- 1Department of Pathology, Leiden University Medical Center (LUMC), Leiden, Netherlands
- 2Department of Pathology and Molecular Pathology, University Hospital and University of Zürich, Zürich, Switzerland
- 3Department of Radiotherapy, Leiden University Medical Center, Leiden, Netherlands
Endometrial cancer (EC) diagnostics is evolving into a system in which molecular aspects are increasingly important. The traditional histological subtype-driven classification has shifted to a molecular-based classification that stratifies EC into DNA polymerase epsilon mutated (POLEmut), mismatch repair deficient (MMRd), and p53 abnormal (p53abn), and the remaining EC as no specific molecular profile (NSMP). The molecular EC classification has been implemented in the World Health Organization 2020 classification and the 2021 European treatment guidelines, as it serves as a better basis for patient management. As a result, the integration of the molecular class with histopathological variables has become a critical focus of recent EC research. Pathologists have observed and described several morphological characteristics in association with specific genomic alterations, but these appear insufficient to accurately classify patients according to molecular subgroups. This requires pathologists to rely on molecular ancillary tests in routine workup. In this new era, it has become increasingly challenging to assign clinically relevant weights to histological and molecular features on an individual patient basis. Deep learning (DL) technology opens new options for the integrative analysis of multi-modal image and molecular datasets with clinical outcomes. Proof-of-concept studies in other cancers showed promising accuracy in predicting molecular alterations from H&E-stained tumor slide images. This suggests that some morphological characteristics that are associated with molecular alterations could be identified in EC, too, expanding the current understanding of the molecular-driven EC classification. Here in this review, we report the morphological characteristics of the molecular EC classification currently identified in the literature. Given the new challenges in EC diagnostics, this review discusses, therefore, the potential supportive role that DL could have, by providing an outlook on all relevant studies using DL on histopathology images in various cancer types with a focus on EC. Finally, we touch upon how DL might shape the management of future EC patients.
Introduction
The incorporation of the molecular endometrial cancer (EC) classification in the fifth edition of the World Health Organization (WHO) classification of female genital tumors and the 2021 European treatment guidelines (1, 2) has marked a new era in EC diagnostics. This moved the field farther away from the classic dualistic model proposed by Bockman in 1983 (3), who divided endometrial carcinomas into type I and type II cancers. Type I EC is endometrioid and estrogen driven and can be graded using the International Federation of Gynaecology and Obstetrics (FIGO) grading system (4). Type II EC includes a variety of non-endometrioid histological subtypes, such as uterine serous carcinoma, clear cell carcinoma, mixed carcinomas, un-/dedifferentiated carcinomas, and uterine carcinosarcomas. The new molecular EC classification that is now recommended by the WHO (1, 2) completely changes the diagnostic perspective by placing histological subtype secondary to molecular class. It utilizes surrogate markers paralleling the four molecular classes originally described by The Cancer Genome Atlas (TCGA) (5). First, targeted sequencing (Sanger or panel next-generation sequencing, NGS) of exons 9–14 of DNA polymerase epsilon (POLE) can identify POLE-mutated (POLEmut) EC. Second, mismatch repair-deficient (MMRd) EC is defined by loss of expression of one of the mismatch repair proteins (MLH1, PMS2, MSH6, and MSH2) by immunohistochemistry (IHC). Third, p53 IHC is performed to identify EC with abnormal p53 expression patterns (p53abn) (6, 7). Finally, EC without a pathogenic POLE variant, with retained MMR protein expression, and wild-type p53 IHC, is classified as “no specific molecular profile” (NSMP) EC. Studies on EC with more than one molecular alteration, commonly referred to as “multiple-classifiers,” have served to identify the order by which these tests should be performed (8). It has resulted in the EC diagnostic algorithm endorsed by the WHO 2020 classification that first assesses POLE status regardless of other markers, then MMR, and finally p53 (9) (Figure 1).
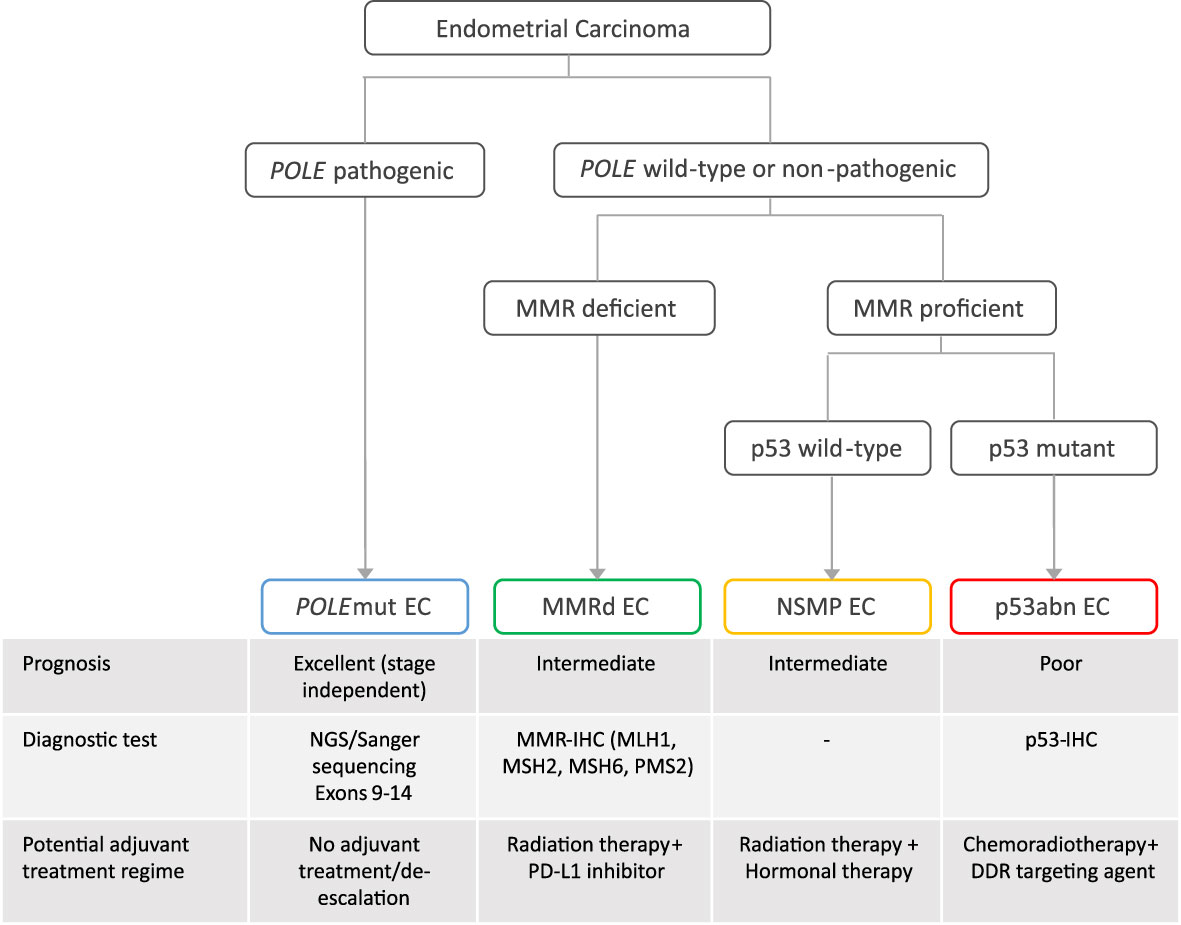
Figure 1 The diagnostic algorithm of the molecular classification of endometrial cancer, associated prognosis, diagnostic test, and potential adjuvant treatment regime. EC, endometrial cancer; NGS, panel next-generation sequencing; POLEmut, polymerase epsilon mutated; MMRd, mismatch repair deficient; NSMP, no specific molecular profile; p53abn, p53 abnormal; DDR, DNA damage response; PD-L1, programmed death ligand.
The molecular classification resolves one of the main issues of the histology-driven EC classification, which is the high level of interobserver variability (10). Particularly high-grade and non-endometrioid histological subtypes are only moderately reproducible (11), which provides a poor basis for patient management (12). The recent paradigm shift in EC diagnostics follows preceding developments in surgical pathology, in which a series of technological breakthroughs such as immunohistochemistry and molecular testing have continually improved diagnostic accuracy (13).
The molecular EC classification has also shown a prognostic value across cohorts of different risk populations and is predictive of response to treatment, specifically in p53abn EC, which has a poor outcome and may benefit from addition of adjuvant chemotherapy (14), and in POLEmut EC, which has an excellent outcome regardless of adjuvant treatment, whereas MMRd and NSMP EC have intermediate prognoses (5, 14–20). This has been the rationale for adapting the clinical risk stratification system of EC patients (21) wherein it is encouraged to apply the molecular classification in the management of EC, especially high-risk EC (1, 2); ongoing and new trials such as PORTEC-4a (22) and RAINBO (23) exploit the molecular classification as a basis for targeted adjuvant therapy (Figure 1) (24). Consequently, the gynecological oncology community has started to utilize the molecular classification; however, the current risk stratification system does not clearly indicate which of the histological or molecular variables are most clinically relevant, or leverage the combination of both.
New technological breakthroughs in pathology are now driving progress in cancer diagnostics. Since the emergence of convolutional neural networks in 2012 (25), deep learning (DL) models have continuously demonstrated their high accuracy for the classification of medical (26) and non-medical image datasets (27). This was followed by the start of a digital revolution in pathology, wherein state-of-the-art DL models from the computer vision community were used on digital histopathology slides. Hematoxylin and eosin (H&E) staining procedure is the most common in cancer diagnostics, and large, well-characterized retrospective cohorts and clinical trial sets are available, enabling the collection of large-scale histopathology imaging datasets to train state-of-the-art DL models. A number of proof-of-concept papers showed the potential of DL models to aid the diagnosis and molecular classification of cancers (28–55) or predicting patient prognosis (56–60), by recognizing phenotypes on H&E-stained tumor slide images. Image-based DL models have been frequently trained onto colorectal cancer (29–37) and breast cancer (35, 38–44). However, in EC, only two studies (53, 54) so far have been published using DL for predicting one to various EC molecular alterations or gene mutations from publicly available datasets. They have obtained promising performance; however, the size of the dataset and application to a few non-endometrioid histological EC subtypes limit the generalizability of the findings. Furthermore, in these studies, DL models have not been trained to predict the newly established four-class molecular classification in EC diagnostics. Opportunities for future image-based DL models to impact EC diagnostics and thus guide clinical management decisions include the following: improve EC diagnostic classification by serving as a pre-screening tool to prioritize molecular testing, refine EC risk stratification by identifying morphological features with prognostic relevance, and predict patient outcome or even response to treatment.
In the light of the redefinition of EC on the basis of molecular features, we here provide a concise summary of the histopathological features associated with the four molecular classes (Table 1). These morpho-molecular correlates may serve to explore the feasibility of histology-directed molecular testing, particularly in low-income countries, and deepen our understanding of the underlying biological processes. We also present possible avenues by which image-based DL may be able to support these objectives, by discussing the landmark studies that have used DL onto histopathology slide images in EC and other cancers, which illustrates how these novel DL applications may impact the field of EC diagnostics in the future (Figure 2).
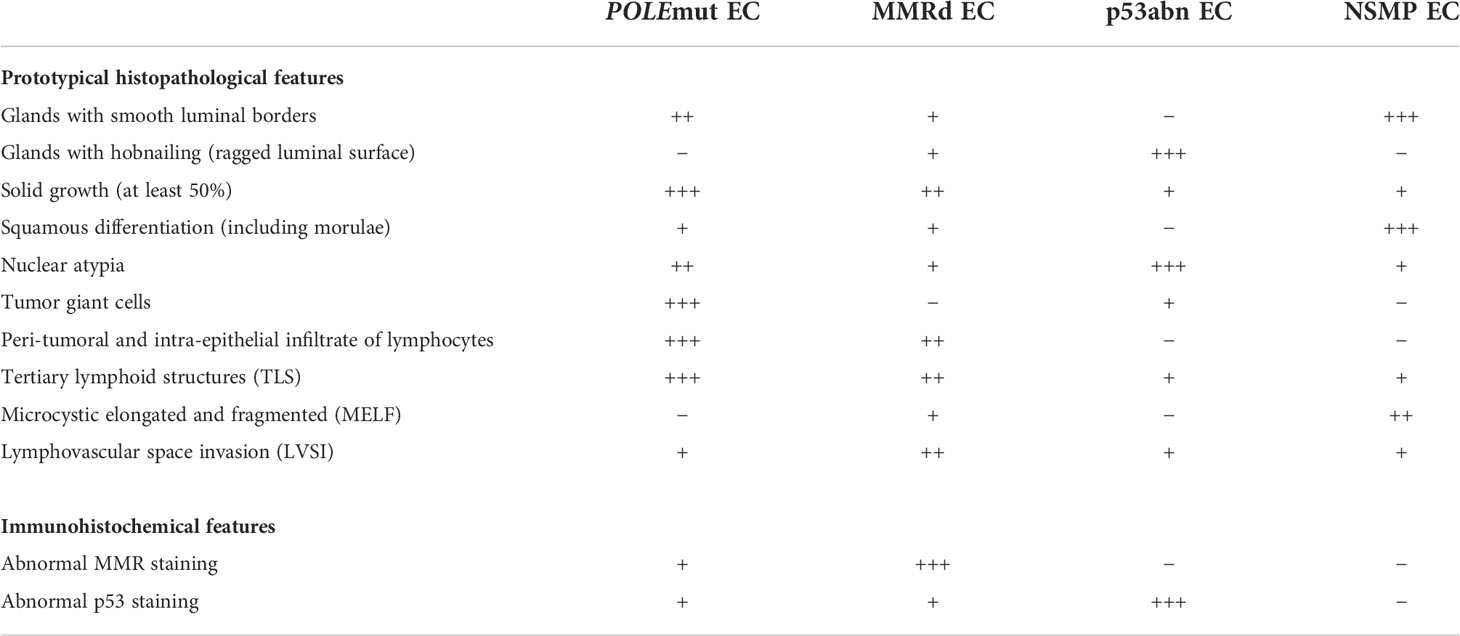
Table 1 Summary of the histopathological and immunohistochemical features correlated with the molecular endometrial cancer (EC) classification, dividing EC into POLE-mutated (POLEmut) EC, mismatch repair deficient (MMRd) EC, p53 abnormal (p53abn) EC, and non-specific molecular profile (NSMP) EC.
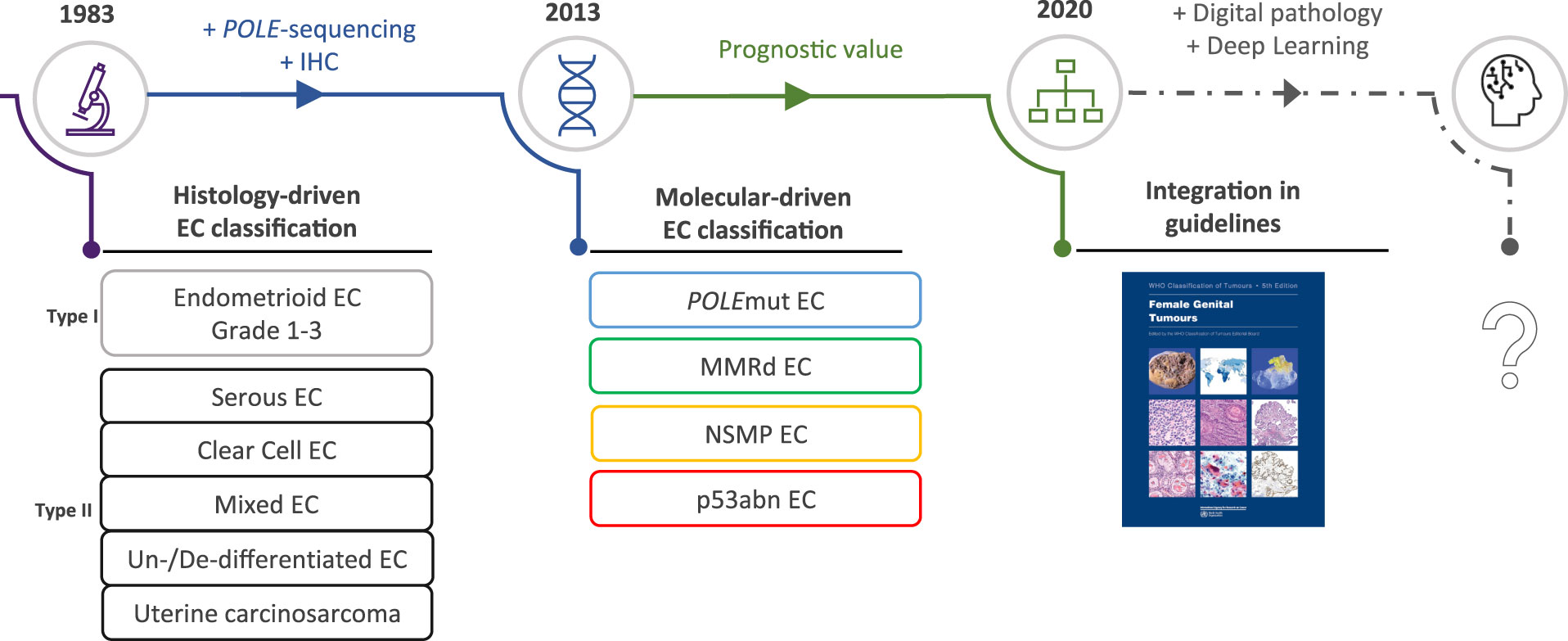
Figure 2 The evolving role of morphology in endometrial cancer diagnostics. EC, endometrial cancer; POLEmut, polymerase epsilon mutated; MMRd, mismatch repair deficient; NSMP, no specific molecular profile; p53abn, p53 abnormal.
Morpho-molecular correlates of the current EC classification
POLE-mutated endometrial cancers
Pathogenic mutations in the exonuclease domain of DNA polymerase epsilon (POLE) in EC, POLEmut EC, were first described by Church et al. (61) and quickly thereafter by the TCGA (5). Mutations in POLE result in a defective proofreading domain during DNA leading-strand replication, yielding a very high mutation burden and increased neoantigen load. In these original publications, only a limited number of non-endometrioid EC were tested, and these did not carry POLE mutations. This led to the assumption that POLE mutations could only occur in endometrioid-type EC. However, subsequent larger studies challenged this idea by showing that POLE mutations can be identified in non-endometrioid carcinomas, including uterine carcinosarcomas, serous carcinomas, clear cell carcinomas, and un-/dedifferentiated carcinomas, albeit in low frequencies (14, 62–65). A search for a POLEmut EC-specific phenotypic trait resulted in the identification of specific morphological features (Figure 3): first, approximately two-thirds of POLEmut EC show at least 50% solid growth (also referred to as FIGO grade 3) (66, 67), and the glandular component, if present, usually consists of glands with smooth luminal borders without hobnailing (66); second, hyperchromatic (multi-nucleated) tumor giant cells scattered throughout the solid sheets of tumor cells have been described as a recurring feature (66, 68); third, a dense peri-tumoral and intra-epithelial infiltrate of lymphocytes is frequently noted, likely the phenotypic response of its high mutational load (66, 67, 69–71); finally, a more recent addition to these features is the presence of (often numerous) tertiary lymphoid structures (TLS) within the myometrial wall of POLEmut EC (72–74).
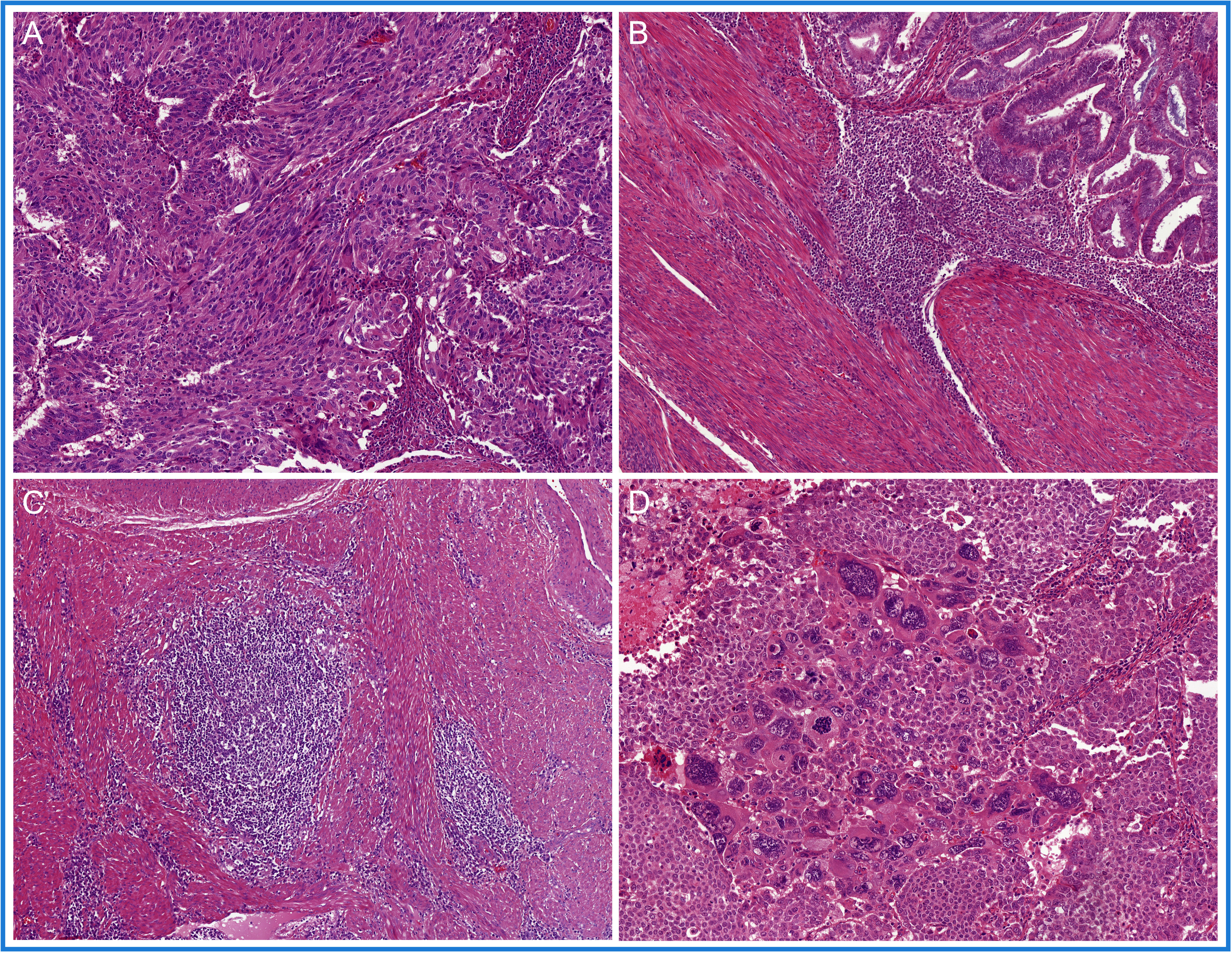
Figure 3 A selection of prototypical morphological features found in POLE-mutated endometrial cancer (POLEmut EC): (A) at least 50% solid growth; (B) hyperchromatic tumor giant cells; (C) a dense peri-tumoral and intra-epithelial infiltrate of lymphocytes; and (D) tertiary lymphoid structures (TLS).
To date, there is no single immunohistochemical stain that can serve to identify POLEmut EC, and only few studies have studied specific markers (75). Among the IHC stains commonly used in diagnostics, abnormal staining for MMR proteins is present in about 20% of POLEmut EC (72). Mutant-type abnormal p53 staining can be identified in 12%–30% of POLEmut EC (5, 7, 8), but no specific morphological substrate has been detected in this subset of cases (8). Among the POLEmut EC with secondary p53 abnormality, subclonal/regional mutant-type overexpression of p53 is a relatively common finding (7). Sequencing of the exonuclease domain of POLE thus remains required to accurately identify POLEmut EC, since morphology and adjunct studies alone are insufficient.
Mismatch repair-deficient endometrial cancers
Damage in the DNA mismatch repair (MMR) pathway leaves unrepaired post-DNA replication errors. Thus, the phenotype of MMR-deficient EC (MMRd EC) much like POLEmut EC is most likely shaped by the biological consequences of a high mutational load, leading to a similar morphological representation (Figure 4). In fact, several studies have described the abundance of stromal and intra-epithelial lymphocytes and, more recently, the presence of TLS in MMRd EC (69, 70, 72, 73, 76), yet in a somewhat lower abundance than in POLEmut EC (69, 72). Furthermore, endometrioid-type EC is the dominant histological subtype of MMRd EC (5, 15, 17–19, 77, 78). Endometrioid EC with solid growth (FIGO grade 3) is relatively more frequent in MMRd EC than in NSMP EC but less frequent than in POLEmut EC (5, 14, 15, 18). However, a variety of other non-endometrioid histological subtypes have also been reported within the MMRd EC subclass. For example, one recent study identified MMR deficiency in uterine carcinosarcomas and interestingly noted that their epithelial components often had an endometrioid morphology (79). A small proportion of MMRd EC has also been observed with a serous or clear cell morphology (14, 18). This serous-like phenotype was found not to be associated with acquired TP53 mutations in these MMRd tumors (8). Instead, there is some indication that MMRd EC with serous-like morphology is more often seen in MMRd EC with underlying germline mutations (Lynch syndrome associated); however this, observation needs to be validated (62, 80, 81). Interestingly, about two-thirds of the un-/dedifferentiated EC have been shown to be MMR deficient (82). Finally, for yet unknown reasons, multiple reports described an association between the presence of lymphovascular space invasion (LVSI) and MMR deficiency in EC (15, 77). Hence, morphology alone is insufficient to accurately detect and classify MMRd EC.
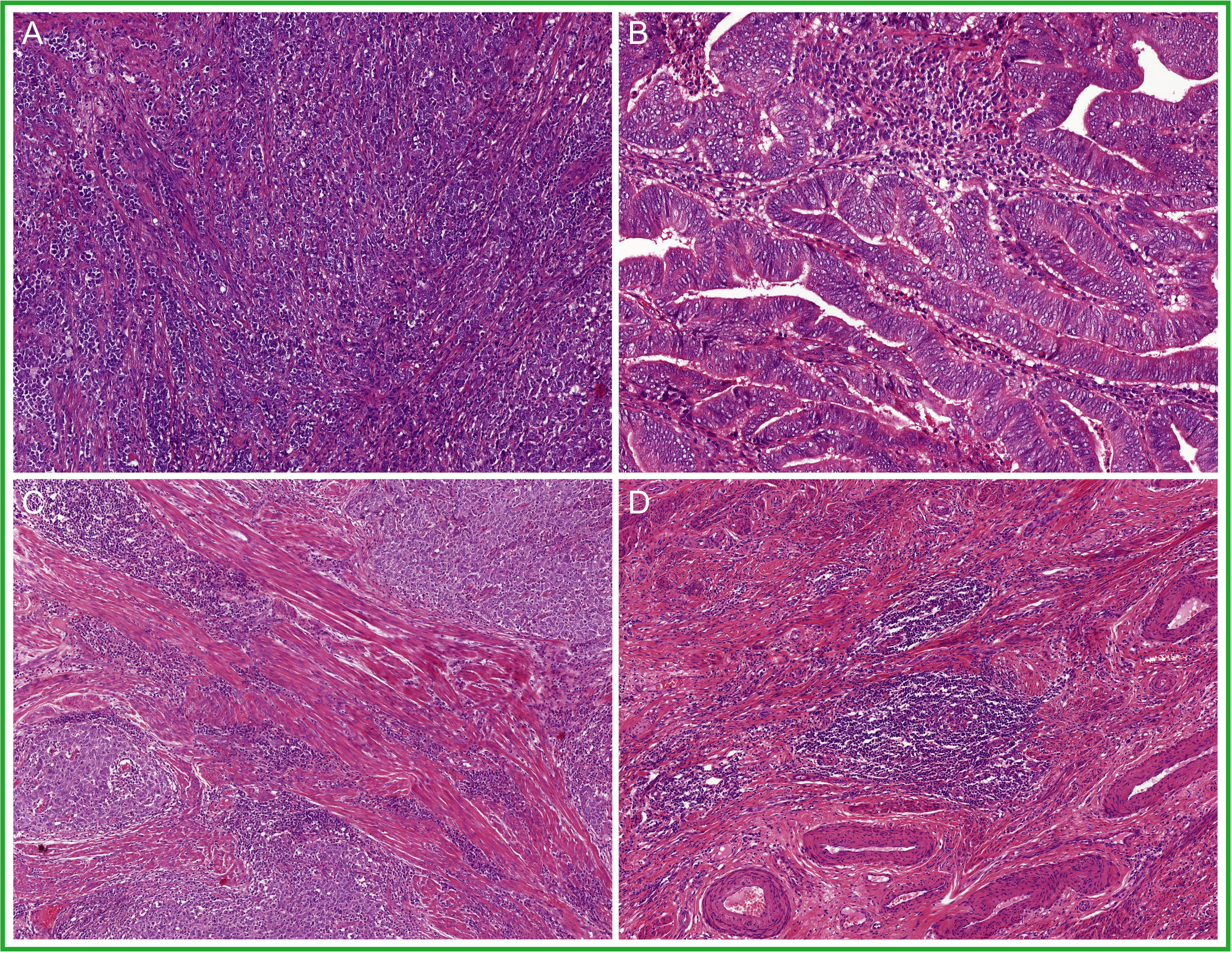
Figure 4 A selection of prototypical morphological features found in mismatch repair deficient endometrial cancer (MMRd EC): (A) solid growth; (B) glandular architecture; (C) a dense to moderate peri-tumoral and intra-epithelial infiltrate of lymphocytes; and (D) tertiary lymphoid structures (TLS).
After excluding pathogenic POLE mutations, immunohistochemical staining of the four MMR proteins is therefore used to identify MMRd EC. Approximately one quarter of all EC show loss of expression of one of the MMR proteins. The most common combination (about 70%) in MMRd EC is loss of MLH1 and PMS2 expression, which is usually caused by promotor hypermethylation of the MLH1 gene. The remaining cases show loss of protein expression in other combinations, namely, single loss of MSH6, single loss of PMS2, or MSH2/MSH6 loss, of which about 10% is Lynch syndrome associated (80). p53 abnormal staining can be seen in 10% of MMRd EC (72), of which approximatively three quarters show p53 subclonal mutant-type overexpression (7).
p53 abnormal endometrial cancers
The prototypical p53 abnormal endometrial cancers (p53abn EC) has a classic serous histology with a (micro-) papillary or (pseudo-) glandular architecture. The papillae or glands are lined by a single layer of tumor cells with strong cytonuclear atypia resulting in a ragged luminal surface (Figure 5). Furthermore, a brisk mitotic activity is consistently found (14, 18, 78, 83–85). The p53abn EC molecular subgroup, however, has a broader histological spectrum, as it also includes uterine carcinosarcomas (78), clear cell carcinomas (14, 18), and FIGO grade 3 endometrioid carcinomas (5, 14, 18, 84, 85). Intriguingly, some studies described that p53abn EC can also present with low-grade endometrioid morphology (15, 70, 84). Whether this observation is true, or that these cases rather represent misclassified pseudo-glandular serous endometrial carcinomas, remains to be determined. The low abundance of tumor-infiltrating lymphocytes and lack of TLS are other histological features that differentiate p53abn EC from MMRd EC and POLEmut EC (69, 70, 72).
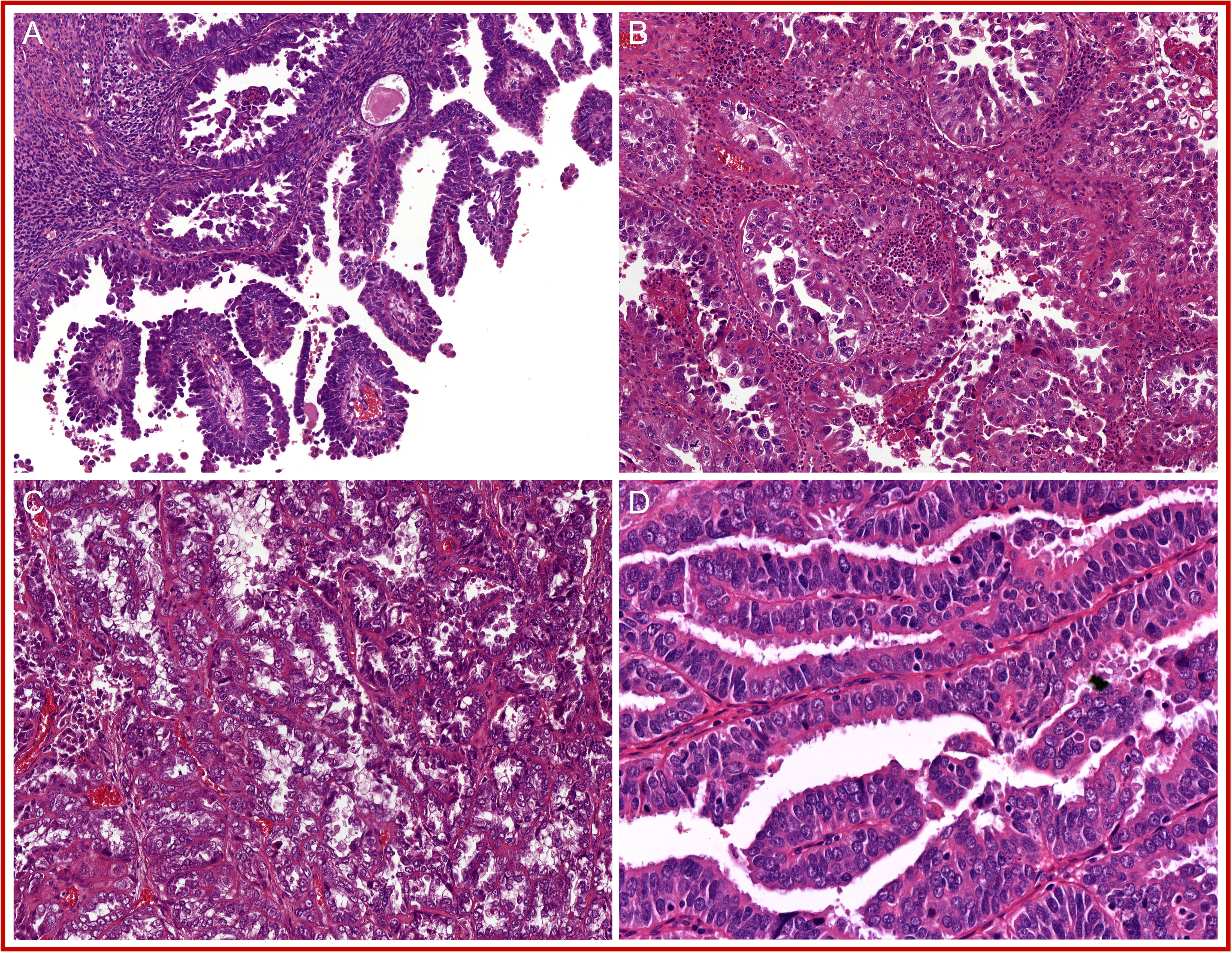
Figure 5 A selection of prototypical morphological features found in p53 abnormal endometrial cancer (p53abn EC): (A) (micro-)papillary glandular architecture; (B) glands with ragged luminal surface; (C) brisk mitotic activity; and (D) strong nuclear atypia.
p53abn ECs, per definition, are MMR proficient and POLE wild type and displays one of the well-described mutant-like immunohistochemical p53 staining patterns (9). This includes abnormal p53 nuclear overexpression in 65%, abnormal null-mutant pattern in 13%, or cytoplasmic p53 overexpression (6, 7). In addition, strong and diffuse positive membranous Her2Neu staining (3+), found in 20%–25% of p53abn EC, may be p53abn subclass specific (7, 86).
No specific molecular profile endometrial cancers
The group of EC that does not carry a pathogenic POLE mutation is MMR proficient and shows wild-type expression of p53 is currently referred to as “no specific molecular profile” (NSMP) EC. The majority shows a predominant glandular proliferation in which the glands have smooth luminal borders and the nuclei have mild to moderate atypia (FIGO grades 1 and 2) (5, 15, 17, 18, 78). A subset of these low-grade NSMP EC present (morular) squamous differentiation to a varying degree, a distinct morphological feature that has been linked to underlying CTNNB1 mutations (87–90). In addition to these prototypical features (Figure 6), approximately 20% of the low-grade NSMP EC present with a specific type of invasion, referred to as “microcystic elongated and fragmented” (MELF) type of invasion (91–93), which is rarely seen outside NSMP EC. A lower abundance of TILS and TLS in the NSMP EC group than in MMRd EC and POLEmut EC has also been reported (69, 70, 72). Finally, non-endometrioid or high-grade NSMP ECs are uncommon but have been described (14, 17–19, 78).
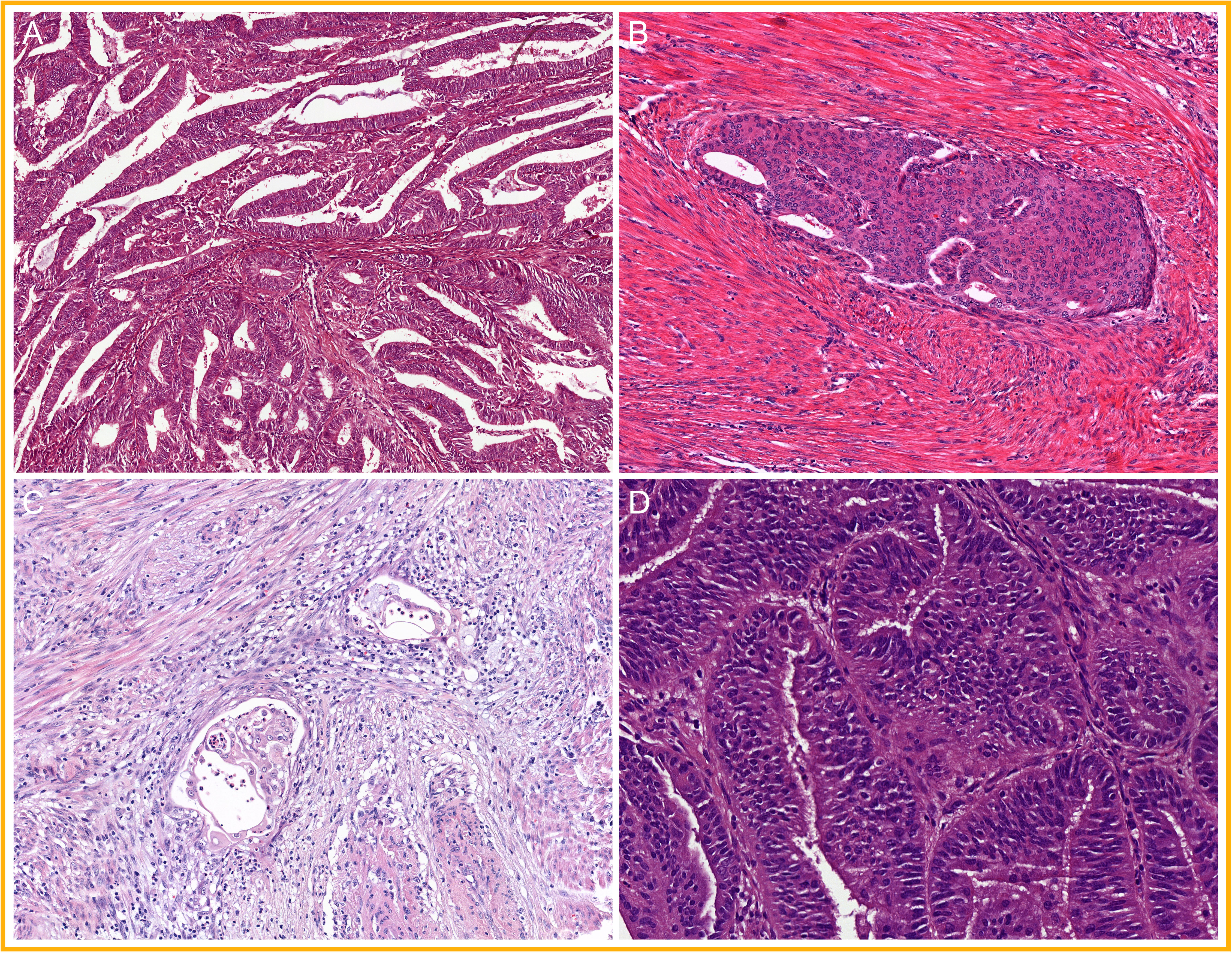
Figure 6 A selection of prototypical morphological features found in non-specific molecular profile endometrial cancer (NSMP EC): (A) glands with smooth luminal borders; (B) squamous differentiation; (C) microcystic elongated and fragmented (MELF) type of invasion; and (D) mild nuclear atypia.
Emerging data suggest that NSMP EC may be further stratified into two groups with a distinct prognosis based on hormone receptor expression status (94). Although the majority of NSMP EC shows high expression of estrogen receptors (ER alpha) and progesterone receptors (PR A/B), a notable subset of approximately 10% of the NSMP EC show complete lack of ER and PR expression. Interestingly, this subgroup is enriched with non-endometrioid morphology, particularly clear cell morphology. It is also conceivable that the recently described mesonephric-like endometrial carcinomas and the gastric-type endometrial carcinomas fall in this group of hormone-receptor-negative NSMP EC (78).
The current role of morphology within the molecular EC classification
All these outlined human-identified morpho-molecular correlates are presently insufficient to accurately predict molecular class on H&E features only, and no exclusive phenotypic trait has been identified for any of the molecular classes. The observed histopathological heterogeneity within defined molecular classes clearly challenges the role of morphology in the context of the molecular EC classification. Morphological information may still refine prognosis within a specific molecular context such as histological subtype and grade in NSMP EC (95) or dense immune infiltrate or presence of TLS in MMRd EC (69, 70). Yet, at the same time, some morphological features may arguably no longer carry prognostic value in some molecular subgroups. For instance, evidence showed that a broad range of histological subtypes and grades can be found in POLEmut EC and p53abn EC while carrying distinct genomic alterations and having excellent and poor prognosis, respectively (5, 14, 15, 17–20). Likewise, the prognostic relevance of other morphological features is under investigation such as the presence of LVSI within POLEmut EC and MMRd EC (15, 66, 77) and the lymphocyte density in NSMP EC and p53abn EC (69, 70, 72).
Implementation of the molecular EC classification is a step forward, but it is questionable whether histological features have become completely obsolete. Morphological features may still contain pertinent information beyond the molecular classification such as additional prognostic indicators. It is, however, becoming increasingly complex for pathologists to distinguish relevant morphological subtleties in EC diagnostics. In this context, DL models may be capable of learning relevant morphological features in association with molecular alterations on digitized H&E-stained EC tumor slides. DL-based research may show that further refining of the EC classification is possible by accurately combining histological and molecular data (Figure 2).
Deep learning can recognize phenotypes of mutations on H&E tumor slides
Landmark studies have recently provided the proof of principle for the prediction of genetic mutations from H&E whole slide images by DL in several types of cancer (28–52, 54, 55), albeit more frequently in colorectal cancer (29–37) and breast cancer (35, 38–44). For example, the feasibility of predicting TP53 mutation status has been explored across breast, colorectal, lung, stomach, pan-gastrointestinal, bladder, and liver cancer (36, 48, 51, 52, 55). In breast cancer, prediction of hormone receptor status (38, 39, 42) and homologous recombination deficiency (35, 40) has also been investigated. A common task for DL has also been the prediction of microsatellite instability, particularly in colorectal cancer (29, 31–36) and gastrointestinal cancer (40, 45, 46). Across all these studies, encouraging performance was measured with the area under the receiver operating characteristic (ROC) curve (AUC) on some external test sets above 0.80 (29, 29, 32, 34, 36–39, 45, 48). Although sensitivity and specificity may be, at this date, insufficient for end-to-end clinical implementations, this is a proof of concept that genotype–phenotype correlations can be identified using DL on H&E-stained tumor slides. Sirinukunwattana et al. (37) tackled a more complex task: a DL model predicted the four-class consensus molecular subtypes (CMS) of colorectal cancer, obtaining a 0.84 AUC on the TCGA external test set (N=431 slides from 430 patients). Among these DL-based studies, one research angle that has got some particular interests is explaining the DL predictions, frequently referred to as a “black box” and deriving human-interpretable features (31, 36, 37, 44). For instance, this can be done by extracting subregions of the input slide image that were assigned strong weights by the DL model to molecularly classify a given case. The visual assessment of these regions of interest can be used to reveal relevant morpho-molecular correlates, although this may not always provide human-interpretable visual information. Another approach can be to correlate the DL predictions with clinicopathological data.
Similar DL performance and interpretability still need to be shown in large cohorts of EC. To date, only Wang et al. (53) and Hong et al. (54) have trained supervised binary classification models from H&E-stained EC tumor slides and labels publicly available from the TCGA and Clinical Proteomic Tumour Analysis Consortium (CPTAC) datasets. Wang et al. (53) limited the predictions to high microsatellite instability (MSI) on the TCGA (N=516 of which 128 MSI patients) and obtained an AUC of 0.73 on 25% hold-out internal test set. Hong et al. (54) predicted various mutations and each of the four TCGA-derived molecular EC classes separately. To this end, they reached an AUC of 0.66 for POLE mutation (N=7 POLE-mutated patients), 0.76 for MSI (N=25 MSI patients), 0.87 for copy-number high (N=20 copy-number high patients), and 0.65 for copy-number low (N=43 copy-number low patients) on the CPTAC external test set. Additionally, they obtained an AUC of 0.77 for the prediction of the TP53 mutational status (N=30 TP53-mutated patients). Although both studies represent first proofs of concept of predicting genetic mutations from H&E slide images that can be expanded to EC, the test sets remained relatively small and did not reflect the heterogeneity of histological subtypes and FIGO grades known to be present in each molecular class. Specifically, with a few non-endometroid samples included in the TCGA (5) and CPTAC (96), the applicability to large cohorts of non-endometrioid EC remains unknown. In addition, the authors limited the scope of mutation prediction to binary classification tasks. Thus, to date, leveraging DL to predict the four-class molecular EC classification and deriving human-interpretable morpho-molecular correlates, have yet to be explored. Finally, the DL models used in both studies first divided the slide images into tiles, which is a standard computational method in the field, and then classified each tile individually with labels assigned at the slide-level. The tile-level classification may introduce training noise because morphological information in a given tile does not always correlate with the given true slide-level label. Hong et al. (54) have also acknowledged this architectural limitation, reporting that non-tumor tiles were often given inconclusive prediction scores. The discrepancy between tile and slide-level classification labels remains a well-known challenge in the field, which has started transitioning to more state-of-the-art DL architectures promising better performance (97–100).
Integrating deep learning into the current molecular EC classification
The gynecopathological community has started exploring how morphology-based information could be used to aid the molecular EC classification for an optimized risk-stratification strategy. Assistance to accurately weigh both histopathological and molecular variables would be welcome, as the number of relevant variables is steadily increasing (101). The innate strength of DL technology for the analysis of multi-modal datasets including both image and molecular information suggests that DL could aid in the refinement of the current morpho-molecular classification of EC.
EC-specific DL tasks could range from predicting one specific molecular alteration to predicting the complete four-class molecular classification from standard H&E images or from a combination of H&E images and special stains. To achieve this, the input data for DL models are digitized whole slide histopathology images of EC with the associated EC molecular classes. Importantly, such models achieve an incrementally increasing performance with the size and quality of the available datasets, ground-truth annotations, and advances in DL technology. Furthermore, such models can be purposely designed to generalize to previously unseen datasets and can be run in a cloud environment, potentially enabling broad access to AI-guided classification in future pathology.
This remarkable technological paradigm chance could support an increase in the fidelity of EC patient diagnosis, prognostic, and predictive classification impacting the whole diagnostic process and treatment decision-making. Theoretically, if a DL model predicts the four-class molecular EC classification at near perfect high specificity and sensitivity, then one could envision that DNA sequencing and immunohistochemistry would only be required for confirmatory testing, if at all. If such a DL model is also shown to be generalizable to external cohorts, EC patients could be molecularly classified using only digitized H&E-stained tumor slides. In this scenario, this automated tool would be clinically relevant by (i) providing a cost-effective alternative to expensive molecular testing without the need of additional tissue and (ii) speeding the diagnosis process up and advancing treatment initiation, which, in a real-world practice, can be delayed by weeks with next-generation sequencing. Until a clinical-grade accuracy of such model has been achieved, alternative and less complex DL tasks can be taken forward to support EC patient management. In fact, a binary predictive model trained toward one single specific molecular alteration may yield a higher sensitivity and specificity than a four-class model and could subsequently serve as a pre-screening tool. In the field of EC, pre-identifying p53abn EC in a population of low-risk EC may potentially be used to identify those few patients with a poor prognosis for confirmatory testing (15). Similarly, it may be possible to identify the aggressive subset of NSMP EC that lack hormone receptor expression (94). Furthermore, preselecting cases that would further require POLE testing given MMR-IHC and p53-IHC would be particularly supportive and cost saving in high-risk ECs, as treatment de-escalation of POLEmut EC with good prognosis is currently being explored (5, 14–17, 19, 65, 70).
An avenue by which DL has currently probably the biggest role is as a research tool combined with gynecopathological expertise. Several studies showed (36, 37, 44) that after training an EC-specific DL model, image-based information associated with EC molecular alterations could be visually extracted and reviewed by gynecopathologists. From there on, the morpho-molecular correlates that are outlined in this review may be confirmed, but the DL model may also reveal morpho-molecular features that have so far not struck the attention of human observers. Increasing knowledge about the morphology of the molecular classes will help to understand the biological processes and dynamics of tumor–host interaction in the tumor microenvironment. The prognostic value of the identified morpho-molecular features can be subsequently explored, which may open new doors to prognostic refinement in EC.
With increasing availability of digitalization aids such as cloud computing and resources, DL-driven diagnostic tools could be made available worldwide as an additional inexpensive, if not free, resource without the need of local hardware or knowledge (102). Particularly, users without access to scanners would only need to generate slide images using microscope cameras or even existing mobile phones for diagnostic classification in a central expert center. However, high-quality slide images may remain a limitation to the applicability in low-income countries and country-specific regulations on patient data transfer.
Discussion
In the past four decades, the classification of EC has evolved from a histology-based to a molecular system. The recent integration into guidelines indicates the increasing prognostic value of the four-class molecular classification over morphology in EC (1, 2), yet the integrated management with former histopathological variables is still a challenge in the diagnostic routine (9). As a result, questions have been raised about the relevance of these histopathological features and the role of morphology beyond the molecular EC classification. Now, given the four molecular EC classes, a number of studies have described some distinct morphological characteristics, but they remain insufficient to achieve accurate classification (66–70, 72, 73, 76, 83), and the pathologist’s eyes are not sufficiently trained to spotlight them. In fact, this review stresses the difficulty in weighing image-based information in relation to the current four molecular EC classes. First, each molecular subclass shows heterogeneity for histological subtype, FIGO grade, and associated microscopic features. Second, many microscopic features appear to be non-exclusive, for instance the presence of high levels of immune cells between POLEmut EC and MMRd EC (69, 70). Lastly, some morphological traits are detectable at different magnifications and growth patterns, and nuclear atypia within p53abn EC is one example.
In the quickly progressing research domain of computer science, DL has demonstrated a well-known capability to work with high-dimensional and multi-modal datasets, up to learn phenotype–genotype correlates from highly complex and extra-large digitized tumor slides (29–52, 54, 55). Hence, future DL-based breakthroughs have legitimate potential to resolve the current dilemma between molecular and histopathological variables or even support EC patient management for pre-screening and decision-making on treatment, ultimately impacting EC diagnostics and patient care as a whole. As for today, an urgent assignment given to DL technology in combination with gynecopathological expertise is bringing to the surface the clinical relevance of each morphological feature associated with the four molecular EC subclasses, while improving morphological and biological understanding of the genomic EC alterations. Combining the strengths of molecular-, clinical-, and DL-based information may refine the EC classification to reach optimal prognostication and prediction for our future EC patients.
Author contributions
SF wrote sections of the review. All authors contributed to manuscript revision, read, and approved the submitted version.
Funding
This work was supported by an unrestricted grant by the Hanarth Foundation.
Conflict of interest
VK: Research grants from Swiss Federal Institute of Technology Strategic Focus Area: Personalized Health and Related Technologies PHRT, the Swiss National Science Foundation and Promedica unrelated to the current works. NH: Unrestricted research grants unrelated to the current work from the Dutch Cancer Society and Varian. TB: Research grants from the Dutch Cancer Society unrelated to the current work.
The remaining authors declare that the research was conducted in the absence of any commercial or financial relationships that could be construed as a potential conflict of interest.
Publisher’s note
All claims expressed in this article are solely those of the authors and do not necessarily represent those of their affiliated organizations, or those of the publisher, the editors and the reviewers. Any product that may be evaluated in this article, or claim that may be made by its manufacturer, is not guaranteed or endorsed by the publisher.
References
1. Concin N, Matias-Guiu X, Vergote I, Cibula D, Mirza M, Marnitz S, et al. ESGO/ESTRO/ESP guidelines for the management of patients with endometrial carcinoma. Int J Gynecol Cancer (2021) 31(1):12–39. doi: 10.1136/ijgc-2020-002230
2. Herrington CS , (ed.), Editorial Board WHOCOT. WHO Classification of Tumours Female Genital Tumours. 5th ed. International Agency for Research on Cancer (2020).
3. Bokhman J. Two pathogenetic types of endometrial carcinoma. Gynecol Oncol (1983) 15(1):10–7. doi: 10.1016/0090-8258(83)90111-7
4. Amant F, Mirza MR, Koskas M, Creutzberg CL. Cancer of the corpus uteri. Int J Gynaecol Obstet Off Organ Int Fed Gynaecol Obstet (2018) 143 (Suppl 2):37–50. doi: 10.1002/ijgo.12612
5. Cancer Genome Atlas Research Network, Kandoth C, Schultz N, Cherniack A, Akbani R, Liu Y, et al. Integrated genomic characterization of endometrial carcinoma. Nature (2013) 497(7447):67–73. doi: 10.1038/nature12325
6. Singh N, Piskorz A, Bosse T, Jimenez-Linan M, Rous B, Brenton J, et al. p53 immunohistochemistry is an accurate surrogate for TP53 mutational analysis in endometrial carcinoma biopsies. J Pathol (2020) 250(3):336–45. doi: 10.1002/path.5375
7. Vermij L, Léon-Castillo A, Singh N, Powell ME, Edmondson RJ, Genestie C. TransPORTEC consortium. p53 immunohistochemistry in endometrial cancer: clinical and molecular correlates in the PORTEC-3 tria. In: Mod Pathol (2022). doi: 10.1038/s41379-022-01102-x.
8. León-Castillo A, Gilvazquez E, Nout R, Smit V, McAlpine J, McConechy M, et al. Clinicopathological and molecular characterisation of “multiple-classifier” endometrial carcinomas. J Pathol (2020) 250:312–22. doi: 10.1002/path.5373
9. Vermij L, Smit V, Nout R, Bosse T. Incorporation of molecular characteristics into endometrial cancer management. Histopathology (2020) 76:52–63. doi: 10.1111/his.14015
10. Hoang L, Kinloch M, Leo J, Grondin K, Lee C, Ewanowich C, et al. Interobserver agreement in endometrial carcinoma histotype diagnosis varies depending on the cancer genome atlas (TCGA)-based molecular subgroup. Am J Surg Pathol (2017) 41:245–52. doi: 10.1097/PAS.0000000000000764
11. Boennelycke M, Peters EEM, Léon-Castillo A, Smit VTHBM, Bosse T, Christensen IJ, et al. Prognostic impact of histological review of high-grade endometrial carcinomas in a large Danish cohort. Virchows Arch Int J Pathol (2021) 479(3):507–14. doi: 10.1007/s00428-021-03133-2
12. de Boer SM, Wortman BG, Bosse T, Powell ME, Singh N, Hollema H, et al. Clinical consequences of upfront pathology review in the randomised PORTEC-3 trial for high-risk endometrial cancer. Ann Oncol (2018) 29(2):424–30. doi: 10.1093/annonc/mdx753
13. Perrone E, Felice FD, Capasso I, Distefano E, Lorusso D, Nero C, et al. The immunohistochemical molecular risk classification in endometrial cancer: A pragmatic and high-reproducibility method. Gynecol Oncol (2022) 165(3):585–93. doi: 10.1016/j.ygyno.2022.03.009
14. León-Castillo A, de Boer S, Powel M, Mileshkin L, Mackay H, Leary A, et al. Molecular classification of the PORTEC-3 trial for high-risk endometrial cancer: Impact on prognosis and benefit from adjuvant therapy. J Clin Oncol (2020) 38:3388–97. doi: 10.1200/JCO.20.00549
15. Stelloo E, Nout R, Osse E, Jürgenliemk-Schulz I, Jobsen J, Lutgens L, et al. Improved risk assessment by integrating molecular and clinicopathological factors in early-stage endometrial cancer–combined analysis of the PORTEC cohorts. Clin Cancer Res (2016) 22:4215–24. doi: 10.1158/1078-0432.CCR-15-2878
16. Bosse T, Nout RA, McAlpine JN, McConechy MK, Britton H, Hussein YR, et al. Molecular classification of grade 3 endometrioid endometrial cancers identifies distinct prognostic subgroups. Am J Surg Pathol (2018) 42(5):561–8. doi: 10.1097/PAS.0000000000001020
17. Kommoss S, McConechy MK, Kommoss F, Leung S, Bunz A, Magrill J, et al. Final validation of the ProMisE molecular classifier for endometrial carcinoma in a large population-based case series. Ann Oncol Off J Eur Soc Med Oncol (2018) 29(5):1180–8. doi: 10.1093/annonc/mdy058
18. Leon-Castillo A, Horeweg N, Peters E, Rutten T, Smit V, Kroon D, et al. Prognostic relevance of the molecular classification in high-grade endometrial cancer for patients staged by lymphadenectomy and without adjuvant treatment. Gynecol Oncol (2022) 164(3):577–586. doi: 10.1016/j.ygyno.2022.01.00
19. Talhouk A, McConechy MK, Leung S, Yang W, Lum A, Senz J, et al. Confirmation of ProMisE: A simple, genomics-based clinical classifier for endometrial cancer. Cancer (2017) 123(5):802–13. doi: 10.1002/cncr.30496
20. Talhouk A, McConechy MK, Leung S, Li-Chang HH, Kwon JS, Melnyk N, et al. A clinically applicable molecular-based classification for endometrial cancers. Br J Cancer (2015) 113(2):299–310. doi: 10.1038/bjc.2015.190
21. Alexa M, Hasenburg A, Battista MJ. The TCGA molecular classification of endometrial cancer and its possible impact on adjuvant treatment decisions. Cancers (Basel) (2021) 13(6):1478. doi: 10.3390/cancers13061478
22. van den Heerik ASVM, Horeweg N, Nout RA, Lutgens LCHW, van der Steen-Banasik EM, Westerveld GH, et al. PORTEC-4a: international randomized trial of molecular profile-based adjuvant treatment for women with high-intermediate risk endometrial cancer. Int J Gynecol Cancer Off J Int Gynecol Cancer Soc (2020) 30(12):2002–7. doi: 10.1136/ijgc-2020-001929
23. Bosse T, Powell M, Crosbie E, Leary A, Kroep J, Han K, et al. 595 implementation of collaborative translational research (TransPORTEC) findings in an international endometrial cancer clinical trials program (RAINBO). Int J Gynecol Cancer (2021) 31(Suppl 3):A108. doi: 10.1136/ijgc-2021-ESGO.171
24. Jamieson A, Bosse T, McAlpine JN. The emerging role of molecular pathology in directing the systemic treatment of endometrial cancer. Ther Adv Med Oncol (2021) 13:17588359211035960. doi: 10.1177/17588359211035959
25. Krizhevsky A, Sutskever I, Hinton GE. ImageNet classification with deep convolutional neural networks. In: Pereira F, Burges CJC, Bottou L, Weinberger KQ, editors. Proceedings of the 25th International Conference on Neural Information Processing Systems - Volume 1. Red Hook, NY,USA: Curran Associates Inc (2012) . p. 1097–105. (NIPS’12).
26. Litjens G, Bandi P, Ehteshami Bejnordi B, Geessink O, Balkenhol M, Bult P, et al. 1399 H&E-stained sentinel lymph node sections of breast cancer patients: the CAMELYON dataset. GigaScience (2018) 7(6):giy065. doi: 10.1093/gigascience/giy065
27. Russakovsky O, Deng J, Su H, Krause J, Satheesh S, Ma S, et al. ImageNet Large scale visual recognition challenge. Int J Comput Vis IJCV (2015) 115(3):211–52. doi: 10.1007/s11263-015-0816-y
28. Cifci D, Foersch S, Kather JN. Artificial intelligence to identify genetic alterations in conventional histopathology. J Pathol (2022) 257(4):430–444. doi: 10.1002/path.5898
29. Echle A, Grabsch HI, Quirke P, van den Brandt PA, West NP, Hutchins GGA, et al. Clinical-grade detection of microsatellite instability in colorectal tumors by deep learning. Gastroenterology (2020) 159(4):1406–1416.e11. doi: 10.1053/j.gastro.2020.06.021
30. Jang HJ, Lee A, Kang J, Song IH, Lee SH. Prediction of clinically actionable genetic alterations from colorectal cancer histopathology images using deep learning. World J Gastroenterol (2020) 26(40):6207–23. doi: 10.3748/wjg.v26.i40.6207
31. Yamashita R, Long J, Longacre T, Peng L, Berry G, Martin B, et al. Deep learning model for the prediction of microsatellite instability in colorectal cancer: a diagnostic study. Lancet Oncol (2021) 22(1):132–41. doi: 10.1016/S1470-2045(20)30535-0
32. Lee SH, Song IH, Jang HJ. Feasibility of deep learning-based fully automated classification of microsatellite instability in tissue slides of colorectal cancer. Int J Cancer (2021) 149(3):728–40. doi: 10.1002/ijc.33599
33. Cao R, Yang F, Ma SC, Liu L, Zhao Y, Li Y, et al. Development and interpretation of a pathomics-based model for the prediction of microsatellite instability in colorectal cancer. Theranostics (2020) 10(24):11080–91. doi: 10.7150/thno.49864
34. Schrammen PL, Ghaffari Laleh N, Echle A, Truhn D, Schulz V, Brinker TJ, et al. Weakly supervised annotation-free cancer detection and prediction of genotype in routine histopathology. J Pathol (2022) 256(1):50–60. doi: 10.1002/path.5800
35. Schirris Y, Gavves E, Nederlof I, Horlings HM, Teuwen J. DeepSMILE: Contrastive selfsupervised pre-training benefits MSI and HRD classification directly from H&E wholeslide images in colorectal and breast cancer. Med Image Anal (2022) 79:102464. doi: 10.1016/j.media.2022.102464
36. Bilal M, Raza SEA, Azam A, Graham S, Ilyas M, Cree IA, et al. Development and validation of a weakly supervised deep learning framework to predict the status of molecular pathways and key mutations in colorectal cancer from routine histology images: a retrospective study. Lancet Digit Health (2021) 3(12):e763–e772. doi: 10.1016/S2589-7500(21)00180-1
37. Sirinukunwattana K, Domingo E, Richman S, Redmond K, Blake A, Verrill C, et al. Image-based consensus molecular subtype (imCMS) classification of colorectal cancer using deep learning. Gut (2021) 70:544–54. doi: 10.1136/gutjnl-2019-319866
38. Naik N, Madani A, Esteva A, Keskar NS, Press MF, Ruderman D, et al. Deep learning-enabled breast cancer hormonal receptor status determination from base-level H&E stains. Nat Commun (2020) 11(1):5727. doi: 10.1038/s41467-020-19334-3
39. Rawat RR, Ortega I, Roy P, Sha F, Shibata D, Ruderman D, et al. Deep learned tissue “fingerprints” classify breast cancers by ER/PR/Her2 status from H&E images. Sci Rep (2020) 10(1):7275. doi: 10.1038/s41598-020-64156-4.
40. Valieris R, Amaro L, Osório CAB de T, Bueno AP, Rosales Mitrowsky RA, Carraro DM, et al. Deep learning predicts underlying features on pathology images with therapeutic relevance for breast and gastric cancer. Cancers (Basel) (2020) 12(12):3687. doi: 10.3390/cancers12123687
41. Qu H, Zhou M, Yan Z, Wang H, Rustgi VK, Zhang S, et al. Genetic mutation and biological pathway prediction based on whole slide images in breast carcinoma using deep learning. NPJ Precis Oncol (2021) 5(1):87. doi: 10.1038/s41698-021-00225-9
42. Farahmand S, Fernandez AI, Ahmed FS, Rimm DL, Chuang JH, Reisenbichler E, et al. Deep learning trained on hematoxylin and eosin tumor region of interest predicts HER2 status and trastuzumab treatment response in HER2+ breast cancer. Mod Pathol Off J U S Can Acad Pathol Inc (2022) 35(1):44–51. doi: 10.1038/s41379-021-00911-w
43. Wang X, Zou C, Zhang Y, Li X, Wang C, Ke F, et al. Prediction of BRCA gene mutation in breast cancer based on deep learning and histopathology images. Front Genet [Internet] (2021) 12:661109. doi: 10.3389/fgene.2021.661109
44. Diao JA, Wang JK, Chui WF, Mountain V, Gullapally SC, Srinivasan R, et al. Human-interpretable image features derived from densely mapped cancer pathology slides predict diverse molecular phenotypes. Nat Commun (2021) 12(1):1613. doi: 10.1038/s41467-021-21896-9
45. Kather J, Pearson A, Halama N, Jäger D, Krause J, Loosen S, et al. Deep learning can predict microsatellite instability directly from histology in gastrointestinal cancer. Nat Med (2019) 25:1054–6. doi: 10.1038/s41591-019-0462-y
46. Muti HS, Heij LR, Keller G, Kohlruss M, Langer R, Dislich B, et al. Development and validation of deep learning classifiers to detect Epstein-Barr virus and microsatellite instability status in gastric cancer: a retrospective multicentre cohort study. Lancet Digit Health (2021) 3(10):e654–64. doi: 10.1016/S2589-7500(21)00133-3
47. Kather JN, Heij LR, Grabsch HI, Loeffler C, Echle A, Muti HS, et al. Pan-cancer image-based detection of clinically actionable genetic alterations. Nat Cancer (2020) 1(8):789–99. doi: 10.1038/s43018-020-0087-6
48. Chen M, Zhang B, Topatana W, Cao J, Zhu H, Juengpanich S, et al. Classification and mutation prediction based on histopathology H&E images in liver cancer using deep learning. NPJ Precis Oncol (2020) 4(1):14. doi: 10.1038/s41698-020-0120-3
49. Coudray N, Ocampo PS, Sakellaropoulos T, Narula N, Snuderl M, Fenyö D, et al. Classification and mutation prediction from non-small cell lung cancer histopathology images using deep learning. Nat Med (2018) 24(10):1559–67. doi: 10.1038/s41591-018-0177-5
50. Schmauch B, Romagnoni A, Pronier E, Saillard C, Maillé P, Calderaro J, et al. A deep learning model to predict RNA-seq expression of tumours from whole slide images. Nat Commun (2020) 11(1):3877. doi: 10.1038/s41467-020-17678-4
51. Noorbakhsh J, Farahmand S, Foroughi Pour A, Namburi S, Caruana D, Rimm D, et al. Deep learning-based cross-classifications reveal conserved spatial behaviors within tumor histological images. Nat Commun (2020) 11(1):6367. doi: 10.1038/s41467-020-20030-5
52. Fu Y, Jung AW, Torne RV, Gonzalez S, Vöhringer H, Shmatko A, et al. Pan-cancer computational histopathology reveals mutations, tumor composition and prognosis. Nat Cancer (2020) 1(8):800–10. doi: 10.1038/s43018-020-0085-8
53. Wang T, Lu W, Yang F, Liu L, Dong Z, Tang W, et al. Microsatellite instability prediction of uterine corpus endometrial carcinoma based on H&E histology whole-slide imaging. In: 2020 IEEE 17th international symposium on biomedical imaging (ISBI) (2020) IEEE. p. 1289–92.
54. Hong R, Liu W, DeLair D, Razavian N, Fenyö D. Predicting endometrial cancer subtypes and molecular features from histopathology images using multi-resolution deep learning models. Cell Rep Med (2021) 2(9):100400. doi: 10.1016/j.xcrm.2021.100400
55. Jang HJ, Lee A, Kang J, Song IH, Lee SH. Prediction of genetic alterations from gastric cancer histopathology images using a fully automated deep learning approach. World J Gastroenterol (2021) 27:7687–704. doi: 10.3748/wjg.v27.i44.7687
56. Vale-Silva LA, Rohr K. Long-term cancer survival prediction using multimodal deep learning. Sci Rep (2021) 11(1):13505. doi: 10.1038/s41598-021-92799-4
57. Schulz S, Woerl AC, Jungmann F, Glasner C, Stenzel P, Strobl S, et al. Multimodal deep learning for prognosis prediction in renal cancer. Front Oncol (2021) 11:788740. doi: 10.3389/fonc.2021.788740
58. Courtiol P, Maussion C, Moarii M, Pronier E, Pilcer S, Sefta M, et al. Deep learning-based classification of mesothelioma improves prediction of patient outcome. Nat Med (2019) 25(10):1519–25. doi: 10.1038/s41591-019-0583-3
59. Yamashita R, Long J, Saleem A, Rubin DL, Shen J. Deep learning predicts postsurgical recurrence of hepatocellular carcinoma from digital histopathologic images. Sci Rep (2021) 11(1):2047. doi: 10.1038/s41598-021-81506-y
60. Wang X, Chen Y, Gao Y, Zhang H, Guan Z, Dong Z, et al. Predicting gastric cancer outcome from resected lymph node histopathology images using deep learning. Nat Commun (2021) 12(1):1637. doi: 10.1038/s41467-021-21674-7
61. Church DN, Briggs SEW, Palles C, Domingo E, Kearsey SJ, Grimes JM, et al. DNA Polymerase ϵ and δ exonuclease domain mutations in endometrial cancer. Hum Mol Genet (2013) 22(14):2820–8. doi: 10.1093/hmg/ddt131
62. Conlon N, Da Cruz Paula A, Ashley C, Segura S, De Brot L, da Silva E, et al. Endometrial carcinomas with a “Serous” component in young women are enriched for DNA mismatch repair deficiency, lynch syndrome, and POLE exonuclease domain mutations. Am J Surg Pathol (2020) 44:641–8. doi: 10.1097/PAS.0000000000001461
63. Kim S, Cloutier B, Leung S, Cochrane D, Britton H, Pina A, et al. Molecular subtypes of clear cell carcinoma of the endometrium: Opportunities for prognostic and predictive stratification. Gynecol Oncol (2020) 158:3–11. doi: 10.1016/j.ygyno.2020.04.043
64. Gotoh O, Sugiyama Y, Takazawa Y, Kato K, Tanaka N, Omatsu K, et al. Clinically relevant molecular subtypes and genomic alteration-independent differentiation in gynecologic carcinosarcoma. Nat Commun (2019) 10:4965. doi: 10.1038/s41467-019-12985-x
65. Imboden S, Nastic D, Ghaderi M, Rydberg F, Rau T, Mueller M, et al. Phenotype of POLE-mutated endometrial cancer. PLoS One (2019) 14:e0214318. doi: 10.1371/journal.pone.0214318
66. Van Gool I, Ubachs J, Stelloo E, de Kroon C, Goeman J, Smit V, et al. Blinded histopathological characterisation of POLE exonuclease domain-mutant endometrial cancers: sheep in wolf’s clothing. Histopathology (2018) 72:248–58. doi: 10.1111/his.13338
67. Bakhsh S, Kinloch M, Hoang L, Soslow R, Köbel M, Lee C, et al. Histopathological features of endometrial carcinomas associated with POLE mutations: implications for decisions about adjuvant therapy. Histopathology (2016) 68:916–24. doi: 10.1111/his.12878
68. Hussein Y, Weigelt B, Levine D, Schoolmeester J, Dao L, Balzer B, et al. Clinicopathological analysis of endometrial carcinomas harboring somatic POLE exonuclease domain mutations. Mod Pathol (2015) 28:505–14. doi: 10.1038/modpathol.2014.143
69. Horeweg N, de Bruyn M, Nout R, Stelloo E, Kedziersza K, León-Castillo A, et al. Prognostic integrated image-based immune and molecular profiling in early-stage endometrial cancer. Cancer Immunol Res (2020) 8:1508–19. doi: 10.1158/2326-6066.CIR-20-0149
70. Talhouk A, Derocher H, Schmidt P, Leung S, Milne K, Gilks C, et al. Molecular subtype not immune response drives outcomes in endometrial carcinoma. Clin Cancer Res (2019) 25:2537–48. doi: 10.1158/1078-0432.CCR-18-3241
71. Raffone A, Travaglino A, Raimondo D, Boccellino M, Maletta M, Borghese G, et al. Tumor-infiltrating lymphocytes and POLE mutation in endometrial carcinoma. Gynecol Oncol (2021) 161:621–8. doi: 10.1016/j.ygyno.2021.02.030
72. Horeweg N, Workel H, Church D, Vermij L, León-Castillo A, Krog R, et al. Tertiary lymphoid structures critical for prognosis in endometrial cancer patients. (2021) 13(1):1373. doi: 10.1038/s41467-022-29040-x
73. Lin Z, Huang L, Li S, Gu J, Cui X, Zhou Y. Pan-cancer analysis of genomic properties and clinical outcome associated with tumor tertiary lymphoid structure. Sci Rep (2020) 10(1):21530. doi: 10.1038/s41598-020-78560-3
74. Shimizu Y, Suzuki S, Ukai M, Hattori S, Yoshikawa N, Kajiyama H. The prognostic significance of peritumoral lymphocytes’ band-like structure in type II endometrial cancer. Anticancer Res (2021) 41(1):249–58. doi: 10.21873/anticanres.14771
75. Kim K, Hwangbo S, Kim H, Kim YB, No JH, Suh DH, et al. Clinicopathologic and protein markers distinguishing the “polymerase epsilon exonuclease” from the “copy number low” subtype of endometrial cancer. J Gynecol Oncol (2022) 33(3):e27. doi: 10.3802/jgo.2022.33.e27
76. Howitt B, Shukla S, Sholl L, Ritterhouse L, Watkins J, Rodig S, et al. Association of polymerase e-mutated and microsatellite-instable endometrial cancers with neoantigen load, number of tumor-infiltrating lymphocytes, and expression of PD-1 and PD-L1. JAMA Oncol (2015) 1:1319–23. doi: 10.1001/jamaoncol.2015.2151
77. Kim SR, Pina A, Albert A, McAlpine J, Wolber R, Blake Gilks C, et al. Does MMR status in endometrial cancer influence response to adjuvant therapy? Gynecol Oncol (2018) 151(1):76–81. doi: 10.1016/j.ygyno.2018.08.020
78. Devereaux KA, Weiel JJ, Pors J, Steiner DF, Ho C, Charu V, et al. Prospective molecular classification of endometrial carcinomas: institutional implementation, practice, and clinical experience. Mod Pathol Off J U S Can Acad Pathol Inc (2021) 35(5):688–696. doi: 10.1038/s41379-021-00963-y
79. Segura SE, Pedra Nobre S, Hussein YR, Abu-Rustum NR, Weigelt B, Soslow RA, et al. DNA Mismatch repair-deficient endometrial carcinosarcomas portend distinct clinical, morphologic, and molecular features compared with traditional carcinosarcomas. Am J Surg Pathol (2020) 44(11):1573–9. doi: 10.1097/PAS.0000000000001561
80. Post C, Stelloo E, Smit V, Ruano D, Tops C, Vermij L, et al. Prevalence and prognosis of lynch syndrome and sporadic mismatch repair deficiency in endometrial cancer. J Natl Cancer Inst (2021) 113:1212–20. doi: 10.1093/jnci/djab029
81. Moukarzel L, Da Cruz Paula A, Ferrando L, Hoang T, Sebastiao A, Pareja F, et al. Clonal relationship and directionality of progression of synchronous endometrial and ovarian carcinomas in patients with DNA mismatch repair-deficiency associated syndrome. Mod Pathol (2021) 34:994–1007. doi: 10.1038/s41379-020-00721-6
82. Zhang K, Liu Y, Liu X, Du J, Wang Y, Yang J, et al. Clinicopathological significance of multiple molecular features in undifferentiated and dedifferentiated endometrial carcinomas. Pathol (Phila) (2021) 53(2):179–86. doi: 10.1016/j.pathol.2020.07.015
83. Kang E, Wiebe N, Aubrey C, Lee C, Anglesio M, Tilley D, et al. Selection of endometrial carcinomas for p53 immunohistochemistry based on nuclear features. J Pathol Clin Res (2022) 8:19–32. doi: 10.1002/cjp2.243
84. Momeni-Boroujeni A, Dahoud W, Vanderbilt CM, Chiang S, Murali R, Rios-Doria EV, et al. Clinicopathologic and genomic analysis of TP53-mutated endometrial carcinomas. Clin Cancer Res Off J Am Assoc Cancer Res (2021) 27(9):2613–23. doi: 10.1158/1078-0432
85. Brett MA, Atenafu EG, Singh N, Ghatage P, Clarke BA, Nelson GS, et al. Equivalent survival of p53 mutated endometrial endometrioid carcinoma grade 3 and endometrial serous carcinoma. Int J Gynecol Pathol Off J Int Soc Gynecol Pathol (2021) 40(2):116–23. doi: 10.1097/PGP.0000000000000674
86. Ross DS, Devereaux KA, Jin C, Lin DY, Zhang Y, Marra A, et al. Histopathologic features and molecular genetic landscape of HER2-amplified endometrial carcinomas. Mod Pathol Off J U S Can Acad Pathol Inc (2022) 35(7):962–971. doi: 10.1038/s41379-021-00997-2
87. Costigan D, Dong F, Nucci M, Howitt B. Clinicopathologic and immunohistochemical correlates of CTNNB1 mutated endometrial endometrioid carcinoma. Int J Gynecol Pathol (2020) 39:119–27. doi: 10.1097/PGP.0000000000000583
88. Stelloo E, Bosse T, Nout RA, MacKay HJ, Church DN, Nijman HW, et al. Refining prognosis and identifying targetable pathways for high-risk endometrial cancer; a TransPORTEC initiative. Mod Pathol Off J U S Can Acad Pathol Inc (2015) 28(6):836–44. doi: 10.1038/modpathol.2015.43
89. Ashihara K, Saito T, Mizumoto H, Adachi K, Tanaka R, Nishimura M, et al. Loss of γ-catenin expression in squamous differentiation in endometrial carcinomas. Int J Gynecol Pathol (2002) 21(3):246–54. doi: 10.1097/00004347-200207000-00007
90. De Leo A, de Biase D, Lenzi J, Barbero G, Turchetti D, Grillini M, et al. ARID1A and CTNNB1/β-catenin molecular status affects the clinicopathologic features and prognosis of endometrial carcinoma: Implications for an improved surrogate molecular classification. Cancers [Internet] (2021) 13(5):950. doi: 10.3390/cancers13050950
91. Stewart CJR, Amanuel B, Grieu F, Carrello A, Iacopetta B. KRAS mutation and microsatellite instability in endometrial adenocarcinomas showing MELF-type myometrial invasion. J Clin Pathol (2010) 63(7):604–8. doi: 10.1136/jcp.2009.069500
92. He D, Wang H, Dong Y, Zhang Y, Zhao J, Lv C, et al. POLE mutation combined with microcystic, elongated and fragmented (MELF) pattern invasion in endometrial carcinomas might be associated with poor survival in Chinese women. Gynecol Oncol (2020) 159(1):36–42. doi: 10.1016/j.ygyno.2020.07.102
93. an den Heerik ASVM, Aiyer KTS, Stelloo E, Jürgenliemk-Schulz IM, Lutgens LCHW, Jobsen JJ, et al. Microcystic elongated and fragmented (MELF) pattern of invasion: Molecular features and prognostic significance in the PORTEC-1 and -2 trials. Gynecol Oncol (2022) S0090-8258(22):00431–0. doi: 10.1016/j.ygyno.2022.06.027
94. Vermij L. Molecular profiling of NSMP high-risk endometrial cancers of the PORTEC-3 trial - prognostic refinement and druggable targets. In: 22nd European congress on gynaecological oncology. Prague, Czech Republic (2021).
95. Vermij L. Prognostic relevance of FIGO grading is limited to NSMP endometrial carcinomas. In: USCAP 111th annual meeting;. Los Angeles, CA (2022).
96. Dou Y, Kawaler EA, Zhou DC, Gritsenko MA, Huang C, Blumenberg L, et al. Proteogenomic characterization of endometrial carcinoma. Cell (2020) 180(4):729–48.e26. doi: 10.1016/j.cell.2020.01.026
97. Campanella G, Hanna MG, Geneslaw L, Miraflor A, Werneck Krauss Silva V, Busam KJ, et al. Clinical-grade computational pathology using weakly supervised deep learning on whole slide images. Nat Med (2019) 25(8):1301–9. doi: 10.1038/s41591-019-0508-1
98. Lu MY, Williamson DFK, Chen TY, Chen RJ, Barbieri M, Mahmood F. Data-efficient and weakly supervised computational pathology on whole-slide images. Nat BioMed Eng (2021) 5(6):555–70. doi: 10.1038/s41551-020-00682-w
99. Lu MY, Chen TY, Williamson DFK, Zhao M, Shady M, Lipkova J, et al. AI-Based pathology predicts origins for cancers of unknown primary. Nature (2021) 594(7861):106–10. doi: 10.1038/s41586-021-03512-4
100. Lipkova J, Chen TY, Lu MY, Chen RJ, Shady M, Williams M, et al. Deep learning-enabled assessment of cardiac allograft rejection from endomyocardial biopsies. Nat Med (2022) 28(3):575–82. doi: 10.1038/s41591-022-01709-2
101. Wu J, Horeweg N, de Bruyn M, Nout RA, Jürgenliemk-Schulz IM, Lutgens LCHW, et al. Automated causal inference in application to randomized controlled clinical trials. Nat Mach Intell (2022) 4:436–44. doi: 10.1038/s42256-022-00470-y
102. Schubert M. Erasing pathology’s borders. how even the simplest outreach can change the lives of pathologists and patients with few resources. Lab Digit Comput Pathol Precis Med Technol Innov Softw Hardw (2019). Available at: https://thepathologist.com/inside-the-lab/erasing-pathologys-borders
Keywords: endometrial carcinoma, tumour morphology, computer vision, deep learning, molecular classification, phenotype, whole slide image, histopathology image
Citation: Fremond S, Koelzer VH, Horeweg N and Bosse T (2022) The evolving role of morphology in endometrial cancer diagnostics: From histopathology and molecular testing towards integrative data analysis by deep learning. Front. Oncol. 12:928977. doi: 10.3389/fonc.2022.928977
Received: 26 April 2022; Accepted: 15 July 2022;
Published: 18 August 2022.
Edited by:
Tea Lanisnik Rizner, University of Ljubljana, SloveniaReviewed by:
Matthew Schlumbrecht, University of Miami Health System, United StatesRita Trozzi, Agostino Gemelli University Polyclinic (IRCCS), Italy
Elena Teodorico, Agostino Gemelli University Polyclinic (IRCCS), Italy
Copyright © 2022 Fremond, Koelzer, Horeweg and Bosse. This is an open-access article distributed under the terms of the Creative Commons Attribution License (CC BY). The use, distribution or reproduction in other forums is permitted, provided the original author(s) and the copyright owner(s) are credited and that the original publication in this journal is cited, in accordance with accepted academic practice. No use, distribution or reproduction is permitted which does not comply with these terms.
*Correspondence: Tjalling Bosse, t.bosse@lumc.nl