysis results are displayed in Table 2. Univariable cox analysis identified icotinib treatment (p < 0.001), clinical stage (p = 0.031), EGFR expression (p = 0.038), and ECOG score (p = 0.006) as potential factors affecting overall survival (OS). A multivariable Cox analysis was performed using these four factors (model 1) and optimized with stepwise regression (model 2). The finally results demonstrated that clinical stage (III vs II, HR 1.72, 95% CI 1.02~2.90), icotinib treatment (Y vs N, HR 0.29, 95% CI 0.16~0.52), and ECOG score (2 vs 1, HR 2.02, 95% CI 1.18~3.46) were independent prognostic factors for OS.
3.3 Development and validation of the nomogram
A nomogram based on model 2 was created to predict OS in LAEEC patients (Figure 2), and a prognostic score (PS) was established using the following formula: 0.545 * stage (III) - 1.252 * drug (Y) + 0.703 * ecog (2). The time-dependent ROC curves (Figure 3A) revealed the AUCs of 0.852 (95% CI 0.762 - 0.942), 0.827 (95% CI 0.728 – 0.926), and 0.792 (95% CI 0.663 – 0.921) for 1-year, 2-year, and 3-year OS, respectively. Calibration curve plots (Figure 3B) indicated that the predicted survival probability closely matched the actual survival probabilities at 1, 2, and 3 years, suggesting good predictive accuracy. Time dependent AUC (Figure 3C) and calibration curves (Figure 3D) were obtained using the bootstrap internal cross-validation method with the “riskRegression” package. The AUC exceeded 0.75 throughout the study period, and the calibration curve closely aligned with the standard line, confirming the model’s robust predictive performance and stability. The decision curve (Figure 4) demonstrated that the model provided a better net clinical benefit than reference curves for threshold probabilities from 0.2 to 0.8.
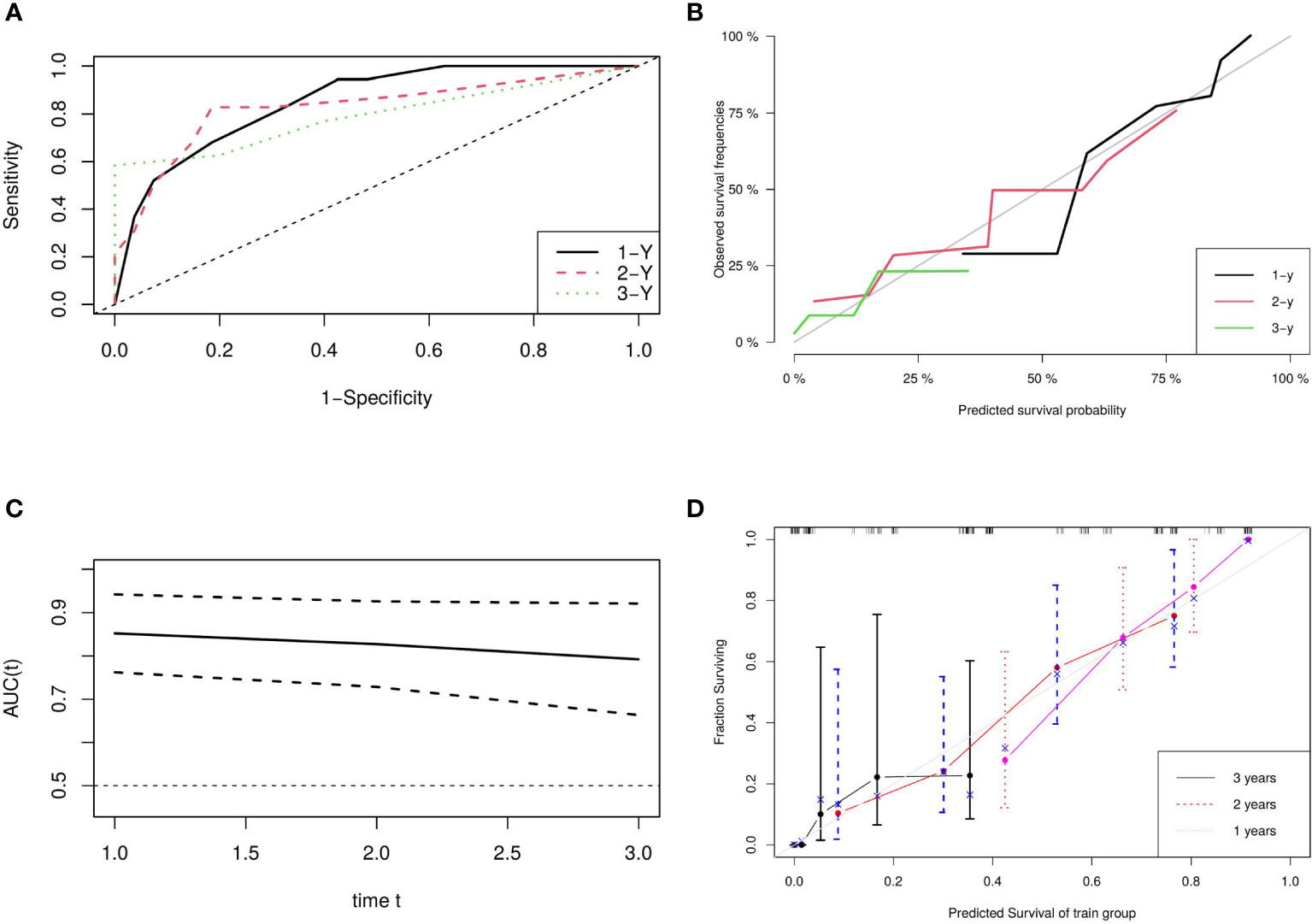
Figure 3 Model Evaluation and Validation: (A) ROC Plots for Predictive Score, (B) Calibration Curves for Predictive Score, (C) Time-Dependent AUC, and (D) Bootstrap Cross-Validation Calibration Curve (1,000 Resamples).
3.4 Risk stratification based on the nomogram
Patients were divided into high- and low-risk groups based on PS, using a cut-off point of -0.549 by the “survminer” package. The risk of death was significantly lower in the low-risk group compared to the high-risk group (HR 0.24, 95% CI 0.13-0.45, P<0.001) (Figure 5).
3.5 Subgroup survival analyses
Subgroup analysis, stratified by prognostic factors such as clinical stage and ECOG score, demonstrated that icotinib significantly improved OS only in the clinical stage III group with a good ECOG score (HR 0.122, 95% CI 0.0248 ~ 0.597, P<0.001). However, no significant improvement in OS was observed in other subgroups (Figure 6).
4 Discussion
Icotinib, an oral EGFR-TKI, modulates cell survival and proliferation by inhibiting the phosphorylation of tyrosine kinase in downstream signaling proteins, obstructing the activation of intracellular signaling pathways, and ultimately regulating the transcription of target genes (35). With EGFR being widely expressed in various solid cancers, icotinib is essential in treating numerous solid malignancies (15, 36–40). Both the EVIDENCE (38) and CONVINCE (41) studies demonstrated that icotinib considerably reduced DFS and serious adverse effects compared to chemotherapy for patients with EGFR-mutated lung adenocarcinoma. The ICAPE study (36) confirmed icotinib’s safety and efficacy in treating EGFR mutant lung adenocarcinoma, regardless of the EGFR mutation type. Zhang et al. (42) found that icotinib enhanced the radiation sensitivity of lung cancer cells by inhibiting the activation of MAPK/ERK/AKT pathway. Zhao et al. (37) indicated that icotinib, combined with concurrent radiotherapy, was safe and effective in the treatment of locally advanced squamous cervical cancer. Wang et al. (28) reported the favorable efficacy and safety of icotinib as a standalone treatment for EGFR-mutated esophageal cancer, suggesting that icotinib could be a viable treatment for elderly patients (43). Our previous study (44) also showed that icotinib combined with radiation therapy was safe and effective for older patients with esophageal cancer, particularly those with EGFR overexpression. Consequently, we designed this study to analyze the impact of icotinib combined with radiotherapy on the prognosis of EGFR-positive LAEEC patients, and to establish and validate a predictive model for clinical decision-making.
In this study, we developed a three-factor proportional risk model, including stage, ECOG, and drug, using cox analysis. The results revealed that the HR of OS in stage III patients were 72% higher than in stage II (HR 1.72, 95% CI 1.02~2.90), and the HR of OS in patients with pool performance status (ECOG 2) was higher than in those with good performance status (ECOG 1) (HR 2.02, 95% CI 1.18~3.46). Icotinib reduced the OS risk by 71% (HR 0.29, 95% CI 0.16~0.52). We then established a predictive nomogram based on the model. The AUCs of the model were 0.852, 0.827, and 0.792 at 1, 2, and 3 years, respectively. Calibration curves demonstrated high agreement between predicted and actual probabilities, and the decision curve displayed a net benefit in the range of 20%-80%. Bootstrap cross-validation by 1000-time resampling indicated that the time-dependent AUC consistently exceeded 0.75, and the calibration curve was close to the diagonal, suggesting that the model had good stability. The survival analysis based on risk stratification by PS showed that the risk of death in the low-risk group was significantly lower than that in the high-risk group (HR 0.243, P<0.001), indicating that the model could effectively identify the death risk of EGFR-position LAEEC patients. Lastly, subgroup analysis revealed that icotinib could significantly reduce the death risk in the clinical stage III, ECOG 1 population. These findings would aid clinicians in assessing the prognosis of elderly patients with EGFR positive and provide a recommendation for icotinib use.
This study, however, had some limitations. First, the cases were collected from 2014 to 2018, during which genetic sequencing technology was not widely available. As a result, EGFR expression in our study was tested using IHC, and the expression of the EGFR resistance gene was not considered. This might also explain why EGFR expression did not emerge as an independent prognostic factor. In subsequent studies, We will introduce the expression of EGFR genes and EGFR resistance genes to optimize our model. Second, this study was a single-institution retrospective study with a small sample size. Although we used bootstrap cross-validation and internal resampling method, selection bias was still inevitable. Therefore, more rigorous prospective studies were needed to validate our conclusions. Overall, our findings contribute to the current understanding of the role of icotinib in treating EGFR-positive LAEEC patients and offer valuable insights for clinical decision-making.
5 Conclusions
In conclusion, stage, ECOG score and icotinib have been identified as independent prognostic factors of overall survival in EGFR-positive LAEEC patients. A nomogram has been developed, which demonstrates good performance in predicting patient outcomes. Notably, icotinib has proven to be beneficial for individuals in clinical stage III with favorable ECOG scores. These findings contribute to the development of more effective treatment strategies for EGFR-positive LAEEC patients, ultimately enhancing patient outcomes and overall survival rates.
Data availability statement
The raw data supporting the conclusions of this article will be made available by the authors, without undue reservation.
Ethics statement
The studies involving human participants were reviewed and approved by The Affiliated Huaian No. 1 People’s Hospital of Nanjing Medical University. Written informed consent for participation was not required for this study in accordance with the national legislation and the institutional requirements.
Author contributions
Conceptualization, JW, HL and YS. Data curation, JW, JP, HL and YS. Formal analysis, JW, JP and YS. Investigation, YS. Methodology, JW, HL and YS. Resources, JW. Validation, YS. Visualization, YS. Writing – original draft, JW and JP. Writing – review & editing, JW, HL and YS. All authors contributed to the article and approved the submitted version.
Conflict of interest
The authors declare that the research was conducted in the absence of any commercial or financial relationships that could be construed as a potential conflict of interest.
Publisher’s note
All claims expressed in this article are solely those of the authors and do not necessarily represent those of their affiliated organizations, or those of the publisher, the editors and the reviewers. Any product that may be evaluated in this article, or claim that may be made by its manufacturer, is not guaranteed or endorsed by the publisher.
Supplementary material
The Supplementary Material for this article can be found online at: https://www.frontiersin.org/articles/10.3389/fonc.2023.1097907/full#supplementary-material
Supplementary Figure 1 | Immunohistochemical images of EGFR expression: (A) EGFR expression (+, low), (B) EGFR expression (++~+++, high).
References
1. Ajani JA, D'Amico TA, Bentrem DJ, Cooke D, Corvera C, Das P, et al. Esophageal and Esophagogastric Junction Cancers, Version 2.2023, NCCN Clinical Practice Guidelines in Oncology. Journal of the National Comprehensive Cancer Network : JNCCN (2023) 21:393–422. doi: 10.6004/jnccn.2023.0019
2. Gong H, Li B. Guidelines for radiotherapy of esophageal carcinoma (2020 edition). Precis Radiat Oncol (2021) 5:54–72. doi: 10.1002/pro6.1119
3. Cowzer D, Janjigian YY. Top advances in esophageal/gastroesophageal junction cancers in 2021. Cancer (2022) 128:1894–1899. doi: 10.1002/cncr.34140
4. Song T, Zhang X, Fang M, Wu S. Concurrent chemoradiotherapy using paclitaxel plus cisplatin in the treatment of elderly patients with esophageal cancer. Onco Targets Ther (2015) 8:3087–94. doi: 10.2147/OTT.S92537
5. Takeuchi S, Ohtsu A, Doi T, Kojima T, Minashi K, Mera K, et al. A retrospective study of definitive chemoradiotherapy for elderly patients with esophageal cancer. Am J Clin Oncol (2007) 30:607–11. doi: 10.1097/COC.0b013e3180ca7c84
6. Tougeron D, Di Fiore F, Thureau S, Berbera N, Iwanicki-Caron I, Hamidou H, et al. Safety and outcome of definitive chemoradiotherapy in elderly patients with oesophageal cancer. Br J Cancer (2008) 99:1586–92. doi: 10.1038/sj.bjc.6604749
7. Wakui R, Yamashita H, Okuma K, Kobayashi S, Shiraishi K, Terahara A, et al. Esophageal cancer: definitive chemoradiotherapy for elderly patients. Dis Esophagus (2010) 23:572–9. doi: 10.1111/j.1442-2050.2010.01062.x
8. Zhang P, Xi M, Zhao L, Shen J-X, Li Q-Q, He L-R, et al. Is there a benefit in receiving concurrent chemoradiotherapy for elderly patients with inoperable thoracic esophageal squamous cell carcinoma? PloS One (2014) 9:e105270. doi: 10.1371/journal.pone.0105270
9. Li Q, Tie Y, Alu A, Ma X, Shi H. Targeted therapy for head and neck cancer: signaling pathways and clinical studies. Signal Transduct Target Ther (2023) 8:31. doi: 10.1038/s41392-022-01297-0
10. Majeed U, Manochakian R, Zhao Y, Lou Y. Targeted therapy in advanced non-small cell lung cancer: current advances and future trends. J Hematol OncolJ Hematol Oncol (2021) 14:108. doi: 10.1186/s13045-021-01121-2
11. Yang Y-M, Hong P, Xu WW, He Q-Y, Li B. Advances in targeted therapy for esophageal cancer. Signal Transduct Target Ther (2020) 5:229. doi: 10.1038/s41392-020-00323-3
12. Xie Y-H, Chen Y-X, Fang J-Y. Comprehensive review of targeted therapy for colorectal cancer. Signal Transduct Target Ther (2020) 5:22. doi: 10.1038/s41392-020-0116-z
13. Król K, Mazur A, Stachyra-Strawa P, Grzybowska-Szatkowska L. Non-small cell lung cancer treatment with molecularly targeted therapy and concurrent radiotherapy-a review. Int J Mol Sci (2023) 24:5858. doi: 10.3390/ijms24065858
14. Gridelli C, Massarelli E, Maione P, Rossi A, Herbst RS, Onn A, et al. Potential role of molecularly targeted therapy in the management of advanced nonsmall cell lung carcinoma in the elderly. Cancer (2004) 101:1733–44. doi: 10.1002/cncr.20572
15. Yuan Z, Yu X, Wu S, Wu X, Wang Q, Cheng W, et al. Instability mechanism of osimertinib in plasma and a solving strategy in the pharmacokinetics study. Front Pharmacol (2022) 13:928983. doi: 10.3389/fphar.2022.928983
16. Chihara Y, Takeda T, Goto Y, Nakamura Y, Tsuchiya-Kawano Y, Nakao A, et al. A phase II trial on osimertinib as a first-line treatment for EGFR mutation-positive advanced NSCLC in elderly patients: the SPIRAL-0 study. Oncol (2022) 27:903–e834. doi: 10.1093/oncolo/oyac193
17. Yamada Y, Imai H, Sugiyama T, Minemura H, Kanazawa K, Kasai T, et al. Effectiveness and safety of EGFR-TKI rechallenge treatment in elderly patients with advanced non-Small-Cell lung cancer harboring drug-sensitive EGFR mutations. Med Kaunas Lith (2021) 57:929. doi: 10.3390/medicina57090929
18. Roviello G, Zanotti L, Cappelletti MR, Gobbi A, Dester M, Paganini G, et al. Are EGFR tyrosine kinase inhibitors effective in elderly patients with EGFR-mutated non-small cell lung cancer? Clin Exp Med (2018) 18:15–20. doi: 10.1007/s10238-017-0460-7
19. Daste A, Chakiba C, Domblides C, Gross-Goupil M, Quivy A, Ravaud A, et al. Targeted therapy and elderly people: a review. Eur J Cancer Oxf Engl 1990 (2016) 69:199–215. doi: 10.1016/j.ejca.2016.10.005
20. Kuwako T, Imai H, Masuda T, Miura Y, Seki K, Yoshino R, et al. First-line gefitinib treatment in elderly patients (aged ≥75 years) with non-small cell lung cancer harboring EGFR mutations. Cancer Chemother Pharmacol (2015) 76:761–9. doi: 10.1007/s00280-015-2841-5
21. Shi Y, Zhang L, Liu X, Zhou C, Zhang L, Zhang S, et al. Icotinib versus gefitinib in previously treated advanced non-small-cell lung cancer (ICOGEN): a randomised, double-blind phase 3 non-inferiority trial. Lancet Oncol (2013) 14:953–61. doi: 10.1016/S1470-2045(13)70355-3
22. Pande AU, Iyer RV, Rani A, Maddipatla S, Yang GY, Nwogu CE, et al. Epidermal growth factor receptor-directed therapy in esophageal cancer. Oncology (2007) 73:281–9. doi: 10.1159/000132393
23. Chinnaiyan P, Huang S, Vallabhaneni G, Armstrong E, Varambally S, Tomlins SA, et al. Mechanisms of enhanced radiation response following epidermal growth factor receptor signaling inhibition by erlotinib (Tarceva). Cancer Res (2005) 65:3328–35. doi: 10.1158/0008-5472.CAN-04-3547
24. Tortora G, Gelardi T, Ciardiello F, Bianco R. The rationale for the combination of selective EGFR inhibitors with cytotoxic drugs and radiotherapy. Int J Biol Markers (2007) 22:47–52. doi: 10.1177/17246008070221s406
25. Zhai Y, Hui Z, Wang J, Zou S, Liang J, Wang X, et al. Concurrent erlotinib and radiotherapy for chemoradiotherapy-intolerant esophageal squamous cell carcinoma patients: results of a pilot study. Dis Esophagus Off J Int Soc Dis Esophagus (2013) 26:503–9. doi: 10.1111/j.1442-2050.2012.01380.x
26. Xie C, Jing Z, Luo H, Jiang W, Ma L, Hu W, et al. Chemoradiotherapy with extended nodal irradiation and/or erlotinib in locally advanced oesophageal squamous cell cancer: long-term update of a randomised phase 3 trial. Br J Cancer (2020) 123:1616–24. doi: 10.1038/s41416-020-01054-6
27. Petty RD, Dahle-Smith A, Stevenson DAJ, Osborne A, Massie D, Clark C, et al. Gefitinib and EGFR gene copy number aberrations in esophageal cancer. J Clin Oncol Off J Am Soc Clin Oncol (2017) 35:2279–87. doi: 10.1200/JCO.2016.70.3934
28. Wang X, Niu H, Fan Q, Lu P, Ma C, Liu W, et al. Predictive value of EGFR overexpression and gene amplification on icotinib efficacy in patients with advanced esophageal squamous cell carcinoma. Oncotarget (2016) 7:24744–51. doi: 10.18632/oncotarget.8271
29. Rice TW, Blackstone EH, Rusch VW. 7th edition of the AJCC cancer staging manual: esophagus and esophagogastric junction. Ann Surg Oncol (2010) 17:1721–4. doi: 10.1245/s10434-010-1024-1
30. R Core Team. R: A language and environment for statistical computing. R Foundation for Statistical Computing, Vienna, Austria (2022). Available at: https://www.R-project.org/.
31. Kassambara A, Kosinski M, Biecek P. Survminer: drawing survival curves using “ggplot2.” (2021). Available at: https://rpkgs.datanovia.com/survminer/index.html.
32. Blanche P, Dartigues J-F, Jacqmin-Gadda H. Estimating and comparing time-dependent areas under receiver operating characteristic curves for censored event times with competing risks. Stat Med (2013) 32:5381–97. doi: 10.1002/sim.5958
33. Gerds TA, Kattan MW. Medical Risk Prediction Models: With Ties to Machine Learning. (1st ed). Chapman and Hall/CRC (2021). doi: 10.1201/9781138384484
34. Sjoberg DD. dcurves: Decision Curve Analysis for Model Evaluation. (2022). Available at: https://github.com/ddsjoberg/dcurves, https://www.danieldsjoberg.com/dcurves/
35. KEGG PATHWAY: ErbB signaling pathway - reference pathway. Available at: https://www.genome.jp/kegg-bin/show_pathway?map04012 (Accessed November 26, 2022).
36. Qian K, Chen Q-R, He M, Wang Z-T, Liu Y, Liang H-G, et al. Icotinib, an EGFR tyrosine kinase inhibitor, as adjuvant therapy for patients with stage IIA-IIIA EGFR-mutant non-small-cell lung adenocarcinoma: a multicenter, open-label, single-arm, phase II study (ICAPE). Invest New Drugs (2022) 41:44–52. doi: 10.1007/s10637-022-01316-5
37. Liu Y-M, Liu H, Sun H-L, Xu Y-Y, Ding Y, Chen W-J, et al. Outcomes of icotinib combined with concurrent chemoradiotherapy in locally advanced cervical cancer. Neoplasma (2021) 68:645–51. doi: 10.4149/neo_2021_201122N1264
38. He J, Su C, Liang W, Xu S, Wu L, Fu X, et al. Icotinib versus chemotherapy as adjuvant treatment for stage II-IIIA EGFR-mutant non-small-cell lung cancer (EVIDENCE): a randomised, open-label, phase 3 trial. Lancet Respir Med (2021) 9:1021–9. doi: 10.1016/S2213-2600(21)00134-X
39. Zhu L, Zhu C, Wang X, Liu H, Zhu Y, Sun X. The combination of icotinib hydrochloride and fluzoparib enhances the radiosensitivity of biliary tract cancer cells. Cancer Manag Res (2020) 12:11833–44. doi: 10.2147/CMAR.S265327
40. Zhao Q, Cheng J, Chen P, Sun J, Guan S. Icotinib: efficacy in different solid tumors and gene mutations. Anticancer Drugs (2020) 31:205–10. doi: 10.1097/CAD.0000000000000861
41. Shi YK, Wang L, Han BH, Li W, Yu P, Liu YP, et al. First-line icotinib versus cisplatin/pemetrexed plus pemetrexed maintenance therapy for patients with advanced EGFR mutation-positive lung adenocarcinoma (CONVINCE): a phase 3, open-label, randomized study. Ann Oncol Off J Eur Soc Med Oncol (2017) 28:2443–50. doi: 10.1093/annonc/mdx359
42. Zhang S, Fu Y, Wang D, Wang J. Icotinib enhances lung cancer cell radiosensitivity in vitro and in vivo by inhibiting MAPK/ERK and AKT activation. Clin Exp Pharmacol Physiol (2018) 45:969–977. doi: 10.1111/1440-1681.12966
43. Tan F, Shen X, Wang D, Xie G, Zhang X, Ding L, et al. Icotinib (BPI-2009H), a novel EGFR tyrosine kinase inhibitor, displays potent efficacy in preclinical studies. Lung Cancer Amst Neth (2012) 76:177–82. doi: 10.1016/j.lungcan.2011.10.023
Keywords: esophageal cancer, unresectable, nomogram, icotinib, stage, ECOG score
Citation: Wang J, Peng J, Luo H and Song Y (2023) Development and internal validation of a nomogram for predicting survival of nonoperative EGFR-positive locally advanced elderly esophageal cancers. Front. Oncol. 13:1097907. doi: 10.3389/fonc.2023.1097907
Received: 14 November 2022; Accepted: 28 April 2023;
Published: 12 May 2023.
Edited by:
Kui Zhang, The University of Chicago, United StatesReviewed by:
Han-Xiang An, Xiamen University, ChinaFang Wang, Yale University, United States
Zheng Yuan, China Academy of Chinese Medical Sciences, China
Copyright © 2023 Wang, Peng, Luo and Song. This is an open-access article distributed under the terms of the Creative Commons Attribution License (CC BY). The use, distribution or reproduction in other forums is permitted, provided the original author(s) and the copyright owner(s) are credited and that the original publication in this journal is cited, in accordance with accepted academic practice. No use, distribution or reproduction is permitted which does not comply with these terms.
*Correspondence: Yaqi Song, c29uZ2FxaUAxNjMuY29t; Honglei Luo, bGhsaGF5eUAxNjMuY29t