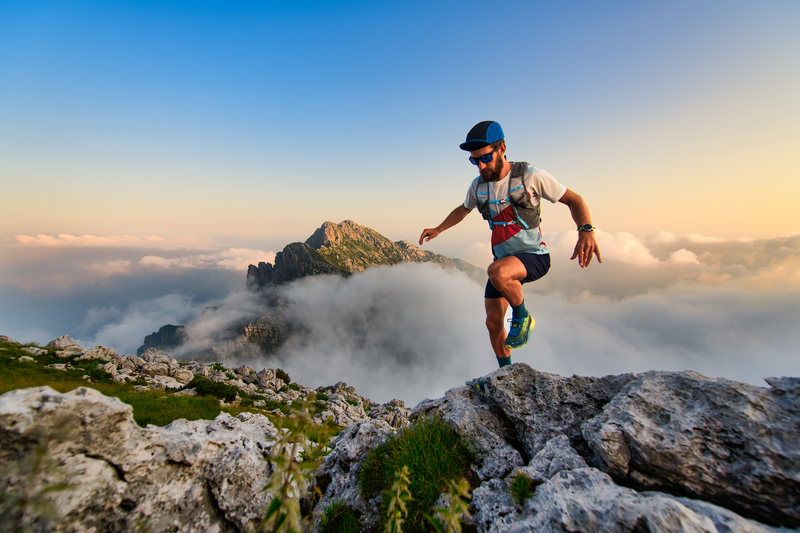
94% of researchers rate our articles as excellent or good
Learn more about the work of our research integrity team to safeguard the quality of each article we publish.
Find out more
REVIEW article
Front. Oncol. , 25 January 2023
Sec. Surgical Oncology
Volume 13 - 2023 | https://doi.org/10.3389/fonc.2023.1065402
This article is part of the Research Topic Artificial Intelligence in Colorectal Cancers View all 7 articles
Artificial Intelligence (AI) is a branch of computer science that utilizes optimization, probabilistic and statistical approaches to analyze and make predictions based on a vast amount of data. In recent years, AI has revolutionized the field of oncology and spearheaded novel approaches in the management of various cancers, including colorectal cancer (CRC). Notably, the applications of AI to diagnose, prognosticate, and predict response to therapy in CRC, is gaining traction and proving to be promising. There have also been several advancements in AI technologies to help predict metastases in CRC and in Computer-Aided Detection (CAD) Systems to improve miss rates for colorectal neoplasia. This article provides a comprehensive review of the role of AI in predicting risk, prognosis, and response to therapies among patients with CRC.
Colorectal Cancer (CRC) is the third most common cancer worldwide in men and the second most common cancer in women with over 1.93 million new diagnoses in 2020 (1). CRC is also the second leading cause of cancer death worldwide with an estimated 935,173 deaths in 2020 (1). Roughly 50% of patients with CRC will develop liver metastasis throughout the course of their disease (2). Projections for 2040 estimate an increase in the global incidence of CRC with an estimated 3.2 million cases (3). In this era of personalized medicine, increased efforts are needed for more effective diagnosis, risk prediction, prognostication, and prediction of treatment response.
Artificial intelligence (AI) is a novel branch of computer science that involves the ability of computer systems to emulate the human behavior that requires intelligence, like thinking and decision-making. AI was born in 1956 through the work of the Darmouth AI conference and has since revolutionized various industries, including medicine (4). Through the development of AI, came various systems such as Machine learning (ML), which is a subset of AI that involves a range of statistical, probabilistic, and optimization models that allows a computer system to learn and adapt from previous iterations to analyze and make inferences from patterns in noise (5, 6). Deep learning (DL) is a subset of ML that employs neural networks with multiple layers of processing to analyze and learn in a similar fashion to humans (7).
One of the exciting applications of AI has been in the field of radiology, which involves the use of imaging to diagnose and treat illnesses, and especially radiomics. Radiomics is a process that refers to the extraction of mineable data from medical images (8). Its importance is based on the fact that a vast number of extracted features, like entropy patterns, skewness, and kurtosis are mostly hidden to the human eye and can inform clinical decision making, quantify tumor phenotype, predict response to treatment, and determine prognosis. AI is not only able to effectively analyze the features generated by radiomics but also analyze the images to create its own radiomic features to forego predefined features, which is conventionally known as deep radiomics (9, 10). Given that radiology intersects with several fields of medicine, the advancements of AI in radiology has influenced several types of medical fields like oncology, including CRC.
The management of patients with CRC is multifaceted and involves various dimensions of care, such as screening, diagnosis, treatment, and follow-up. Because of these multiple components of care and the vast capabilities of AI, these technologies have an incredibly important role in the management of CRC, anywhere from polyp detection to the prediction of response to chemoradiotherapy. Various applications are currently being developed and validated to improve the detection and workup of CRC treatment. Figure 1 shows an overview of the various features considered in these AI models and some of the applications specific to CRC. Table 1 prevents a summary of the major studies of AI in CRC. In this article, we explore the role of artificial intelligence-based technique in risk prediction, prognostication and therapy response assessment in colorectal metastasis.
Figure 1 An overview of the applications of artificial intelligence in colorectal cancers. (Top) Input features from multimodal clinical datasets are integrated into artificial intelligence algorithms (center) that provide key information on the prognostication (left), diagnostics (bottom) and therapeutics (right) of colorectal cancer. EHR, electronic health record; ANN, artificial neural network; LR, logistic regression; SVM, support vector machine; RF, random forest; CNN, convolutional neural network; GAN, generative adversarial network; DNN, deep neural network. Created with BioRender.com.
The prognosis of CRC appears to be substantially improved when detected in its early stages (18). Screening can reduce the mortality and incidence associated with CRC by facilitating early diagnosis and treatment (27). In fact, colonoscopy may lead to a 90% risk reduction for CRC by allowing practitioners to identify suspicious lesions in the walls of the intestine (28, 29). This is particularly important as most adenomatous polyps are asymptomatic (29). However, although colonoscopy is the gold standard for diagnosing neoplastic lesions, it is accompanied by limitations including adenoma miss rates (AMR) ranging from 6% to 28% (18, 30). In fact, more than half of CRC cases which occurred post-colonoscopy arise from lesions that were missed during prior colonoscopies (31).
The ability to detect lesions during colonoscopy can vary significantly based on several factors, including operator performance (29). In addition, polyps which are flat, depressed, or have a diameter smaller than 10 mm may be harder to detect as colonoscopy is dependent on clinical experience and relies on the practitioner’s ability to visually identify the lesion, which is a complex and error-prone task (18, 27, 32). Other factors such as the size of the tumor, its patterns of enhancement, and variability in clinical symptoms can further add to the complexity of making diagnostic decisions (28, 33).
AI algorithms have been shown to augment the accuracy of existing endoscopic screening procedures. For example, computer-aided detection (CADe) can be used during colonoscopy to increase adenoma detection rates by highlighting abnormal areas of an image (18, 32). One systematic review and meta-analysis consisting of five randomized clinical trials (RCTs) found that adenoma detection rates (36.6% vs. 25.2%, P < 0.01) and overall polyp detection rate (50.3% vs. 34.6%, P < 0.01) were significantly higher in the CADe group compared to the control group (11). In another meta-analysis of 10 RCT, adenoma detection rates (35.4% vs. 24.9%, P < 0.001) and polyp detection rates (48.6% vs 33.8%, P < 0.001) were significantly higher when compared with routine colonoscopy (12).
During colonoscopy, computer-aided diagnosis (CADx) algorithms can be used to characterize the region based on the probability of its malignancy (18, 32). Kambe et al. conducted a randomized control trial to investigate whether a CADe model could reduce AMR during colonoscopy (34). Compared to the standard colonoscopy group which had an AMR of 36.7%, the patients who received CADe-assisted colonoscopy had a significantly lower AMR of 13.8% (P < 0.01). Brown et al. investigated AMR, sessile-serrated lesion (SSL) miss-rate and adenomas per colonoscopy (APC) in patients who underwent high-definition white light colonoscopy (HDWL) versus colonoscopy assisted with CADe (35). This randomized control trial found significant lower AMR (20.1% vs. 31.3%, P = 0.025), lower SSL miss-rate (7.1% vs. 42.1%, P = 0.048), and higher first-pass APC (1.19 vs. 0.90, P = 0.03) in the CADe group compared to the HDWL group. Interestingly, another randomized control trial found that CADe-assisted colonoscopy significantly increased adenoma detection rate compared to the control group (53.3% vs. 44.5%, P < 0.01) (36). Other programs such as supervised learning (SL) algorithms can predict clinical outcomes after being trained using pre-labeled endoscopic images (18). In addition, various algorithms using convolutional neural networks have also been used for tumor identification, and the emergence of AI-based computed tomography (CT) colonography has further enabled the detection of flat neoplasms with greater accuracy (27).
Many polyps are missed during current practice, and they may present as precursors to CRC (37). Deep learning (DL) algorithms can enhance adenoma detection due to its ability to efficiently detect premalignant polyps (18). Such detection begins with adequate bowel preparation before colonoscopy, which is a pertinent step for the visualization of premalignant polyps. A recent study found that a novel AI based CNN model have a higher Boston bowel preparation scale and, therefore, higher quality of bowel preparation for detecting polyps compared to current routine practice (38). Polyp detection and localization systems that operate in real time are extremely useful in clinical practice and as of 2022 there has been 20 out of 43 studies with the ability to operate as such (39). Wang et al. were the first to perform a prospective research trial with polyp detection pipelines and had a frame-based evaluation (F1) of 0.91 (40). Recently a large database composed of 28,576 images were modeled into a DL pre-trained YOLOv3 (You Only Look Once) network for real time adenoma detection. The pipeline had an (F1 ) of 0.88 and a sensitivity reaching 90% for detecting and localizing polyps. Additionally Li K. et al. created a large CNN model for both polyp detection and classification, and had a sensitivity reaching 91% and 70%, respectively (41). Ozawa et al. used over 27,000 endoscopic images to design a CNN algorithm that can detect and classify polyps with a detection sensitivity of 0.92 and a classification sensitivity of 0.83 (42). Another CNN algorithm designed by Akbari et al. can perform polyp segmentation and produce an accurate probability map with a 99.3% sensitivity (43). A faster region-based CNN (Faster R-CNN) combined with a single shot multibox detector (SSD) was also used to detect polyps with a precision 0.8154 (44). Similarly, Godkhindi et al. used 825 CT images to develop a CNN algorithm for polyp detection (45). The resulting model could detect polyps with an accuracy of 0.87. In addition, RetinaNet can automatically localize polyps with a precision of 0.537 using a CNN-based algorithm (46). Moreover, as shown in the Medical Image Computer and Computer Assisted Intervention (MICCAI) 2015 Endoscopic Vision Challenge, the methods utilizing AI outperformed those that relied on manually extracted features (47) These AI-based algorithms have proven to be more advantageous than traditional radiomics methods that may be susceptible to errors as they rely on tumor segmentation and subsequent identification of clinically pertinent radiomic features.
That said, its apparent that incorporating AI into routine colonoscopy can decrease adenoma miss rates (AMR) through better detection, identification, and localization of the tumors, especially small ones <10mm, which has achieved a 50% reduction in a recent multicenter, multicounty study (31). This shows that AI-driven colonoscope approaches has important future implications on CRC screening moving toward better risk stratification, prognostication, and improvement of patient outcomes. However, further research is still needed in this area, especially to see if such improvements in adenoma recognition is not as same as a better mucosa exposure for adenoma detection.
Histopathological characterization is often the last step in the diagnosis of CRC and is based on the texture, structure, and morphological features of the tissue (48). Precise tumor classification is vital for prognostication and is particularly important as even experienced pathologists may often disagree on the grading of tissue (49). AI applications may be useful in this step by preventing diagnostic inconsistency and improving inter- and intra-observer variability. One application of a hybrid deep learning (hDL) algorithm was used to distinguish low-grade CRC from high-grade CRC lesions with an accuracy of 99.1% (48). Moreover, a DL algorithm was used to automatically classify tissues without the need for segmentation (49). This model was able to differentiate between normal mucosa, hyperplastic polyps, adenoma, and adenocarcinoma with an accuracy of 80% (49). Another DL algorithm used ResNet to classify colorectal polyps on whole slide images (50). This program was shown to differentiate between hyperplastic polyps, sessile serrated polyps, traditional serrated adenoma, tubular adenoma, and villous adenoma with an accuracy of 93%. One meta-analysis found that AI could improve histology prediction, including those involving diminutive polyps, with a pooled specificity of 89.8% and sensitivity of 92.3% (15). In another systematic review focused on CRC pathology image analysis using artificial intelligence found that while applications were still in early stages, the results were still promising with respect to accurately diagnosing CRC (16). Furthermore, in another systematic review focused on the use of deep learning for the diagnosis of CRC via histopathological images found that various studies have promise in aiding the diagnosis, predicting relevant molecular features, identifying prognostic features with correlations to metastasis, and assessing tumor microenvironments (17).
CNN-based algorithms can also enable precise image classification; in fact, these algorithms have been shown to more accurately classify colorectal tumors when compared to endoscopists (51). Iizuka et al. used a CNN-based algorithm to automatically classify tissues as non-neoplastic, adenoma, or adenocarcinoma (52). Using annotated whole slide images, a CNN program was trained by a max-pooling method and a recurrent neural network (RNN). The resulting program could differentiate the tissues with an AUC of 0.96 for adenocarcinoma and 0.99 for adenoma.
Moreover, SL algorithms can be applied to support vector machines (SVMs) for the grading of unlabeled biopsies. One SVM algorithm was employed by Takemura et al. to discriminate neoplastic from nonneoplastic lesions with a high predictive power and detection accuracy of 97.8% (53). CADx algorithms can also be used to differentiate neoplastic from non-neoplastic polyps. Tamai et al. utilized a CADx algorithm in conjunction with narrow-band imaging (NBI) to accurately classify and differentiate between hyperplastic lesions, adenocarcinomas, and submucosal lesions (54). Similarly, Chen et al. used neural networks (NNET) to detect hyperplastic and neoplastic polyps that are smaller than 5 mm with 96.3% sensitivity (55).
In conclusion, several AI based algorithms has shown promise on accurate histopathologic diagnosis with some showing high performance on pathologic image analysis (56). These new techniques can ease the identification of cancer status in an accurate manner, which can eventually revolutionize the prediction performance in a way that may exceed the accuracy of pathologist analysis.
CRC can also be detected using noninvasive procedures including blood testing. These procedures may be advantageous over endoscopic procedures such as colonoscopy which requires bowel preparation, carries a risk of bowel rupture, and is not indicated in patients with peritoneal irritation and anorectal stenosis (57). Pan et al., evaluated the predictive value of serum glycomic profiling in tandem with AI algorithms to identify advanced colorectal adenomas (57). In this study, the biomarkers of interest were multi-antennary N-glycans and core-fucosylated N-glycans, which were positively and negatively correlated with CRC stage, respectively. Subsequently, an algorithm which utilized random forests, logistic model trees (LMT), and SVM programs was employed to identify those patients who were at an advanced stage of CRC with an accuracy of 75%.
Moreover, Ivancic et al. investigated various serum protein biomarkers to identify those that were indicative of CRC (58). Using machine learning and SVM algorithms, it was shown that five biomarkers were strongly predictive of CRC, including superoxide dismutase 3, leucine-rich alpha-2-glycoprotein 1, inter-alpha- trypsin inhibitor heavy-chain family member 4, hemopexin, and epidermal growth factor receptor, with a specificity of 70% and a sensitivity of 89%. In one study, an AI learning algorithm (ColonFlag™) was used in patients with iron deficiency anemia who underwent a fecal immunochemical test. The study found that the AI learning algorithm may improve the prioritization of urgent referral as it was able to reduce the prioritization of patients from 592, who were referred based on their hemoglobin concentration, to 304 (59). A recent review summarizes various studies that utilize liquid biopsy and AI in CRC in order to detect signatures of colorectal malignancies and aid in stratification (18).
With such advancement in AI-based blood testing to diagnose CRC, it becomes clear that the future holds promise toward fewer procedural screening to accurately diagnose and identify high risk CRC, which can become an efficient alternative method to decrease procedural complications and improve patient flow.
Molecular imaging can be crucial in the practitioners’ ability to make effective decisions regarding the detection, diagnosis, and staging of colorectal tumors, in addition to predicting response to therapy (60). Existing molecular imaging techniques can be improved with the use of AI algorithms. For instance, feature-detection algorithms used in conjunction with magnifying chromoendoscopy has been shown to improve the discernment of neoplastic from non-neoplastic lesions (18). In addition, SVMs can be applied to endocytoscopy procedures to improve the discrimination of between benign lesions and neoplastic carcinomas (18). A systematic review and meta-analysis found that AI could improve the characterization of polyps after detection, with a sensitivity of 92% and accuracy of 87% (19).
AI algorithms can use confocal laser endomicroscopy (CLE) to augment the detection of polyps (61). CLE is a useful imaging technique that can identify subcellular features of the gastrointestinal mucosa due to its high magnifying capabilities (62). One AI model used the k-nearest neighbor algorithm to discriminate malignant lesions from benign lesions with 90% accuracy (63). In more recent developments, Stefanescu et al. used CADx algorithms in conjunction with CLE to more readily identify morphometric and malignant characteristics of the mucosa, which can aid in the diagnosis of CRC (61).
For patients that have a higher risk of complications associated with colonoscopy and those that cannot tolerate sedation, an alternative method of CRC screening is possible, including colon capsule endoscopy (CE) (18, 64). Colon CE is a minimally invasive procedure that allows practitioners to obtain images of the gastrointestinal lumen. This imaging procedure can also be augmented using AI algorithms. For instance, CNN algorithms have been applied to colon CE procedures which has allowed for the improved identification and localization of polyps with a high degree of sensitivity and specificity (28).
With further advancement in AI- based imaging modalities, we anticipate a paradigm shift toward improved image-guided detection of CRC, leading to fewer procedural interventions and complications. This can potentially increase patient convenience and medical cost, while keeping precision medicine intact.
Lymph node metastasis (LNM) is the most common method for the spread of CRC (65). The precise assessment of LNM is vital for making clinical decisions as it can help establish the most effective treatment for CRC (66). However, LNM is cumbersome to predict before surgery, as the current standards for staging such as CT have an accuracy of 56.5% (67). Other techniques such as magnetic imaging resonance (MRI) can also be used to determine nodal staging; however, this also has a low diagnostic accuracy of 63% and a relatively low sensitivity (65, 67). To this end, current research shows that AI programs may be used to facilitate treatment decisions by improving the prediction of LNM. Liu et al. designed a multimodal multiple instance learning (MMIL) algorithm to predict lymph node metastasis (67). The MMIL was designed using a two-pronged approach: first, tumor-specific serum biomarkers (including CEA, CA125, CA19-9, and AFP) were inputted into a feed-forward network. Subsequently, this information was integrated with a feature extraction tool based on annotated whole slide images of the tumor microenvironment. The resulting MMIL model could predict lymph node metastasis with an area-under-the-curve (AUC) of 0.93, 0.88, 0.81, and 0.86 for stages T1, T2, T3, and T4, respectively. One systematic review and meta-analysis found that AI could improve pre-operative staging of lymph nodes and lead to a more accurate prediction of metastasis compared to traditional radiomics models (20).
Another algorithm applied five ML models to predict LNM, including SVM, random forests (RFs), NNET, logistic regression and extreme gradient boosting (66). The latter two models could predict LNM with an AUC of 0.87 and 0.90, respectively. Moreover, both of these algorithms were more effective in predicting LNM when compared to standard 18F-FDG PET/CT (18F-Fluorodeoxy Glucose Positron Emission/Computed Tomography) imaging. Ding et al. utilized a DL algorithm that could target specific features of 414 MRI images to predict metastatic lymph nodes (65). Subsequently, a faster R-CNN model was applied which resulted in LNM prediction with an AUC of 0.91.
In addition, AI algorithms can also be used to determine liver metastasis in CRC, which is a significant contributor to mortality in these patients. Kiritani et al. applied probe electrospray ionization-mass spectrometry in combination with ML to identify malignant spectrum patterns from tissue samples (68). The resulting algorithm was shown to predict liver metastasis with an accuracy of 99.5%.
Such progressive advancement in AI based metastatic detection has the potential to make the staging of CRC easily achievable with high accuracy, leading to a more efficient, personalized, and precise therapeutic approach. These advancements hold promise to the patient and the healthcare system as a whole due to a more efficient diagnostic processes while accurately individualizing patient care.
In conclusion, many AI-based applications holds promise toward the diagnoses of colorectal cancer through effective polyp detection, localization, identification, segmentation, and classification, which can eventually decrease adenoma miss rates and better predict clinical outcomes. The expansion of such approaches to accurate blood and histopathologic diagnoses, along with the use of various image-guided modalities to accurately determine the stage of CRC, has an overall ability to improve diagnostic variability and can revolutionize the current prognostication systems for a precise therapeutic approaches and better patient outcomes.
The carcinogenesis of CRC can be explained by several different molecular pathways, including the chromosomal instability pathway, microsatellite instability pathway, and the CpG island methylation pathway. In particular, microsatellite instability (MSI) is a key biomarker in CRC and is linked to deficient DNA mismatch repair (69). This indicator is very useful when making treatment decisions; for instance, it is used to screen for Lynch syndrome and patients with advanced CRC who present MSI are eligible to receive immune checkpoint blockade therapy (69, 70). MSI status is also useful after surgery when clinicians are selecting adjuvant chemotherapy. Existing tests for MSI status rely on methods that are resource-intensive and not readily available in most treatment facilities, including PCR analysis of microsatellite markers (69).
Several studies have shown that AI algorithms may be useful in detecting MSI. For instance, Echle et al. trained deep neural networks (DNNs) to detect MSI using hematoxylin-eosin (HE) tissue slides (69). The model could successfully identify MSI status with an AUC greater than 0.85 in eight out of the nine cohorts tested. Cao et al. used an integrated AI model—Ensemble Patch Likelihood Aggregation model (EPLA)—to predict MSI status based on whole slide images from the Cancer Genome Atlas (TCGA-COAD) cohort and the Asian CRC cohort (Asian-CRC) (70). An MSI-sensor algorithm assigned MSI status using paired genome sequencing based on MSI-sensor scores greater than or equal to 10. Furthermore, distinct microsatellite loci were analyzed using capillary electrophoresis to classify the cohort into MSI-high and MSI-low groups. A CNN-based algorithm—Resnet-18—was used to generate patch-level prediction of MSI, and the resulting data was inputted into the Patch Likelihood Histogram (PALHI) pipeline and the Bag of Words (BOW) pipeline. The combination of both pipelines using ensemble learning allowed for the prediction of MSI with an AUC of 0.89 in the test cohort.
In addition, AI algorithms can also be used in other genetic applications; for instance, a CNN model was used to predict tumor mutational burden-high (TMB-H) with an AUC of 0.93 (71). Moreover, AI has been shown to detect the presence of the KRAS proto-oncogene which may be implicated in the pathogenesis of CRC (72). In fact, 65% of carcinomas in the colon have been linked to mutations in the RAS family of genes, which includes the KRAS proto-oncogene (73). Identifying whether a patient has a mutated KRAS gene may be crucial as some individuals with this mutation may not be responsive to existing therapies, including anti-EGFR agents. To this end, one study conducted by Gonzalez-Castro et al. utilized several ML algorithms including SVM, Grade Boosting Machines, NNET, and RFs to identify if the KRAS gene is mutated (72). After extracting textural characteristics from CT images, the algorithms were able to classify the images as KRAS positive or negative. It was found that NNET in conjunction with Haralick texture analysis was most efficient, with an accuracy of 83% and sensitivity of 88.9%. In a systematic review focused on the prediction of microsatellite instability based on tumor histomorphology using AI, the algorithms for CRC had great performance with the highest standard of 0.972 (21).
Prognostic predictors are valuable tools for treatment decision-making by helping clinicians choose the most suitable treatment modality for each patient (74, 75). Survival prediction may be particularly valuable in early-stage CRC, as it can help clinicians decide whether adjuvant chemotherapy is suitable or not. Skrede et al. used CNN algorithms to stratify CRC patients based on survival rate to identify those patients who would likely not benefit from adjuvant chemotherapy versus those patients who would require such treatment (76). Similarly, another CNN algorithm developed by Jiang et al. could predict disease recurrence risk and overall survival for stage III CRC using gradient boosting (74). A CNN algorithm was also used to predict survival based on stromal microenvironment data obtained from HE slides (77). The HE slides were categorized into nine distinct classes, including adipose tissue, background, debris, lymphocytes, mucus, smooth muscle, normal colonic mucosa, cancer-associated stroma, and CRC epithelia. Subsequently, the CNN algorithm was trained, and a deep-stroma score was obtained to determine overall survival as the primary endpoint, and disease-specific survival and relapse-free survival as the secondary outpoints. The resulting model was found to predict these endpoints with a nine-class accuracy of 94%. Our group has also shown that MRI-based texture features of intra-tumor heterogeneity were associated with survival outcomes and improved the performance of standard clinicopathological variables in predicting survival in 55 patients stage IV CRC (78). A random forest ML model found an AUC of 0.83 for the standard clinicopathological prognostic variables and 0.94 when imaging-based heterogeneity features were added.
AI algorithms have not only shown to be capable of predicting survival data, but they may also be able to predict remaining lifespan for advanced-stage CRC. Wang et al. used the Surveillance, Epidemiology, and End Results (SEER) database and tree-based classification to predict whether patients will survive in five years, along with their estimated remaining months if they are predicted to die within five years (75). The model was shown to predict survival with an accuracy of 0.71 and a sensitivity of 0.85. Al-bahrani et al. also used the SEER database and DNN for survival prognostication of CRC (79). The DNN algorithm was trained using patient characteristics including tumor size, age at diagnosis, reason for no surgery, grade, and diagnostic confirmation. The resulting model could predict one, two, and five-year survival with an AUC of 0.87. Finally, Gupta et al. used several ML algorithms including random forests and SVMs to predict tumor stage and disease-free survival based on tumor aggression score (80). An accuracy of 84% was obtained using this model. In one systematic review focused on radiomics for the prediction of treatment outcome and survival, Staal et al. found that the literature had heterogenous methods and included features, but they nonetheless found good performance with respect to predicting response in rectal cancer in robust studies (22).
Planning treatment for colorectal lesions is a multifaceted approach and involves several therapeutic modalities depending on TNM staging criteria, and other clinicopathological characteristics (81, 82). For instance, for patients with stage IV CRC, anti-EGFR, immunotherapy or anti-VEGF may be selected depending on mismatch repair and MSI status (74). In other cases, preoperative neoadjuvant chemoradiotherapy in combination with mesorectal excision might be recommended for T3 and T4 node positive tumors, while T1 and T2 node negative cases may be more suitable for submucosal excision with no preoperative therapy (67, 81). To this end, AI can help in disease staging for treatment planning in patient with CRC. One study conducted by Kim et al. showed that AI could be used to differentiate T2 and T3 rectal cancers (81). Using 290 MRI images from 133 patients, a CNN algorithm was developed to automatically segment and classify tumors as either T2 or T3 with an accuracy of 94%. More recently, Wu et al. utilized faster region-based CNNs to create an automatic diagnosis platform for T staging of rectal cancer via MRI (83). The study found AUC of 1 for T1-T4 stages in the horizontal plane and 0.96, 0.97, 0.97, and 0.97 for T1-T4, respectively. In addition to T stage, AI is being increasingly used in lymph node staging for CRC. A recent systematic review and meta-analysis included 17 studies focused on detecting lymph node metastasis in CRC that were published from January 2010 to October 2020 (20). 12 (70.6%) of the studies that met inclusion criteria utilized radiomics models and 5 (29.4%) used deep learning models. The analysis found a per-patient AUC of 0.92 for the deep learning and 0.81 the radiomics models, which were significantly greater than that of the radiologists 0.69 in rectal cancer. Similar results were seen in CRC, where the per-patient AUC in the radiomics model 0.73 was greater than that of the radiologist 0.68. Furthermore, lymph node metastasis is an important consideration for additional surgery in T1 CRCs following endoscopic resection. Ichimasa et al. analyzed 690 patients with T1 CRCs that were surgically resected and developed an AI model with 45 clinicopathological factors to predict presence or absence of lymph node metastasis compared to American, European, and Japanese guidelines (84). The study found a significantly lower rate of unnecessary additional surgery attributable to the false positive detection of lymph node metastasis (AI model: 77% versus 85%, 91%, and 91% in the American (NCCN), European (ESMO), and Japanese (JSCCR) guidelines, respectively; all P < 0.001).
Apart from staging, AI can also aid in the treatment decision-making for CRC via personalized evidence-based consulting through supporting systems such as IBM’s Watson for Oncology (WFO). Aikemu et al. evaluated the concordance between treatment recommendations for 250 patients with CRC from WFO and those from a multidisciplinary team at a major center (23). The study found an overall concordance of 91%, and in subgroup analyses, they found overall concordance rates of 83, 94, and 88% for stages II, III, and IV, respectively, and 97, 93, 89, 87, and 100% for neoadjuvant, surgery, adjuvant, first line, and second line treatments, respectively.
A recent systematic review analyzed the use of radiomics in predicting response to treatment for both primary and metastatic CRC (24). The review included 27 studies in primary CRC 2015-2019. All of the included studies focused on the response to chemoradiotherapy. 21 (77.8%) studies obtained radiomic features from MRI. 26 (96.3%) of these studies evaluated the pathologic response to treatment with various methods. While 10 studies evaluated the predictive power of individual radiomic features, most studies either found multiple radiomic feature that were significantly associated with the response to treatment or had developed combination models that were predictive of the response to chemoradiotherapy. Interestingly, four of the identified studies were able to incorporate clinicopathological and/or treatment characteristics into the pre-treatment radiomic features to generate prediction models. For instance, Bibault et al. combined clinical and radiomics features from pretreatment CT scans to create a DNN to predict the complete response to neoadjuvant chemoradiation in 95 patients with T2-4 N0-1 rectal adenocarcinoma (85). The study found that the DNN had an 80% accuracy in predicting complete response and was better than a linear regression model (69.5% accuracy) that used only TNM stage as a predictor and a SVM model (71.58% accuracy) utilizing the same features of the DNN. Cusumano et al. obtained morphological (tumor geometry and shape), statistical (entropy, skewness, and kurtosis), and fractal (tumor heterogeneity) features from the gross tumor volume of T2-weighted pre-treatment MR scans in 198 patients with locally advanced rectal cancer (LARC) (86). They found that most predictive model had accounted for clinical T and N stage. Yi et al. developed a radiomics SVM-based model that incorporated both MRI-based texture analysis and clinicopathological features to predict response to neoadjuvant chemoradiotherapy in 134 patients with LARC (87). The study found that their predictions of pathologic complete response, good-response, and down-staging had high classification efficiencies with AUC of 0.91, 0.90, and 0.93, respectively. Liu et al. also developed a radiomics model that incorporated radiomics signatures and clinicopathologic risk factors, which showed AUC of 0.98 (88).
More recent efforts have focused on predicting pathologic complete response to neoadjuvant chemoradiotherapy in LARC in innovative ways with larger cohorts. Feng et al. utilized a previously validated radiopathomics model, RAdioPathomics Integrated prediction System (RAPIDS), that integrated radiomics features from MR scans and pathomics features from H&E-stained biopsy slides to predict the pathological complete response in patients with LARC (89). They found an AUC of 0.87 in their training cohort of 303 patients, an AUC of 0.86 in their validation cohort of 280 patients, an AUC of 0.81 in another validation cohort of 150 patients, and an AUC of 0.81 in a prospective cohort of 100 patients. Lou et al. developed an AI model by utilizing digital pathological images on 842 patients with LARC and found an AUC of 0.71 in the testing cohort and 0.72 in the external validation cohort (90).
A systematic review included six studies in patients with metastatic colorectal cancer, five of which were focused on colorectal liver metastases (24). Most of these studies evaluated response to chemotherapy and radiomic features from CT imaging. However, only three (60%) of the studies found moderate predictive power (AUC 0.74-0.81). Ahn et al. utilized baseline CT texture analysis in 235 patients with colorectal liver metastasis who underwent chemotherapy using FOLFOX and FOLFIRI (91). They found that the lower skewness in 2D showed an AUC of 0.80 and a narrower SD on 3D showed an AUC of 0.79. In contrast, Zhang et al. used baseline MR texture analysis in 26 patients with 193 colorectal liver metastasis and found that a higher variance, entropy, contrast, and a lower angular second moment, correlation, and inverse difference moment were associated with response to chemotherapy with AUCs of 0.60-0.78 (92). In their multivariable logistic regression analysis, variance and angular second momentum could predict lesions that responded to therapy from those that did not. Helden et al. evaluated radiomic features in patients with metastatic CRC who had pre-treatment 18F-FDG PET/CT scans and underwent first- or third-line palliative systemic treatment, and they found significant correlations with clinical outcome and select radiomic features (93).
In a more recent systematic review and meta-analysis, Russo et al. analyzed the use of AI in predictive models of the response to cytotoxic chemotherapy alone or combined with targeted therapy in patients with metastatic CRC in 26 original articles (25). In their meta-analysis, which included ten articles, they found that the overall weighted means of the AUC were 0.90 in the training sets and 0.83 in the validation sets. Additionally, the delta radiomics and gene signatures were able to accurately identify up to 99% of patients with metastatic CRC that were responders and up to 100% of patients who were non-responders.
Artificial intelligence has also begun to make an impact in CRC surgery (94). One application of AI is in phase recognition, which involves classifying segments of an operation into predetermined surgical phases. Kitaguchi et al. used CNN-based deep learning for automatic surgical phase recognition on 71 laparoscopy sigmoidectomy cases (95). The study found good accuracy of for the automatic surgical phase recognition (91.9%) and 89.4% and 82.5% for the automatic surgical action recognition of extracorporeal action (89.4%) and irrigation (82.5%). In another study, Kitaguchi et al. developed an annotated video dataset of 50 transanal total mesorectal excision procedure, and their deep learning-based model in automatic surgical step recognition resulted in an overall accuracy for all classification steps of 93.2% (96). Moreover, AI has increasing potential for intraoperative guidance with image-based recognition. Kolbinger et al. recently trained CNNs to discriminate surgical phases, anatomical structures, and tissue planes in 57 robot-assisted rectal resection cases (97). Igaki et al. similarly developed a deep learning-based model that could detect the areolar tissue area in a total mesorectal excision plane with a dice coefficient of 0.84 (98). AI has also allowed for real-time microcirculation analysis of colonic perfusion status via indocyanine green angiography to predict anastomotic complications following laparoscopic colorectal surgery (99). Park et al. analyzed and found significantly greater accuracy and consistency in their AI model, which predicted risk of anastomotic complications in patients who underwent laparoscopic surgery for their CRC and that were based on a self-organizing map network, than in the conventional quantitative parameter-based method (99). Mazaki et al. utilized auto-AI model to develop a model that predicted anastomotic leakage in patients who underwent curative surgery for CRC, and they found an AUC of 0.77 (100).
AI has allowed for improvements in surgical training in colorectal surgery. For instance, Kitaguchi et al. generated a 3-D CNN to automate surgical skill assessment in 1480 videos from 74 laparoscopic colorectal surgeries (101). The study found that model was able to automatically classify video clips into screening categories with a mean accuracy of 75.0% and a standard deviation of 6.3%.
Finally, AI has been used to predict outcomes and surgical management of patients with CRC. A recent study by Masum et al. analyzed 4336 patients who underwent colorectal surgery between 2003 and 2019 and built a prediction model for length of stay, readmission, and mortality (102). They achieved an accuracy of 83% with support vector regression algorithms to predict length of stay, an accuracy of 87.5% with a Bidirectional Long Short-Term Memory (BI-LSTM) model that predicted readmission, and an accuracy of 80-96% in their classification predictive modeling predicted three different CRC mortality measures–overall, 31-, and 91-days mortality. A meta-analysis focused on using deep learning networks to analyze videos of laparoscopic procedures found 32 studies with various applications in instrument recognition and detection, phase recognition, anatomy recognition and detection, action recognition, surgery time prediction, and gauze recognition (26).
Several ML models have been clinically validated to a limited extent. One machine learning-based model successfully predicted the ability to distinguish patients with metastatic colorectal cancer who showed increased overall survival and time-to-next treatment benefit with FOLFOX chemotherapy from those with decreased benefit in clinical trial cohorts (103). A weakly supervised DL framework that incorporated three separate CNNs was developed to predict the status of molecular pathways and mutations, such as MSI, in CRC from histology images (104). The algorithm was externally validated on the Pathology Artificial Intelligence Platform challenge cohort, which included 47 slides from three centers in South Korea (105). Furthermore, several ongoing clinical trials are currently validating the use of PolyDeep, which is a CADe and CADx model. PolyDeep Advance 1 (106) aims to explore whether PolyDeep is more sensitive than blinded endoscopists to detect colorectal polyps, PolyDeep Advance 2 (107) aims to evaluate whether Polydeep assisted colonoscopy can reduce the rate of missed adenomas in the first withdrawal, while PolyDeep Advance 3 (108) aims to see if Polydeep can improve the adenoma detection rate. In another clinical trial, a gradient-boosted machine learning model was developed and validated on participants in the prostate, lung, colorectal and ovarian cancer screening (PLCO) Trial who were diagnosed with CRC during follow-up to predict the risk of death within 10 years from diagnosis (109). Moreover, one DNN model developed from 326 histopathological slides for automated classification of colorectal polypos achieved an internal accuracy of 93.5% and an accuracy of 87% following external validation in 24 US-based institutions (110). Similarly, another AI-based radiopathomics model was developed to predict pathological complete response to neoadjuvant chemoradiation in LARC, and was verified in two external, retrospective cohorts and in a multicenter, prospective observational study (89).
Although recent strides have been made to validate current AI models, the application of AI systems into clinical practice still requires further investigation (111). It is possible that health information technologies such as AI may be an over-confident approach due to its potential risks and limitations. In particular, there may be inherent biases in the data that is used to train the AI models (111). For instance, racial biases and healthcare inequalities may go unnoticed by AI systems, which may be due to the underrepresentation of minority groups and lack of diversity in the training data. In particular, one AI model for MSI detection had lower performance in a cohort involving a high number of Ashkenazi Jews due to their elevated proportion of the BRAF mutation (69). To this end, there may also be overrepresentation of certain groups and the presence of inter-rater variability in the data-labelling process (111, 112). For this reason, algorithms must be externally validated in which outcomes are reproduced in different contexts to ensure the rigor of the models. Moreover, AI algorithms may not be able to adapt to the evolving nature of real-world data (111). Discrepancy between the training data and subsequent data can create data drifts which may hinder the clinical utility of the algorithm. Other concerns involve ethical responsibility and accountability in the presence of AI errors. There is also a lack of structure and standardization regarding the storage and collection of data (111, 112).
While promising, the use of AI in clinical medicine is still at an early stage (113). One of the biggest limitations with AI is that models tend to be limited by the amount and quality of available labeled data for model development and validation. The generalizability of the models is also based on the type of data used in training. Large datasets that are ethnographically diverse will be required to ensure that models can be applied for decision-making in diverse patient populations. Furthermore, there is a need to establish ethical guidelines before models can ever be widely employed to ensure their appropriate use and access (27). Another issue with the clinical application of AI and ML is the “black box” problem, in which we are able to see the inputs and outputs of a model, but not the variables that are used by the model to generate those outputs. More efforts are needed to make the algorithms, especially deep learning algorithms, interpretable to clinicians and to allow streamlining of data preprocessing (114). Additionally, most of the studies have a retrospective design, and more evidence on the effectiveness of AI is needed from multicenter, prospective studies. Finally, standards need to be established for the required accuracy rates to ensure the safe use and legality of AI technology. Efforts are needed to ensure that sensitive data is kept confidential (115). Nonetheless, the potential of AI in medicine, and specifically CRC is promising. AI is likely to prove beneficial and be closely intertwined with the practice of medicine.
In conclusion, the use of AI for CRC is highly promising despite being in its early stages of development. AI has exceeding potential to revolutionize the scope of CRC management with substantial progress already made in diagnostics, prognostication, and therapeutics during the past decade. While there remains certain challenges to overcome with regards to the generalizability, validation, and clinical application of these technologies, future developments may eventually lead to improved outcomes and to a paradigm shift in how we care for patients with suspected or diagnosed CRC.
Conceptualization: AM, ZS, TE, DD, investigation: AM, ZS, TE, writing-original draft preparation: AM, ZS, TE, writing-review and editing: AM, ZS, TE, DD, supervision and project administration: DD. All authors contributed to the article and approved the submitted version.
The authors declare that the research was conducted in the absence of any commercial or financial relationships that could be construed as a potential conflict of interest.
All claims expressed in this article are solely those of the authors and do not necessarily represent those of their affiliated organizations, or those of the publisher, the editors and the reviewers. Any product that may be evaluated in this article, or claim that may be made by its manufacturer, is not guaranteed or endorsed by the publisher.
1. Observatory. TGC. colorectum fact sheet (2020). Available at: https://gco.iarc.fr/today/data/factsheets/cancers/10_8_9-Colorectum-fact-sheet.pdf.
2. Zhou H, Liu Z, Wang Y, Wen X, Amador EH, Yuan L, et al. Colorectal liver metastasis: Molecular mechanism and interventional therapy. Signal Transduct Target Ther (2022) 7(1):70. doi: 10.1038/s41392-022-00922-2
3. Xi Y, Xu P. Global colorectal cancer burden in 2020 and projections to 2040. Transl Oncol (2021) 14(10):101174. doi: 10.1016/j.tranon.2021.101174
4. Hamet P, Tremblay J. Artificial intelligence in medicine. Metabolism (2017) 69s:S36–s40. doi: 10.1016/j.metabol.2017.01.011
5. Bhardwaj V, Sharma A, Parambath SV, Gul I, Zhang X, Lobie PE, et al. Machine learning for endometrial cancer prediction and prognostication. Front Oncol (2022) 12:852746. doi: 10.3389/fonc.2022.852746
6. Deo RC. Machine learning in medicine. Circulation (2015) 132(20):1920–30. doi: 10.1161/circulationaha.115.001593
7. Chan HP, Samala RK, Hadjiiski LM, Zhou C. Deep learning in medical image analysis. Adv Exp Med Biol (2020) 1213:3–21. doi: 10.1007/978-3-030-33128-3_1
8. Koçak B, Durmaz E, Ateş E, Kılıçkesmez Ö. Radiomics with artificial intelligence: A practical guide for beginners. Diagn Interv Radiol (2019) 25(6):485–95. doi: 10.5152/dir.2019.19321
9. Wagner MW, Namdar K, Biswas A, Monah S, Khalvati F, Ertl-Wagner BB. Radiomics, machine learning, and artificial intelligence-what the neuroradiologist needs to know. Neuroradiology (2021) 63(12):1957–67. doi: 10.1007/s00234-021-02813-9
10. Hosny A, Parmar C, Coroller TP, Grossmann P, Zeleznik R, Kumar A, et al. Deep learning for lung cancer prognostication: A retrospective multi-cohort radiomics study. PloS Med (2018) 15(11):e1002711. doi: 10.1371/journal.pmed.1002711
11. Hassan C, Spadaccini M, Iannone A, Maselli R, Jovani M, Chandrasekar VT, et al. Performance of artificial intelligence in colonoscopy for adenoma and polyp detection: A systematic review and meta-analysis. Gastrointest Endosc (2021) 93(1):77–85.e6. doi: 10.1016/j.gie.2020.06.059
12. Huang D, Shen J, Hong J, Zhang Y, Dai S, Du N, et al. Effect of artificial intelligence-aided colonoscopy for adenoma and polyp detection: A meta-analysis of randomized clinical trials. Int J Colorectal Dis (2022) 37(3):495–506. doi: 10.1007/s00384-021-04062-x
13. Gong D, Wu L, Zhang J, Mu G, Shen L, Liu J, et al. Detection of colorectal adenomas with a real-time computer-aided system (Endoangel): A randomised controlled study. Lancet Gastroenterol Hepatol (2020) 5(4):352–61. doi: 10.1016/s2468-1253(19)30413-3
14. Su JR, Li Z, Shao XJ, Ji CR, Ji R, Zhou RC, et al. Impact of a real-time automatic quality control system on colorectal polyp and adenoma detection: A prospective randomized controlled study (with videos). Gastrointest Endosc (2020) 91(2):415–24.e4. doi: 10.1016/j.gie.2019.08.026
15. Lui TKL, Guo CG, Leung WK. Accuracy of artificial intelligence on histology prediction and detection of colorectal polyps: A systematic review and meta-analysis. Gastrointest Endosc (2020) 92(1):11–22.e6. doi: 10.1016/j.gie.2020.02.033
16. Thakur N, Yoon H, Chong Y. Current trends of artificial intelligence for colorectal cancer pathology image analysis: A systematic review. Cancers (Basel) (2020) 12(7):1884 doi: 10.3390/cancers12071884
17. Davri A, Birbas E, Kanavos T, Ntritsos G, Giannakeas N, Tzallas AT, et al. Deep learning on histopathological images for colorectal cancer diagnosis: A systematic review. Diagnostics (Basel) (2022) 12(4):837 doi: 10.3390/diagnostics12040837
18. Ginghina O, Hudita A, Zamfir M, Spanu A, Mardare M, Bondoc I, et al. Liquid biopsy and artificial intelligence as tools to detect signatures of colorectal malignancies: A modern approach in patient's stratification. Front Oncol (2022) 12:856575. doi: 10.3389/fonc.2022.856575
19. Nazarian S, Glover B, Ashrafian H, Darzi A, Teare J. Diagnostic accuracy of artificial intelligence and computer-aided diagnosis for the detection and characterization of colorectal polyps: Systematic review and meta-analysis. J Med Internet Res (2021) 23(7):e27370. doi: 10.2196/27370
20. Bedrikovetski S, Dudi-Venkata NN, Kroon HM, Seow W, Vather R, Carneiro G, et al. Artificial intelligence for pre-operative lymph node staging in colorectal cancer: A systematic review and meta-analysis. BMC Cancer (2021) 21(1):1058. doi: 10.1186/s12885-021-08773-w
21. Park JH, Kim EY, Luchini C, Eccher A, Tizaoui K, Shin JI, et al. Artificial intelligence for predicting microsatellite instability based on tumor histomorphology: A systematic review. Int J Mol Sci (2022) 23(5):2462 doi: 10.3390/ijms23052462
22. Staal FCR, van der Reijd DJ, Taghavi M, Lambregts DMJ, Beets-Tan RGH, Maas M. Radiomics for the prediction of treatment outcome and survival in patients with colorectal cancer: A systematic review. Clin Colorectal Cancer (2021) 20(1):52–71. doi: 10.1016/j.clcc.2020.11.001
23. Aikemu B, Xue P, Hong H, Jia H, Wang C, Li S, et al. Artificial intelligence in decision-making for colorectal cancer treatment strategy: An observational study of implementing Watson for oncology in a 250-case cohort. Front Oncol (2020) 10:594182. doi: 10.3389/fonc.2020.594182
24. Wesdorp NJ, Hellingman T, Jansma EP, van Waesberghe JTM, Boellaard R, Punt CJA, et al. Advanced analytics and artificial intelligence in gastrointestinal cancer: A systematic review of radiomics predicting response to treatment. Eur J Nucl Med Mol Imaging (2021) 48(6):1785–94. doi: 10.1007/s00259-020-05142-w
25. Russo V, Lallo E, Munnia A, Spedicato M, Messerini L, D'Aurizio R, et al. Artificial intelligence predictive models of response to cytotoxic chemotherapy alone or combined to targeted therapy for metastatic colorectal cancer patients: A systematic review and meta-analysis. Cancers (Basel) (2022) 14(16):4012 doi: 10.3390/cancers14164012
26. Anteby R, Horesh N, Soffer S, Zager Y, Barash Y, Amiel I, et al. Deep learning visual analysis in laparoscopic surgery: A systematic review and diagnostic test accuracy meta-analysis. Surg Endosc (2021) 35(4):1521–33. doi: 10.1007/s00464-020-08168-1
27. Qiu H, Ding S, Liu J, Wang L, Wang X. Applications of artificial intelligence in screening, diagnosis, treatment, and prognosis of colorectal cancer. Curr Oncol (2022) 29(3):1773–95. doi: 10.3390/curroncol29030146
28. Wang Y, He X, Nie H, Zhou J, Cao P, Ou C. Application of artificial intelligence to the diagnosis and therapy of colorectal cancer. Am J Cancer Res (2020) 10(11):3575–98.
29. Young PE, Womeldorph CM. Colonoscopy for colorectal cancer screening. J Cancer (2013) 4(3):217–26. doi: 10.7150/jca.5829
30. Lee J, Park SW, Kim YS, Lee KJ, Sung H, Song PH, et al. Risk factors of missed colorectal lesions after colonoscopy. Med (Baltimore) (2017) 96(27):e7468. doi: 10.1097/md.0000000000007468
31. Wallace MB, Sharma P, Bhandari P, East J, Antonelli G, Lorenzetti R, et al. Impact of artificial intelligence on miss rate of colorectal neoplasia. Gastroenterology (2022) 163(1):295–304.e5. doi: 10.1053/j.gastro.2022.03.007
32. Jones MA, Islam W, Faiz R, Chen X, Zheng B. Applying artificial intelligence technology to assist with breast cancer diagnosis and prognosis prediction. Front Oncol (2022) (12):980793. doi: 10.3389/fonc.2022.980793
33. Vega P, Valentín F, Cubiella J. Colorectal cancer diagnosis: Pitfalls and opportunities. World J Gastrointest Oncol (2015) 7(12):422–33. doi: 10.4251/wjgo.v7.i12.422
34. Kamba S, Tamai N, Saitoh I, Matsui H, Horiuchi H, Kobayashi M, et al. Reducing adenoma miss rate of colonoscopy assisted by artificial intelligence: A multicenter randomized controlled trial. J Gastroenterol (2021) 56(8):746–57. doi: 10.1007/s00535-021-01808-w
35. Glissen Brown JR, Mansour NM, Wang P, Chuchuca MA, Minchenberg SB, Chandnani M, et al. Deep learning computer-aided polyp detection reduces adenoma miss rate: A united states multi-center randomized tandem colonoscopy study (Cadet-Cs trial). Clin Gastroenterol Hepatol (2022) 20(7):1499–507.e4. doi: 10.1016/j.cgh.2021.09.009
36. Repici A, Spadaccini M, Antonelli G, Correale L, Maselli R, Galtieri PA, et al. Artificial intelligence and colonoscopy experience: Lessons from two randomised trials. Gut (2022) 71(4):757–65. doi: 10.1136/gutjnl-2021-324471
37. Pacal I, Karaman A, Karaboga D, Akay B, Basturk A, Nalbantoglu U, et al. An efficient real-time colonic polyp detection with yolo algorithms trained by using negative samples and Large datasets. Comput Biol Med (2022) 141:105031. doi: 10.1016/j.compbiomed.2021.105031
38. Lu YB, Lu SC, Huang YN, Cai ST, Le PH, Hsu FY, et al. A novel convolutional neural network model as an alternative approach to bowel preparation evaluation before colonoscopy in the covid-19 era: A multicenter, single-blinded, randomized study. Am J Gastroenterol (2022) 117(9):1437–43. doi: 10.14309/ajg.0000000000001900
39. Nogueira-Rodríguez A, Domínguez-Carbajales R, López-Fernández H, Iglesias Á, Cubiella J, Fdez-Riverola F, et al. Deep neural networks approaches for detecting and classifying colorectal polyps. Neurocomputing (2021) 423:721–34. doi: 10.1016/j.neucom.2020.02.123
40. Wang P, Xiao X, Glissen Brown JR, Berzin TM, Tu M, Xiong F, et al. Development and validation of a deep-learning algorithm for the detection of polyps during colonoscopy. Nat BioMed Eng (2018) 2(10):741–8. doi: 10.1038/s41551-018-0301-3
41. Li K, Fathan MI, Patel K, Zhang T, Zhong C, Bansal A, et al. Colonoscopy polyp detection and classification: Dataset creation and comparative evaluations. PloS One (2021) 16(8):e0255809. doi: 10.1371/journal.pone.0255809
42. Ozawa T, Ishihara S, Fujishiro M, Kumagai Y, Shichijo S, Tada T. Automated endoscopic detection and classification of colorectal polyps using convolutional neural networks. Therap Adv Gastroenterol (2020) 13:1756284820910659. doi: 10.1177/1756284820910659
43. Akbari M, Mohrekesh M, Nasr-Esfahani E, Soroushmehr SMR, Karimi N, Samavi S, et al. Polyp segmentation in colonoscopy images using fully convolutional network. Annu Int Conf IEEE Eng Med Biol Soc (2018) 2018:69–72. doi: 10.1109/embc.2018.8512197
44. Qadir HA, Balasingham I, Solhusvik J, Bergsland J, Aabakken L, Shin Y. Improving automatic polyp detection using cnn by exploiting temporal dependency in colonoscopy video. IEEE J BioMed Health Inform (2020) 24(1):180–93. doi: 10.1109/jbhi.2019.2907434
45. Godkhindi AM, Gowda RM. Automated detection of polyps in ct colonography images using deep learning algorithms in colon cancer diagnosis. In: 2017 International Conference on Energy. Communication, Data Analytics and Soft Computing (ICECDS). Chennai, India (2017).
46. Kayser M, Soberanis-Mukul RD, Zvereva A-M, Klare P, Navab N, Albarqouni S. Understanding the effects of artifacts on automated polyp detection and incorporating that knowledge Via learning without forgetting. arXiv preprint arXiv:200202883 (2020). 3:1–19 doi: https://doi.org/10.48550/arXiv.2002.02883
47. Bernal J, Tajkbaksh N, Sanchez FJ, Matuszewski BJ, Hao C, Lequan Y, et al. Comparative validation of polyp detection methods in video colonoscopy: Results from the miccai 2015 endoscopic vision challenge. IEEE Trans Med Imaging (2017) 36(6):1231–49. doi: 10.1109/tmi.2017.2664042
48. Theodosi A, Ouzounis S, Kostopoulos S, Glotsos D, Kalatzis I, Tzelepi V, et al. Design of a hybrid deep learning system for discriminating between low-and high-grade colorectal cancer lesions, using microscopy images of ihc stained for Aib1 expression biopsy material. Mach Vision Appl (2021) 32(3):1–17. doi: 10.1007/s00138-021-01184-8
49. Sena P, Fioresi R, Faglioni F, Losi L, Faglioni G, Roncucci L. Deep learning techniques for detecting preneoplastic and neoplastic lesions in human colorectal histological images. Oncol Lett (2019) 18(6):6101–7. doi: 10.3892/ol.2019.10928
50. Korbar B, Olofson AM, Miraflor AP, Nicka CM, Suriawinata MA, Torresani L, et al. Deep learning for classification of colorectal polyps on whole-slide images. J Pathol Inf (2017) 8(1):30. doi: 10.4103/jpi.jpi_34_17
51. Lee KS, Son SH, Park SH, Kim ES. Automated detection of colorectal tumors based on artificial intelligence. BMC Med Inform Decis Mak (2021) 21(1):33. doi: 10.1186/s12911-020-01314-8
52. Iizuka O, Kanavati F, Kato K, Rambeau M, Arihiro K, Tsuneki M. Deep learning models for histopathological classification of gastric and colonic epithelial tumours. Sci Rep (2020) 10(1):1504. doi: 10.1038/s41598-020-58467-9
53. Takemura Y, Yoshida S, Tanaka S, Kawase R, Onji K, Oka S, et al. Computer-aided system for predicting the histology of colorectal tumors by using narrow-band imaging magnifying colonoscopy (with video). Gastrointest Endosc (2012) 75(1):179–85. doi: 10.1016/j.gie.2011.08.051
54. Tamai N, Saito Y, Sakamoto T, Nakajima T, Matsuda T, Sumiyama K, et al. Effectiveness of computer-aided diagnosis of colorectal lesions using novel software for magnifying narrow-band imaging: A pilot study. Endosc Int Open (2017) 5(8):E690–e4. doi: 10.1055/s-0043-105490
55. Chen PJ, Lin MC, Lai MJ, Lin JC, Lu HH, Tseng VS. Accurate classification of diminutive colorectal polyps using computer-aided analysis. Gastroenterology (2018) 154(3):568–75. doi: 10.1053/j.gastro.2017.10.010
56. Wang KS, Yu G, Xu C, Meng XH, Zhou J, Zheng C, et al. Accurate diagnosis of colorectal cancer based on histopathology images using artificial intelligence. BMC Med (2021) 19(1):76. doi: 10.1186/s12916-021-01942-5
57. Pan Y, Zhang L, Zhang R, Han J, Qin W, Gu Y, et al. Screening and diagnosis of colorectal cancer and advanced adenoma by bionic glycome method and machine learning. Am J Cancer Res (2021) 11(6):3002–20.
58. Ivancic MM, Megna BW, Sverchkov Y, Craven M, Reichelderfer M, Pickhardt PJ, et al. Noninvasive detection of colorectal carcinomas using serum protein biomarkers. J Surg Res (2020) 246:160–9. doi: 10.1016/j.jss.2019.08.004
59. Ayling RM, Lewis SJ, Cotter F. Potential roles of artificial intelligence learning and faecal immunochemical testing for prioritisation of colonoscopy in anaemia. Br J Haematol (2019) 185(2):311–6. doi: 10.1111/bjh.15776
60. García-Figueiras R, Baleato-González S, Padhani AR, Marhuenda A, Luna A, Alcalá L, et al. Advanced imaging of colorectal cancer: From anatomy to molecular imaging. Insights into Imaging (2016) 7(3):285–309. doi: 10.1007/s13244-016-0465-x
61. Ştefănescu D, Streba C, Cârţână ET, Săftoiu A, Gruionu G, Gruionu LG. Computer aided diagnosis for confocal laser endomicroscopy in advanced colorectal adenocarcinoma. PloS One (2016) 11(5):e0154863. doi: 10.1371/journal.pone.0154863
62. Fugazza A, Gaiani F, Carra MC, Brunetti F, Lévy M, Sobhani I, et al. Confocal laser endomicroscopy in gastrointestinal and pancreatobiliary diseases: A systematic review and meta-analysis. BioMed Res Int (2016) 2016:4638683. doi: 10.1155/2016/4638683
63. André B, Vercauteren T, Buchner AM, Krishna M, Ayache N, Wallace MB. Software for automated classification of probe-based confocal laser endomicroscopy videos of colorectal polyps. World J Gastroenterol (2012) 18(39):5560–9. doi: 10.3748/wjg.v18.i39.5560
64. Robertson KD, Singh R. Capsule endoscopy. In: Statpearls. [StatPearls Publishing: Treasure Island (FL)] (2021).
65. Ding L, Liu GW, Zhao BC, Zhou YP, Li S, Zhang ZD, et al. Artificial intelligence system of faster region-based convolutional neural network surpassing senior radiologists in evaluation of metastatic lymph nodes of rectal cancer. Chin Med J (Engl) (2019) 132(4):379–87. doi: 10.1097/cm9.0000000000000095
66. He J, Wang Q, Zhang Y, Wu H, Zhou Y, Zhao S. Preoperative prediction of regional lymph node metastasis of colorectal cancer based on (18)F-fdg Pet/Ct and machine learning. Ann Nucl Med (2021) 35(5):617–27. doi: 10.1007/s12149-021-01605-8
67. Liu H, Zhao Y, Yang F, Lou X, Wu F, Li H, et al. Preoperative prediction of lymph node metastasis in colorectal cancer with deep learning. BME Front (2022) 2022:1058 doi: 10.34133/2022/9860179
68. Kiritani S, Yoshimura K, Arita J, Kokudo T, Hakoda H, Tanimoto M, et al. A new rapid diagnostic system with ambient mass spectrometry and machine learning for colorectal liver metastasis. BMC Cancer (2021) 21(1):262. doi: 10.1186/s12885-021-08001-5
69. Echle A, Ghaffari Laleh N, Quirke P, Grabsch HI, Muti HS, Saldanha OL, et al. Artificial intelligence for detection of microsatellite instability in colorectal cancer-a multicentric analysis of a pre-screening tool for clinical application. ESMO Open (2022) 7(2):100400. doi: 10.1016/j.esmoop.2022.100400
70. Cao R, Yang F, Ma SC, Liu L, Zhao Y, Li Y, et al. Development and interpretation of a pathomics-based model for the prediction of microsatellite instability in colorectal cancer. Theranostics (2020) 10(24):11080–91. doi: 10.7150/thno.49864
71. Shimada Y, Okuda S, Watanabe Y, Tajima Y, Nagahashi M, Ichikawa H, et al. Histopathological characteristics and artificial intelligence for predicting tumor mutational burden-high colorectal cancer. J Gastroenterol (2021) 56(6):547–59. doi: 10.1007/s00535-021-01789-w
72. González-Castro V, Cernadas E, Huelga E, Fernández-Delgado M, Porto J, Antunez JR, et al. Ct radiomics in colorectal cancer: Detection of kras mutation using texture analysis and machine learning. Appl Sci (2020) 10(18):6214. doi: https://doi.org/10.3390/app10186214
73. Feldman M, Frodtran SY. Enfermedades gastrointestinales y hepaticas. In: Sleisenger y frodtran enfermedades gastrointestinales y hepaticas. (Spain: Elsevier) (2004).
74. Jiang A, Liu N, Zhao R, Liu S, Gao H, Wang J, et al. Construction and validation of a novel nomogram to predict the overall survival of patients with combined small cell lung cancer: A surveillance, epidemiology, and end results population-based study. Cancer Control (2021) 28:10732748211051228. doi: 10.1177/10732748211051228
75. Wang Y, Wang D, Ye X, Wang Y, Yin Y, Jin Y. A tree ensemble-based two-stage model for advanced-stage colorectal cancer survival prediction. Inf Sci (2019) 474:106–24. doi: 10.1016/j.ins.2018.09.046
76. Skrede OJ, De Raedt S, Kleppe A, Hveem TS, Liestøl K, Maddison J, et al. Deep learning for prediction of colorectal cancer outcome: A discovery and validation study. Lancet (2020) 395(10221):350–60. doi: 10.1016/s0140-6736(19)32998-8
77. Kather JN, Krisam J, Charoentong P, Luedde T, Herpel E, Weis CA, et al. Predicting survival from colorectal cancer histology slides using deep learning: A retrospective multicenter study. PloS Med (2019) 16(1):e1002730. doi: 10.1371/journal.pmed.1002730
78. Daye D, Tabari A, Kim H, Chang K, Kamran SC, Hong TS, et al. Quantitative tumor heterogeneity mri profiling improves machine learning-based prognostication in patients with metastatic colon cancer. Eur Radiol (2021) 31(8):5759–67. doi: 10.1007/s00330-020-07673-0
79. Al-Bahrani R, Agrawal A, Choudhary A. Survivability prediction of colon cancer patients using neural networks. Health Inf J (2019) 25(3):878–91. doi: 10.1177/1460458217720395
80. Gupta P, Chiang SF, Sahoo PK, Mohapatra SK, You JF, Onthoni DD, et al. Prediction of colon cancer stages and survival period with machine learning approach. Cancers (Basel) (2019) 11(12):2007 doi: 10.3390/cancers11122007
81. Kim J, Oh JE, Lee J, Kim MJ, Hur BY, Sohn DK, et al. Rectal cancer: Toward fully automatic discrimination of T2 and T3 rectal cancers using deep convolutional neural network. Int J Imaging Syst Technol (2019) 29(3):247–59. doi: 10.1002/ima.22311
82. American Cancer Society. Treatment of colon cancer, by stage (2020). Available at: https://www.cancer.org/cancer/colon-rectal-cancer/treating/by-stage-colon.html.
83. Wu QY, Liu SL, Sun P, Li Y, Liu GW, Liu SS, et al. Establishment and clinical application value of an automatic diagnosis platform for rectal cancer T-staging based on a deep neural network. Chin Med J (Engl) (2021) 134(7):821–8. doi: 10.1097/cm9.0000000000001401
84. Ichimasa K, Kudo SE, Mori Y, Misawa M, Matsudaira S, Kouyama Y, et al. Artificial intelligence may help in predicting the need for additional surgery after endoscopic resection of T1 colorectal cancer. Endoscopy (2018) 50(3):230–40. doi: 10.1055/s-0043-122385
85. Bibault JE, Giraud P, Housset M, Durdux C, Taieb J, Berger A, et al. Deep learning and radiomics predict complete response after neo-adjuvant chemoradiation for locally advanced rectal cancer. Sci Rep (2018) 8(1):12611. doi: 10.1038/s41598-018-30657-6
86. Cusumano D, Dinapoli N, Boldrini L, Chiloiro G, Gatta R, Masciocchi C, et al. Fractal-based radiomic approach to predict complete pathological response after chemo-radiotherapy in rectal cancer. Radiol Med (2018) 123(4):286–95. doi: 10.1007/s11547-017-0838-3
87. Yi X, Pei Q, Zhang Y, Zhu H, Wang Z, Chen C, et al. Mri-based radiomics predicts tumor response to neoadjuvant chemoradiotherapy in locally advanced rectal cancer. Front Oncol (2019) 9:552. doi: 10.3389/fonc.2019.00552
88. Liu Z, Zhang XY, Shi YJ, Wang L, Zhu HT, Tang Z, et al. Radiomics analysis for evaluation of pathological complete response to neoadjuvant chemoradiotherapy in locally advanced rectal cancer. Clin Cancer Res (2017) 23(23):7253–62. doi: 10.1158/1078-0432.Ccr-17-1038
89. Feng L, Liu Z, Li C, Li Z, Lou X, Shao L, et al. Development and validation of a radiopathomics model to predict pathological complete response to neoadjuvant chemoradiotherapy in locally advanced rectal cancer: A multicentre observational study. Lancet Digit Health (2022) 4(1):e8–e17. doi: 10.1016/s2589-7500(21)00215-6
90. Lou X, Zhou N, Feng L, Li Z, Fang Y, Fan X, et al. Deep learning model for predicting the pathological complete response to neoadjuvant chemoradiotherapy of locally advanced rectal cancer. Front Oncol (2022) 12:807264. doi: 10.3389/fonc.2022.807264
91. Ahn SJ, Kim JH, Park SJ, Han JK. Prediction of the therapeutic response after folfox and folfiri treatment for patients with liver metastasis from colorectal cancer using computerized ct texture analysis. Eur J Radiol (2016) 85(10):1867–74. doi: 10.1016/j.ejrad.2016.08.014
92. Zhang H, Li W, Hu F, Sun Y, Hu T, Tong T. Mr Texture analysis: Potential imaging biomarker for predicting the chemotherapeutic response of patients with colorectal liver metastases. Abdom Radiol (NY) (2019) 44(1):65–71. doi: 10.1007/s00261-018-1682-1
93. van Helden EJ, Vacher YJL, van Wieringen WN, van Velden FHP, Verheul HMW, Hoekstra OS, et al. Radiomics analysis of pre-treatment [(18)F]Fdg Pet/Ct for patients with metastatic colorectal cancer undergoing palliative systemic treatment. Eur J Nucl Med Mol Imaging (2018) 45(13):2307–17. doi: 10.1007/s00259-018-4100-6
94. Quero G, Mascagni P, Kolbinger FR, Fiorillo C, De Sio D, Longo F, et al. Artificial intelligence in colorectal cancer surgery: Present and future perspectives. Cancers (Basel) (2022) 14(15):3803 doi: 10.3390/cancers14153803
95. Kitaguchi D, Takeshita N, Matsuzaki H, Takano H, Owada Y, Enomoto T, et al. Real-time automatic surgical phase recognition in laparoscopic sigmoidectomy using the convolutional neural network-based deep learning approach. Surg Endosc (2020) 34(11):4924–31. doi: 10.1007/s00464-019-07281-0
96. Kitaguchi D, Takeshita N, Matsuzaki H, Hasegawa H, Igaki T, Oda T, et al. Deep learning-based automatic surgical step recognition in intraoperative videos for transanal total mesorectal excision. Surg Endosc (2022) 36(2):1143–51. doi: 10.1007/s00464-021-08381-6
97. Kolbinger FR, Leger S, Carstens M, Rinner FM, Krell S, Chernykh A, et al. Artificial intelligence for context-aware surgical guidance in complex robot-assisted oncological procedures: An exploratory feasibility study. medRxiv (2022). doi: 10.1101/2022.05.02.22274561
98. Igaki T, Kitaguchi D, Kojima S, Hasegawa H, Takeshita N, Mori K, et al. Artificial intelligence-based total mesorectal excision plane navigation in laparoscopic colorectal surgery. Dis Colon Rectum (2022) 65(5):e329–e33. doi: 10.1097/dcr.0000000000002393
99. Park SH, Park HM, Baek KR, Ahn HM, Lee IY, Son GM. Artificial intelligence based real-time microcirculation analysis system for laparoscopic colorectal surgery. World J Gastroenterol (2020) 26(44):6945–62. doi: 10.3748/wjg.v26.i44.6945
100. Mazaki J, Katsumata K, Ohno Y, Udo R, Tago T, Kasahara K, et al. A novel predictive model for anastomotic leakage in colorectal cancer using auto-artificial intelligence. Anticancer Res (2021) 41(11):5821–5. doi: 10.21873/anticanres.15400
101. Kitaguchi D, Takeshita N, Matsuzaki H, Igaki T, Hasegawa H, Ito M. Development and validation of a 3-dimensional convolutional neural network for automatic surgical skill assessment based on spatiotemporal video analysis. JAMA Netw Open (2021) 4(8):e2120786. doi: 10.1001/jamanetworkopen.2021.20786
102. Masum S, Hopgood A, Stefan S, Flashman K, Khan J. Data analytics and artificial intelligence in predicting length of stay, readmission, and mortality: A population-based study of surgical management of colorectal cancer. Discovery Oncol (2022) 13(1):11. doi: 10.1007/s12672-022-00472-7
103. Abraham JP, Magee D, Cremolini C, Antoniotti C, Halbert DD, Xiu J, et al. Clinical validation of a machine-Learning-Derived signature predictive of outcomes from first-line oxaliplatin-based chemotherapy in advanced colorectal cancer. Clin Cancer Res (2021) 27(4):1174–83. doi: 10.1158/1078-0432.Ccr-20-3286
104. Bilal M, Raza SEA, Azam A, Graham S, Ilyas M, Cree IA, et al. Development and validation of a weakly supervised deep learning framework to predict the status of molecular pathways and key mutations in colorectal cancer from routine histology images: A retrospective study. Lancet Digit Health (2021) 3(12):e763–e72. doi: 10.1016/s2589-7500(21)00180-1
105. Kang Y, Kim YJ, Park S, Ro G, Hong C, Jang H, et al. Development and operation of a digital platform for sharing pathology image data. BMC Med Inform Decis Mak (2021) 21(1):114. doi: 10.1186/s12911-021-01466-1
106. Clinical validation of polydeep: An artificial intelligence-based computer-aided polyp detection (Cade) and characterization (Cadx) system . Available at: https://ClinicalTrials.gov/show/NCT05514301.
107. Clinical validation of polydeep: An artificial intelligence-based computer-aided polyp detection (Cade) and characterization (Cadx) system. polydeep advance 2 . Available at: https://ClinicalTrials.gov/show/NCT05512793.
108. Clinical validation of polydeep: An artificial intelligence-based computer-aided polyp detection (Cade) and characterization (Cadx) system. polydeep advance 3 . Available at: https://ClinicalTrials.gov/show/NCT05513261.
109. Bibault JE, Chang DT, Xing L. Development and validation of a model to predict survival in colorectal cancer using a gradient-boosted machine. Gut (2021) 70(5):884–9. doi: 10.1136/gutjnl-2020-321799
110. Wei JW, Suriawinata AA, Vaickus LJ, Ren B, Liu X, Lisovsky M, et al. Evaluation of a deep neural network for automated classification of colorectal polyps on histopathologic slides. JAMA Netw Open (2020) 3(4):e203398. doi: 10.1001/jamanetworkopen.2020.3398
111. Ameen S, Wong M-C, Yee K-C, Turner P. Ai and clinical decision making: The limitations and risks of computational reductionism in bowel cancer screening. Appl Sci (2022) 12(7):3341. doi: 10.3390/app12073341
112. Shreve JT, Khanani SA, Haddad TC. Artificial intelligence in oncology: Current capabilities, future opportunities, and ethical considerations. Am Soc Clin Oncol Educ Book (2022) 42:1–10. doi: 10.1200/edbk_350652
113. Yu C, Helwig EJ. The role of ai technology in prediction, diagnosis and treatment of colorectal cancer. Artif Intell Rev (2022) 55(1):323–43. doi: 10.1007/s10462-021-10034-y
114. Liang F, Wang S, Zhang K, Liu TJ, Li JN. Development of artificial intelligence technology in diagnosis, treatment, and prognosis of colorectal cancer. World J Gastrointest Oncol (2022) 14(1):124–52. doi: 10.4251/wjgo.v14.i1.124
Keywords: artificial intelligence, radiomics, deep learning, machine learning, colorectal cancer
Citation: Mansur A, Saleem Z, Elhakim T and Daye D (2023) Role of artificial intelligence in risk prediction, prognostication, and therapy response assessment in colorectal cancer: current state and future directions. Front. Oncol. 13:1065402. doi: 10.3389/fonc.2023.1065402
Received: 09 October 2022; Accepted: 09 January 2023;
Published: 25 January 2023.
Edited by:
Pasquale Cianci, Azienda Sanitaria Localedella Provincia di Barletta Andri Trani (ASL BT), ItalyReviewed by:
Wenbing Lv, Southern Medical University, ChinaCopyright © 2023 Mansur, Saleem, Elhakim and Daye. This is an open-access article distributed under the terms of the Creative Commons Attribution License (CC BY). The use, distribution or reproduction in other forums is permitted, provided the original author(s) and the copyright owner(s) are credited and that the original publication in this journal is cited, in accordance with accepted academic practice. No use, distribution or reproduction is permitted which does not comply with these terms.
*Correspondence: Dania Daye, ZGRheWVAbWdoLmhhcnZhcmQuZWR1
Disclaimer: All claims expressed in this article are solely those of the authors and do not necessarily represent those of their affiliated organizations, or those of the publisher, the editors and the reviewers. Any product that may be evaluated in this article or claim that may be made by its manufacturer is not guaranteed or endorsed by the publisher.
Research integrity at Frontiers
Learn more about the work of our research integrity team to safeguard the quality of each article we publish.