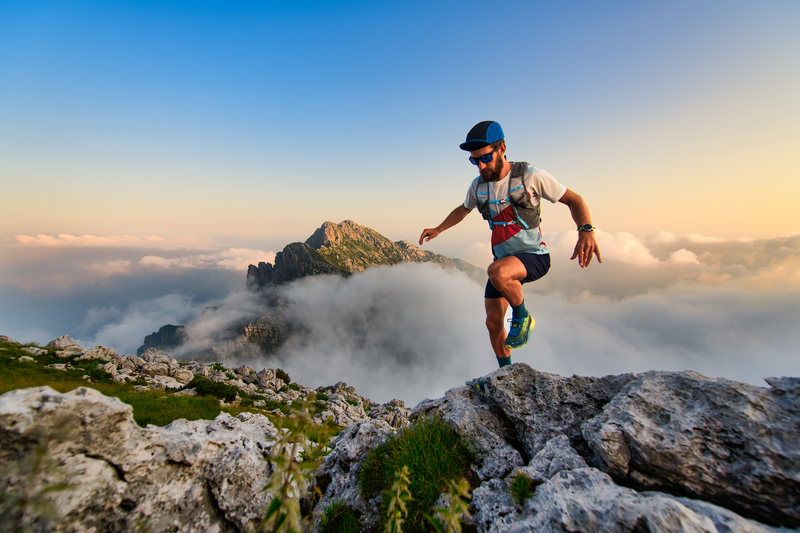
94% of researchers rate our articles as excellent or good
Learn more about the work of our research integrity team to safeguard the quality of each article we publish.
Find out more
REVIEW article
Front. Oncol. , 20 July 2022
Sec. Cancer Genetics
Volume 12 - 2022 | https://doi.org/10.3389/fonc.2022.943253
This article is part of the Research Topic Circulating Tumor DNA in Cancer: A Role as a Response and Monitoring “Next-Generation” Biomarker in Cancer therapy View all 11 articles
Cancer cells release nucleic acids, freely or associated with other structures such as vesicles into body fluids, including blood. Among these nucleic acids, circulating tumor DNA (ctDNA) has emerged as a minimally invasive biomarker for tumor molecular profiling. However, certain biological characteristics of ctDNA are still unknown. Here, we provide an overview of the current knowledge about ctDNA biological features, including size and structure as well as the mechanisms of ctDNA shedding and clearance, and the physio-pathological factors that determine ctDNA levels. A better understanding of ctDNA biology is essential for the development of new methods that enable the analysis of ctDNA.
Cancer ranks as the leading cause of death worldwide and the main barrier that hinders life expectancy (1). The emergence of precision medicine in the field of medical oncology brought a halo of hope for cancer patients and has improved notably in the past few decades due to the rapid expansion of knowledge in cancer genomics and the identification of targetable genomic biomarkers (2). Although the discovery of therapeutic biomarkers marked a turning point in cancer patients’ treatment, several challenges arose with them. For example, in lung cancer patients, the increasing number of biomarkers to be assessed compromises the availability of tumor tissue. Moreover, tissue biopsy, apart from being a very invasive procedure that can imply potential complications for the patients, does not reflect tumor heterogeneity, making it more difficult to have an overview of the molecular characteristics of the tumor (3, 4).
In this scenario, liquid biopsy arose as a minimally invasive approach, particularly useful when tumor tissue is inadequate or non-existent, that enables the identification of significant tumor-derived biomarkers throughout the course of the disease, including resistance mutations (5, 6). Different components can be isolated from body fluids and used in liquid biopsy analysis such as circulating tumor cells (CTCs), extracellular vesicles (EVs), tumor-educated platelets (TEPs), or circulating tumor DNA (ctDNA) (7). Among them, we are going to focus on ctDNA as it is the biomarker with more diagnostic and prognostic potential.
The cell free-DNA (cfDNA) was first described in healthy individuals by Mandel et al. in 1948 (8) and it was not until the year 1977 that Leon et al. found out increased levels of cfDNA in the serum of cancer patients (9), highlighting its huge potential as a tumor biomarker. cfDNA is generally at a concentration between 0 and 100 ng/mL in the blood of healthy patients, and is upped to >1000 ng/mL in cancer patients (4, 10). The fraction of plasma cfDNA derived from tumor cells, known as ctDNA, is the most extensively studied and the most used non-invasive alternative, from a clinical point of view, for the molecular characterization of solid tumors, including non-small-cell lung cancer (NSCLC), colorectal cancer (CRC), breast cancer (11), head and neck (12) and melanoma (13). ctDNA was first validated in clinical oncology by examining the KRAS/BRAF mutation in CRC patients (14) and then, it was introduced into clinical practice for the detection of mutations in the EGFR gene in NSCLC (15). Since then, the interest in this biomarker has exponentially risen being the topic of more than 870 publications in 2021 (Web of Science™ database, Figure 1) and being currently used in 359 different trials, listed in the database ClinicalTrials.gov.
Figure 1 ctDNA publications: Number of publications with ctDNA as a topic between 1990 and 2021, collected in Web of ScienceTM database.
Although liquid biopsies often refer to blood biopsies, other biofluids such as urine, saliva, cerebrospinal fluid (CSF), pleural effusion, pericardial effusion, and ascites effusion, can be also used (16, 17). In this way, malignant effusions that occur as a consequence of disease progression are highly informative. Indeed, tumors shed higher amounts of ctDNA into nearby body fluids than into the bloodstream (18). Moreover, peritoneal washings, which are routinely performed in surgeries of ovarian cancer patients, have been shown to be useful for BRCA testing (19). Therefore, although obtaining these biofluids may be a more aggressive procedure, they constitute an informative source for biomarker testing (18).
The study of ctDNA has multiple potential uses in oncology such as early diagnosis, tumor molecular profiling, or early detection of resistance mutations. ctDNA levels correlate well with tumor bulk and therefore it can be used as a surrogate for tumor size and staging (20, 21). In the same way, fluctuations in ctDNA levels have been shown to correlate well with the course of the disease, being an adequate approach for noninvasive tumor response to treatment monitoring for many cancer types (22–24).
However, the structure and origin of ctDNA, as well as the mechanisms of ctDNA shedding, filtering, degradation, and clearance remain unclear. In this review, we summarize the dynamics of extracellular tumor DNA, including the balance between ctDNA release and clearance and the influence of clinicopathological factors in these processes.
cfDNA comprises small fragments of double-stranded nuclear (coding and non-coding) and mitochondrial DNA (mtDNA) of approximately 40-200 base pairs (bp) in size, with a peak at about 166 bp that corresponds with nucleosome-associated DNA fragments (4, 25). Although the main source of cfDNA is the hematopoietic system (55% white blood cells and 30% erythrocyte progenitors) (25), there is still a huge interest in understanding how different organs contribute to the overall amount of cfDNA in the physiological and pathological conditions.
ctDNA can be released by a multitude of mechanisms, not only when cells die via apoptosis, necrosis, oncosis, ferroptosis, pyroptosis, and phagocytosis, but also by senescence or the active secretion in extracellular vesicles (EVs) and mtDNA egestion (26, 27) (Figure 2).
Figure 2 Mechanisms of ctDNA release: Tumor cells shed DNA into the bloodstream by different types of cell death, including necrosis (larger fragments of >200 bp), apoptosis (shorter fragments of <200 bp enriched with tumor-derived genomic alterations), pyroptosis, phagocytosis, oncosis or ferroptosis, but also by non-death associated mechanisms such as senescence, or the active secretion of EVs and mtDNA. Thus, ctDNA analysis provides tumor-relevant clinical information.
Fragment length and nucleosome occupancy might provide clues for cfDNA shedding mechanisms. Indeed, short fragments of <200 bp are assumed to be released during apoptosis as a consequence of caspase-dependent cleavage. Multiple of these fragments are packed in apoptotic blebs and phagocytized by macrophages, to be finally released into the blood and lymphatic circulation (28, 29). Interestingly, shorter fragments (<100 bp) might be enriched with ctDNA and mtDNA which preferentially carried tumor-derived genomic alterations (30, 31). Indeed, a higher ctDNA fragmentation pattern was observed in melanoma, lung cancer, and metastatic CRC patients with high levels of mutation burden compared with healthy individuals (32). Conversely, large fragments of >200 bp are originated during the necrosis process (33), however, the contribution of necrosis in the amount of cfDNA remains unclear (34). Interestingly, DNA of necrotic cells can be further degraded by DNase I, and necrotic cells can be engulfed by macrophages, originating smaller fragments of circulating DNA (35, 36). For all those reasons, the development of methods based on cfDNA size and fragmentation pattern is crucial to enhance the enrichment of ctDNA and consequently, improve the sensitivity of methods for ctDNA analysis. Furthermore, the distribution of cfDNA fragments with different sizes is important, since it reflects cfDNA integrity. Although ctDNA derived from apoptotic bodies would be more informative in terms of tumor molecular information, cfDNA integrity seems to be higher in cancer patients compared to healthy individuals, suggesting that necrotic cell death plays an important role in ctDNA release, especially in advanced stages and aggressive tumors (37, 38). This observation could be explained by the fact that healthy cells die primarily by apoptosis, while malignant cells die not only from apoptosis but also from necrosis or autophagy (9). In this regard, cancer cells could activate autophagy to obtain an alternative energy source from the digestion of their damaged organelles or their self-digestion, shedding ctDNA as a consequence (39).
DNA from necrotic or apoptotic cells can be also released into the circulation by different immune cell types; however, it is not clear how much each mechanism contributes to the amount of ctDNA. After the phagocytosis of necrotic and apoptotic cells, macrophages or other scavenger cells digest the DNA into smaller fragment sizes and release them into the tissue microenvironment and bloodstream, actively or dying (36, 40).
ctDNA can also be actively released by living tumor cells, from primary tumors or metastases via EVs. Like cfDNA, there is a wide variety of EVs in terms of size whose role in cancer and, specifically, in the transport of ctDNA between distant tissues for cell communication seems to differ. In this sense, Vagner et al. showed that both ctDNA and EVs size seems to be a key element in genomic alteration transport (41). Indeed, large vesicles (from 100 nm up to 1 μm in diameter) from prostate cancer patients, such as microvesicles or apoptotic bodies, appear to be enriched with smaller fragments of ctDNA (<200 pb), compared with small EVs, from 30 to 150 nm in diameter, such as exosomes (41, 42). Still, nanoscale EV-derived DNA (approximately 114 nm average size in stage-I EV samples) has been demonstrated to be a superior mutation detection method in early-stage NSCLC compared to cfDNA (43). In line with these data, additional studies have identified the presence of DNA in EVs isolated from cancer patient samples and described the identification of different mutations in oncogenes such as KRAS or TP53 (44–47). However, the proportion of ctDNA engulfed into EVs actively released by tumor cells and the effect of different treatments on this active secretion is not clear (41, 48).
Irrespective of the mechanism of ctDNA shedding; nucleosome footprints, DNA methylation profiles, DNA preferred end motifs, and genetic alterations can be used to characterize and identify the origin of cfDNA as they carry information from the original tissues (49–51) (Figure 3). In this regard, certain human genomic locations have been described as preferential ends when ctDNA is generated (52), suggesting that DNA cleavage is a non-random process. Interestingly, a greater end motif diversity has been associated with cancer patients (53, 54), suggesting that ctDNA tail motifs could be used to enhance the performance of cancer diagnosis by identifying the fragments of cfDNA from tumor cells (ctDNA) and filtering out fragments from healthy cells. Another interesting approach for determining the tissue source of ctDNA was proposed by Snyder et al. (49), who hypothesized that it is feasible to identify cfDNA origin based on nucleosome positioning. Nucleosomes are distributed along DNA following different patterns that correlate with characteristic epigenetic features of different cell types or even according to cancer types. Matching the epigenetic footprint of these ctDNA fragments against reference databases would enable the molecular classification of cancers of unknown origin. In line with these data, the stability of DNA methylation and the presence of cell-specific methylation patterns can also contribute to the identification of tumor origin or even the detection of metastasis through cfDNA analysis (55). Specifically, the analysis of differentially methylated regions in colon and liver tissues enabled the differentiation of patients with liver or colon cancer but also, the discrimination between colon cancer patients with and without liver metastasis (56). Indeed, the analysis of cfDNA methylation has been already approved by the FDA for its use in the clinic (57), being the Epi proColon test the first screening analyzing a cfDNA methylation biomarker approved in 2016 for colorectal cancer patients (58).
Figure 3 Biological features of ctDNA: The integrated analysis of ctDNA somatic alterations, methylation and fragmentomic information, improves ctDNA detection and provides useful information about original tissue.
The amount of cfDNA, and ctDNA in particular, depends on a balance between DNA shedding and DNA clearance. Overall, the half-life of cfDNA ranges from 16 minutes to 2.5 hours (59), as a consequence of the action of three main different mechanisms: (i) the action of DNases present in the bloodstream (60), (ii) the active clearance of nucleosomes and DNA and (iii) filtration in organs such as kidney or lymph nodes (Figure 4).
Figure 4 Mechanisms of ctDNA clearance: Kupffer cells from the liver are primarily responsible for ctDNA clearance, followed by circulating enzymes and immune system cells and other filtering organs such as kidneys, spleen and lymph nodes.
ctDNA clearance can be carried out by various filtering organs (60). Kupffer cells within the liver are responsible for clearing the majority of cfDNA, specifically longer fragments (61, 62), followed by kidneys, which are involved in DNA fragmentation through their deoxyribonuclease activity (62). In this way, in vivo experiments injecting radiolabeled mononucleosomes in mice demonstrated that the liver removed approximately 70 to 85% of the nucleosomes within 10 min (61). The macrophages of the spleen and lymph nodes play also a minor role in ctDNA clearance (Figure 4). In addition to these organs, lymphatic drainage may constitute the main source of ctDNA clearance within the tumor microenvironment (60). In cancer patients, cfDNA level is higher than in healthy individuals in part due to the excess of cell death by the whole set of mechanisms aforementioned, which leads to the overload of the clearance systems and subsequent accumulation. Nevertheless, the kinetic dynamics of ctDNA in cancer patients need to be further studied.
Finally, the association with molecular or macromolecular complexes, as well as encapsulation in EVs, prevent the rapid degradation of ctDNA by circulating enzymes and immune system cells (63). Another factor that seems to play a role in ctDNA clearance is fragment size, but it is still not clear how they affect half-life.
The first time that cfDNA was measured in different cancer types was in 1977 by Leon et al. (9), who reported that levels of cfDNA in patients with various cancers were higher compared with healthy individuals. A few years later, in 1989, Stroun et al. (64) stated that increased levels of cfDNA in cancer patients were caused by a fraction of DNA released into the bloodstream by cancer cells, this portion of cfDNA was named ctDNA. Nowadays, it is well established that ctDNA levels vary depending on the cancer type (Figure 5). It has been especially characterized that tumors located in the central nervous system release the lowest levels of ctDNA into the bloodstream due to the blood-brain barrier (21, 65, 66). Of note, more than 90% of patients with gliomas did not harbor detectable levels of ctDNA according to Huang et al. (67). Similarly, Zill et al. (68) analyzed 25,578 samples from 21,807 patients in more than 50 tumor types, reporting a ctDNA detection rate of 93%. Remarkably, no differences were found in terms of ctDNA detection except for patients with brain tumors or brain-only metastases, who shed significantly less ctDNA into the bloodstream. Likewise, some studies have also pointed out that patients with visceral metastases have higher levels of ctDNA than those with brain metastases (69–72). Noteworthy, about 30% of cancer patients develop intracranial metastases, a severe complication that decisively affects the patient’s prognosis and quality of life (73,74). Thus, it would be important to optimize the detection of ctDNA for these patients in other body fluids such as CSF. In addition, ctDNA detection is rather challenging in medulloblastomas, or kidney, prostate or thyroid cancer. On the other hand, ctDNA can be easily detected in samples from advanced stages of ovarian, liver, pancreas, bladder, colon, lung, stomach, breast, liver, esophagus, and head and neck cancer patients as well as neuroblastoma and melanoma patients (21).
Figure 5 ctDNA amount in different cancer types: The amount of ctDNA is correlated with tumor type, size, stage and metastasis.
Interestingly, there are also variations within the same type of cancer depending on tumor histology. For example, in lung cancer, a higher percentage of ctDNA detection has been described in squamous tumors compared to adenocarcinomas. The most plausible explanation is that squamous tumors have a more necrotic profile (75). These results have also been observed in patients with triple-negative breast cancer, whose ctDNA levels are higher than those of other breast cancer subtypes, which can be related to a higher rate of necrosis and cell proliferation (76, 77).
Lastly, tumors harboring certain alterations such as TP53 mutations and copy number gains seem to have increased ctDNA shedding, which may be due to increased metabolic activity or cellular turnover (71). In this regard, TP53 alterations have been suggested to be markers of aggressiveness and poor prognosis (78).
The amount of ctDNA has been associated with tumor size, stage, and metastasis in multiple studies (Figure 5). Specifically, a retrospective study of serially collected liquid biopsy samples from 40 ovarian cancer patients demonstrated a significant correlation between lesion volume and ctDNA level (79). Another study that analyzed samples from 640 patients with different tumor types described a clear correlation between ctDNA and cancer stage, reaching higher levels in patients with advanced disease and lower levels in premalignant and early-stage cancers (21). In line with these results, analyzing samples from more than 20,000 patients with different tumors, Zill and colleagues showed that those patients with premalignant lesions or earlier stages shed less ctDNA than those with advanced stages (68). Specifically, in NSCLC patients, Chabon et al. were able to detect ctDNA in 42%, 67%, and 88% of patients with stage I, II, and III diseases respectively (80). In fact, 50% of localized tumors shed ctDNA without reaching 0.01% of the ctDNA level (80); whereas advanced-stage tumors release concentrations of ctDNA than can exceed 10% of the cfDNA (21).
On the other hand, in ctDNA-positive patients, tumor size and volume correlate broadly with ctDNA levels, as measured by the mean of variant allele frequency (VAF) of single nucleotide variants detected in plasma ctDNA (81). Currently, it is not well established how ctDNA should be quantified. This issue is especially controversial in tumors that do not harbor druggable mutations. In this sense, it is not clear whether it is more appropriate to select the highest VAF among all detected mutations or to take all of VAFs into account through summation, arithmetic mean, or other approaches.
In summary, ctDNA levels increase proportionally according to tumor burden, disease stage, and metastasis, highlighting the use of ctDNA as a prognostic biomarker. Indeed, it is well established that patients with high levels of ctDNA have worsened survival outcomes compared with those with lower or even undetectable levels of ctDNA (82–85).
Numerous studies show that ctDNA levels correlate well with tumor load and therefore ctDNA dynamics can be used as a surrogate of treatment response (86–88). In addition, the modification of the ctDNA methylation profile has been proposed as an alternative biomarker for treatment response (89). It has been shown that the type of treatment, as well as the time interval between exposures and the dose, may rate affect ctDNA shedding. In this way, it has been suggested that targeted therapies used in cancer patients, such as EGFR or ALK tyrosine kinase inhibitors, promote faster ctDNA clearance than immunotherapy (90). Furthermore, cytotoxic therapies such as chemotherapy or ionizing radiation seem to increase cfDNA levels due to cellular senescence (91, 92). Of note, it is well established that some chemotherapy agents produce leukopenia. cfDNA from dying cells dilutes ctDNA in wild-type (wt) DNA leading to decreased levels in VAF, which may bias results. Conversely, other cancer treatments do not release as much cfDNA due to its mainly cytostatic effect, implying cell growth arrest (93). In the neoadjuvant setting, ctDNA has been shown to correlate well with tumor response to treatment. Recently, NADIM investigators have shown that ctDNA clearance after neoadjuvant chemo-immunotherapy outperformed tumor response to treatment measured by CT-scans and according to RECIST criteria in the prediction of survival (94). Similarly, a significant association between pathological complete response and ctDNA clearance was reported in the CheckMate 816 trial (95). Measurement of residual disease following neoadjuvant treatment that accurately predicts long-term survival is an essential requirement for clinical trial development. Although further studies are needed, ctDNA postulates as an early surrogate of survival being a promising trial endpoint in the neoadjuvant setting.
Finally, patients with surgically resected tumors show a sharp drop in ctDNA levels after surgery (59). However, the amount of nonspecific cfDNA increased after tumor resection (96), due to injury of surrounding tissue during surgery. In this sense, the appropriate time point for plasma collection after surgery needs to be established. In these patients, ctDNA detection allows monitoring of minimal residual disease (MRD) after tumor resection (97). Several platforms with exceptional sensitivities such as cancer personalized profiling by deep sequencing (CAPP-Seq) (98) targeted error correction sequencing (TEC-Seq) (99), the Tracking Cancer Evolution Through Therapy (TRACERx, Signatera) (81, 100) or CancerSEEK5 multiplex PCR (mPCR) (101) have been shown to be useful for detection of minimal residual disease (MRD) or early detection of cancer. In this regard, it appears that measuring not just ctDNA can boost sensitivity. Combining ctDNA analysis with the study of informative methylation regions improves sensitivity (102) (Figure 3).
The use of ctDNA to noninvasively assess tumor genomic variants is increasing. However, some pre-analytical and analytical issues may affect the detection and quantification of ctDNA.
Regarding starting material, blood plasma is a preferential choice compared with serum because wt cfDNA released from leukocytes during the clotting process in serum samples dilutes ctDNA in wt DNA (103, 104). Particularly, Soo et al. reported a higher level of cfDNA in serum (481 ng/mL) than in plasma (17.7 ng/mL). Of note in a cohort of 33 pre-treatment serum and 75 pre-treatment plasma samples from patients with diffuse large B cell lymphoma, Soo et al. were able to detect more genomic alterations in plasma samples (186 vs. 22 mutations) with higher tumor allele fraction (2.8% vs. 0.85%) (105), compared with serum samples. In addition, plasma samples have shown less inter-patient variability (106). The use of K2 EDTA tubes to collect plasma samples is therefore recommended when samples are processed within 6 hours after blood extraction (107). For longer periods between extraction and processing, the use of special collection tubes with stabilizing agents is recommended. Of note successful preservation of cfDNA over 14 days at room temperature is possible using collection tubes with stabilizing agents (108).
Concerning sample processing, the complete removal of any cellular component is essential. For this goal, the best option is a two-step centrifugation at 1600g for 10 minutes for plasma isolation (109). According to this recommendation, Herrera et al. reported less concentration of cfDNA in plasma samples that were centrifuged twice compared with samples that were centrifuged only once (13 µg/l vs. 819 µg/l), revealing that cfDNA concentrations were contaminated with genomic DNA (110). These observations confirm that the second centrifugation step is crucial for ctDNA analysis. Finally, it is well known that ctDNA integrity is better conserved as cfDNA extracts compared to plasma when samples are stored at -80°C and avoiding freeze-thaw cycles (103).
As already mentioned, body fluids other than blood have shown a higher concentration of cfDNA compared to blood samples in patients with lung adenocarcinoma with EGFR mutations (1.90 vs. 0.36 ng/µL; p=0.0130). Likewise, CSF from patients with primary brain tumors such as glioblastoma, glioma, or primary central nervous system lymphoma showed higher amounts of ctDNA compared to peripheral blood (18, 19).
Technical procedures for cfDNA isolation can be classified into three categories: phase isolation, silicon membrane-based spin column, and magnetic bead-based isolation. Phase isolation methods may lead to a high cfDNA isolation yield and a wide range of DNA fragment sizes. On the other hand, spin column and magnetic bead-based methods have lower efficiency but show a higher selective recovery for DNA fragments of a certain size (111, 112). Specifically, cfDNA purification using magnetic beads appears to recover higher amounts of small cfDNA fragments compared to silica membrane methods (113). In any case, automated processing should be performed to reduce operator variability (114). Nevertheless, within automatic methods, Pérez-Barrios et al. reported different recovery of mono-, di- and tri-nucleosomes DNA fragments when analyzing 34 cfDNA samples obtained from 17 plasma samples from cancer patients extracted by Maxwell® RSC ccfDNA Plasma Kit (Promega Corporation, Madison, WI, USA) and MagNA Pure Compact Nucleic Acid Isolation Kit I (Roche Diagnostics, Penzberg, Germany) methodologies (112).
Currently, several platforms are available for noninvasive biomarker testing some of which have received approval from regulatory agencies. There is a wide range of reported sensitivities of the different methodological approaches, in this way PCR-based approaches have a significantly lower limit of detection (LOD) compared to other technologies such as dPCR and NGS (115). Although dPCR offers an ultra-high sensitivity for ctDNA analysis, only a few known mutations can be tested at a time, whereas NGS technologies allow the screening of multiple genomic alterations, known or unknown. In addition, NGS enables the combination of genomic data and epigenomic signatures, which may improve sensitivity (116) (Figure 3). In any case, the knowledge of the limitations of the different technical approaches for ctDNA analysis is crucial for the accurate interpretation of the results (117).
cfDNA input remains the major limiting factor, and for most techniques using less than 20 ng of cfDNA may impair results. A study by Zhang Y et al. showed that the sensitivity declined from 82.6% to 46.7% when using cfDNA inputs of ≥ 5 ng per reaction and < 2 ng, respectively (118). Furthermore, several comparative studies have clearly reported that, among other technical factors, discordant calls mostly occur at low VAF (115, 119), and therefore VAFs should always be reported in clinical reports.
Finally, it is important to point out that clonal hematopoiesis (CH) constitutes an important source of false-positive calls. CH is defined by the presence of a somatic mutation in blood or hematopoietic progenitor cells, but without other diagnostic criteria for hematological malignancy. It is more frequent in aged patients and patients with solid tumors and of course, it is more likely to be detected with deeper sequencing approaches (120). Importantly, CH-derived mutations can lead to erroneous sequencing results which thereby might guide erratic treatment recommendations (121).
Liquid biopsy overcomes some tissue biopsy limitations such as tumor heterogeneity, tissue availability, and risks associated with the invasive procedure. Among the biological components of body fluids, ctDNA has emerged as a pivotal analyte for the management of cancer patients. However, ctDNA detection and quantification are affected by several physio-pathological conditions and a deeper knowledge of factors affecting ctDNA kinetics is needed. The size fragment pattern, nucleosome, and methylation profile of ctDNA may differ according to the original tissue and the mechanism of release, which may be clinically informative, and methodological approaches capable to explode this information are of particular interest.
ES-H, RS-B and AR drafted and critically revised the manuscript. All authors reviewed the draft. All authors contributed to the article and approved the submitted version.
ES-H was funded by the Consejería de Ciencia, Universidades e Innovación of the Comunidad de Madrid (Doctorados Industriales of the Comunidad de Madrid IND2019/BMD-17258). RS-B was funded by the Ministerio de Ciencia e Innovación (Programa Estatal de Investigación, Desarrollo e Innovacion Orientada a los Retos de la Sociedad, RTC2019-007359-1).
Authors ES-H and VG-R were employed by Atrys Health. AR reports the following conflict of interest: Consulting or Advisory Role: Takeda, AstraZeneca. Research Funding: Bristol Myers Squibb Foundation (Inst), Boehringer Ingelheim (Inst), Takeda (Inst) Expert Testimony: Vivo Diagnostics. MP reports grants, personal fees, and travel expenses from BristolMyers Squibb, Roche, and AstraZeneca; and personal fees from Merck Sharpe & Dohme and Takeda, outside the submitted work.
The remaining authors declare that the research was conducted in the absence of any commercial or financial relationships that could be construed as a potential conflict of interest.
All claims expressed in this article are solely those of the authors and do not necessarily represent those of their affiliated organizations, or those of the publisher, the editors and the reviewers. Any product that may be evaluated in this article, or claim that may be made by its manufacturer, is not guaranteed or endorsed by the publisher.
bp, base pair; cfDNA, cell-free DNA; CH, clonal hematopoiesis; CRC, colorectal cancer; CTC, circulating tumor cell; CSF, cerebrospinal fluid; ctDNA, circulating tumor DNA; ddPCR, digital droplet PCR; dPCR, digital PCR; EVs, extracellular vesicles; LOD, limit of detection; MRD, minimal residual disease; mtDNA, mitochondrial DNA; NGS, next-generation sequencing; NSCLC, non-small-cell lung cancer; VAF, variant allele frequency; wt, wild-type; ALK, ALK receptor tyrosine kinase; BRAF, B-Raf proto-oncogene, serine/threonine kinase; EGFR, epidermal growth factor receptor; KRAS, KRAS proto-oncogene, GTPase; TP53, tumor protein p53.
1. Sung H, Ferlay J, Siegel RL, Laversanne M, Soerjomataram I, Jemal A, et al. Global Cancer Statistics 2020: GLOBOCAN Estimates of Incidence and Mortality Worldwide for 36 Cancers in 185 Countries. CA Cancer J Clin (2021) 71(3):209–49. doi: 10.3322/caac.21660
2. Tsimberidou AM, Fountzilas E, Nikanjam M, Kurzrock R. Review of Precision Cancer Medicine: Evolution of the Treatment Paradigm. Cancer Treat Rev (2020) 86:102019. doi: 10.1016/j.ctrv.2020.102019
3. Bennett CW, Berchem G, Kim YJ, El-Khoury V. Cell-Free DNA and Next-Generation Sequencing in the Service of Personalized Medicine for Lung Cancer. Oncotarget (2016) 7(43):71013–35. doi: 10.18632/oncotarget.11717
4. Schwarzenbach H, Hoon DSB, Pantel K. Cell-Free Nucleic Acids as Biomarkers in Cancer Patients. Nat Rev Cancer (2011) 11:426–37. doi: 10.1038/nrc3066
5. Abate RE, Frezzetti D, Maiello MR, Gallo M, Camerlingo R, De Luca A, et al. Next Generation Sequencing-Based Profiling of Cell Free DNA in Patients With Advanced non-Small Cell Lung Cancer: Advantages and Pitfalls. Cancers (2020) 12(12):3804. doi: 10.3390/cancers12123804
6. Planchard D, Popat S, Kerr K, Novello S, Smit EF, Faivre-Finn C, et al. Updated Version Published 15 September 2020 by the ESMO Guidelines Committee Metastatic non-Small Cell Lung Cancer: ESMO Clinical Practice Guidelines for Diagnosis, Treatment and Follow-Up †. Ann Oncol (2018) 4:192–237. doi: 10.1093/annonc/mdy275
7. Romero A, Serna-Blasco R, Calvo V, Provencio M. Use of Liquid Biopsy in the Care of Patients With Non-Small Cell Lung Cancer. Curr Treat Options Oncol (2021) 22(10):86. doi: 10.1007/s11864-021-00882-9
8. Mandel P, Metais P. Les Acides Nucléiques Du Plasma Sanguin Chez L’homme [Nuclear Acids In Human Blood Plasma]. C R Seances Soc Biol Fil (1948) 142(3-4):241–3.
9. Leon SA, Shapiro B, Sklaroff DM, Yaros MJ. Free DNA in the Serum of Cancer Patients and the Effect of Therapy. Cancer Res (1977) 37(3):646–50.
10. Thierry AR, El Messaoudi S, Gahan PB, Anker P, Stroun M. Origins, Structures, and Functions of Circulating DNA in Oncology. Cancer Metastasis Rev (2016) 35(3):347–76. doi: 10.1007/s10555-016-9629-x
11. Zhang L, Riethdorf S, Wu G, Wang T, Yang K, Peng G, et al. Meta-Analysis of the Prognostic Value of Circulating Tumor Cells in Breast Cancer. Clin Cancer Res (2012) 18(20):5701–10. doi: 10.1158/1078-0432.CCR-12-1587
12. Kulasinghe A, Schmidt H, Perry C, Whitfield B, Kenny L, Nelson C, et al. A Collective Route to Head and Neck Cancer Metastasis. Sci Rep (2018) 8(1):746. doi: 10.1038/s41598-017-19117-9
13. Bardelli A, Pantel K. Liquid Biopsies, What We Do Not Know (Yet). Cancer Cell Cell Press (2017) 31(2):172–9. doi: 10.1016/j.ccell.2017.01.002
14. Thierry AR, Mouliere F, El Messaoudi S, Mollevi C, Lopez-Crapez E, Rolet F, et al. Clinical Validation of the Detection of KRAS and BRAF Mutations From Circulating Tumor DNA. Nat Med (2014) 20(4):430–5. doi: 10.1038/nm.3511
15. Cescon DW, Bratman SV, Chan SM, Siu LL. Circulating Tumor DNA and Liquid Biopsy in Oncology. Nat Cancer (2020) 1(3):276–90. doi: 10.1038/s43018-020-0043-5
16. Siravegna G, Marsoni S, Siena S, Bardelli A. Integrating Liquid Biopsies Into the Management of Cancer. Nat Rev Clin Oncol (2017) 14(9):531–48. doi: 10.1038/nrclinonc.2017.14
17. Villatoro S, Mayo-de-las-Casas C, Jordana-Ariza N, Viteri-Ramírez S, Garzón-Ibañez M, Moya-Horno I, et al. Prospective Detection of Mutations in Cerebrospinal Fluid, Pleural Effusion, and Ascites of Advanced Cancer Patients to Guide Treatment Decisions. Mol Oncol (2019) 13(12):2633–45. doi: 10.1002/1878-0261.12574
18. Pérez-Barrios C, Sánchez-Herrero E, Garcia-Simón N, Barquín M, Clemente MB, Provencio M, et al. ctDNA From Body Fluids is an Adequate Source for EGFR Biomarker Testing in Advanced Lung Adenocarcinoma. Clin Chem Lab Med (2021) 59(7):1221–9. doi: 10.1515/cclm-2020-1465
19. Barquín M, Maximiano C, Pérez-Barrios C, Sanchez-Herrero E, Soriano M, Colmena M, et al. Peritoneal Washing Is an Adequate Source for Somatic BRCA1/2 Mutation Testing in Ovarian Malignancies. Pathol Res Pract (2019) 215(2):392–4. doi: 10.1016/j.prp.2018.10.028
20. Vu P, Khagi Y, Riviere P, Goodman A, Kurzrock R. Total Number of Alterations in Liquid Biopsies Is an Independent Predictor of Survival in Patients With Advanced Cancers. JCO Precis Oncol (2020) 4:PO.19.00204. doi: 10.1200/PO.19.00204
21. Bettegowda C, Sausen M, Leary RJ, Kinde I, Wang Y, Agrawal N, et al. Detection of Circulating Tumor DNA in Early- and Late-Stage Human Malignancies. Sci Transl Med (2014) 6(224):224ra24. doi: 10.1126/scitranslmed.3007094
22. Provencio M, Torrente M, Calvo V, Gutiérrez L, Pérez-Callejo D, Pérez-Barrios C, et al. Dynamic Circulating Tumor DNA Quantificaton for the Individualization of Non-Small-Cell Lung Cancer Patients Treatment. Oncotarget (2017) 8(36):60291–8. doi: 10.18632/oncotarget.20016
23. Provencio M, Torrente M, Calvo V, Pérez-Callejo D, Gutiérrez L, Franco F, et al. Prognostic Value of Quantitative ctDNA Levels in non Small Cell Lung Cancer Patients. Oncotarget (2018) 9(1):488–94. doi: 10.18632/oncotarget.22470
24. García-Saenz JA, Ayllón P, Laig M, Acosta-Eyzaguirre D, García-Esquinas M, Montes M, et al. Tumor Burden Monitoring Using Cell-Free Tumor DNA Could be Limited by Tumor Heterogeneity in Advanced Breast Cancer and Should be Evaluated Together With Radiographic Imaging. BMC Cancer (2017) 17(1):210. doi: 10.1186/s12885-017-3185-9
25. Kustanovich A, Schwartz R, Peretz T, Grinshpun A. Life and Death of Circulating Cell-Free DNA. Cancer Biol Ther (2019) 20(8):1057–67. doi: 10.1080/15384047.2019.1598759
26. Keller L, Belloum Y, Wikman H, Pantel K. Clinical Relevance of Blood-Based ctDNA Analysis: Mutation Detection and Beyond. Br J Cancer (2021) 124(2):345–58. doi: 10.1038/s41416-020-01047-5
27. Stroun M, Lyautey J, Lederrey C, Olson-Sand A, Anker P. About the Possible Origin and Mechanism of Circulating DNA: Apoptosis and Active DNA Release. Clinica Chimica Acta (2001) 313(1-2):139–42. doi: 10.1016/s0009-8981(01)00665-9
28. Enari M, Sakahira H, Yokoyama H, Okawa K, Iwamatsu A, Nagata S. A Caspase-Activated DNase That Degrades DNA During Apoptosis, and its Inhibitor ICAD. Nature (1998) 391(6662):43–50. doi: 10.1038/34112
29. Mouliere F, Robert B, Peyrotte E, Del Rio M, Ychou M, Molina F, et al. High Fragmentation Characterizes Tumour-Derived Circulating DNA. PLoS One (2011) 6(9):e23418. doi: 10.1371/journal.pone.0023418
30. Diehl F, Li M, Dressman D, He Y, Shen D, Szabo S, et al. Detection and Quantification of Mutations in the Plasma of Patients With Colorectal Tumors. Proc Natl Acad Sci U S A (2005) 102(45):16368–73. doi: 10.1073/pnas.0507904102
31. Jiang P, Chan CWM, Chan KCA, Cheng SH, Wong J, Wong VWS, et al. Lengthening and Shortening of Plasma DNA in Hepatocellular Carcinoma Patients. Proc Natl Acad Sci U S A (2015) 112(11):E1317–25. doi: 10.1073/pnas.1500076112
32. El Messaoudi S, Mouliere F, Du Manoir S, Bascoul-Mollevi C, Gillet B, Nouaille M, et al. Circulating DNA as a Strong Multimarker Prognostic Tool in Metastatic Colorectal Cancer Patients. Clin Cancer Res (2016) 22(12):3067–77. doi: 10.1158/1078-0432.CCR-15-0297
33. Walker NI, Harmon BV, Gobé GC, Kerr JF. Patterns of Cell Death. Methods Achiev Exp Pathol (1988) 13:18–54.
34. Heitzer E, Auinger L, Speicher MR. Cell-Free DNA and Apoptosis: How Dead Cells Inform About the Living. Trends Mol Med (2020) 26(5):519–28. doi: 10.1016/j.molmed.2020.01.012
35. Stephan F, Marsman G, Bakker LM, Bulder I, Stavenuiter F, Aarden LA, et al. Cooperation of Factor Vii-Activating Protease and Serum Dnase I in the Release of Nucleosomes From Necrotic Cells. Arthritis Rheumatol (2014) 66(3):686–93. doi: 10.1002/art.38265
36. Jiang N, Reich CF 3rd, Pisetsky DS. Role of Macrophages in the Generation of Circulating Blood Nucleosomes From Dead and Dying Cells. Blood (2003) 102(6):2243–50. doi: 10.1182/blood-2002-10-3312
37. Agostini M, Pucciarelli S, Enzo MV, Del Bianco P, Briarava M, Bedin C, et al. Circulating Cell-Free DNA: A Promising Marker of Pathologic Tumor Response in Rectal Cancer Patients Receiving Preoperative Chemoradiotherapy. Ann Surg Oncol (2011) 18(9):2461–8. doi: 10.1245/s10434-011-1638-y
38. Chen H, Sun LY, Zheng HQ, Zhang QF, Jin XM. Total Serum DNA and DNA Integrity: Diagnostic Value in Patients With Hepatitis B Virus-Related Hepatocellular Carcinoma. Pathology (2012) 44(4):318–24. doi: 10.1097/PAT.0b013e328353a24c
39. Sato K, Tsuchihara K, Fujii S, Sugiyama M, Goya T, Atomi Y, et al. Autophagy Is Activated in Colorectal Cancer Cells and Contributes to the Tolerance to Nutrient Deprivation. Cancer Res (2007) 67(20):9677–84. doi: 10.1158/0008-5472.CAN-07-1462
40. Stroun M, Maurice P, Vasioukhin V, Lyautey J, Lederrey C, Lefort F, et al. The Origin and Mechanism of Circulating DNA. Ann N Y Acad Sci (2000) 906:161–8. doi: 10.1111/j.1749-6632.2000.tb06608.x
41. Vagner T, Spinelli C, Minciacchi VR, Balaj L, Zandian M, Conley A, et al. Large Extracellular Vesicles Carry Most of the Tumour DNA Circulating in Prostate Cancer Patient Plasma. J Extracell Vesicles (2018) 7(1):1505403. doi: 10.1080/20013078.2018.1505403
42. Jeppesen DK, Fenix AM, Franklin JL, Higginbotham JN, Zhang Q, Zimmerman LJ, et al. Reassessment of Exosome Composition. Cell (2019) 177(2):428–45.e18. doi: 10.1016/j.cell.2019.02.029
43. Wan Y, Liu B, Lei H, Zhang B, Wang Y, Huang H, et al. Nanoscale Extracellular Vesicle-Derived DNA is Superior to Circulating Cell-Free DNA for Mutation Detection in Early-Stage Non-Small-Cell Lung Cancer. Ann Oncol (2018) 29(12):2379–83. doi: 10.1093/annonc/mdy458
44. Kahlert C, Melo SA, Protopopov A, Tang J, Seth S, Koch O, et al. Identification of Doublestranded Genomic Dna Spanning All Chromosomes With Mutated KRAS and P53 DNA in the Serum Exosomes of Patients With Pancreatic Cancer. J Biol Chem (2014) 289(7):3869–75. doi: 10.1074/jbc.C113.532267
45. Allenson K, Castillo J, San Lucas FA, Scelo G, Kim DU, Bernard V, et al. High Prevalence of Mutant KRAS in Circulating Exosome-Derived DNA From Early-Stage Pancreatic Cancer Patients. Ann Oncol (2017) 28(4):741–7. doi: 10.1093/annonc/mdx004
46. Kamyabi N, Abbasgholizadeh R, Maitra A, Ardekani A, Biswal SL, Grande-Allen KJ. Isolation and Mutational Assessment of Pancreatic Cancer Extracellular Vesicles Using a Microfluidic Platform. BioMed Microdevices (2020) 22(2):23. doi: 10.1007/s10544-020-00483-7
47. Wang ZY, Wang RX, Ding XQ, Zhang X, Pan XR, Tong JH. A Protocol for Cancer-Related Mutation Detection on Exosomal DNA in Clinical Application. Front Oncol (2020) 10:558106. doi: 10.3389/fonc.2020.558106
48. Lázaro-Ibáñez E, Lässer C, Shelke GV, Crescitelli R, Jang SC, Cvjetkovic A, et al. DNA Analysis of Low- and High-Density Fractions Defines Heterogeneous Subpopulations of Small Extracellular Vesicles Based on Their DNA Cargo and Topology. J Extracell Vesicles (2019) 8(1):1656993. doi: 10.1080/20013078.2019.1656993
49. Snyder MW, Kircher M, Hill AJ, Daza RM, Shendure J. Cell-Free DNA Comprises an in Vivo Nucleosome Footprint That Informs Its Tissues-Of-Origin. Cell (2016) 164(1-2):57–68. doi: 10.1016/j.cell.2015.11.050
50. Sun K, Jiang P, Chan KCA, Wong J, Cheng YKY, Liang RHS, et al. Plasma DNA Tissue Mapping by Genome-Wide Methylation Sequencing for Noninvasive Prenatal, Cancer, and Transplantation Assessments. Proc Natl Acad Sci U S A (2015) 112(40):E5503–12. doi: 10.1073/pnas.1508736112
51. Chan KCA, Jiang P, Sun K, Cheng YKY, Tong YK, Cheng SH, et al. Second Generation Noninvasive Fetal Genome Analysis Reveals De Novo Mutations, Single-Base Parental Inheritance, and Preferred DNA Ends. Proc Natl Acad Sci USA (2016) 113(50):E8159–68. doi: 10.1073/pnas.1615800113
52. Jiang P, Sun K, Tong YK, Cheng SH, Cheng THT, Heung MMS, et al. Preferred End Coordinates and Somatic Variants as Signatures of Circulating Tumor DNA Associated With Hepatocellular Carcinoma. Proc Natl Acad Sci USA (2018) 115(46):E10925–33. doi: 10.1073/pnas.1814616115
53. Serpas L, Chan RWY, Jiang P, Ni M, Sun K, Rashidfarrokhi A, et al. Dnase1l3 Deletion Causes Aberrations in Length and End-Motif Frequencies in Plasma DNA. Proc Natl Acad Sci U S A (2019) 116(2):641–9. doi: 10.1073/pnas.1815031116
54. Jiang P, Sun K, Peng W, Cheng SH, Ni M, Yeung PC, et al. Plasma DNA End-Motif Profiling as a Fragmentomic Marker in Cancer, Pregnancy, and Transplantation. Cancer Discovery (2020) 10(5):664–73. doi: 10.1158/2159-8290.CD-19-0622
55. Barefoot ME, Loyfer N, Kiliti AJ, McDeed AP, Kaplan T, Wellstein A. Detection of Cell Types Contributing to Cancer From Circulating, Cell-Free Methylated DNA. Front Genet (2021) 12. doi: 10.3389/fgene.2021.671057
56. Gai W, Ji L, Lam WKJ, Sun K, Jiang P, Chan AWH, et al. Liver- and Colon-Specific DNA Methylation Markers in Plasma for Investigation of Colorectal Cancers With or Without Liver Metastases. Clin Chem (2018) 64(8):1239–49. doi: 10.1373/clinchem.2018.290304
57. Luo H, Wei W, Ye Z, Zheng J, Xu Rh. Liquid Biopsy of Methylation Biomarkers in Cell-Free DNA. Trends Mol Med (2021) 27(5):482–500. doi: 10.1016/j.molmed.2020.12.011
58. FDA. Premarket Approval (PMA) . Available at: https://www.accessdata.fda.gov/scripts/cdrh/cfdocs/cfpma/pma.cfm?id=P130001 (Accessed June 10,2022).
59. Diehl F, Schmidt K, Choti MA, Romans K, Goodman S, Li M, et al. Circulating Mutant DNA to Assess Tumor Dynamics. Nat Med (2008) 14(9):985–90. doi: 10.1038/nm.1789
60. Leung F, Kulasingam V, Diamandis EP, Hoon DSB, Kinzler K, Pantel K, et al. Circulating Tumor DNA as a Cancer Biomarker: Fact or Fiction? Clin Chem (2016) 62(8):1054–60. doi: 10.1373/clinchem.2016.260331
61. Gauthier VJ, Tyler LN, Mannik M. Blood Clearance Kinetics and Liver Uptake of Mononucleosomes in Mice. J Immunol (1996) 156(3):1151–6.
62. Celec P, Vlková B, Lauková L, Bábíčková J, Boor P. Cell-Free DNA: The Role in Pathophysiology and as a Biomarker in Kidney Diseases. Expert Rev Mol Med (2018) 20:e1. doi: 10.1017/erm.2017.12
63. Mittra I, Nair NK, Mishra PK. Nucleic Acids in Circulation: Are They Harmful to the Host? J Biosci (2012) 37(2):301–12. doi: 10.1007/s12038-012-9192-8
64. Stroun M, Anker P, Maurice P, Lyautey J, Lederrey C, Beljanski M. Neoplastic Characteristics of the DNA Found in the Plasma of Cancer Patients. Oncology (1989) 46(5):318–22. doi: 10.1159/000226740
65. Pan W, Gu W, Nagpal S, Gephart MH, Quake SR. Brain Tumor Mutations Detected in Cerebral Spinal Fluid. Clin Chem (2015) 61(3):514–22. doi: 10.1373/clinchem.2014.235457
66. Jiang BY, Li YS, Guo WB, Zhang XC, Chen ZH, Su J, et al. Detection of Driver and Resistance Mutations in Leptomeningeal Metastases of NSCLC by Next-Generation Sequencing of Cerebrospinal Fluid Circulating Tumor Cells. Clin Cancer Res (2017) 23(18):5480–8. doi: 10.1158/1078-0432.CCR-17-0047
67. Huang Z, Gu B. Circulating Tumor DNA: A Resuscitative Gold Mine? Ann Transl Med (2015) 3(17):253. doi: 10.3978/j.issn.2305-5839.2015.09.11
68. Zill OA, Banks KC, Fairclough SR, Mortimer SA, Vowles JV, Mokhtari R, et al. The Landscape of Actionable Genomic Alterations in Cell-Free Circulating Tumor DNA From 21,807 Advanced Cancer Patients. Clin Cancer Res (2018) 24(15):3528–38. doi: 10.1158/1078-0432.CCR-17-3837
69. Sacher AG, Paweletz C, Dahlberg SE, Alden RS, O’Connell A, Feeney N, et al. Prospective Validation of Rapid Plasma Genotyping for the Detection of EGFR and Kras Mutations in Advanced Lung Cancer. JAMA Oncol (2016) 2(8):1014–22. doi: 10.1001/jamaoncol.2016.0173
70. Aggarwal C, Thompson JC, Black TA, Katz SI, Fan R, Yee SS, et al. Clinical Implications of Plasma-Based Genotyping With the Delivery of Personalized Therapy in Metastatic Non–Small Cell Lung Cancer. JAMA Oncol (2019) 5(2):173–80. doi: 10.1001/jamaoncol.2018.4305
71. Lam VK, Zhang J, Wu CC, Tran HT, Li L, Diao L, et al. Genotype-Specific Differences in Circulating Tumor DNA Levels in Advanced NSCLC. J Thorac Oncol (2021) 16(4):601–9. doi: 10.1016/j.jtho.2020.12.011
72. Elshimali YI, Khaddour H, Sarkissyan M, Wu Y, Vadgama JV. The Clinical Utilization of Circulating Cell Free DNA (CCFDNA) in Blood of Cancer Patients. Int J Mol Sci (2013) 14(9):18925–58. doi: 10.3390/ijms140918925
73. Komaki R, Cox JD, Stark R. Frequency of Brain Metastasis in Adenocarcinoma and Large Cell Carcinoma of the Lung: Correlation With Survival. Int J Radiat Oncol (1983) 9(10):1467–70. doi: 10.1016/0360-3016(83)90319-x
74. Srivastava G, Rana V, Wallace S, Taylor S, Debnam M, Feng L, et al. Risk of Intracranial Hemorrhage and Cerebrovascular Accidents in non-Small Cell Lung Cancer Brain Metastasis Patients. J Thorac Oncol (2009) 4(3):333–7. doi: 10.1097/JTO.0b013e318194fad4
75. Zhang B, Niu X, Zhang Q, Wang C, Liu B, Yue D, et al. Circulating Tumor DNA Detection Is Correlated to Histologic Types in Patients With Early-Stage non-Small-Cell Lung Cancer. Lung Cancer (2019) 134:108–16. doi: 10.1016/j.lungcan.2019.05.034
76. Livasy CA, Karaca G, Nanda R, Tretiakova MS, Olopade OI, Moore DT, et al. Phenotypic Evaluation of the Basal-Like Subtype of Invasive Breast Carcinoma. Mod Pathol (2006) 19(2):264–71. doi: 10.1038/modpathol.3800528
77. Keam B, Im SA, Kim HJ, Oh DY, Kim JH, Lee SH, et al. Prognostic Impact of Clinicopathologic Parameters in Stage II/III Breast Cancer Treated With Neoadjuvant Docetaxel and Doxorubicin Chemotherapy: Paradoxical Features of the Triple Negative Breast Cancer. BMC Cancer (2007) 7:203. doi: 10.1186/1471-2407-7-203
78. Nientiedt C, Budczies J, Endris V, Kirchner M, Schwab C, Jurcic C, et al. Mutations in TP53 or DNA Damage Repair Genes Define Poor Prognostic Subgroups in Primary Prostate Cancer. Urol Oncol (2022) J40(1):8.e11–8. doi: 10.1016/j.urolonc.2021.06.024
79. Parkinson CA, Gale D, Piskorz AM, Biggs H, Hodgkin C, Addley H, et al. Exploratory Analysis of TP53 Mutations in Circulating Tumour DNA as Biomarkers of Treatment Response for Patients With Relapsed High-Grade Serous Ovarian Carcinoma: A Retrospective Study. PLoS Med (2016) 13(12):e1002198. doi: 10.1371/journal.pmed.1002198
80. Chabon JJ, Hamilton EG, Kurtz DM, Esfahani MS, Moding EJ, Stehr H, et al. Integrating Genomic Features for non-Invasive Early Lung Cancer Detection. Nature (2020) 580(7802):245–51. doi: 10.1038/s41586-020-2140-0
81. Abbosh C, Birkbak NJ, Wilson GA, Jamal-Hanjani M, Constantin T, Salari R, et al. Phylogenetic ctDNA Analysis Depicts Early Stage Lung Cancer Evolution. Nature (2017) 545(7655):446–51. doi: 10.1038/nature22364
82. Provencio M, Serna-Blasco R, Franco F, Calvo V, Royuela A, Auglytė M, et al. Analysis of Circulating Tumour DNA to Identify Patients With Epidermal Growth Factor Receptor–Positive non-Small Cell Lung Cancer Who Might Benefit From Sequential Tyrosine Kinase Inhibitor Treatment. Eur J Cancer (2021) 149:61–72. doi: 10.1016/j.ejca.2021.02.031
83. Giroux Leprieur E, Herbretau G, Dumenil C, Julie C, Giraud V, Labrune S, et al. Circulating Tumor DNA Evaluated by Next-Generation Sequencing is Predictive of Tumor Response and Prolonged Clinical Benefit With Nivolumab in Advanced non-Small Cell Lung Cancer. Oncoimmunology (2018) 7(5):e1424675. doi: 10.1080/2162402X.2018.1424675
84. Lipson EJ, Velculescu VE, Pritchard TS, Sausen M, Pardoll DM, Topalian SL, et al. Circulating Tumor DNA Analysis as a Real-Time Method for Monitoring Tumor Burden in Melanoma Patients Undergoing Treatment With Immune Checkpoint Blockade. J Immunother Cancer (2014) 2(1):42. doi: 10.1186/s40425-014-0042-0
85. Provencio M, Majem M, Guirado M, Massuti B, de las Peñas R, Ortega AL, et al. Phase II Clinical Trial With Metronomic Oral Vinorelbine and Tri-Weekly Cisplatin as Induction Therapy, Subsequently Concomitant With Radiotherapy (RT) in Patients With Locally Advanced, Unresectable, Non-Small Cell Lung Cancer (NSCLC). Analysis of Survival and Value of ctDNA for Patient Selection. Lung Cancer (2021) 153:25–34. doi: 10.1016/j.lungcan.2021.01.005
86. Marsavela G, McEvoy AC, Pereira MR, Reid AL, Al-Ogaili Z, Warburton L, et al. Detection of Clinical Progression Through Plasma ctDNA in Metastatic Melanoma Patients: A Comparison to Radiological Progression. Br J Cancer (2022) 126(3):401–8. doi: 10.1038/s41416-021-01507-6
87. Reece M, Saluja H, Hollington P, Karapetis CS, Vatandoust S, Young GP, et al. The Use of Circulating Tumor DNA to Monitor and Predict Response to Treatment in Colorectal Cancer. Front Genet (2019) 10:1118. doi: 10.3389/fgene.2019.01118
88. Yi X, Ma J, Guan Y, Chen R, Yang L, Xia X. The Feasibility of Using Mutation Detection in ctDNA to Assess Tumor Dynamics. Int J Cancer (2017) 140(12):2642–7. doi: 10.1002/ijc.30620
89. Thomsen CB, Hansen TF, Andersen RF, Lindebjerg J, Jensen LH, Jakobsen A. Early Identification of Treatment Benefit by Methylated Circulating Tumor DNA in Metastatic Colorectal Cancer. Ther Adv Med Oncol (2020) 12:1758835920918472. doi: 10.1177/1758835920918472
90. Goldberg SB, Narayan A, Kole AJ, Decker RH, Teysir J, Carriero NJ, et al. Early Assessment of Lung Cancer Immunotherapy Response via Circulating Tumor DNA. Clin Cancer Res (2018) 24(8):1872–80. doi: 10.1158/1078-0432.CCR-17-1341
91. Lo YMD, Leung SF, Chan LYS, Chan ATC, Lo KW, Johnson PJ, et al. Kinetics of Plasma Epstein-Barr Virus DNA During Radiation Therapy for Nasopharyngeal Carcinoma. Cancer Res (2000) 60(9):2351–5.
92. Mair R, Mouliere F, Smith CG, Chandrananda D, Gale D, Marass F, et al. Measurement of Plasma Cell-Free Mitochondrial Tumor DNA Improves Detection of Glioblastoma in Patient-Derived Orthotopic Xenograft Models. Cancer Res (2019) 79(1):220–30. doi: 10.1158/0008-5472.CAN-18-0074
93. Rostami A, Lambie M, Yu CW, Stambolic V, Waldron JN, Bratman SV. Senescence, Necrosis, and Apoptosis Govern Circulating Cell-Free DNA Release Kinetics. Cell Rep (2020) 31(13):107830. doi: 10.1016/j.celrep.2020.107830
94. Provencio M, Serna-Blasco R, Nadal E, Insa A, García-Campelo MR, Casal Rubio J, et al. Overall Survival and Biomarker Analysis of Neoadjuvant Nivolumab Plus Chemotherapy in Operable Stage IIIA Non-Small-Cell Lung Cancer (NADIM Phase II Trial). J Clin Oncol (2022) JCO2102660. doi: 10.1200/JCO.21.02660
95. Forde PM, Spicer J, Lu S, Provencio M, Mitsudomi T, Awad MM, et al. Neoadjuvant Nivolumab Plus Chemotherapy in Resectable Lung Cancer. N Engl J Med (2022) 386(21):1973–85. doi: 10.1056/NEJMoa2202170
96. Szpechcinski A, Chorostowska-Wynimko J, Kupis W, Maszkowska-Kopij K, Dancewicz M, Kowalewski J, et al. Quantitative Analysis of Free-Circulating DNA in Plasma of Patients With Resectable NSCLC. Expert Opin Biol Ther (2012) 12 Suppl 1:S3–9. doi: 10.1517/14712598.2012.668519
97. Parikh AR, van Seventer EE, Siravegna G, Hartwig AV, Jaimovich A, He Y, et al. Minimal Residual Disease Detection Using a Plasma-Only Circulating Tumor DNA Assay in Patients With Colorectal Cancer. Clin Cancer Res (2021) 27(20):5586–94. doi: 10.1158/1078-0432.CCR-21-0410
98. Chaudhuri AA, Chabon JJ, Lovejoy AF, Newman AM, Stehr H, Azad TD, et al. Early Detection of Molecular Residual Disease in Localized Lung Cancer by Circulating Tumor DNA Profiling. Cancer Discovery (2017) 7(12):1394–403. doi: 10.1158/2159-8290.CD-17-0716
99. Phallen J, Sausen M, Adleff V, Leal A, Hruban C, White J, et al. Direct Detection of Early-Stage Cancers Using Circulating Tumor DNA. Sci Transl Med (2017) 9(403):eaan2415. doi: 10.1126/scitranslmed.aan2415
100. Jamal-Hanjani M, Wilson GA, McGranahan N, Birkbak NJ, Watkins TBK, Veeriah S, et al. Tracking the Evolution of Non–Small-Cell Lung Cancer. N Engl J Med (2017) 376(22):2109–21. doi: 10.1056/NEJMoa1616288
101. Cohen JD, Li L, Wang Y, Thoburn C, Afsari B, Danilova L, et al. Detection and Localization of Surgically Resectable Cancers With a Multi-Analyte Blood Test. Science (2018) 359(6378):926–30. doi: 10.1126/science.aar3247
102. Liu MC, Oxnard GR, Klein EA, Swanton C, Seiden MV, Liu MC, et al. Sensitive and Specific Multi-Cancer Detection and Localization Using Methylation Signatures in Cell-Free DNA. Ann Oncol (2020) 31(6):745–59. doi: 10.1016/j.annonc.2020.02.011
103. Chan KCA, Yeung SW, Lui WB, Rainer TH, L̀o YMD. Effects of Preanalytical Factors on the Molecular Size of Cell-Free DNA in Blood. Clin Chem (2005) 51(4):781–4. doi: 10.1373/clinchem.2004.046219
104. Rolfo C, Mack PC, Scagliotti GV, Baas P, Barlesi F, Bivona TG, et al. Liquid Biopsy for Advanced Non-Small Cell Lung Cancer (NSCLC): A Statement Paper From the IASLC. J Thorac Oncol (2018) 13(9):1248–68. doi: 10.1016/j.jtho.2018.05.030
105. Soo J, Kurtz DM, Jin M, Scherer F, Schroers-Martin JG, Esfahani MS, et al. Comparison of Circulating Tumor DNA Recovery From Plasma and Serum. Blood. (2017) 130:2756. doi: 10.1182/blood.V130.Suppl_1.2756.2756
106. Bronkhorst AJ, Aucamp J, Pretorius PJ. Cell-Free DNA: Preanalytical Variables. Clin Chim Acta (2015) 450:243–53. doi: 10.1016/j.cca.2015.08.028
107. Merker JD, Oxnard GR, Compton C, Diehn M, Hurley P, Lazar AJ, et al. Circulating Tumor DNA Analysis in Patients With Cancer: American Society of Clinical Oncology and College of American Pathologists Joint Review. J Clin Oncol (2018) 36(16):1631–41. doi: 10.1200/JCO.2017.76.8671
108. Fernando MR, Chen K, Norton S, Krzyzanowski G, Bourne D, Hunsley B, et al. A New Methodology to Preserve the Original Proportion and Integrity of Cell-Free Fetal DNA in Maternal Plasma During Sample Processing and Storage. Prenat Diagn (2010) 30(5):418–24. doi: 10.1002/pd.2484
109. El Messaoudi S, Rolet F, Mouliere F, Thierry AR. Circulating Cell Free DNA: Preanalytical Considerations. Clin Chim Acta (2013) 424:222–30. doi: 10.1016/j.cca.2013.05.022
110. Herrera LJ, Raja S, Gooding WE, El-Hefnawy T, Kelly L, Luketich JD, et al. Quantitative Analysis of Circulating Plasma DNA as a Tumor Marker in Thoracic Malignancies. Clin Chem (2005) 51(1):113–8. doi: 10.1373/clinchem.2004.039263
111. Lu J-L, Liang Z-Y. Circulating Free DNA in the Era of Precision Oncology: Pre- and Post-Analytical Concerns. Chronic Dis Transl Med (2016) 2(4):223–30. doi: 10.1016/j.cdtm.2016.12.001
112. Pérez-Barrios C, Nieto-Alcolado I, Torrente M, Jiménez-Sánchez C, Calvo V, Gutierrez-Sanz L, et al. Comparison of Methods for Circulating Cell-Free DNA Isolation Using Blood From Cancer Patients: Impact on Biomarker Testing. Transl Lung Cancer Res (2016) 5(6):665–72. doi: 10.21037/tlcr.2016.12.03
113. Markus H, Contente-Cuomo T, Farooq M, Liang WS, Borad MJ, Sivakumar S, et al. Evaluation of Pre-Analytical Factors Affecting Plasma DNA Analysis. Sci Rep (2018) 8(1):7375. doi: 10.1038/s41598-018-25810-0
114. Rolfo C, Mack P, Scagliotti GV, Aggarwal C, Arcila ME, Barlesi F, et al. Liquid Biopsy for Advanced NSCLC: A Consensus Statement From the International Association for the Study of Lung Cancer. J Thorac Oncol (2021) 16(10):1647–62. doi: 10.1016/j.jtho.2021.06.017
115. Romero A, Jantus-Lewintre E, García-Peláez B, Royuela A, Insa A, Cruz P, et al. Comprehensive Cross-Platform Comparison of Methods for non-Invasive EGFR Mutation Testing: Results of the RING Observational Trial. Mol Oncol (2021) 15(1):43–56. doi: 10.1002/1878-0261.12832
116. Raymond VM, Higashi L, Marino E, Lang K. Evaluation of the ctDNA LUNAR-2 Test In an Average Patient Screening Episode (ECLIPSE). JCO (2021) 39:TPS142–2. doi: 10.1200/JCO.2021.39.3_suppl.TPS142
117. Rolfo C, Cardona AF, Cristofanilli M, Paz-Ares L, Diaz Mochon JJ, Duran I, et al. Challenges and Opportunities of cfDNA Analysis Implementation in Clinical Practice: Perspective of the International Society of Liquid Biopsy (ISLB). Crit Rev Oncol Hematol (2020) 151:102978. doi: 10.1016/j.critrevonc.2020.102978
118. Zhang Y, Xu Y, Zhong W, Zhao J, Chen M, Zhang L, et al. Total DNA Input is a Crucial Determinant of the Sensitivity of Plasma Cell-Free DNA EGFR Mutation Detection Using Droplet Digital PCR. Oncotarget (2017) 8(4):5861–73. doi: 10.18632/oncotarget.14390
119. O’Leary B, Hrebien S, Beaney M, Fribbens C, Garcia-Murillas I, Jiang J, et al. Comparison of BEAMing and Droplet Digital PCR for Circulating Tumor DNA Analysis. Clin Chem (2019) 65(11):1405–13. doi: 10.1373/clinchem.2019.305805
120. Genovese G, Kähler AK, Handsaker RE, Lindberg J, Rose SA, Bakhoum SF, et al. Clonal Hematopoiesis and Blood-Cancer Risk Inferred From Blood DNA Sequence. N Engl J Med (2014) 371(26):2477–87. doi: 10.1056/NEJMoa1409405
Keywords: ctDNA= circulating tumor DNA, ctDNA kinetics, biomarker, liquid biopsy, monitoring
Citation: Sánchez-Herrero E, Serna-Blasco R, Robado de Lope L, González-Rumayor V, Romero A and Provencio M (2022) Circulating Tumor DNA as a Cancer Biomarker: An Overview of Biological Features and Factors That may Impact on ctDNA Analysis. Front. Oncol. 12:943253. doi: 10.3389/fonc.2022.943253
Received: 13 May 2022; Accepted: 16 June 2022;
Published: 20 July 2022.
Edited by:
Lixuan Wei, Mayo Clinic, United StatesReviewed by:
Valeria Pereira Ferrer, Federal Fluminense University, BrazilCopyright © 2022 Sánchez-Herrero, Serna-Blasco, Robado de Lope, González-Rumayor, Romero and Provencio. This is an open-access article distributed under the terms of the Creative Commons Attribution License (CC BY). The use, distribution or reproduction in other forums is permitted, provided the original author(s) and the copyright owner(s) are credited and that the original publication in this journal is cited, in accordance with accepted academic practice. No use, distribution or reproduction is permitted which does not comply with these terms.
*Correspondence: Atocha Romero, YXRvY2hhMTBAaG90bWFpbC5jb20=; orcid.org/0000-0002-1634-7397
†These authors have contributed equally to this work
Disclaimer: All claims expressed in this article are solely those of the authors and do not necessarily represent those of their affiliated organizations, or those of the publisher, the editors and the reviewers. Any product that may be evaluated in this article or claim that may be made by its manufacturer is not guaranteed or endorsed by the publisher.
Research integrity at Frontiers
Learn more about the work of our research integrity team to safeguard the quality of each article we publish.