- 1Guangdong Provincial Key Laboratory of Digestive Cancer Research, The Seventh Affiliated Hospital of Sun Yat-sen University, Shenzhen, Guangdong, China
- 2School of Nursing, Shandong First Medical University Shandong Academy of Medical Sciences, Jinan, Shandong, China
- 3Department of Gastrointestinal Surgery, The First Affiliated Hospital of Sun Yat-Sen University, Guangzhou, Guangdong, China
Background: Although the overall global incidence of gastric cancer has been declining, the number of new cases in people under the age of 50 is increasing, which is related to metastasis, late pathological stages, and poor prognosis. There is a scarcity of large-scale studies to evaluate and predict distant metastasis in patients with early-onset gastric cancer.
Methods: From January 2010 to December 2019, data on early-onset GC patients undergoing surgery were gathered from the Surveillance, Epidemiology, and End Results (SEER) database. We investigated the independent risk factors for distant metastasis in patients with early-onset gastric cancer. Based on these risk factors, we developed a nomogram to predict distant metastasis. The model underwent internal validation on the test set and external validation on 205 patients from the First Affiliated Hospital of Sun Yat-sen University and the seventh Affiliated Hospital of Sun Yat-sen University. The novel nomogram model was then evaluated using the receiver operating characteristic (ROC) curve, calibration, the area under the curve (AUC), and decision curve analysis (DCA). The training set nomogram score was used to classify the different risk clusters of distant metastasis.
Results: Our study enrolled 2217 patients after establishing the inclusion and exclusion criteria, with 1873 having no distant metastasis and 344 having distant metastasis. The tumor size, total lymph nodes, whether or not receiving radiotherapy and chemotherapy, T stage, and N stage were significant predictors of advanced distant metastasis (p < 0.05). The AUC of the ROC analysis demonstrated our model’s high accuracy. Simultaneously, the prediction model shows high stability and clinical practicability in the calibration curve and DCA analysis.
Conclusions: We developed an innovative nomogram containing clinical and pathological characteristics to predict distant metastasis in patients younger than 50 years old with gastric cancer. The tool can alert clinicians about distant metastasis and help them develop more effective clinical treatment plans.
Introduction
Despite regular screening and advanced treatment effectively reducing gastric cancer (GC) mortality, GC still ranks fourth in the cause of cancer deaths worldwide (1). The risk factors closely related to gastric cancer include Helicobacter pylori infection, smoking, drinking, low income, high salt intake, family tendency, previous history of gastric surgery, and pernicious anemia (2). Under the influence of various environmental and genetic factors, gastric cancer mainly occurs in middle-aged and elderly patients aged 50-70. However, it is relatively uncommon in the younger population (under 50 years old) (2, 3), which is known as early-onset GC (4, 5). The Surveillance Epidemiology and End Results (SEER) program recently revealed a significant rise in the incidence of early-onset GC in both female and male patients (6). Young patients are more likely to ignore clinical manifestations because the early symptoms are not obvious. Meanwhile, studies have shown that younger patients in various cancers have more distant metastasis (DM) and poorer outcomes than older patients (7, 8). Nevertheless, many GC patients are typically diagnosed at an advanced or metastatic stage, indicating a dismal 5-year survival rate of less than 30% (9). In early-onset GC, it is critical to accurately evaluate and predict metastasis status for treatment choice and prognostic assessment.
This study sought to investigate the clinicopathological traits and prognostic factors of DM patients with early-onset GC who were under 50 years old. In this retrospective analysis, 2217 patients from the SEER database diagnosed with early-onset GC were assigned randomly to the training and test sets. Using logistic regression analysis, we discovered the clinicopathological factors influencing the DM of early-onset GC. Then, utilizing these risk factors, we developed a nomogram to optimize the precision of predicting DM in early-onset GC. Our center’s early-onset GC patients served as validation subjects for the model. The findings indicate that our model can accurately predict the DM of early-onset GC.
Materials and methods
Clinical information
We downloaded and filtered the clinical data of GC individuals diagnosed between 2010 and 2019 using the software seer*stata 8.4.0. The following criteria were used to exclude patients: (1) Patients with carcinoma in situ or without TNM staging data, according to the 7th American Joint Commission on Cancer (AJCC). (2) Patients who have been diagnosed with “autopsy only” or “death certificate only.” (3) Patients whose primary disease site is not the stomach (4) Patients who have not received surgery (5) Patients suffering from neuroendocrine and gastrointestinal stromal tumors (6) Patients without follow-up information. The flow chart of this retrospective study was shown in Figure 1. We analyzed and compared the age, gender, race, marital status, location of the tumor, tumor differentiation, tumor stage, tumor diameter, chemo and radiation, the total number of lymph nodes acquired, overall survival (OS), and cancer-specific survival (CSS) between groups with different metastatic status.
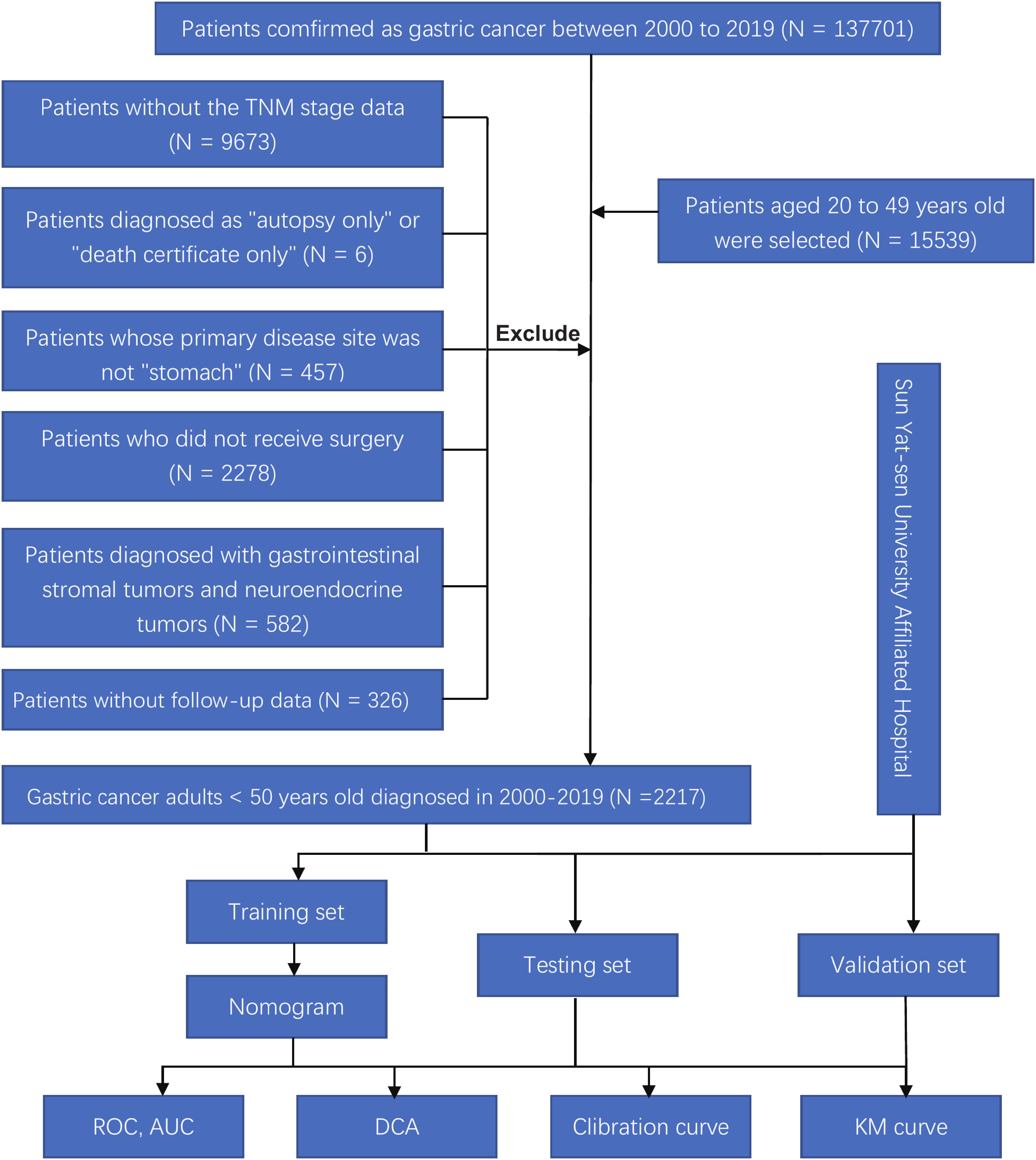
Figure 1 Flow chart of this study. ROC, receiver operating characteristic; AUC, area under the curve; DCA, and decision curve analysis; KM curve, Kaplan-Meier curve.
After identifying 2217 patients, they were arbitrarily split into a training and an internal test set in the proportion of 6:4. External validation of the model was performed on 205 early-onset GC patients from the First Affiliated Hospital of Sun Yat-sen University and the seventh Affiliated Hospital of Sun Yat-sen University. The ethical committees of the first and seventh Affiliated Hospital of Sun Yat-sen University approved this study.
Risk factor analysis and prediction model development
We investigated the risk factors for DM in early-onset GC patients. First, we utilized the Kaplan-Meier method to assess the impact of DM on the OS and CSS of the selected cases in the database and our follow-up cases. The chi-square test and logistic regression were then performed to investigate the potential risk factors for DM in patients with early-onset GC. After that, in the training set, a logistic regression nomogram was established and validated in the test and validation sets to predict the probability of DM. By plotting the ROC curve, we measured the model’s sensitivity and specificity to estimate patients’ OS and then obtained the AUC to evaluate the model’s accuracy. The calibration curve was then drawn to assess the reliability between the expected and observed outcomes of the nomogram. Meanwhile, we evaluated the clinical efficacy of the model using DCA curves. Next, we calculated the scores of all the patients in the training sets using the nomogram, classified them as low or high risk, and examined the OS of patients in each risk group. Finally, we applied the scoring system to our external validation set to test the clinical applicability of the model further and observed whether the scoring system was applicable in clinical practice in the inter-group comparison between subgroups with different clinical-pathological characteristics.
Statistical evaluation
All statistical analyses in this study were conducted with R version 4.1.0. Categorical variables were represented numerically with percentages. The Chi-square test was utilized to compare variables of pathological characteristics. The survival of patients with or without DM was analyzed using Kaplan-Meier curves. To identify the independent risk factors of DM, Variables with a p-value < 0.1 in the univariate logistic regression analysis were included in the multivariate logistic regression analysis to determine the risk factors for DM in early-onset GC. The nomogram and calibration curve are generated with the R package “rms.” The “pROC” package was used to draw the ROC curve and obtain the specific value of AUC. The “rmda” package was used to plot a DCA curve to describe the nomogram model’s clinical performance compared to the single factors. A p-value < 0.05 (two‐tailed) was deemed statistically significant in all statistical calculations.
Results
Baseline characteristics of patients
In the SEER database, 2217 early-onset GC patients who had undergone surgery were screened, 344 of whom developed DM. Table 1 summarizes the general characteristics of all patients. Age, gender, primary site, tumor size, whether radiation or chemotherapy was utilized, and the total number of lymph nodes removed during surgery varied statistically between the two groups (p < 0.05). Following that, patients were randomly assigned to two groups: a training group of 1330 individuals and an internal test group of 887 individuals. As shown in Table 2, demographic and pathological disparity were not statistically significant. Then, we gathered 205 early-onset GC patients from the First Affiliated Hospital of Sun Yat-sen University and the seventh Affiliated Hospital of Sun Yat-sen University as an external validation set to ensure the study’s universality. Table S1 shows the basic features of these patients, 39 of whom had DM. The SEER database and our validation cohort exhibited a shorter OS for patients with DM, as indicated by the Kaplan Meier curve (Figures 2A, B). The DM patients also had significantly lower CCS (Figure S1). At the same time, we examined the effect of different distant metastasis states on the prognosis of different T (Figures 2C–F) and N (Figures 2G–J) stages.
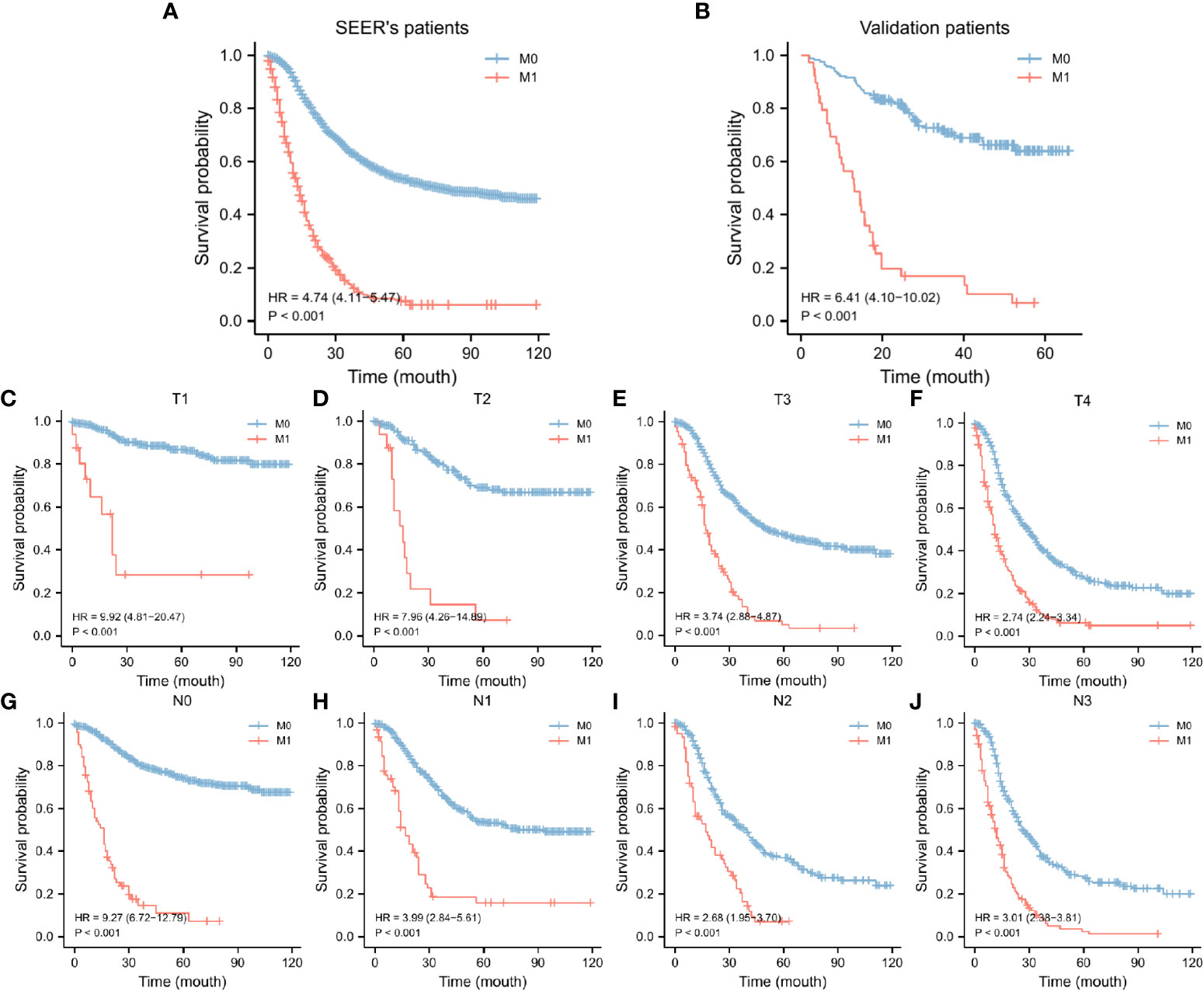
Figure 2 KM curves of OS for GC in the SEER data set (A) and our validation set (B), KM curves of OS for GC in the T stages (C-F) and N stages (G-J). SEER, Surveillance, Epidemiology, and End Results database; OS, overall survival; GC, gastric cancer; KM curve, Kaplan-Meier curve.
Independent risk factors of DM in patients with early-onset GC
Univariate logistic regression analysis of the training set revealed that DM was significantly correlated with sex, primary site, tumor size, number of lymph nodes cleaned during surgery, and whether chemotherapy or radiation was used (Table 3; Figure 3A). These variables were considered in the subsequent multivariate logistic regression analysis (Table 3; Figure 3B). T4 patients (OR = 5.462, 95% CI = 3.055-10.198, p < 0.001) and Tx patients (OR = 3.793, 95% CI = 1.693-8.557, p = 0.007) were more likely to develop DM than T1 patients. Patients in the N3 stage were more likely than those in the N0 stage to have DM (OR = 3.049, 95% CI = 1.964-4.781, p < 0.001). Patients with tumors larger than 100 mm had an increased risk of developing DM (OR = 2.47, 95% CI = 1.421-4.264, p = 0.007) compared to those with tumors smaller than 50 mm. If more lymph nodes are removed during surgery, patients with GC have a lower risk of developing DM. Intriguingly, we discovered that radiotherapy in conjunction with a surgical procedure (OR =0.299, 95% CI = 0.211-0.418, p = 0.001) was a protective factor for DM of early-onset GC. Additionally, chemotherapy treatment was a significant risk factor (OR = 1.764, 95% CI = 1.2-2.619, p = 0.017).
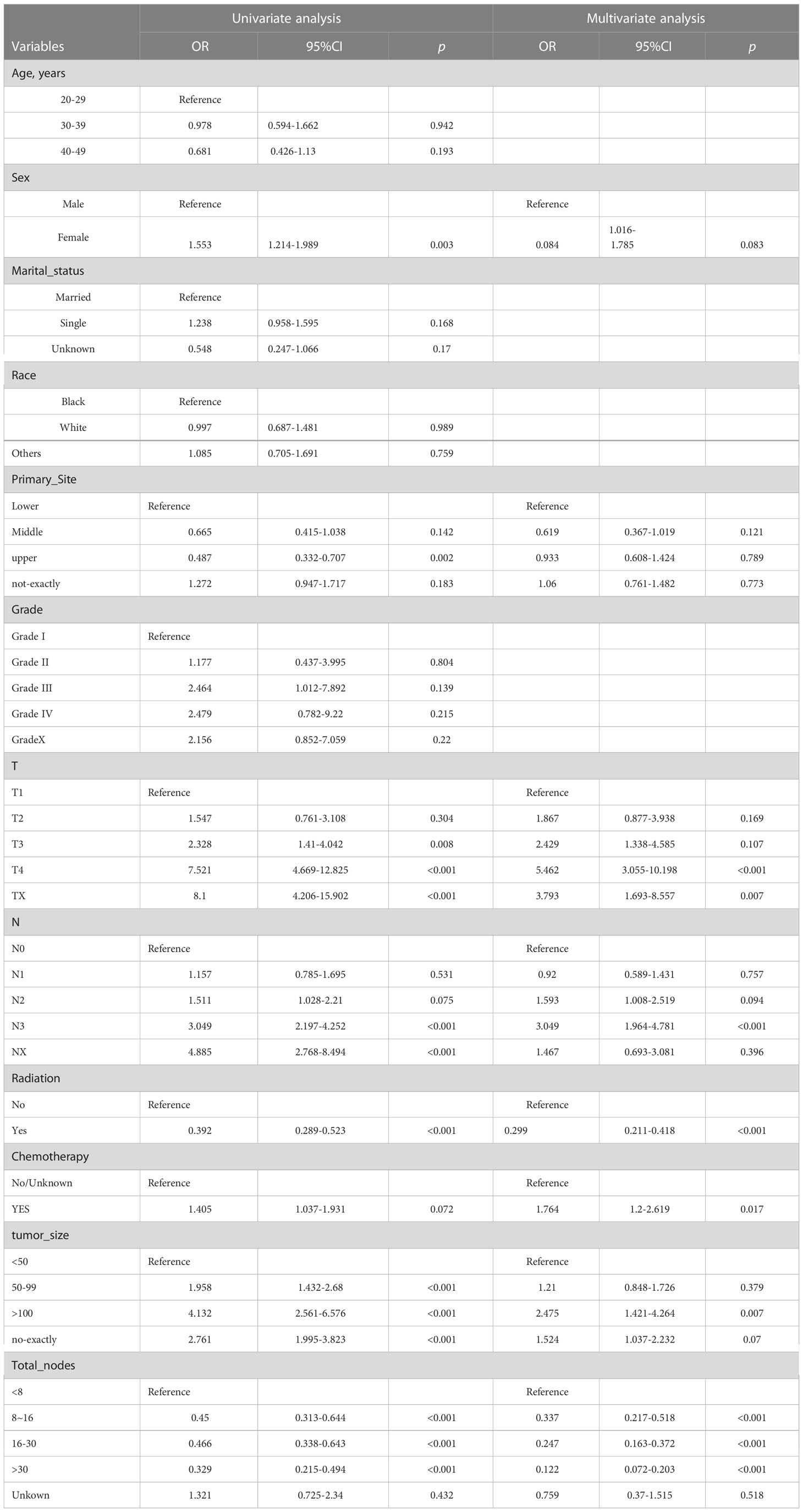
Table 3 Univariate and multivariate logistic regression analyses of distant metastasis in early-onset gastric cancer from training set.
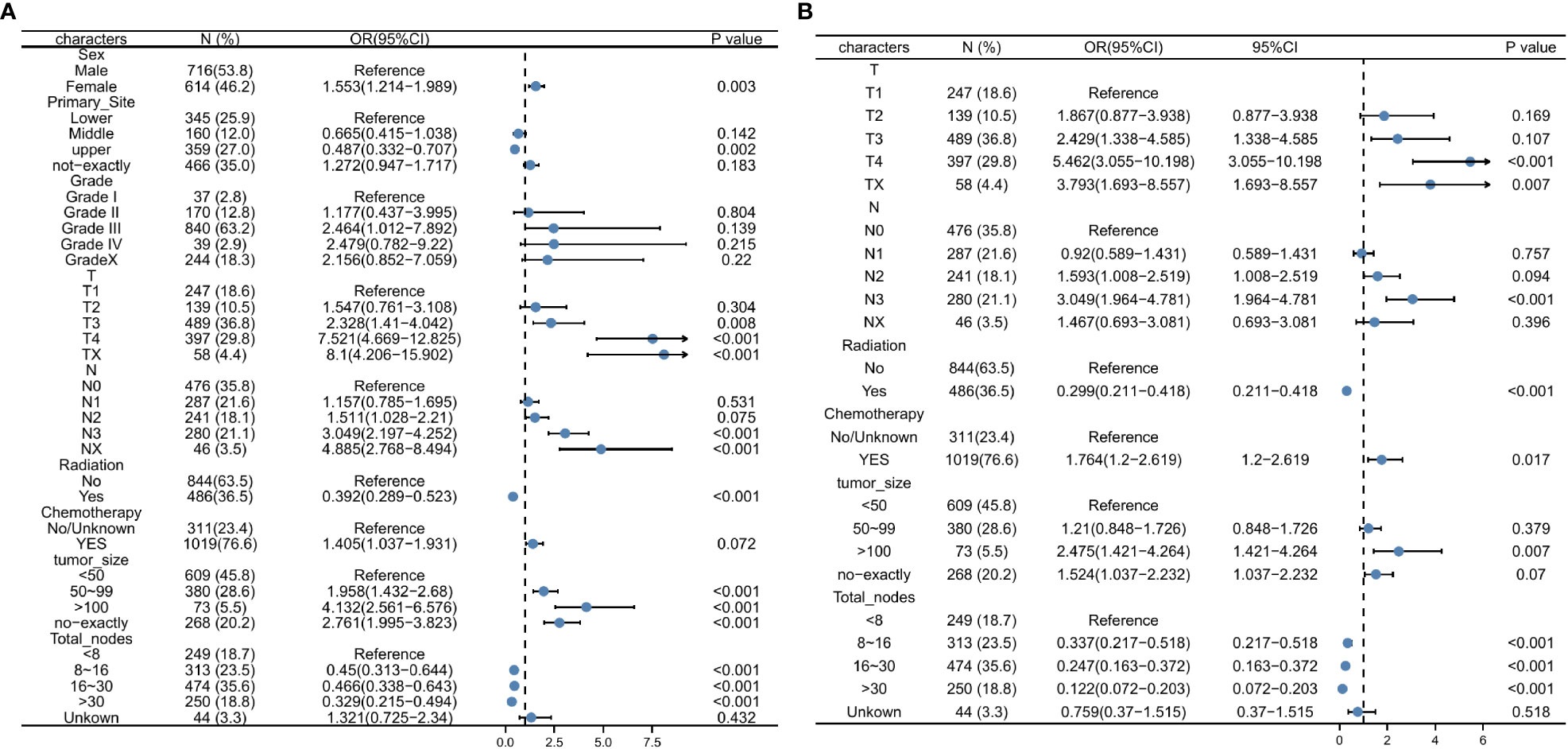
Figure 3 Univariate logistic regression analysis for predicting the DM (A), Multivariate logistic regression analysis for predicting the DM (B). DM, distant metastasis.
Development and validation of a nomogram for predicting DM in early-onset GC
A nomogram was developed containing independent influencing factors identified through multiple logistic regression to predict DM in early-onset GC (Figure 4). Using the “pROC” package, we draw the ROC curves for the training and validation sets to test the model’s accuracy (Figures 5A–C). The AUC of our model in the training set was 0.827, while it was 0.793 and 0.775 in the internal test and external validation sets, indicating good prediction accuracy. Next, the calibration curves of the nomogram for evaluating the consistency of the expected and observed outcomes of DM revealed a high level of consistency between the nomogram-predicted overall survival and the actual result (Figures 5D–F). Finally, using the DCA analysis, we compared the nomogram model’s clinical value to that of various tumor clinicopathological characteristics (such as tumor size, T stage, and N stage) and found that the nomogram’s value was higher (red line in Figures 5G–I).
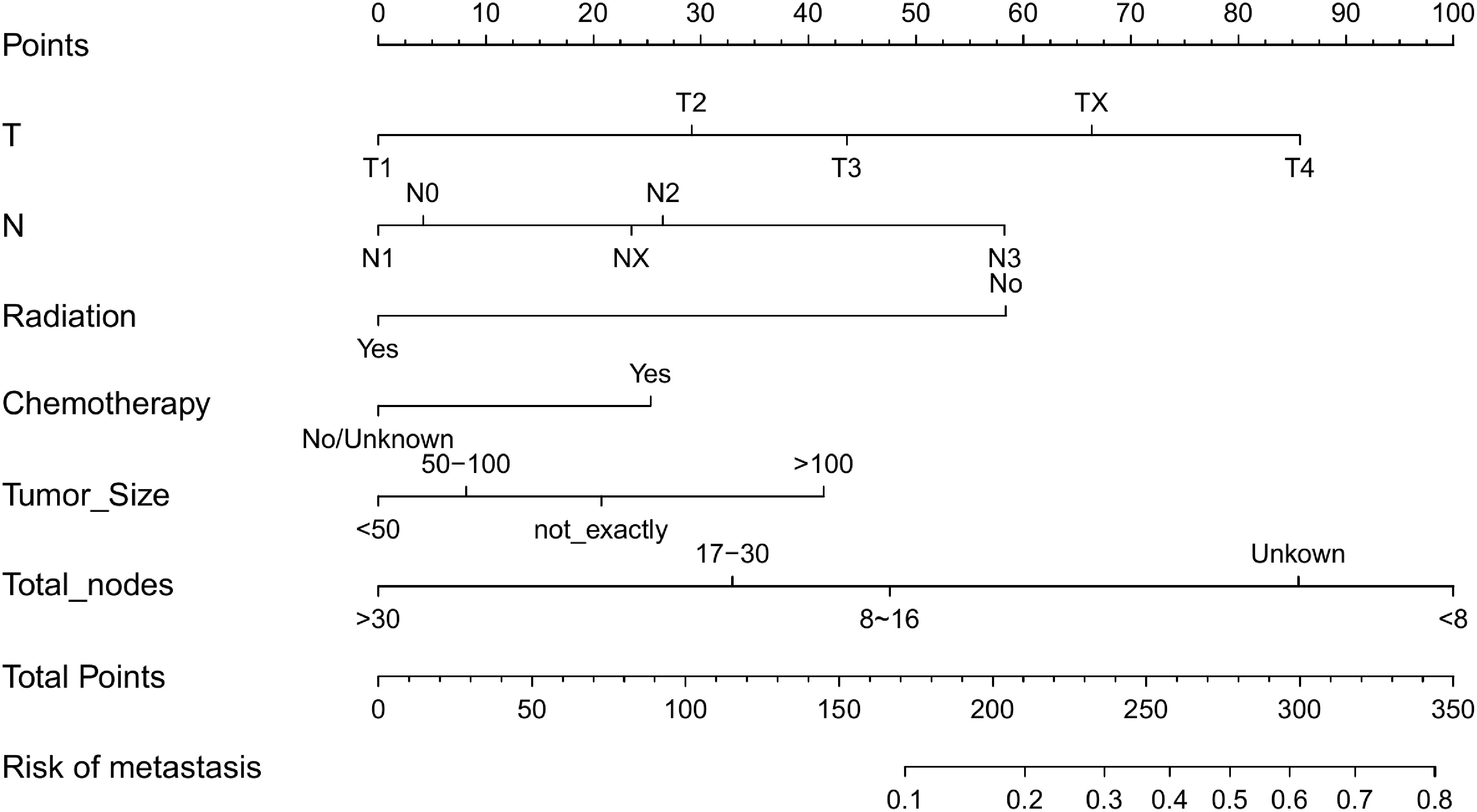
Figure 4 Nomogram for predicting DM in the training set of early-onset GC(C). DM, distant metastasis; GC, gastric cancer.
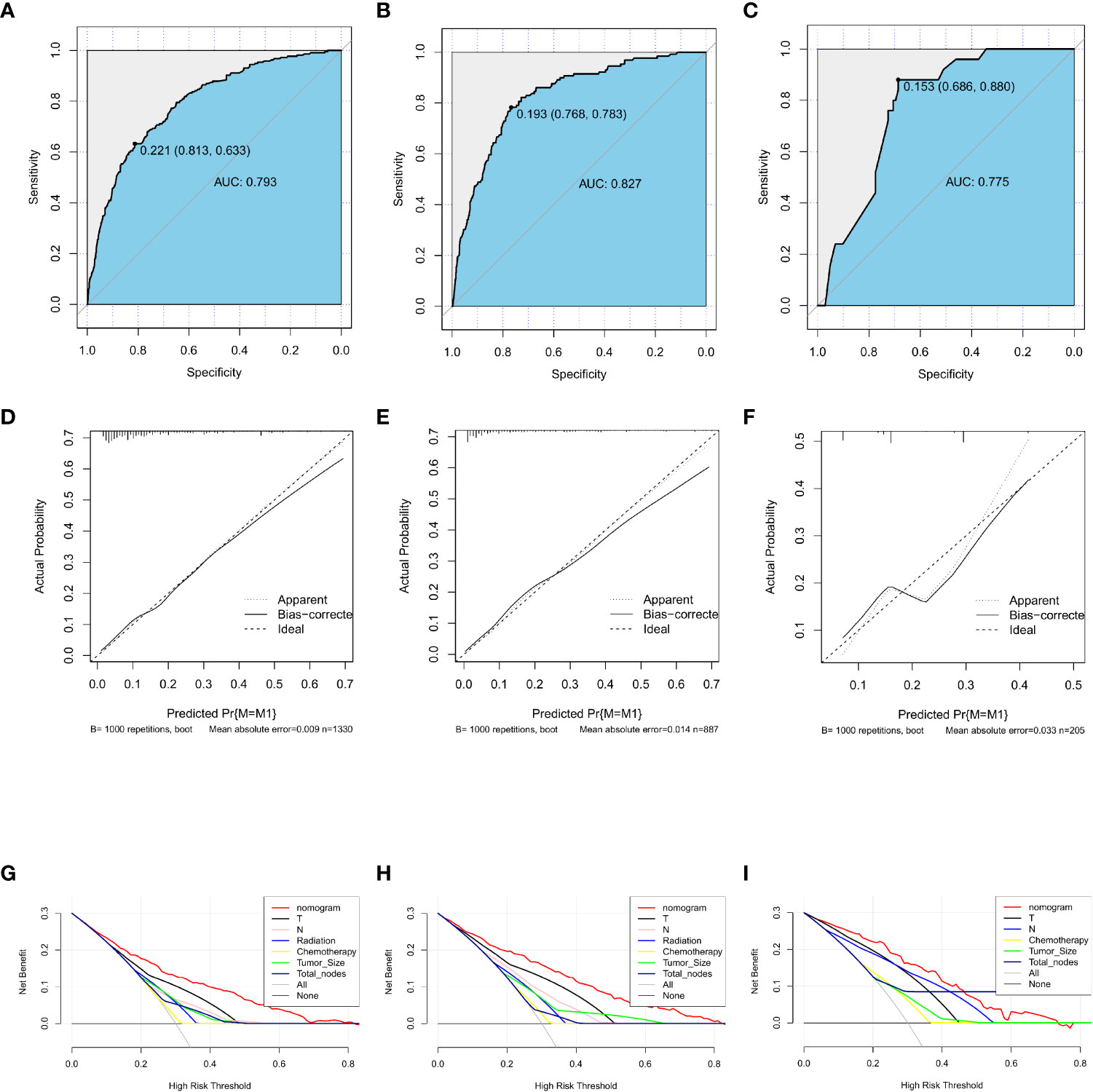
Figure 5 Training, testing, and validation sets of receiver operating characteristic (ROC) curves (A–C), calibration curves (D–F), and discriminant curve analyses (DCA) (G–I) for early-onset GC. GC, gastric cancer.
Survival of patients with various nomogram scores
According to the nomogram, we extracted the patients from the training set again and then measured the risk scores for each GC patient. Table 4 displays the risk scores associated with each clinicopathological variable. On the basis of the median of their total score, all patients were divided into two categories: low-risk (score < 174) and high-risk (score >= 174). We discovered that as the risk score increased, the likelihood of DM in patients with early-stage GC increased as well (Figure 6A). An increased risk score of DM in patients with early-onset GC was closely related to a worse prognosis, as shown by the Kaplan-Meier curve, which depicted the relationship between risk classification and prognosis (Figure 6B). The test and validation sets analysis confirmed the same result (Figures 6C, D).
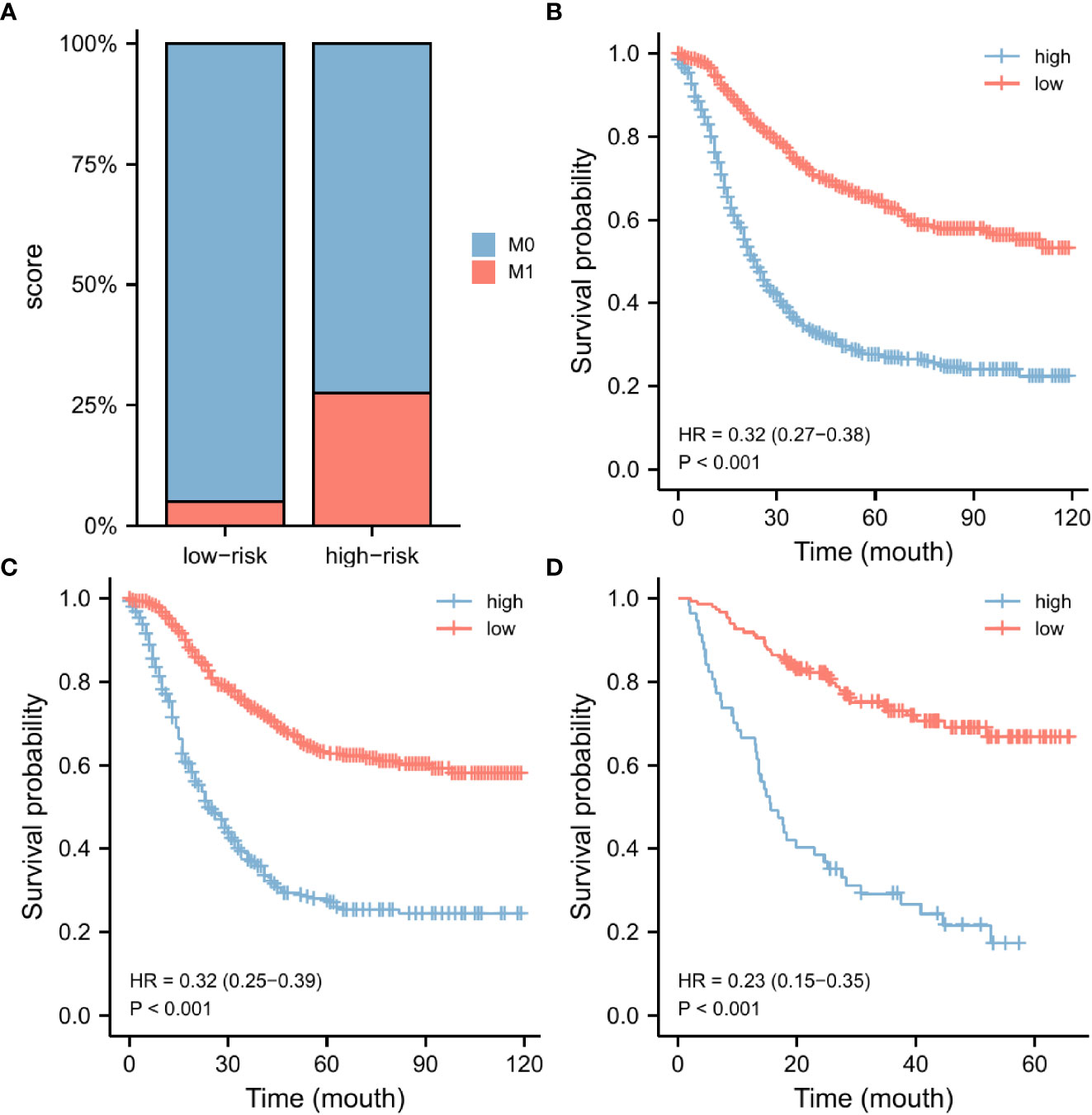
Figure 6 Nomogram risk score clinical application. According to the median risk score, our nomogram divides GC patients into two subgroups. There were various numbers of distant metastases in each subgroup (A). The OS was evaluated in the training set (B), the testing set (C), and the external validation set (D). GC, gastric cancer; OS, overall survival.
Clinical and pathological differences in patients with different nomogram scores
To further explore the applicability of nomogram scores in clinical practice, we scored 205 patients with early-onset gastric cancer in our cohort according to the scoring principles of the training set. We analyzed the correlation between their scoring results and clinical-pathological factors. In the chi-square test of the case T stage, we found that patients with high scores were more likely to be in T3 and T4 stages (p < 0.001) (Figure 7A), and similarly, patients with N3 stage were more likely to obtain higher risk scores (Figure 7B). In Comparing different subgroups, higher T and N stages also have higher risk scores (Figures 7C, D). As an essential factor in the pathological characteristics of tumors, we used the same method to analyze the tumor size and the total number of lymph node dissections. The results showed that larger tumor diameter and fewer lymph node dissections could lead to higher scores (Figures S2A, B). In comparison, smaller tumor diameters and more lymph node dissections were often closely related to patients’ low-risk scores. (Figures S2C, D).
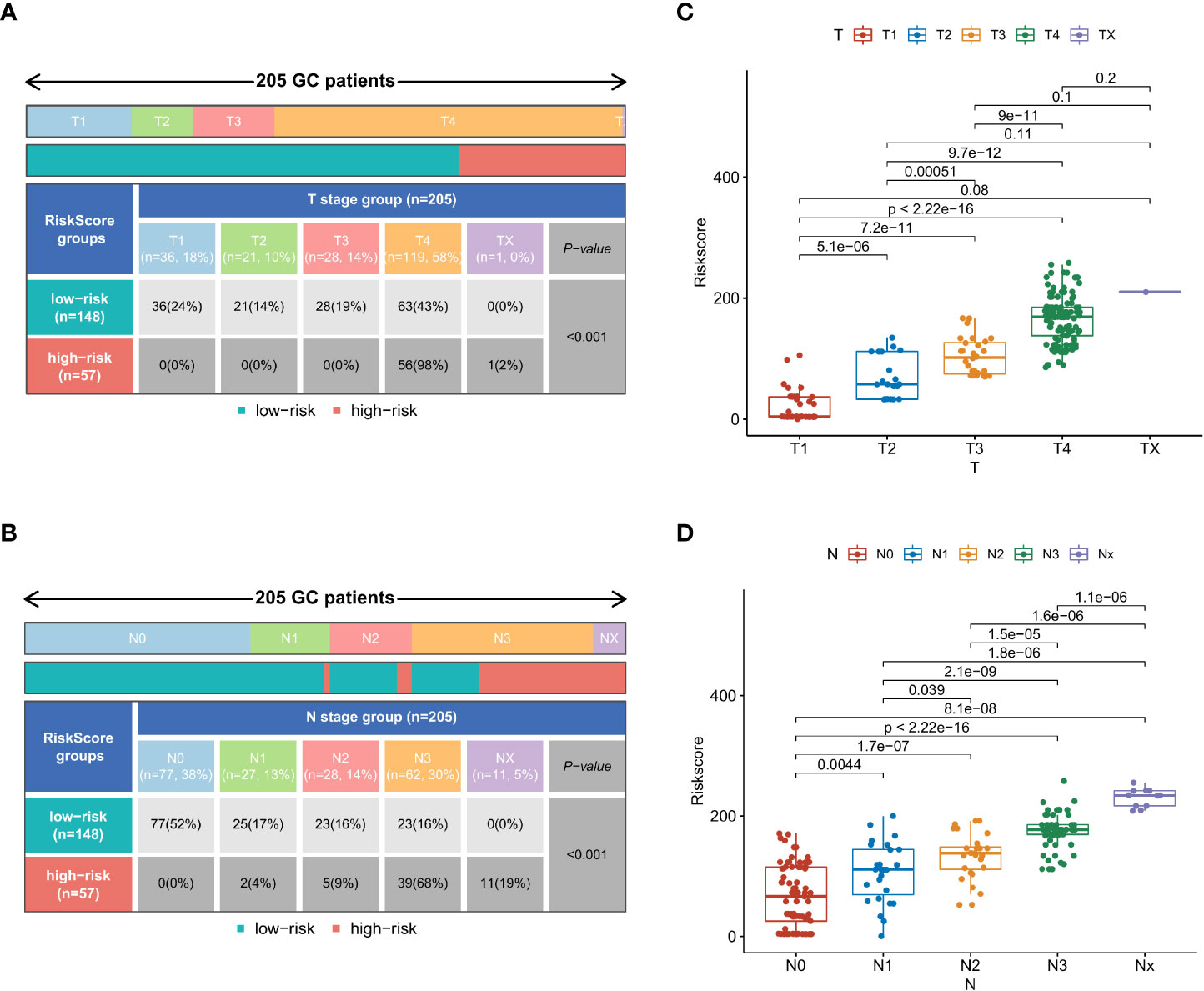
Figure 7 In the external validation set, the chi-square test results of risk stratification patients with different T stages (A) and N stages (B). Comparison of risk scores between different subgroups in T-stage (C) and N-stage (D).
Discussion
When compared to elderly patients, young patients are thought to have a poor prognosis for GC (10). Early-onset GC has a poor prognosis due to undifferentiated histology, unresectable lymphatic vascular infiltration, and late-stage diagnosis (11, 12). In reality, DM is the most important cause of therapeutic failure in the majority of malignant tumors. Most patients have locally advanced or DM when diagnosed with GC for the first time. The presence of DM has a direct impact on the treatment options available to patients as well as their overall prognosis. As a result, it is critical to comprehend the clinicopathological features of DM in GC. However, no research has been conducted on the risk factors for DM in GC under the age of 50. Many studies have shown that surgical resection combined with other systemic therapies could significantly improve the prognosis for patients with distantly metastatic GC (13). Therefore, we evaluated the potential risk factors for DM in early-onset GC and established a nomogram based on the clinical and pathological characteristics of the tumor to predict DM.
GC occurs at different rates in men and women. Men had a substantially higher incidence rate than women, and there was evidence that women had a favorable prognosis than men for gastric cancer (14). Interestingly, in our study, the metastatic rate of women with early-onset gastric cancer tends to be higher than that of men, because it was close to a significant value in the statistical calculation (p = 0.083). Gastric cancer is classified into two types based on its anatomical location: cardia GC and non-cardia GC. Non-cardia GC has a better prognosis and is frequently associated with Helicobacter pylori infection (15). However, our study found that the tumor location of patients with early-onset GC does not affect whether cancer has DM (p>0.05), which may need to be further confirmed in clinical studies at a more evidential level.
In addition, this study showed that in patients with early-onset GC, the size of the tumor, the total number of lymph nodes removed during surgery, the T4 and N3 tumor stages, and therapeutic interventions without radiation and chemotherapy were associated with the development of DM after surgery. Tumor grade, primary tumor diameter, T stage, and N stage were found to be predictors of synchronous DM in GC by Zikai Lin et al. (16). In accordance with the prior study, and we also included the size of the tumor, T stage, and N stage in our model. In our study, demographic factors such as age and gender were not defined as DM risk factors.
Furthermore, our study discovered a correlation between a larger tumor size and DM in early-onset GC. Previous research identified a tumor diameter greater than 2.5cm as an independent prognostic factor for patients with gastric signet ring cell carcinoma (17). Jingyu Chen et al. established a DM prediction model for superficial GC with no lymph node metastasis based on tumor diameter and other factors, they believed that a tumor larger than 2cm was a risk factor for DM of GC (18). Different target populations may result in different tumor diameters. We discovered that patients with tumor tissues larger than 10 cm had a greater risk of developing distant metastasis. Therefore, these patients require special attention. According to Qian Huang et al., early-onset GC is associated with diffuse Lauren type and poorly differentiated tumors (19). Surprisingly, after adjusting for other variables, the degree of tumor differentiation was eventually excluded from our prediction nomogram. We must recognize the clinical significance of tumor cell differentiation in early-onset GC completely because it has been defined as one of the most important features of GC patients in various studies.
Chemotherapy improved the survival and quality of life in patients with locally advanced gastric cancer (20). Patients with GC who received combined chemotherapy had a one-year median overall survival time (the survival time for Asian patients was often slightly longer). In contrast, patients receiving supportive treatment alone had a survival time of 3-4 months (21–23). It also explained that in our study, the majority of patients with DM and early-onset GC received chemotherapy. In contrast, randomized controlled trials have demonstrated that radiotherapy does not affect the overall survival of patients undergoing quality-assured D1 and D2 gastrectomy (24, 25). Patients with less than D1 + or D2 lymphadenectomy or R1 resection, on the other hand, can benefit from postoperative chemoradiotherapy (26). This is why our model considers radiotherapy to be a protective factor.
The nomogram is a useful and practical prediction tool that is widely used in research. Jie Cheng and colleagues developed a model to predict DM in patients with early-onset colorectal cancer (27). This approach was also widely used in the treatment of other cancers, such as lung (28) and esophageal cancer (29). However, most studies were unable to extend the risk model’s external validation. As a result, they cannot assess the model’s accuracy in actual clinical work. The prediction model developed in this study was evaluated using a similar internal validation set to the training set and the external validation set from two Chinese medical centers for GC, and we used the ROC curve, calibration curve, and DCA curve to verify our model’s accuracy, consistency, and clinical applicability. Finally, all patients were categorized as low-risk (score < 174) or high-risk (score >= 174) according to the risk score. The prognosis of the two groups differed significantly. The findings indicate that our model was trustworthy and could provide clinicians with additional information. Therefore, close supervision of DM should be considered for patients with early-onset GC who have a large tumor size, few lymph nodes discovered, tumor stages T4 or N3, and surgery combined with chemotherapy or without radiation. However, the limitation of our prediction model is that our follow-up sample size is not large enough. The accuracy and applicability of this model need to be verified in the follow-up data with a larger sample size.
In conclusion, our study found several independent risk factors for DM in people with GC who was younger than 50 years old. We also developed a nomogram that can predict DM in people with early-onset GC. The model’s prediction performance was good in both internal and external validation. As a result, they may be able to aid clinicians in predicting the progression of the disease and developing appropriate treatments.
Data availability statement
The original contributions presented in the study are included in the article/Supplementary Material, further inquiries can be directed to the corresponding author/s.
Ethics statement
Ethical approval was obtained from the Medical Ethics Committee of the Seventh affiliated Hospital of Sun Yat-sen University (No: KY-2020-024-01). All patient records and information were anonymized and deidentified prior to analysis.
Author contributions
All authors contributed to the study’s conception and design. Y-LH and BB conceived the research. BB and G-FD performed material preparation, data collection, and analysis. BB and Y-MD wrote the first draft of the manuscript. Z-JH and X-YC participated in the revision of the article and the polishing of the language. Y-LH and C-HZ reviewed and edited the manuscript. All authors contributed to the article and approved the submitted version.
Funding
Guangdong Provincial Key Laboratory of Digestive Cancer Research (No. 2021B1212040006). Sanming Project of Medicine in Shenzhen (No. SZSM201911010).
Acknowledgments
The authors appreciate the SEER database for providing open access for valuable data. Finally, we want to express our special thanks to Dr. He for his constant advice.
Conflict of interest
The authors declare that the research was conducted in the absence of any commercial or financial relationships that could be construed as a potential conflict of interest.
Publisher’s note
All claims expressed in this article are solely those of the authors and do not necessarily represent those of their affiliated organizations, or those of the publisher, the editors and the reviewers. Any product that may be evaluated in this article, or claim that may be made by its manufacturer, is not guaranteed or endorsed by the publisher.
Supplementary material
The Supplementary Material for this article can be found online at: https://www.frontiersin.org/articles/10.3389/fonc.2023.1003977/full#supplementary-material
References
1. Sung H, Ferlay J, Siegel RL, Laversanne M, Soerjomataram I, Jemal A, et al. Global cancer statistics 2020: GLOBOCAN estimates of incidence and mortality worldwide for 36 cancers in 185 countries. CA: Cancer J Clin (2021) 71(3):209–49. doi: 10.3322/caac.21660
2. Lordick F, Carneiro F, Cascinu S, Fleitas T, Haustermans K, Piessen G, et al. Gastric cancer: ESMO clinical practice guideline for diagnosis, treatment and follow-up. Ann oncol: Off J Eur Soc Med Oncol (2022) 33(10):1005–20. doi: 10.1016/j.annonc.2022.07.004
3. Isobe Y, Nashimoto A, Akazawa K, Oda I, Hayashi K, Miyashiro I, et al. Gastric cancer treatment in Japan: 2008 annual report of the JGCA nationwide registry. Gastric cancer: Off J Int Gastric Cancer Assoc Japanese Gastric Cancer Assoc (2011) 14(4):301–16. doi: 10.1007/s10120-011-0085-6
4. Sung H, Siegel RL, Rosenberg PS, Jemal A. Emerging cancer trends among young adults in the USA: Analysis of a population-based cancer registry. Lancet Public Health (2019) 4(3):e137–e47. doi: 10.1016/S2468-2667(18)30267-6
5. Ugai T, Sasamoto N, Lee HY, Ando M, Song M, Tamimi RM, et al. Is early-onset cancer an emerging global epidemic? Current evidence and future implications. Nat Rev Clin Oncol (2022) 19(10):656–73. doi: 10.1038/s41571-022-00672-8
6. Kulig J, Popiela T, Kolodziejczyk P, Sierzega M, Jedrys J, Szczepanik AM. Clinicopathological profile and long-term outcome in young adults with gastric cancer: Multicenter evaluation of 214 patients. Langenbeck’s Arch surg (2008) 393(1):37–43. doi: 10.1007/s00423-007-0208-z
7. Azim HA Jr., Partridge AH. Biology of breast cancer in young women. Breast Cancer research: BCR (2014) 16(4):427. doi: 10.1186/s13058-014-0427-5
8. Jin X, Zhao X, Liu X, Han K, Lu G, Zhang Y. Non-small cell lung cancer in young patients: An analysis of clinical, pathologic and TNM stage characteristics compared to the elderly. Risk Manage healthcare policy (2020) 13:1301–7. doi: 10.2147/RMHP.S264274
9. Wadhwa R, Song S, Lee JS, Yao Y, Wei Q, Ajani JA. Gastric cancer-molecular and clinical dimensions. Nat Rev Clin Oncol (2013) 10(11):643–55. doi: 10.1038/nrclinonc.2013.170
10. Murphy G, Dawsey SM, Engels EA, Ricker W, Parsons R, Etemadi A, et al. Cancer risk after pernicious anemia in the US elderly population. Clin Gastroenterol hepatol: Off Clin Pract J Am Gastroenterological Assoc (2015) 13(13):2282–9.e1-4. doi: 10.1016/j.cgh.2015.05.040
11. Nakamura R, Saikawa Y, Takahashi T, Takeuchi H, Asanuma H, Yamada Y, et al. Retrospective analysis of prognostic outcome of gastric cancer in young patients. Int J Clin Oncol (2011) 16(4):328–34. doi: 10.1007/s10147-011-0185-7
12. Pisanu A, Podda M, Cois A, Uccheddu A. Gastric cancer in the young: is it a different clinical entity? a retrospective cohort study. Gastroenterol Res practice (2014) 2014:125038. doi: 10.1155/2014/125038
13. Smyth EC, Nilsson M, Grabsch HI, van Grieken NC, Lordick F. Gastric cancer. Lancet (London England) (2020) 396(10251):635–48. doi: 10.1016/S0140-6736(20)31288-5
14. Bray F, Ferlay J, Soerjomataram I, Siegel RL, Torre LA, Jemal A. Global cancer statistics 2018: GLOBOCAN estimates of incidence and mortality worldwide for 36 cancers in 185 countries. CA: Cancer J Clin (2018) 68(6):394–424. doi: 10.3322/caac.21492
15. Plummer M, Franceschi S, Vignat J, Forman D, de Martel C. Global burden of gastric cancer attributable to helicobacter pylori. Int J cancer (2015) 136(2):487–90. doi: 10.1002/ijc.28999
16. Lin Z, Wang R, Zhou Y, Wang Q, Yang CY, Hao BC, et al. Prediction of distant metastasis and survival prediction of gastric cancer patients with metastasis to the liver, lung, bone, and brain: research based on the SEER database. Ann Trans Med (2022) 10(1):16. doi: 10.21037/atm-21-6295
17. Wei Q, Gao Y, Qi C, Yuan X, Li J, Xu Q, et al. Clinicopathological characteristics and prognosis of signet ring gastric cancer: A population-based study. Front Oncol (2021) 11:580545. doi: 10.3389/fonc.2021.580545
18. Chen J, Wu L, Zhang Z, Zheng S, Lin Y, Ding N, et al. A clinical model to predict distant metastasis in patients with superficial gastric cancer with negative lymph node metastasis and a survival analysis for patients with metastasis. Cancer Med (2021) 10(3):944–55. doi: 10.1002/cam4.3680
19. Huang Q, Zheng X, Jiao Y, Lei Y, Li X, Bi F, et al. A distinct clinicopathological feature and prognosis of young gastric cancer patients aged ≤ 45 years old. Front Oncol (2021) 11:674224. doi: 10.3389/fonc.2021.674224
20. Cunningham D, Allum WH, Stenning SP, Thompson JN, Van de Velde CJ, Nicolson M, et al. Perioperative chemotherapy versus surgery alone for resectable gastroesophageal cancer. New Engl J Med (2006) 355(1):11–20. doi: 10.1056/NEJMoa055531
21. Van Cutsem E, Moiseyenko VM, Tjulandin S, Majlis A, Constenla M, Boni C, et al. Phase III study of docetaxel and cisplatin plus fluorouracil compared with cisplatin and fluorouracil as first-line therapy for advanced gastric cancer: A report of the V325 study group. J Clin oncol: Off J Am Soc Clin Oncol (2006) 24(31):4991–7. doi: 10.1200/JCO.2006.06.8429
22. Shah MA, Bang YJ, Lordick F, Alsina M, Chen M, Hack SP, et al. Effect of fluorouracil, leucovorin, and oxaliplatin with or without onartuzumab in HER2-negative, MET-positive gastroesophageal adenocarcinoma: The METGastric randomized clinical trial. JAMA Oncol (2017) 3(5):620–7. doi: 10.1001/jamaoncol.2016.5580
23. Fuchs CS, Shitara K, Di Bartolomeo M, Lonardi S, Al-Batran SE, Van Cutsem E, et al. Ramucirumab with cisplatin and fluoropyrimidine as first-line therapy in patients with metastatic gastric or junctional adenocarcinoma (RAINFALL): a double-blind, randomised, placebo-controlled, phase 3 trial. Lancet Oncol (2019) 20(3):420–35. doi: 10.1016/S1470-2045(18)30791-5
24. Cats A, Jansen EPM, van Grieken NCT, Sikorska K, Lind P, Nordsmark M, et al. Chemotherapy versus chemoradiotherapy after surgery and preoperative chemotherapy for resectable gastric cancer (CRITICS): an international, open-label, randomised phase 3 trial. Lancet Oncol (2018) 19(5):616–28. doi: 10.1016/S1470-2045(18)30132-3
25. Lee J, Lim DH, Kim S, Park SH, Park JO, Park YS, et al. Phase III trial comparing capecitabine plus cisplatin versus capecitabine plus cisplatin with concurrent capecitabine radiotherapy in completely resected gastric cancer with D2 lymph node dissection: the ARTIST trial. J Clin oncol: Off J Am Soc Clin Oncol (2012) 30(3):268–73. doi: 10.1200/JCO.2011.39.1953
26. Macdonald JS, Smalley SR, Benedetti J, Hundahl SA, Estes NC, Stemmermann GN, et al. Chemoradiotherapy after surgery compared with surgery alone for adenocarcinoma of the stomach or gastroesophageal junction. New Engl J Med (2001) 345(10):725–30. doi: 10.1056/NEJMoa010187
27. Cheng J, Lao YJ, Wang Q, Huang K, Mou JL, Feng JH, et al. Predicting distant metastasis in young-onset colorectal cancer after surgery: A retrospective study. Front Oncol (2022) 12:804038. doi: 10.3389/fonc.2022.804038
28. Wang S, Yang L, Ci B, Maclean M, Gerber DE, Xiao G, et al. Development and validation of a nomogram prognostic model for SCLC patients. J Thorac oncol: Off Publ Int Assoc Study Lung Cancer (2018) 13(9):1338–48. doi: 10.1016/j.jtho.2018.05.037
Keywords: gastric cancer, metastasis, early-onset, prediction model, nomogram
Citation: Bi B, Deng G-f, Duan Y-m, Huang Z-j, Chen X-y, Zhang C-h and He Y-l (2023) Retrospective analysis of risk factors for distant metastasis of early-onset gastric cancer during the perioperative period. Front. Oncol. 13:1003977. doi: 10.3389/fonc.2023.1003977
Received: 26 July 2022; Accepted: 20 January 2023;
Published: 03 February 2023.
Edited by:
Chih-Yang Wang, Taipei Medical University, TaiwanReviewed by:
Shiva Basnet, Southern University of Science and Technology, ChinaYf Gu, Zhejiang University, China
Wei-Jan Wang, China Medical University (Taiwan), Taiwan
Copyright © 2023 Bi, Deng, Duan, Huang, Chen, Zhang and He. This is an open-access article distributed under the terms of the Creative Commons Attribution License (CC BY). The use, distribution or reproduction in other forums is permitted, provided the original author(s) and the copyright owner(s) are credited and that the original publication in this journal is cited, in accordance with accepted academic practice. No use, distribution or reproduction is permitted which does not comply with these terms.
*Correspondence: Yu-long He, aGV5dWxvbmdAbWFpbC5zeXN1LmVkdS5jbg==; Chang-hua Zhang, emhjaGFuZ2hAbWFpbC5zeXN1LmVkdS5jbg==
†These authors have contributed equally to this work