- 1PhD Program in Clinical Drug Development of Herbal Medicine, College of Pharmacy, Taipei Medical University, Taipei, Taiwan
- 2Department of Pharmacy, Faculty of Health Science, University of Muhammadiyah Pekajangan Pekalongan, Pekalongan, Indonesia
- 3Department of Clinical Pharmacy, School of Pharmacy, College of Pharmacy, Taipei Medical University, Taipei, Taiwan
- 4Department of Pharmacy, Faculty of Health Science, University of Muhammadiyah Mataram, Mataram, Indonesia
- 5Faculty of Pharmacy, University of Ahmad Dahlan, Yogyakarta, Indonesia
- 6Institute of Cellular and Organismic Biology, Academia Sinica, Taipei, Taiwan
- 7Department of Pharmacy, Wan Fang Hospital, Taipei Medical University, Taipei, Taiwan
- 8Integrative Research Center for Critical Care, Department of Pharmacy, Wan Fang Hospital, Taipei Medical University, Taipei, Taiwan
According to the National Comprehensive Cancer Network and the American Society of Clinical Oncology, the standard treatment for pancreatic cancer (PC) is gemcitabine and fluorouracil. Other chemotherapeutic agents have been widely combined. However, drug resistance remains a huge challenge, leading to the ineffectiveness of cancer therapy. Therefore, we are trying to discover new treatments for PC by utilizing genomic information to identify PC-associated genes as well as drug target genes for drug repurposing. Genomic information from a public database, the cBio Cancer Genomics Portal, was employed to retrieve the somatic mutation genes of PC. Five functional annotations were applied to prioritize the PC risk genes: Kyoto Encyclopedia of Genes and Genomes; biological process; knockout mouse; Gene List Automatically Derived For You; and Gene Expression Omnibus Dataset. DrugBank database was utilized to extract PC drug targets. To narrow down the most promising drugs for PC, CMap Touchstone analysis was applied. Finally, ClinicalTrials.gov and a literature review were used to screen the potential drugs under clinical and preclinical investigation. Here, we extracted 895 PC-associated genes according to the cBioPortal database and prioritized them by using five functional annotations; 318 genes were assigned as biological PC risk genes. Further, 216 genes were druggable according to the DrugBank database. CMap Touchstone analysis indicated 13 candidate drugs for PC. Among those 13 drugs, 8 drugs are in the clinical trials, 2 drugs were supported by the preclinical studies, and 3 drugs are with no evidence status for PC. Importantly, we found that midostaurin (targeted PRKA) and fulvestrant (targeted ESR1) are promising candidate drugs for PC treatment based on the genomic-driven drug repurposing pipelines. In short, integrated analysis using a genomic information database demonstrated the viability for drug repurposing. We proposed two drugs (midostaurin and fulvestrant) as promising drugs for PC.
Introduction
Pancreatic cancer (PC) is the 12th most prevalent cancer in men and the 11th most common cancer in women, with about 450,000 new cases diagnosed worldwide every year (1). The high mortality and poor prognosis are primarily due to lack of noticeable and distinctive clinical signs or biomarkers for early detection. Aggressive metastatic spread of PC contributes to the difficulty of treatment (2). Generally, PC is classified into two types: the most frequent pancreatic adenocarcinoma, which arises in the pancreas’ exocrine glands, and the less common pancreatic neuroendocrine tumor, which occurs in the pancreas’ endocrine tissue (1). Another forms of categorization is based on whether the tumors present in the entire (solid or cystic) or on the prevailing cell differentiation structure (ductal, acinar, or endocrine). Solid types include pancreatic ductal adenocarcinoma, neuroendocrine neoplasms, acinar cell carcinomas, and pancreatoblastomas. Mucinous cystic neoplasms, intraductal papillary mucinous neoplasms, and strong pseudopapillary neoplasms are some of the less harmful cystic forms (3).
The options of PC treatment are very restricted and highly dependent on the stage of the disease. Chemotherapy treatment remains the primary choice for patients with advanced and metastatic tumors. Radiation is another treatment for unresectable, metastatic cancer when combined with chemotherapy (4). The National Comprehensive Cancer Network (NCCN) and the American Society of Clinical Oncology (ASCO) recommend modified FOLFIRINOX, gemcitabine and capecitabine, and single-agent gemcitabine or fluorouracil. S-1, an oral 5-fluorouracil prodrug as a standard treatment for PC. However, those treatments only marginally prolong life expectancy by approximately 3% (5). Furthermore, patients with PC are typically diagnosed at advanced stage with remote metastases. Therapeutic resistance is a persistent challenge, dumping therapeutic efficacy and prognosis of PC (6). Therefore, it is urgent to develop more non-surgical therapeutic approaches to effectively treat PC. One of the strategies to tackle those issues is by utilizing the available drugs for new indications.
Drug repurposing is a method to identify new indications of a drug (7). This approach has several advantages in the drug discovery for specific indications. First, the risk of failure is lower because the repurposed drugs are relatively safe if early-stage studies have been completed in animal models and humans. Second, this approach is able to reduce the time for pharmacokinetic and toxicological studies. Third, less capital is required (8). Genomic information has been widely utilized for drug repurposing. One of the databases that provide genomic information is cBioPortal. cBioPortal is an open-access resource for the interactive exploration of multidimensional cancer genomics datasets (9). Herein, we employed cBioPortal as a main resource and integrated different annotations to prioritize the PC risk genes. The detailed flowchart of genomic-driven drug repurposing is illustrated in Figure 1.
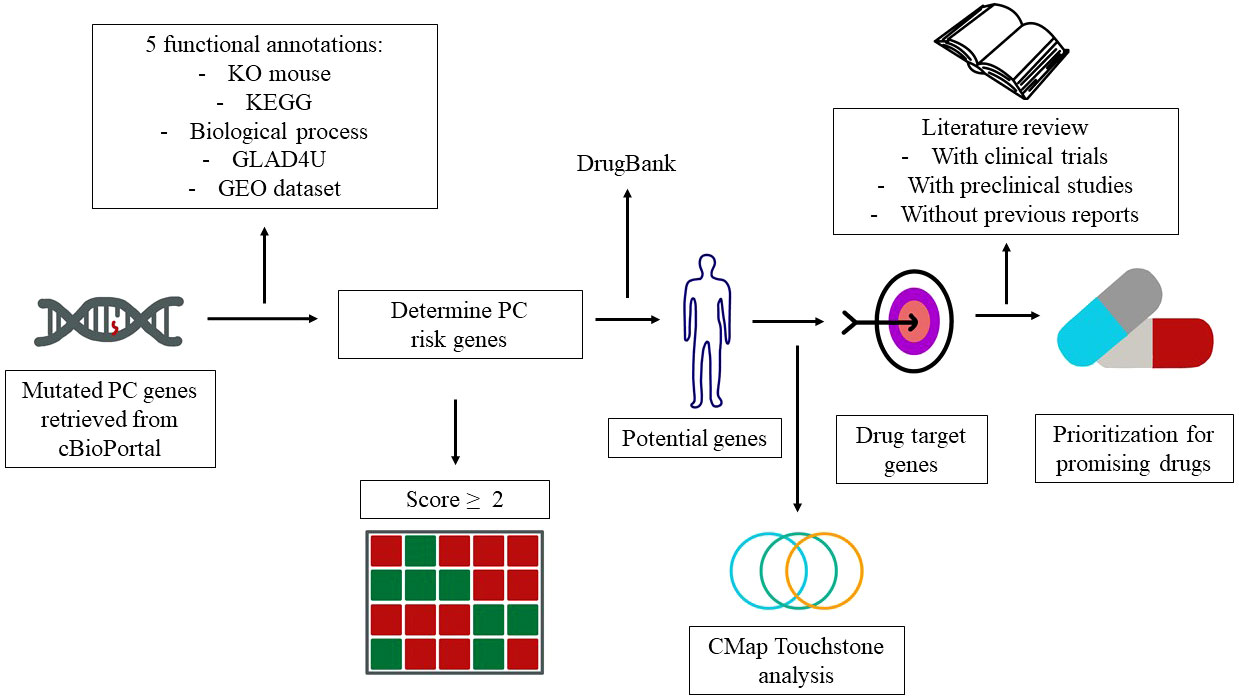
Figure 1 Study design and genes that contribute to pancreatic cancer (PC). The workflow elucidates the PC risk genes and candidate drugs derived from the public databases.
Methods
Retrieving pancreatic cancer associated genes
PC genes with somatic mutation (PC-associated gene) were retrieved from the cBioPortal (https://www.cbioportal.org/) database (9). PC-associated genes were provided by cBioPortal, which were extracted from nine studies with 1,023 samples (Supplementary Table 1).
Identifying biological pancreatic cancer risk genes
Subsequently, five functional annotations: (1) Kyoto encyclopedia of genes and genomes (KEGG); (2) Biological process (BP); (3) Knock out mouse (KO); (4) Gene list automatically derived for you (GLAD4U); and (5) Gene Expression Omnibus Dataset (GEO) were utilized to build a scoring system to prioritize PC-associated genes. The first functional annotation was KEGG to determine the molecular pathway. The second was gene ontology BP to identify genes involved in the biological protein network. The third was KO mouse to investigate whether the gene contributes to specific phenotype disease in the mouse. The fourth was GLAD4U to analyze which gene is related to a particular disease (10) , and the fifth applied four datasets from the GEO database to highlight up-regulated genes in PC samples. Only data from human PC patients was collected from each dataset for investigation. The dataset we retrieved from the GEO database were GSE28735, GSE15471, GSE16515, and GSE19650 and were depicted in Table 1. We used Within-Array Normalization to normalized each dataset before extracting the differential expression genes (DEGs). The DEGs were found by setting an adjusted p-value cut-off of 0.05 and log fold cut>2 (Supplementary Figure 1). The annotation applied for this study was from the WebGestalt 2019 database (http://www.webgestalt.org/), a popular tool for the interpretation of gene lists derived from large-scale-omics studies (11) and GEO database (12). The significant result for annotation utilizing WebGestalt was set at a false discovery rate (FDR) < 0.05. The scoring system was utilized in a previous study by Okada et al., which identified potential treatments for rheumatoid arthritis (13). Genes that fulfilled two or more of the criteria were defined as biologicalPC risk genes.
Overlapping drug targets for pancreatic cancer with DrugBank
To uncover therapeutic candidates for repurposing in PC, we mapped biological PC risk genes to DrugBank databases (https://go.drugbank.com). The DrugBank database is a bioinformatics and cheminformatics database that provides detailed information on drug compounds and gene targets to the drug discovery and clinical medicine communities (14). Drugs having pharmacological activity, human effectiveness, approved annotations, clinical trials (http://www.ClinicalTrials.gov), and experimental drugs were among the factors utilized to query the databases.
Prioritizing candidate drugs
The core principle behind Connectivity Map (CMap) Touchstone analysis is to compare a drug-specific gene expression profile with a disease-specific gene signature using a reference database (15). We used the CMap Touchstone database tool (https://clue.io) to grade drugs as shown in a connectivity score to prioritize the drugs for PC repurposing (-100 to 100) (16). Here, gemcitabine was used as a standard treatment for PC according to the NCCN guideline (5). The CMap database provides nine cell lines, namely A375, A549, HA1E, HCC515, HEPG2, HT29, MCF7, PC3, and VCAP. However, by using gemcitabine as the gold-standard, only three cell lines (A549, MCF7, and PC3) are available to match with gemcitabine in the CMap database.
According to the DrugBank database, the target protein of gemcitabine is the ribonucleoside-diphosphate reductase large subunit (RRM1). Thus, CMap analysis generated a score that indicates the interaction strength between candidate drugs and the protein. The ranked candidate drug was prioritized based on the score (≥ 80). Finally, a PubMed literature review and ClinicalTrials.gov were used to evaluate the reliability of candidate drugs based on the evidence from preclinical studies and clinical trials.
Statistical analysis
All analytic workflows were performed using R Studio version 1.3.1073 (https://www.r-project.org). DEGs were obtained from the GEO dataset (https://www.ncbi.nlm.nih.gov/gds) using the R limma package. Overrepresentation analysis (ORA) was utilized to prioritize the gene in KEGG, BP, KO mouse, and GLAD4U provided by Webgestalt 2019 (11, 17). The statistical significance was established using an FDR of 0.05. R studio were used for graphic visualization.
Results
Prioritizing genes associated with pancreatic cancer
We retrieved sample data from the cBioPortal database. Nine studies related to PC were obtained with 1,032 participating patients (Supplementary Table 1). From nine studies, 895 genes were identified as PC-associated genes (Supplementary Table 2). Applications of previous approaches, five functional annotations were used to generate a risk score representing the most probable candidate genes as biological PC risk genes (18–20). The result was shown in Figure 2A and the detailed information was in Supplementary Table 3. 19.32%, 34.41%, 30.17%, 27.93%, and 15.53% of PC-associated genes were found from KEGG, BP, KO mouse, GLAD4U, and GEO, respectively. Among 895 genes, 318 genes met the criteria of score ≥2 and were further defined as biological PC risk genes. The highest score (score = 5) from the five criteria was achieved by 12 genes, including CDKN2A, CTNNB1, BRCA2, AR, CCND2, PML, MYC, CCND3, STAT3, ZBTB16, EGFR, ESR1. Among the highest score genes, CDKN2A and BRCA2 have been previously reported as dominated mutation genes in PC (21, 22).
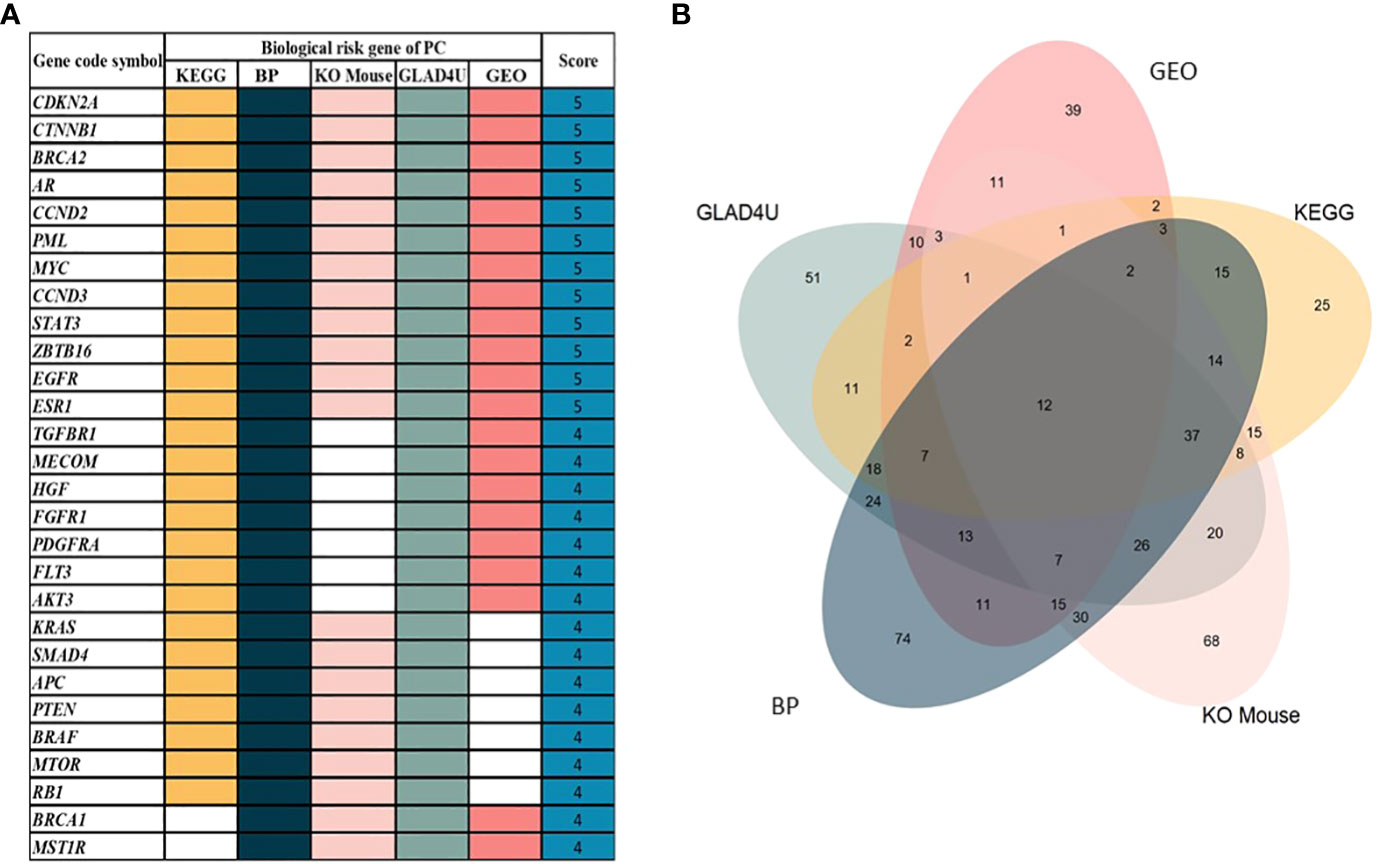
Figure 2 (A) 30 out of 318 genes after prioritization based on five functional annotations. White box indicated that no significant annotation was found within the database. (B) The Venn diagram shows the distribution of genes in each annotation. From 895 genes, 318 genes were identified as biological PC risk genes with accumulated scores ≥2.
Additionally, we analyzed the correlation among five functional annotations. Correlation coefficient analysis was conducted to assess whether the five functional annotations have possible linear relationships with each other (to avoid overlap between these functional annotations). Results showed that those five functional annotations had values of 0.30–0.50, which indicated low (weak) correlations between each other, and the results are depicted in Supplementary Figure 2. Additionally, the distribution score of each criterion is shown in Figure 2B. The number of intersection among five annotations was 12 genes. In addition, the gene number without overlapping among annotations were 25, 74, 68, 51, and 39 for KEGG, BP, KO mouse, GLAD4U and GEO, respectively.
Furthermore, the results of the four functional annotations, KEGG, BP, KO mouse, and GLAD4U, showed that these biological-PC risk genes are highly correspond with cancer development, especially in cell proliferation. In KEGG, the major pathway was indicated as EGFR tyrosine inhibitor resistance, prolactin signaling pathway, and pathway in cancer. In BP, the dominant biological process is in cell proliferation process. Regarding to KO mouse, results pointed out the importance of bone marrow cell proliferation. Finally, GLAD4U results suggested a strong correlation between candidate genes and neoplasms (Figures 3A–D). Taken together, these results indicated that 318 biological PC risk genes highly correspond to cancer growth-related signals.
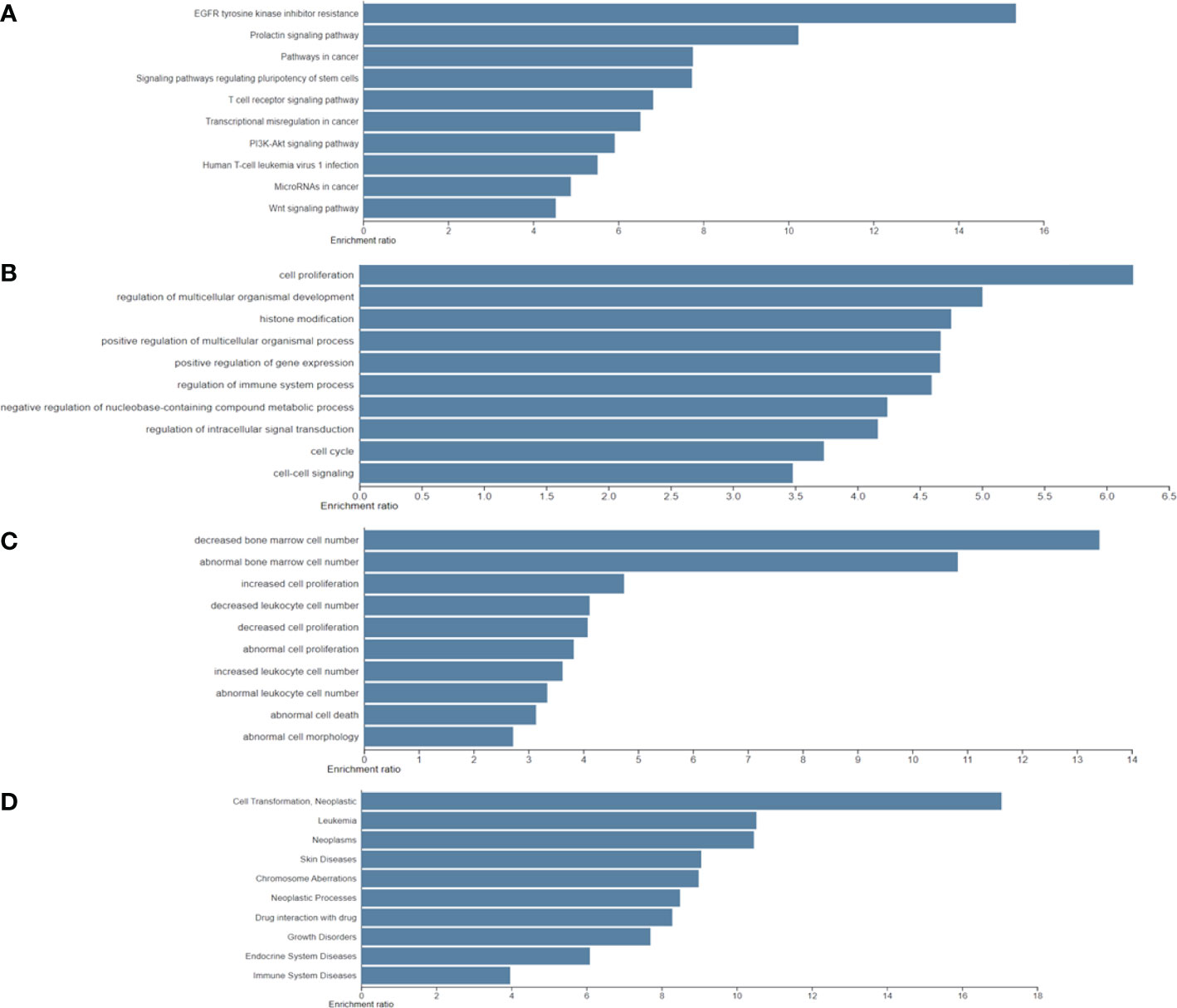
Figure 3 Significantly identified top 10 enriched analysis for four functional annotations: (A) Kyoto Encyclopedia of Genes and Genomes; (B) biological process; (C); knockout mouse; and (D) Gene List Automatically Derived For You by differentially expressed genes.
Repurposing drugs for pancreatic cancer
The next step is to map 318 biological PC risk genes into the DrugBank database. Among those genes, only 77 genes overlapped with DrugBank, indicating that not all biological PC risk genes are druggable. 77 drug target genes correspond with 216 drugs. Among the 77 target genes, over expression of EGFR has been previously identified in pancreatic tumors (23, 24) and it is associated with poor prognosis and disease progression (25, 26). Indeed, erlotinib is an EGFR tyrosine kinase inhibitor and the combination with gemcitabine demonstrated a moderate advantage (23). The results demonstrated that this process of repurposing drugs is a reasonable approach.
Prioritization of candidate drug for pancreatic cancer
To prioritize the most potential candidate drugs for PC, 216 drugs were tested by using the CMap Touchstone database. We used the profiles of gemcitabine in the MCF7 cell line as the standard for PC treatment. Importantly, 77 drugs exhibited positive correlations. We ranked the 77 drugs according to the CMap Touchstone database scores. 13 drugs with a score>80 were defined as PC candidate drugs (Table 2).
Among the 13 drugs extracted from the CMap Touchstone database, 8 drugs are under clinical trials for PC including tamoxifen, sunitinib, bosutinib, everolimus, afatinib, palbociclib, vorinostat, and axitinib. Two drugs are with preclinical studies clomifene and raloxifene; and three drugs are without evidence from clinical trials: fulvestrant, midostaurin, and toremifene. The connection among target proteins, PC candidate drugs, and the evidence level status is demonstrated in Figure 4. Among the 13 drugs, fulvestrant is a promising drug repurposing for PC because the target gene, ESR1, is one of the most dominated biological-PC risk genes in this analysis.
Discussion
This study utilized a genomic database to retrieve somatic mutation genes of PC. Five functional annotations were applied to build a scoring system and prioritized PC risk drug target identifications. We found 318 drug target genes.12 genes of them had the highest score (score = 5) after prioritization. Molecular mechanism of the 12 genes were listed in Table 3. After prioritization of candidate drugs, we found 13 potential drugs (tamoxifen, sunitinib, bosutinib, everolimus, afatinib, palbociclib, vorinostat, axitinib, clomifene, raloxifene fulvestrant, midostaurin and toremifene) for PC.
Out of 12 genes, some potential gene targets were highlighted by our pipelines, including BCR and MTOR (55, 56), which were targeted by bosutinib and everolimus, respectively. Genes including KRAS, TP53, and SMAD4 had also been reported from previous studies (57, 58). These results indicated that five functional annotations are useful tools to priortize the important genes.
CMap Touchstone analysis showed that ESR1 as a dominated target protein for PC. ESR1 is a ligand-activated transcription factor that belongs to the steroid/nuclear receptor superfamily (59). The estrogen-responsive element (ERE) on the promoters of ESR1 target genes dimerizes and binds to coactivators in response to estrogen binding (60). ESR1 is known to involve in various cancers, such as endometrial and ovarian cancers (61). A previous study mentioned that ESR1 is also expressed in a subset of pancreatic adenocarcinoma, most notably in mucinous tumors (55, 56). Tamoxifen is the ESR1-targeted drug with both preclinical trial and clinical results for PC (62, 63). Furthermore, several clinical trials have reported using estrogen therapy on PC treatment (53, 63–65). However, the results are still controversial. Another drug that targeted ESR1 is fulvestrant, originally used for metastatic breast cancer. Fulvestrant works by inhibiting the dimerization of the ESR1 receptor and exerts no estrogen agonist effect (66). Therefore, if compared with tamoxifen, side effects of fulvestrant seem to be more favorable.
Midostaurin is a candidate drug from our prioritization process. The target for midostaurin is PRKCA that is a phospholipid-dependent, cytoplasmic serine/threonine kinase acting as an intracellular signal transducer (67). PRKCA activates different proteins such as cellular proliferation, differentiation, and gene expression (68). In addition, the expression of PRKCA has been associated with an elevated expression of the multidrug resistance phenotype (MDR) (69). Clinically, PRKCA is a target for types of cancer including acute myeloid leukemia (AML), breast cancer, and ovarian cancer (70). However, the reference of PRKCA as a treatment target for PC is still limited. Therefore, we propose PRKCA as a potential novel target. In this study, midostaurin targeted PRKCA with a score of 89, indicating the similar profiles between midostaurin andgemcitabine.
This study focused on bioinformatic approaches to prioritize drug candidates for PC. However, we should emphasize that further experimental and clinical studies are necessary to confirm the findings. The advantage of these approaches is narrowing down the drug targets and improving the success rate of drug development by prioritizing the best candidates. It is worth noting that this study has some limitations. First, not all genes retrieved from our analysis were druggable. Second, we used only one drug, gemcitabine, as the standard treatment for PC; this approach omits other potential candidate drugs to be found. Finally, CMap did not provide specific cell lines for PC.
Conclusions
Using our pipeline, we reported that there are 13 compounds that are the most potential PC candidate drugs. Two drugs are with preclinical results, eight are under clinical trials, and three have no previous reports yet. Two drugs (midostaurin and fulvestrant) were proposed as novel drugs repurposing for PC treatment. Here, we highlighted that our research demonstrated the viability of using public genomic information as a potential drug discovery method.
Data availability statement
The original contributions presented in the study are included in the article/Supplementary Material. Further inquiries can be directed to the corresponding authors.
Author contributions
Conceptualization: EM and W-CC. Data curation: EM, WA, LMI, C-NK and W-CC. Formal analyses: EM, WA, LMI and W-CC. Data interpretation and discussion: EM, WA, LMI, W-CH, C-NK and W-CC. Writing—review and editing: EM, WA, LMI, W-CH, C-NK and W-CC. Manuscript revision: EM, C-NK and W-CC. Supervision: W-CH, C-NK and W-CC. All authors contributed to the article and approved the submitted version.
Funding
This work was supported by grants from the Ministry of Science and Technology, Taiwan (no. MOST109-2320-B-038-013) and Taipei Medical University, Taiwan (12310-106079;12310-10739) for Yusuke Nakamura Chair Professorship.
Acknowledgments
We thank Min-Rou Lin, Wan-Hsuan Chou, Dr. Che-Mai Chang (Taipei Medical University, Taiwan) for helpful comments during the revision and Nien-Yu Yang (Taipei Medical University, Taiwan; The University of Manchester, UK) for the assistance with Figure 1 draft.
Conflict of interest
The authors declare that the research was conducted in the absence of any commercial or financial relationships that could be construed as a potential conflict of interest.
Publisher’s note
All claims expressed in this article are solely those of the authors and do not necessarily represent those of their affiliated organizations, or those of the publisher, the editors and the reviewers. Any product that may be evaluated in this article, or claim that may be made by its manufacturer, is not guaranteed or endorsed by the publisher.
Supplementary material
The Supplementary Material for this article can be found online at: https://www.frontiersin.org/articles/10.3389/fonc.2022.989077/full#supplementary-material
Abbreviations
PC, pancreatic cancer; cBioPortal, Cancer genomic bio portal; MCF7, Michigan Cancer Foundation-7; RRM1, ribonucleoside-diphosphate reductase large subunit 1; PRKA, protein kinase A; ESR1, estrogen receptor 1; NCCN, National Comprehensive Cancer Network; ASCO, American Society of Clinical Oncology; KEGG, Kyoto Encyclopedia of Genes and Genomes; BP, biological process; KO, knockout mouse; GLAD4U, Gene List Automatically Derived For You; GSE, Gene Omnibus Dataset Series; ORA, Overrepresentation analysis; FDR, false discovery rate; CDKN2A, cyclin-dependent kinase inhibitor 2A; BRCA2, BReast CAncer gene 2; EGFR, epidermal growth factor receptor; BCR, breakpoint cluster region; MTOR, mammalian target of rapamycin; KRAS, K-Ras; TP53, tumor protein 53; SMAD4, suppressor of mothers against decapentaplegic 4; ERE, estrogen-responsive element; PRKCA, protein kinase C alpha; MDR, multidrug resistance phenotype; CML, chronic myelogenous leukemia; NSCLC, non-small cell lung cancer; PDGFRB, platelet-derived growth factor receptor beta; CDK4, cyclin-dependent kinase 4; FLT, fms-related receptor tyrosine kinase 1; HDAC1, histone deacetylase 1; CTNNB1, catenin beta 1; AR, androgen receptor; CCND2, cyclin D2; MYC, C-Myc; and CCND3, cyclin D3.
Glossary
References
1. Bray F, Ferlay J, Soerjomataram I, Siegel RL, Torre LA, Jemal A. Global cancer statistics 2018: Globocan estimates of incidence and mortality worldwide for 36 cancers in 185 countries. CA Cancer J Clin (2018) 68(6):394–424. doi: 10.3322/caac.21492
2. Maitra A, Hruban RH. Pancreatic cancer. Annu Rev Pathol (2008) 3:157–88. doi: 10.1146/annurev.pathmechdis.3.121806.154305
3. Grützmann R, Niedergethmann M, Pilarsky C, Klöppel G, Saeger HD. Intraductal papillary mucinous tumors of the pancreas: Biology, diagnosis, and treatment. Oncologist (2010) 15(12):1294–309. doi: 10.1634/theoncologist.2010-0151
4. Hammel P, Huguet F, van Laethem JL, Goldstein D, Glimelius B, Artru P, et al. Effect of chemoradiotherapy vs chemotherapy on survival in patients with locally advanced pancreatic cancer controlled after 4 months of gemcitabine with or without erlotinib: The Lap07 randomized clinical trial. JAMA (2016) 315(17):1844–1853. doi: 10.1001/jama.2016.4324
5. Adamska A, Domenichini A, Falasca M. Pancreatic ductal adenocarcinoma: Current and evolving therapies. Int J Mol Sci (2017) 18(7):1338. doi: 10.3390/ijms18071338
6. Hidalgo M, Cascinu S, Kleeff J, Labianca R, Löhr JM, Neoptolemos J, et al. Addressing the challenges of pancreatic cancer: Future directions for improving outcomes. Pancreatology (2015) 15(1):8–18. doi: 10.1016/j.pan.2014.10.001
7. Ashburn TT, Thor KB. Drug repositioning: Identifying and developing new uses for existing drugs. Nat Rev Drug Discov (2004) 3(8):673–83. doi: 10.1038/nrd1468
8. Breckenridge A, Jacob R. Overcoming the legal and regulatory barriers to drug repurposing. Nat Rev Drug Discov (2019) 18(1):1–2. doi: 10.1038/nrd.2018.92
9. Cerami E, Gao J, Dogrusoz U, Gross BE, Sumer SO, Aksoy BA, et al. The cbio cancer genomics portal: An open platform for exploring multidimensional cancer genomics data. Cancer Discov (2012) 2(5):401–4. doi: 10.1158/2159-8290.Cd-12-0095
10. Jourquin J, Duncan D, Shi Z, Zhang B. Glad4u: Deriving and prioritizing gene lists from pubmed literature. BMC Genomics (2012) 13(8):S20. doi: 10.1186/1471-2164-13-S8-S20
11. Wang J, Vasaikar S, Shi Z, Greer M, Zhang B. Webgestalt 2017: A more comprehensive, powerful, flexible and interactive gene set enrichment analysis toolkit. Nucleic Acids Res (2017) 45(W1):W130–7. doi: 10.1093/nar/gkx356
12. Barrett T, Wilhite SE, Ledoux P, Evangelista C, Kim IF, Tomashevsky M, et al. Ncbi geo: Archive for functional genomics data sets–update. Nucleic Acids Res (2013) 41(D1):D991–5. doi: 10.1093/nar/gks1193
13. Okada Y, Wu D, Trynka G, Raj T, Terao C, Ikari K, et al. Genetics of rheumatoid arthritis contributes to biology and drug discovery. Nature (2014) 506(7488):376–81. doi: 10.1038/nature12873
14. Wishart DS, Feunang YD, Guo AC, Lo EJ, Marcu A, Grant JR, et al. Drugbank 5.0: A major update to the drugbank database for 2018. Nucleic Acids Res (2018) 46(D1):D1074–82. doi: 10.1093/nar/gkx1037
15. Musa A, Ghoraie LS, Zhang S-D, Glazko G, Yli-Harja O, Dehmer M, et al. A review of connectivity map and computational approaches in pharmacogenomics. Brief Bioinform (2018) 19(3):506–23. doi: 10.1093/bib/bbw112
16. Subramanian A, Narayan R, Corsello SM, Peck DD, Natoli TE, Lu X, et al. A next generation connectivity map: L1000 platform and the first 1,000,000 profiles. Cell (2017) 171(6):1437–52.e17. doi: 10.1016/j.cell.2017.10.049
17. Liao Y, Wang J, Jaehnig EJ, Shi Z, Zhang B. Webgestalt 2019: Gene set analysis toolkit with revamped uis and apis. Nucleic Acids Res (2019) 47(W1):W199–w205. doi: 10.1093/nar/gkz401
18. Irham LM, Wong HS-C, Chou W-H, Adikusuma W, Mugiyanto E, Huang W-C, et al. Integration of genetic variants and gene network for drug repurposing in colorectal cancer. Pharmacol Res (2020) 161:105203. doi: 10.1016/j.phrs.2020.105203
19. Adikusuma W, Irham LM, Chou WH, Wong HS, Mugiyanto E, Ting J, et al. Drug repurposing for atopic dermatitis by integration of gene networking and genomic information. Front Immunol (2021) 12:724277. doi: 10.3389/fimmu.2021.724277
20. Adikusuma W, Chou W-H, Lin M-R, Ting J, Irham LM, Perwitasari DA, et al. Identification of druggable genes for asthma by integrated genomic network analysis. Biomedicines (2022) 10(1):113. doi: 10.3390/biomedicines10010113
21. Hu Y, Guo M. Synthetic lethality strategies: Beyond Brca1/2 mutations in pancreatic cancer. Cancer Sci (2020) 111(9):3111–21. doi: 10.1111/cas.14565
22. Kamisawa T, Wood LD, Itoi T, Takaori K. Pancreatic cancer. Lancet (Lond Engl) (2016) 388(10039):73–85. doi: 10.1016/s0140-6736(16)00141-0
23. Moore MJ, Goldstein D, Hamm J, Figer A, Hecht JR, Gallinger S, et al. Erlotinib plus gemcitabine compared with gemcitabine alone in patients with advanced pancreatic cancer: A phase iii trial of the national cancer institute of Canada clinical trials group. J Clin Oncol (2007) 25(15):1960–6. doi: 10.1200/JCO.2006.07.9525
24. Tzeng C-WD, Frolov A, Frolova N, Jhala NC, Howard JH, Buchsbaum DJ, et al. Epidermal growth factor receptor (Egfr) is highly conserved in pancreatic cancer. Surgery (2007) 141(4):464–9. doi: 10.1016/j.surg.2006.09.009
25. Xiong HQ. Molecular targeting therapy for pancreatic cancer. Cancer Chemother Pharmacol (2004) 54 Suppl 1:S69–77. doi: 10.1007/s00280-004-0890-2
26. Ueda S, Ogata S, Tsuda H, Kawarabayashi N, Kimura M, Sugiura Y, et al. The correlation between cytoplasmic overexpression of epidermal growth factor receptor and tumor aggressiveness: Poor prognosis in patients with pancreatic ductal adenocarcinoma. Pancreas (2004) 29(1):e1–8. doi: 10.1097/00006676-200407000-00061
27. McWilliams RR, Wieben ED, Rabe KG, Pedersen KS, Wu Y, Sicotte H, et al. Prevalence of Cdkn2a mutations in pancreatic cancer patients: Implications for genetic counseling. Eur J Hum Genet (2011) 19(4):472–8. doi: 10.1038/ejhg.2010.198
28. Bartsch DK, Sina-Frey M, Lang S, Wild A, Gerdes B, Barth P, et al. Cdkn2a germline mutations in familial pancreatic cancer. Ann Surg (2002) 236(6):730–7. doi: 10.1097/00000658-200212000-00005
29. Overbeek KA, Rodríguez-Girondo MDM, Wagner A, van der Stoep N, van den Akker PC, Oosterwijk JC, et al. Genotype-phenotype correlations for pancreatic cancer risk in Dutch melanoma families with pathogenic Cdkn2avariants. J Med Genet (2021) 58(4):264. doi: 10.1136/jmedgenet-2019-106562
30. Rodriguez-Matta E, Hemmerich A, Starr J, Mody K, Severson EA, Colon-Otero G. Molecular genetic changes in solid pseudopapillary neoplasms (Spn) of the pancreas. Acta Oncol (2020) 59(9):1024–7. doi: 10.1080/0284186X.2020.1792549
31. Jiang X, Cao Y, Li F, Su Y, Li Y, Peng Y, et al. Targeting B-catenin signaling for therapeutic intervention in Men1-deficient pancreatic neuroendocrine tumours. Nat Commun (2014) 5(1):5809. doi: 10.1038/ncomms6809
32. Zeng G, Germinaro M, Micsenyi A, Monga NK, Bell A, Sood A, et al. Aberrant Wnt/Beta-catenin signaling in pancreatic adenocarcinoma. Neoplasia (2006) 8(4):279–89. doi: 10.1593/neo.05607
33. Saukkonen K, Hagström J, Mustonen H, Juuti A, Nordling S, Kallio P, et al. Prox1 and B-catenin are prognostic markers in pancreatic ductal adenocarcinoma. BMC Cancer (2016) 16(1):472. doi: 10.1186/s12885-016-2497-5
34. Holter S, Borgida A, Dodd A, Grant R, Semotiuk K, Hedley D, et al. Germline brca mutations in a Large clinic-based cohort of patients with pancreatic adenocarcinoma. J Clin Oncol (2015) 33(28):3124–9. doi: 10.1200/jco.2014.59.7401
35. Brand R, Borazanci E, Speare V, Dudley B, Karloski E, Peters MLB, et al. Prospective study of germline genetic testing in incident cases of pancreatic adenocarcinoma. Cancer (2018) 124(17):3520–7. doi: 10.1002/cncr.31628
36. Mizukami K, Iwasaki Y, Kawakami E, Hirata M, Kamatani Y, Matsuda K, et al. Genetic characterization of pancreatic cancer patients and prediction of carrier status of germline pathogenic variants in cancer-predisposing genes. EBioMedicine (2020) 60:103033. doi: 10.1016/j.ebiom.2020.103033
37. Lowery MA, Wong W, Jordan EJ, Lee JW, Kemel Y, Vijai J, et al. Prospective evaluation of germline alterations in patients with exocrine pancreatic neoplasms. J Natl Cancer Inst (2018) 110(10):1067–74. doi: 10.1093/jnci/djy024
38. Kanda T, Jiang X, Yokosuka O. Androgen receptor signaling in hepatocellular carcinoma and pancreatic cancers. World J Gastroenterol (2014) 20(28):9229–36. doi: 10.3748/wjg.v20.i28.9229
39. Okitsu K, Kanda T, Imazeki F, Yonemitsu Y, Ray R, Chang C, et al. Involvement of interleukin-6 and androgen receptor signaling in pancreatic cancer. Genes Cancer (2010) 1:859–67. doi: 10.1177/1947601910383417
40. Matsubayashi H, Sato N, Fukushima N, Yeo C, Walter K, Brune K, et al. Methylation of cyclin D2 is observed frequently in pancreatic cancer but is also an age-related phenomenon in gastrointestinal tissues. Clin Cancer Res an Off J Am Assoc Cancer Res (2003) 9:1446–52.
41. Schneeweis C, Hassan Z, Schick M, Keller U, Schneider G. The sumo pathway in pancreatic cancer: Insights and inhibition. Br J Cancer (2021) 124(3):531–8. doi: 10.1038/s41416-020-01119-6
42. Swayden M, Alzeeb G, Masoud R, Berthois Y, Audebert S, Camoin L, et al. Pml hyposumoylation is responsible for the resistance of pancreatic cancer. FASEB J (2019) 33(11):12447–63. doi: 10.1096/fj.201901091R
43. Ala M. Target c-myc to treat pancreatic cancer. Cancer Biol Ther (2022) 23(1):34–50. doi: 10.1080/15384047.2021.2017223
44. Buchholz M, Schatz A, Wagner M, Michl P, Linhart T, Adler G, et al. Overexpression of c-myc in pancreatic cancer caused by ectopic activation of Nfatc1 and the Ca2+/Calcineurin signaling pathway. EMBO J (2006) 25(15):3714–24. doi: 10.1038/sj.emboj.7601246
45. Radulovich N, Pham N-A, Strumpf D, Leung L, Xie W, Jurisica I, et al. Differential roles of cyclin D1 and D3 in pancreatic ductal adenocarcinoma. Mol Cancer (2010) 9(1):24. doi: 10.1186/1476-4598-9-24
46. Ding Z-Y, Li R, Zhang Q-J, Wang Y, Jiang Y, Meng Q-Y, et al. Prognostic role of cyclin D2/D3 in multiple human malignant neoplasms: A systematic review and meta-analysis. Cancer Med (2019) 8(6):2717–29. doi: 10.1002/cam4.2152
47. Chen H, Bian A, Yang L-f, Yin X, Wang J, Ti C, et al. Targeting Stat3 by a small molecule suppresses pancreatic cancer progression. Oncogene (2021) 40(8):1440–57. doi: 10.1038/s41388-020-01626-z
48. Corcoran RB, Contino G, Deshpande V, Tzatsos A, Conrad C, Benes CH, et al. Stat3 plays a critical role in kras-induced pancreatic tumorigenesis. Cancer Res (2011) 71(14):5020–9. doi: 10.1158/0008-5472.CAN-11-0908
49. Scholz A, Heinze S, Detjen KM, Peters M, Welzel M, Hauff P, et al. Activated signal transducer and activator of transcription 3 (Stat3) supports the malignant phenotype of human pancreatic cancer. Gastroenterology (2003) 125(3):891–905. doi: 10.1016/S0016-5085(03)01064-3
50. Zhang Q, Li X, Li Y, Chen S, Shen X, Dong X, et al. Expression of the PTEN/FOXO3a/PLZF signalling pathway in pancreatic cancer and its significance in tumourigenesis and progression. Invest New Drugs. (2020) 38(2):321–8. doi: 10.1007/s10637-019-00791-7
51. Troiani T, Martinelli E, Capasso A, Morgillo F, Orditura M, De Vita F, et al. Targeting egfr in pancreatic cancer treatment. Curr Drug Targets (2012) 13(6):802–10. doi: 10.2174/138945012800564158
52. Fagman JB, Ljungman D, Falk P, Iresjö BM, Engström C, Naredi P, et al. Egfr, but not Cox−2, protein in resected pancreatic ductal adenocarcinoma is associated with poor survival. Oncol Lett (2019) 17(6):5361–8. doi: 10.3892/ol.2019.10224
53. Boldes T, Merenbakh-Lamin K, Journo S, Shachar E, Lipson D, Yeheskel A, et al. R269c variant of Esr1: High prevalence and differential function in a subset of pancreatic cancers. BMC Cancer (2020) 20(1):531. doi: 10.1186/s12885-020-07005-x
54. Konduri S, Schwarz RE. Estrogen receptor B/A ratio predicts response of pancreatic cancer cells to estrogens and phytoestrogens. J Surg Res (2007) 140(1):55–66. doi: 10.1016/j.jss.2006.10.015
55. Ishida K, Sasano H, Moriya T, Takahashi Y, Sugimoto R, Mue Y, et al. Immunohistochemical analysis of steroidogenic enzymes in ovarian-type stroma of pancreatic mucinous cystic neoplasms: Comparative study of subepithelial stromal cells in intraductal papillary mucinous neoplasms of the pancreas. Pathol Int (2016) 66(5):281–7. doi: 10.1111/pin.12406
56. Iwao K, Miyoshi Y, Ooka M, Ishikawa O, Ohigashi H, Kasugai T, et al. Quantitative analysis of estrogen receptor-alpha and -beta messenger rna expression in human pancreatic cancers by real-time polymerase chain reaction. Cancer Lett (2001) 170(1):91–7. doi: 10.1016/s0304-3835(01)00563-8
57. Qian ZR, Rubinson DA, Nowak JA, Morales-Oyarvide V, Dunne RF, Kozak MM, et al. Association of Alterations in Main Driver Genes with Outcomes of Patients with Resected Pancreatic Ductal Adenocarcinoma. JAMA Oncol (2018) 4(3):e173420. doi: 10.1001/jamaoncol.2017.3420
58. Eser S, Schnieke A, Schneider G, Saur D. Oncogenic Kras Signalling in Pancreatic Cancer. (2014) 111(5):817–22. doi: 10.1038/bjc.2014.215
59. Ascenzi P, Bocedi A, Marino M. Structure-function relationship of estrogen receptor alpha and beta: Impact on human health. Mol Aspects Med (2006) 27(4):299–402. doi: 10.1016/j.mam.2006.07.001
60. Klinge CM. Estrogen receptor interaction with Co-activators and Co-repressors. Steroids (2000) 65(5):227–51. doi: 10.1016/s0039-128x(99)00107-5
61. Jemal A, Bray F, Center MM, Ferlay J, Ward E, Forman D. Global cancer statistics. CA Cancer J Clin (2011) 61(2):69–90. doi: 10.3322/caac.20107
62. Greenway B, Duke D, Pym B, Iqbal MJ, Johnson PJ, Williams R. The control of human pancreatic adenocarcinoma xenografts in nude mice by hormone therapy. Br J Surg (1982) 69(10):595–7. doi: 10.1002/bjs.1800691013
63. Tomao S, Romiti A, Massidda B, Ionta MT, Farris A, Zullo A, et al. A phase ii study of gemcitabine and tamoxifen in advanced pancreatic cancer. Anticancer Res (2002) 22(4):2361–4.
64. Bakkevold KE, Pettersen A, Arnesjø B, Espehaug B. Tamoxifen therapy in unresectable adenocarcinoma of the pancreas and the papilla of vater. Br J Surg (1990) 77(7):725–30. doi: 10.1002/bjs.1800770704
65. Wong A, Chan A. Survival benefit of tamoxifen therapy in adenocarcinoma of pancreas. a case-control study. Cancer (1993) 71(7):2200–3. doi: 10.1002/1097-0142(19930401)71:7<2200::aid-cncr2820710706>3.0.co;2-2
66. Wakeling AE, Dukes M, Bowler J. A potent specific pure antiestrogen with clinical potential. Cancer Res (1991) 51(15):3867–73.
67. Newton AC. Regulation of protein kinase c. Curr Opin Cell Biol (1997) 9(2):161–7. doi: 10.1016/s0955-0674(97)80058-0
68. O'Brian C, Vogel VG, Singletary SE, Ward NE. Elevated protein kinase c expression in human breast tumor biopsies relative to normal breast tissue. Cancer Res (1989) 49(12):3215–7.
69. Gupta KP, Ward NE, Gravitt KR, Bergman PJ. O'Brian CA. partial reversal of multidrug resistance in human breast cancer cells by an n-myristoylated protein kinase c-alpha pseudosubstrate peptide. J Biol Chem (1996) 271(4):2102–11. doi: 10.1074/jbc.271.4.2102
Keywords: genomic, pancreatic cancer, drug repurposing, fulvestrant, midostaurin
Citation: Mugiyanto E, Adikusuma W, Irham LM, Huang W-C, Chang W-C and Kuo C-N (2022) Integrated genomic analysis to identify druggable targets for pancreatic cancer. Front. Oncol. 12:989077. doi: 10.3389/fonc.2022.989077
Received: 08 July 2022; Accepted: 19 October 2022;
Published: 01 December 2022.
Edited by:
Todd M. Pitts, University of Colorado Anschutz Medical Campus, United StatesReviewed by:
Yan Chen, Sichuan Cancer Hospital, ChinaWafaa M. Rashed, Children’s Cancer Hospital, Egypt
Copyright © 2022 Mugiyanto, Adikusuma, Irham, Huang, Chang and Kuo. This is an open-access article distributed under the terms of the Creative Commons Attribution License (CC BY). The use, distribution or reproduction in other forums is permitted, provided the original author(s) and the copyright owner(s) are credited and that the original publication in this journal is cited, in accordance with accepted academic practice. No use, distribution or reproduction is permitted which does not comply with these terms.
*Correspondence: Wei-Chiao Chang, d2NjQHRtdS5lZHUudHc=; Chun-Nan Kuo, OTcyOTRAdy50bXUuZWR1LnR3