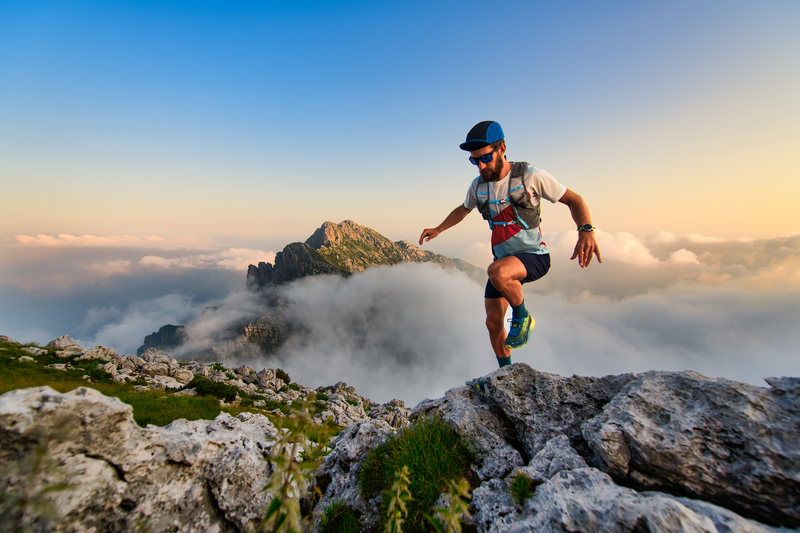
94% of researchers rate our articles as excellent or good
Learn more about the work of our research integrity team to safeguard the quality of each article we publish.
Find out more
ORIGINAL RESEARCH article
Front. Oncol. , 11 July 2023
Sec. Breast Cancer
Volume 13 - 2023 | https://doi.org/10.3389/fonc.2023.1048242
This article is part of the Research Topic Methods in Breast Cancer View all 17 articles
De-escalation trials in oncology evaluate therapies that aim to improve the quality of life of patients with low-risk cancer by avoiding overtreatment. Non-inferiority randomized trials are commonly used to investigate de-intensified regimens with similar efficacy to that of standard regimens but with fewer adverse effects (ESMO evidence tier A). In cases where it is not feasible to recruit the number of patients needed for a randomized trial, single-arm prospective studies with a hypothesis of non-inferiority can be conducted as an alternative. Single-arm studies are also commonly used to evaluate novel treatment strategies (ESMO evidence tier B). A single-arm design that includes both non-inferiority and superiority primary objectives will enable the ranking of clinical activity and other parameters such as safety, pharmacokinetics, and pharmacodynamics data. Here, we describe the statistical principles and procedures to support such a strategy. The non-inferiority margin is calculated using the fixed margin method. Sample size and statistical analyses are based on the maximum likelihood method for exponential distributions. We present example analyses in metastatic and adjuvant settings to illustrate the usefulness of our methodology. We also explain its implementation with nonparametric methods. Single-arm designs with non-inferiority and superiority analyses are optimal for proof-of-concept and de-escalation studies in oncology.
Molecular diagnostics and biomarkers have enabled many cancers to be divided into clinical and biological subtypes, some of which have a low risk of relapse or death (1–4). In patients with low-risk breast cancer, de-escalation trials are increasingly being conducted to evaluate therapies that aim to improve quality of life by avoiding overtreatment (1, 5, 6). These trials use non-inferiority designs to investigate de-intensified regimens with efficacy similar to that of standard treatments but with fewer toxic effects (5, 7–9). Although randomized trials provide the strongest evidence (ESMO evidence tier A) for the efficacy of de-escalation strategies (10–13), randomized designs are not always the most efficient option and cannot be used to answer all research questions (14–18). Furthermore, in certain cancer types and phases of clinical development, it is not feasible to recruit the number of patients needed for a randomized clinical trial. In such cases, de-escalation strategies can be investigated using single-arm or non-comparative trials (ESMO evidence tier B) (6, 13, 19–22). Single-arm trials can also be used to evaluate novel therapies, agents with a high expectation of tumor response, rare cancers, salvage therapies, and therapies for late-stage disease, especially when no standard-of-care exists and a robust historical database is available (15, 18, 23). The inclusion of both non-inferiority and superiority primary objectives in single-arm study designs enables informed decisions that rank the magnitude of clinical activity along with other parameters such as safety, pharmacokinetics, and pharmacodynamics data (24–26).
Some treatments have been successful in phase III trials even after producing negative results in phase II single-arm trials. In these situations, a new treatment was deemed non-inferior to standard-of-care therapy when considered in the context of relevant parameters such as safety, duration of clinical benefit, or targeting of a new biological pathway (27, 28). However, the likelihood of a type I error (α) increases when a post-hoc non-inferiority analysis is performed after an unsuccessful proof-of-concept trial (25, 29). The probability of such an error can be reduced by including the non-inferiority analysis in the experimental design a priori (24–26). It is easy to include non-inferiority and superiority analyses in single-arm one-stage or two-stage studies with response rate as the primary endpoint (24); however, the most reliable and preferred endpoint in cancer studies is overall survival.
It is common to plan proof-of-concept and confirmatory studies in oncology using time-to-event endpoints (19, 30, 31). Most approvals for breast cancer drugs in adjuvant and advanced settings are supported by improvements in overall survival, disease-free survival (DFS), and progression-free survival (PFS) (32). Although there are a few single-arm trials that used a historic control arm to set a non-inferiority threshold for a time-to-event outcome (5, 20, 33), these trials did not include and additional superiority analysis for the primary objective. Here, we propose a single-arm, time-to-event study design that includes both superiority and non-inferiority analyses.
Single-arm studies with a time-to-event primary endpoint usually include a superiority analysis that aims to show that the probability of survival (e.g., median PFS [mPFS]) with a certain treatment is greater than the probability of survival estimated for an active control arm (mPFS0) in a previous trial (34). Conversely, the risk of progression or death with the treatment, represented by a hazard rate (λ) equal to the Napierian logarithm of 2 (LN[2]) divided by mPFS, is expected to be lower than the risk of progression or death in the active control arm (λ0) (34). In contrast to such superiority analyses, a non-inferiority analysis aims to show that the effect of a test drug in terms of survival is not inferior to that of the historical comparator by more than a specified amount called the non-inferiority margin (NIM) (29). The NIM calculation is based on the difference in observed effects between the historical comparator and placebo in previous studies, which is represented by a hazard ratio (HR) that is greater than 1 and equal to either the mPFS0 divided by the mPFS in the placebo arm (mPFSplacebo) or the λ in the placebo arm (λplacebo) divided by the λ0 (7, 24, 29). For example, if the HR is 2.4 with a 95% confidence interval (CI) of 1.44–3.56, the fixed margin method is applied to select the 95% CI lower bound (1.44) and adjust it to retain at least 50% of the historical effect of the active control versus the placebo: 1.44(1–0.5) = 1.2. Accordingly, the calculated NIM describes a ratio reflecting the largest loss of the effect previously observed in the active control arm that would be clinically acceptable (29).
The null hypothesis (H0) for superiority and non-inferiority analyses in a one-sided test can be defined in terms of survival (mPFS) or hazard (λ) parameters as follows:
Additionally, the magnitude of the difference between the treatment arm and the historical control (i.e., the effect size) can be defined in terms of mPFS or λ for superiority and non-inferiority analysis as follows:
The cutoff for H0non-inferiority will be always lower than the cutoff for H0superiority (i.e., mPFS0non-inferiority < mPFS0superiority), and the converse will be true when H0 is defined in terms of hazard rates (i.e., λ0non-inferiority > λ0superiority). At the time of final analysis in a single-arm trial, the number of patients recruited (n), the events observed, the mPFS, and the λ will be equal for the non-inferiority and superiority analyses. Therefore, the difference in effect size between the superiority and non-inferiority analyses is totally dependent on the magnitude of the preplanned H0. In a one-sided test that will accept H0 if the study mPFS is less than the mPFS of the historical control, H0 is rejected only if mPFS is greater than mPFS0 (or λ is less than λ0). In all these scenarios, the absolute value of the effect size in the non-inferiority analysis will be greater than the absolute value of the effect size in the superiority analysis:
The same is true in terms of hazard rates:
As the number of events is equal in the superiority and non-inferiority analyses, it follows that the probability of detecting an effect (i.e., the power of the test) will always be greater in the non-inferiority analysis than in the superiority analysis. Therefore, the type II error level (β) planned for the superiority analysis is retained in the inferiority analysis (24, 35).
As stated in the United States Food and Drug Administration’s multiple endpoint guidelines, despite evaluating multiple hypotheses, “after demonstrating non-inferiority on the endpoint, it is possible to then test for superiority at an unadjusted alpha” Thus, in a superiority analysis with a time-to-event primary endpoint, analysis of a non-inferiority hypothesis does not inflate the type I error rate when the non-inferiority analysis and NIM are properly pre-specified (29, 36).
The design proposed here can be used to assess both superiority and non-inferiority criteria with the same sample size, type I error rate (α), and β that would be used in a superiority-only strategy. This applies to both parametric (exponential or Weibull distribution estimator) and nonparametric (Kaplan–Meier or life table estimator) approaches (34, 37).
The following section provides a numerical example of the proposed design for a typical phase II single-arm (proof-of-concept) trial that includes both non-inferiority and superiority analyses in a metastatic setting. Suppose that mPFS for a standard therapy is 12 months. This corresponds to a λ0 (LN[2]/mPFS[12]) of 0.058. We would design a study to detect mPFS improvement to at least 18 months (λ1 = LN[2]/18 = 0.039), producing an HR of 0.67 (HR = 12/18). We plan a 12-month accrual period (ap) and a 24-month follow-up period (fp). We design the study to attain 90% power (1 – β) using the maximum likelihood method for exponential distributions at a nominal one-sided α level of 10%. The maximum accepted α level in our example is higher than what is usually used in confirmatory trials (i.e., one-sided α of 2.5% or two-sided α of 5%). This is appropriate because of the exploratory nature of our trial. We also assume a 10% dropout rate. The required number of patients and events is calculated as follows, where Z is the standard normal cumulative distribution function for a one-sided test (34):
The Excel functions to resolve this are “INV.NORM.ESTAND(1–(α=0.1))” and “INV.NORM.ESTAND(1–(β=0.1))”; eX represents the natural exponential function (“exp(x)” in Excel).
Continuing with this example, it is supposed that by the end of the study 66 patients have been accrued, 54 PFS events have occurred, and the final mPFS is 12 months, with a hazard rate (λobs) of 0.058. Based on an NIM of 1.2, H0non-inferiority is an mPFS (mPFS0/NIM = 12/1.2) of 10 months, which is equivalent to a non-inferiority hazard rate (λNI = LN[2]/10) of 0.069. Final statistical analyses for the superiority and non-inferiority objectives are performed using the maximum likelihood method for exponential distributions as follows (34):
Non-inferiority:
Superiority:
The expression “ “ is the standard normal cumulative distribution, which is used to back-transform Z-scores into p values.
Usually, the primary objective of clinical trials in adjuvant settings is DFS. The DFS rate is usually higher than 50%, so the median survival is not estimable. However, the previous analyses can be conducted in an adjuvant setting if the DFS rates are transformed into hazard rates and HRs. In a study investigating an adjuvant therapy in early-stage breast cancer by Cardoso et al. (2016), the DFS rate without distant metastasis for a standard therapy was 95% at 5 years (60 months). This corresponds to a λ0 of 0.0009 (5).
The pre-specified NIM corresponds to a 3% difference in DFS without distant metastases at 5 years (i.e., from 95% to 92%). This corresponds to a λ0NI of 0.0014 (5).
The study was designed to attain 80% power at a nominal two-sided α level of 5%. Based on equation (7), the required number of events for this single-arm design is 34. By contrast, 135 events would be needed in a randomized study. The criteria for the primary analysis were met with 748 patients recruited from the primary test population; however, we would need about 3000 patients if we used a comparative design.
De-intensification strategies are often developed in response to new diagnostics that can select patients who do not need aggressive therapy. Our proof-of-concept example shows how a novel de-intensified treatment can be shown to be non-inferior to the standard of care in selected patients. In such a case, if safety data show that the novel treatment is better tolerated than the standard-of-care, further confirmatory studies should be developed to evaluate the novel treatment. Our method can also be used to explore the efficacy of new drugs. For example, if a new drug achieves the non-inferiority objective, as in our proof-of-concept example, and also shows good tolerability, an appropriate pharmacokinetic profile, and/or a novel molecular target, then it might be expected to show further promising results when combined with standard treatment in a phase II/III randomized trial. Similarly, if a new drug achieves the superiority objective in our study design, this would suggest that it may be effective as a monotherapy (24).
Our approach enables the design of non-inferiority breast cancer studies in settings where it is not feasible to recruit enough patients for a comparative analysis (5, 6, 13). Although the sample size needed for a non-inferiority study is usually expected to be greater than the sample size needed for a superiority study, this is a misunderstanding. Actually, when a non-inferiority study uses the same assumptions as a superiority study, the non-inferiority study will always need fewer patients than the superiority study. The reason that non-inferiority studies are thought to need more patients than superiority studies is because non-inferiority studies assume that two treatments are equally effective, whereas superiority studies never make this assumption (38). In addition to the use of a single-arm primary analysis, there are some other ways to improve the quality of data in de-intensification studies. For example, a randomized non-comparative design can be used, in which the randomized control arm has far fewer patients than would be needed for a powered comparative analysis (5, 6). Other approaches might be to use an external control based on previous clinical trials, detailed cancer registries, real-world evidence, and synthetic control arms (39).
A single-arm trial designed to analyze both non-inferiority and superiority objectives enables ranking of early efficacy and other parameters such as safety, pharmacokinetics, and pharmacodynamics data (26), making such an approach more informative than a trial designed to analyze only superiority or non-inferiority (24–26). As non-inferiority analysis is allowed, it could be conjectured that this design makes easier that ineffective therapies were assessed as promising. This assumes that evaluation of the treatment is based exclusively on the non-inferiority result, without considering other objectives. Risk-benefit assessments weighing all endpoints are usually performed when establishing development plans for new drugs. Accordingly, our method supports a comprehensive approach to drug development by enabling the totality of the evidence to be considered in favor of a therapy (40, 41).
Non-inferiority analysis can be implemented in time-to-event studies using the log-rank test methodology (42). In addition, our proposed design can be easily implemented with nonparametric methods such as Kaplan–Meier analysis, which usually estimates median survival or survival rates based on CIs. For instance, we would achieve a positive non-inferiority result with a 90% CI of 3.6–5.6, because the lower bound of the CI would be greater than 3.3, the H0 for the non-inferiority test. Conversely, the superiority objective would not be achieved, because the lower bound of the 90% CI would be lower than 4, the H0 for the superiority test. The sample size in time-to-event designs based on nonparametric tests can be easily calculated with various methods and online calculators (43–45). The same strategy can be used to prespecify thresholds for null hypotheses under a Bayesian framework (31).
Our method includes the inherent drawbacks of studies that rely on historical controls and non-inferiority analyses. These limitations are common and well-known in non-inferiority comparative study designs because the NIM must be based on historical evidence (46). In randomized controlled designs of non-inferiority, it is necessary to demonstrate assay sensitivity to declare a therapy non-inferior in a single-arm trial (12). Additionally, selection of inappropriate patients, premature discontinuations, and poor compliance all favor conclusions of a lack of difference between experimental and control arms in randomized trials. This can lead to erroneous declarations of non-inferiority of the experimental treatment. This bias is reduced when comparisons are based on a theoretical rate of efficacy deduced from historical controls (12, 46). Accordingly, single-arm designs with non-inferiority analyses that are properly preplanned and conducted are not more challenging than the usual randomized or single-arm designs (5, 24).
Various strategies to evaluate multiple endpoints of efficacy and safety in proof-of-concept trials have been proposed in Bayesian and frequentist paradigms (e.g., EFFTox, Gumbel model, continual reassessment method, and single-stage and two-stage time-to-event designs). However, these designs do not rank non-inferiority and superiority hypotheses to grade the magnitude of clinical activity in the early clinical stages (19, 47–49).
Altogether, analyses of non-inferiority and superiority in single-arm trials are easily implemented in typical time-to-event designs for adjuvant and metastatic settings. This approach is useful for weighing additional factors such as safety, cost, and biomarkers while also assessing efficacy, making it optimal for proof-of-concept and de-intensification investigations in oncology.
The raw data supporting the conclusions of this article will be made available by the authors, without undue reservation.
MS-C, AL-C, and JC conceived the study. MS-C, BM-H, DA-L, and EA developed the statistical methods. EL-M, MG, AM, JP-G, JP-E, AL-C, and JC reviewed the methods. MS-C, BM-H, DA-L, and EA conducted the statistical analysis. EL-M, MG, JP-G, AL-C, and JC reviewed the statistical results. MS-C, AM, and JP-G drafted the manuscript. All authors contributed to the article and approved the submitted version.
The authors declare that this study received funding from Medica Scientia Innovation Research (MEDSIR). The funder was not involved in the study design, collection, analysis, interpretation of data, the writing of this article, or the decision to submit it for publication.
Authors MS-C, AM, EL-M, EA, DA-L, JP-E, JP-G, AL-C, JC were employed by company Medica Scientia Innovation Research (MEDSIR).
MS-C declares grants from Medica Scientia Innovation Research MEDSIR, Syntax for Science, Optimapharm, MD Anderson Cancer Center Madrid, and Ability Pharma that are outside the funding for the submitted work. AM is a medical writer at MEDSIR. JP-G declares a consulting role with Roche, and Daichii-Sankyo; receiving travel expenses from Roche, to being a part-time employee of MEDSIR during the study period. AL-C declares playing a leadership role with Eisai, Celgene, Lilly, Pfizer, Roche, Novartis, and MSD; intellectual property with MEDSIR and Initia-Research; consulting roles with Lilly, Roche, Pfizer, Novartis, Pierre-Fabre, GenomicHealth, and GSK; membership in the speaker bureaus of Lilly, AstraZeneca, and MSD; research funding from Roche, Foundation Medicine, Pierre-Fabre, and Agendia; and travel expenses from Roche, Lilly, Novartis, Pfizer, and Astra-Zeneca. JC declares consulting roles with Roche, Celgene, Cellestia, AstraZeneca, Seattle Genetics, Daiichi Sankyo, Erytech, Athenex, Polyphor, Lilly, Merck Sharp&Dohme, GSK, Leuko, Bioasis, Clovis Oncology, Boehringer Ingelheim, Ellipses, Hibercell, BioInvent, Gemoab, Gilead Sciences, Menarini, Zymeworks, and Reveal Genomics; honoraria from Roche, Novartis, Celgene, Eisai, Pfizer, Samsung Bioepis, Lilly, Merck Sharp&Dohme, and Daiichi Sankyo; research funding from Roche, Ariad pharmaceuticals, AstraZeneca, Baxalta GMBH/Servier Affaires, Bayer healthcare, Eisai, F.Hoffman-La Roche, Guardanth health, Merck Sharp&Dohme, Pfizer, Piqur Therapeutics, Puma C, and Queen Mary University of London; intellectual property with MEDSIR, Nektar Pharmaceuticals, and Leuko relative; and receiving travel compensation from Roche, Novartis, Eisai, Pfizer, Daiichi Sankyo, and AstraZeneca.
The remaining authors declare that the research was conducted in the absence of any commercial or financial relationships that could be construed as a potential conflict of interest.
All claims expressed in this article are solely those of the authors and do not necessarily represent those of their affiliated organizations, or those of the publisher, the editors and the reviewers. Any product that may be evaluated in this article, or claim that may be made by its manufacturer, is not guaranteed or endorsed by the publisher.
1. Byng D, Retèl V, Engelhardt E, Groothuis-Oudshoorn C, van Til J, Schmitz R, et al. Preferences of treatment strategies among women with low-risk DCIS and oncologists. Cancers (2021) 13:3962. doi: 10.3390/cancers13163962
2. Administration F and D. Paving the way for personalized medicine: fda’s role in a new era of medical product development. United States: Createspace Independent Pub (2014). p. 64.
3. Bartlett JMS, Parelukar W. Breast cancers are rare diseases–and must be treated as such. npj. Breast Cancer (2017) 3. doi: 10.1038/s41523-017-0013-y
4. Khoury T, Quinn M, Tian W, Yan L, Zhan H. Touching tumor-infiltrating lymphocytes in low risk ductal carcinoma in situ correlates with upgrade to high grade DCIS. Histopathology (2021) 80(2):291–303. doi: 10.1111/his.14539
5. Cardoso F, van’t Veer LJ, Bogaerts J, Slaets L, Viale G, Delaloge S, et al. 70-gene signature as an aid to treatment decisions in early-stage breast cancer. New Engl J Med (2016) 375:717–29. doi: 10.1056/NEJMoa1602253
6. Pérez-García JM, Gebhart G, Ruiz Borrego M, Stradella A, Bermejo B, Schmid P, et al. Chemotherapy de-escalation using an 18F-FDG-PET-based pathological response-adapted strategy in patients with HER2-positive early breast cancer (PHERGain): a multicentre, randomised, open-label, non-comparative, phase 2 trial. Lancet Oncol (2021) 22:858–71. doi: 10.1016/S1470-2045(21)00122-4
7. Earl HM, Hiller L, Vallier A-L, Loi S, McAdam K, Hughes-Davies L, et al. 6 versus 12 months of adjuvant trastuzumab for HER2-positive early breast cancer (PERSEPHONE): 4-year disease-free survival results of a randomised phase 3 non-inferiority trial. Lancet (2019) 393:2599–612. doi: 10.1016/S0140-6736(19)30650-6
8. Pivot X, Romieu G, Debled M, Pierga J-Y, Kerbrat P, Bachelot T, et al. 6 months versus 12 months of adjuvant trastuzumab in early breast cancer (PHARE): final analysis of a multicentre, open-label, phase 3 randomised trial. Lancet (2019) 393:2591–8. doi: 10.1016/S0140-6736(19)30653-1
9. Giuliano AE, Ballman KV, McCall L, Beitsch PD, Brennan MB, Kelemen PR, et al. Effect of axillary dissection vs no axillary dissection on 10-year overall survival among women with invasive breast cancer and sentinel node metastasis: the ACOSOG Z0011 (Alliance) randomized clinical trial. JAMA (2017) 318:918. doi: 10.1001/jama.2017.11470
10. Cochrane handbook for systematic reviews of interventions. Cochrane Training. Available at: http://training.cochrane.org/handbook (Accessed January 31, 2020).
11. ICH Harmonised Tripartite Guideline. ICH topic e 9 statistical principles for clinical trials (1998). Available at: https://www.ema.europa.eu/en/documents/scientific-guideline/ich-e-9-statistical-principles-clinical-trials-step-5_en.pdf.
12. Choice of control group and related issues in clinical trials: ICH E10. Available at: http://www.ich.org/products/guidelines/efficacy/efficacy-single/article/choice-of-control-group-and-related-issues-in-clinical-trials.html (Accessed January 31, 2021).
13. Trapani D, Franzoi MA, Burstein HJ, Carey LA, Delaloge S, Harbeck N, et al. Risk-adapted modulation through de-intensification of cancer treatments: an ESMO classification. Ann Oncol (2022) 33:702–12. doi: 10.1016/j.annonc.2022.03.273
14. Concato J, Shah N, Horwitz RI. Randomized, controlled trials, observational studies, and the hierarchy of research designs. New Engl J Med (2000) 342:1887–92. doi: 10.1056/NEJM200006223422507
15. Gan HK, Grothey A, Pond GR, Moore MJ, Siu LL, Sargent D. Randomized phase II trials: inevitable or inadvisable? J Clin Oncol (2010) 28:2641–7. doi: 10.1200/JCO.2009.26.3343
16. Frieden TR. Evidence for health decision making {{/amp]]mdash; beyond randomized, controlled trials. New Engl J Med (2017) 377:465–75. doi: 10.1056/NEJMra1614394
17. Sampayo-Cordero M, Miguel-Huguet B, Malfettone A, Pérez-García JM, Llombart-Cussac A, Cortés J, et al. The value of case reports in systematic reviews from rare diseases. the example of enzyme replacement therapy (ERT) in patients with mucopolysaccharidosis type II (MPS-II). Int J Environ Res Public Health (2020) 17:6590. doi: 10.3390/ijerph17186590
18. Grayling MJ, Dimairo M, Mander AP, Jaki TF. A review of perspectives on the use of randomization in phase II oncology trials. J Natl Cancer Inst (2019) 111:1255–62. doi: 10.1093/jnci/djz126
19. Ortega V, Antón A, Garau I, Afonso N, Calvo L, Fernández Y, et al. Multicenter, single-arm trial of eribulin as first-line therapy for patients with aggressive taxane-pretreated HER2-negative metastatic breast cancer: the MERIBEL study. Clin Breast Cancer (2019) 19:105–12. doi: 10.1016/j.clbc.2018.12.012
20. Tolaney SM, Guo H, Pernas S, Barry WT, Dillon DA, Ritterhouse L, et al. Seven-year follow-up analysis of adjuvant paclitaxel and trastuzumab trial for node-negative, human epidermal growth factor receptor 2–positive breast cancer. JCO (2019) 37:1868–75. doi: 10.1200/JCO.19.00066
21. Llombart-Cussac A, Cortés J, Paré L, Galván P, Bermejo B, Martínez N, et al. HER2-enriched subtype as a predictor of pathological complete response following trastuzumab and lapatinib without chemotherapy in early-stage HER2-positive breast cancer (PAMELA): an open-label, single-group, multicentre, phase 2 trial. Lancet Oncol (2017) 18:545–54. doi: 10.1016/S1470-2045(17)30021-9
22. König L, Dreyling M, Dürig J, Engelhard M, Hohloch K, Viardot A, et al. Therapy of nodal follicular lymphoma (WHO grade 1/2) in clinical stage I/II using response adapted involved site radiotherapy in combination with obinutuzumab (Gazyvaro) - GAZAI trial (GAZyvaro and response adapted involved-site radiotherapy): a study protocol for a single-arm, non-randomized, open, national, multi-center phase II trial. Trials (2019) 20:544. doi: 10.1186/s13063-019-3614-y
23. Sampayo-Cordero M, Miguel-Huguet B, Malfettone A, Pérez-García JM, Llombart-Cussac A, Cortés J, et al. The impact of excluding nonrandomized studies from systematic reviews in rare diseases: “The example of meta-analyses evaluating the efficacy and safety of enzyme replacement therapy in patients with mucopolysaccharidosis”. Front Mol Biosci (2021) 8:690615. doi: 10.3389/fmolb.2021.690615
24. Sampayo-Cordero M, Miguel-Huguet B, Pérez-García J, Páez D, Guerrero-Zotano ÁL, Garde-Noguera J, et al. Inclusion of non-inferiority analysis in superiority-based clinical trials with single-arm, two-stage simon’s design. Contemp Clin Trials Commun (2020). doi: 10.1016/j.conctc.2020.100678
25. Murray GD. Switching between superiority and non-inferiority. Br J Clin Pharmacol (2001) 52:219–9. doi: 10.1046/j.0306-5251.2001.01397.x
26. Committee For Proprietary medicinal products (CPMP). The European agency for the evaluation of medicinal products. points to consider on switching between superiority and non-inferiority (2000). Available at: http://www.ema.europa.eu/docs/en_GB/document_library/Scientific_guideline/2009/09/WC500003658.pdf.
27. Jardim DL, Groves ES, Breitfeld PP, Kurzrock R. Factors associated with failure of oncology drugs in late-stage clinical development: a systematic review. Cancer Treat Rev (2017) 52:12–21. doi: 10.1016/j.ctrv.2016.10.009
28. Monzon JG, Hay AE, McDonald GT, Pater JL, Meyer RM, Chen E, et al. Correlation of single arm versus randomised phase 2 oncology trial characteristics with phase 3 outcome. Eur J Cancer (2015) 51:2501–7. doi: 10.1016/j.ejca.2015.08.004
29. U.S. Department of Health and Human Services Food and Drug Administration, Center for Drug Evaluation and Research (CDER), Center for Biologics Evaluation and Research (CBER). Non-inferiority clinical trials to establish effectiveness. guidance for industry (2016). Available at: https://www.fda.gov/downloads/Drugs/Guidances/UCM202140.pdf.
30. Carles J, Alonso T, Mellado Gonzalez B, Mendez Vidal MJ, Vazquez Estevez S, González del Alba A, et al. 639P 223Ra in asymptomatic patients (pts) with metastatic castration-resistant prostate cancer (mCRPC) who progressed to first-line abiraterone acetate or enzalutamide. Ann Oncol (2020) 31:S525–6. doi: 10.1016/j.annonc.2020.08.898
31. Wu J, Pan H, Hsu C. Bayesian Single-arm phase II trial designs with time-to-event endpoints. Pharm Stat (2021) 20(6):1235–48. doi: 10.1002/pst.2143
32. Gion M, Pérez-García JM, Llombart-Cussac A, Sampayo-Cordero M, Cortés J, Malfettone A. Surrogate endpoints for early-stage breast cancer: a review of the state of the art, controversies, and future prospects. Ther Adv Med Oncol (2021) 13:175883592110595. doi: 10.1177/17588359211059587
33. Sparano JA, Gray RJ, Makower DF, Pritchard KI, Albain KS, Hayes DF, et al. Adjuvant chemotherapy guided by a 21-gene expression assay in breast cancer. New Engl J Med (2018) 379:111–21. doi: 10.1056/NEJMoa1804710
34. Jung S-H. Randomized phase II cancer clinical trials. Boca Raton: CRC Press/Taylor & Francis Group (2013). p. 229.
35. U.S. Department of Health and Human Services Food and Drug Administration, Center for Drug Evaluation and Research (CDER), Center for Biologics Evaluation and Research (CBER). Multiple endpoints in clinical trials - guidance for industry (2017). Available at: https://www.fda.gov/downloads/drugs/guidancecomplianceregulatoryinformation/guidances/ucm536750.pdf.
36. U.S. Department of Health and Human Services Food and Drug Administration, Center for Drug Evaluation and Research (CDER), Center for Biologics Evaluation and Research (CBER). Clinical trial endpoints for the approval of cancer drugs and biologics - guidance for industry (2018). Available at: https://www.fda.gov/regulatory-information/search-fda-guidance-documents/clinical-trial-endpoints-approval-cancer-drugs-and-biologics.
37. Time to event data analysis. Columbia public health. Available at: https://www.publichealth.columbia.edu/research/population-health-methods/time-event-data-analysis (Accessed January 26, 2021).
38. Chang M. Introductory adaptive trial designs: a practical guide with r. s.l. United States: CRC PRESS (2019).
39. Thorlund K, Dron L, Park JJ, Mills EJ. Synthetic and external controls in clinical trials – a primer for researchers. CLEP (2020) 12:457–67. doi: 10.2147/CLEP.S242097
40. Lackey L, Thompson G, Eggers S. FDA’s benefit–risk framework for human drugs and biologics: role in benefit–risk assessment and analysis of use for drug approvals. Ther Innov Regul Sci (2021) 55:170–9. doi: 10.1007/s43441-020-00203-6
41. Parker RA, Weir CJ. Non-adjustment for multiple testing in multi-arm trials of distinct treatments: rationale and justification. Clin Trials (2020) 17:562–6. doi: 10.1177/1740774520941419
42. Kwak M, Jung S-H. Phase II clinical trials with time-to-event endpoints: optimal two-stage designs with one-sample log-rank test. Statist Med (2014) 33:2004–16. doi: 10.1002/sim.6073
43. Sample size calculator | kengo nagashima - the institute of statistical mathematics. Available at: https://nshi.jp/en/js/ (Accessed January 26, 2021).
44. Statistic tools - SWOG cancer research network . Available at: https://stattools.crab.org/index.html (Accessed January 26, 2021).
45. Wu J. Single-arm phase II survival trial design under the proportional hazards model. Stat Biopharmaceutical Res (2017) 9:25–34. doi: 10.1080/19466315.2016.1174147
46. Neuenschwander B, Rouyrre N, Hollaender N, Zuber E, Branson M. A proof of concept phase II non-inferiority criterion. Stat Med (2011) 30:1618–27. doi: 10.1002/sim.3997
48. Zhong W, Koopmeiners JS, Carlin BP. A trivariate continual reassessment method for phase I/II trials of toxicity, efficacy, and surrogate efficacy. Stat Med (2012) 31:3885–95. doi: 10.1002/sim.5477
Keywords: time-to-event, non-inferiority, single-arm, phase II, clinical trial, superiority
Citation: Sampayo-Cordero M, Miguel-Huguet B, Malfettone A, López-Miranda E, Gion M, Abad E, Alcalá-López D, Pérez-Escuredo J, Pérez-García JM, Llombart-Cussac A and Cortés J (2023) A single-arm study design with non-inferiority and superiority time-to-event endpoints: a tool for proof-of-concept and de-intensification strategies in breast cancer. Front. Oncol. 13:1048242. doi: 10.3389/fonc.2023.1048242
Received: 19 September 2022; Accepted: 19 June 2023;
Published: 11 July 2023.
Edited by:
Paula R. Pohlmann, University of Texas MD Anderson Cancer Center, United StatesReviewed by:
Ning Zhang, Shandong University, ChinaCopyright © 2023 Sampayo-Cordero, Miguel-Huguet, Malfettone, López-Miranda, Gion, Abad, Alcalá-López, Pérez-Escuredo, Pérez-García, Llombart-Cussac and Cortés. This is an open-access article distributed under the terms of the Creative Commons Attribution License (CC BY). The use, distribution or reproduction in other forums is permitted, provided the original author(s) and the copyright owner(s) are credited and that the original publication in this journal is cited, in accordance with accepted academic practice. No use, distribution or reproduction is permitted which does not comply with these terms.
*Correspondence: Miguel Sampayo-Cordero, c2FtcGF5by5tY0BnbWFpbC5jb20=
Disclaimer: All claims expressed in this article are solely those of the authors and do not necessarily represent those of their affiliated organizations, or those of the publisher, the editors and the reviewers. Any product that may be evaluated in this article or claim that may be made by its manufacturer is not guaranteed or endorsed by the publisher.
Research integrity at Frontiers
Learn more about the work of our research integrity team to safeguard the quality of each article we publish.