- 1Department of Pathophysiology, Key Laboratory of Cell Differentiation and Apoptosis of Chinese Ministry of Education, Shanghai Jiao Tong University, School of Medicine, Shanghai, China
- 2Zhiyuan Innovative Research Center, Shanghai Jiao Tong University, Shanghai, China
- 3State Key Laboratory of Quality Research in Chinese Medicine, Institute of Chinese Medical Sciences, University of Macau, Macao, Macao SAR, China
- 4Medicinal Chemistry and Bioinformatics Center, Shanghai Jiao Tong University, School of Medicine, Shanghai, China
- 5Oncology Department, Xin Hua Hospital Affiliated to Shanghai Jiao Tong University, School of Medicine, Shanghai, China
Special oncogenic mutations in the RAS proteins lead to the aberrant activation of RAS and its downstream signaling pathways. AMG510, the first approval drug for KRAS, covalently binds to the mutated cysteine 12 of KRASG12C protein and has shown promising antitumor activity in clinical trials. Recent studies have reported that the clinically acquired Y96D mutation could severely affect the effectiveness of AMG510. However, the underlying mechanism of the drug-resistance remains unclear. To address this, we performed multiple microsecond molecular dynamics simulations on the KRASG12C−AMG510 and KRASG12C/Y96D−AMG510 complexes at the atomic level. The direct interaction between the residue 96 and AMG510 was impaired owing to the Y96D mutation. Moreover, the mutation yielded higher flexibility and more coupled motion of the switch II and α3-helix, which led to the departing motion of the switch II and α3-helix. The resulting departing motion impaired the interaction between the switch II and α3-helix and subsequently induced the opening and loosening of the AMG510 binding pocket, which further disrupted the interaction between the key residues in the pocket and AMG510 and induced an increased solvent exposure of AMG510. These findings reveal the resistance mechanism of AMG510 to KRASG12C/Y96D, which will help to offer guidance for the development of KRAS targeted drugs to overcome acquired resistance.
Introduction
The RAS proteins, as a group of small GTPases, play a critical role in transduction of intracellular signaling pathways controlling cell proliferation, differentiation and apoptosis (1). It cycles between the GTP-bound active state and GDP-bound inactive state, acting as a binary switch. The cycle regulates downstream signaling pathways such as Raf/MEK/ERK pathway and PI3K/AKT/mTOR pathway (2). The activation of RAS relies on guanine nucleotide exchange factors (GEFs) that catalyze the exchange of GDP by GTP. In contrast, RAS is inactivated by its intrinsic GTPase activity and can be assisted by GTPase-activating proteins (GAPs) (3). Specific mutations of RAS inhibit its inactivation and induces the aberrant activation of downstream pathways, leading to oncogenesis (4, 5). Accumulating evidence indicates that oncogenic RAS mutations participate in approximately 30% of human cancers and induce over 1 million deaths per year worldwide (6, 7). There are four closely related RAS isoforms: HRAS, KRAS-4A, KRAS-4B and NRAS. Among them, KRAS mutations account for 85% of observed RAS mutations in cancer (8). KRAS mutations are dominated by single-base missense mutations, and 98% of which are found at three mutation hotspots: G12, G13, and Q61 (6, 9). Compared with other isoforms, KRAS is the only RAS isoform where the position 12 mutations are predominant (10). GTPase cycle is based on the conformational transition of RAS. Three functional elements in the effector lobe (residues 1–87) of Ras, including P-loop (residues 10–17), switch I (residues 32– 38), and switch II (residues 59–76), are pivotal for such conformational changes (11). With GTP bound, the three elements form the closed conformation of the catalytic site residing in the active state, while they adopt the open conformation after GTP hydrolysis residing in the inactive state (12). In the active state, Tyr32 on the switch I domain is in the “up” conformation and the α2-helix on the switch II domain is positioned in the “inward” conformation towards the α3-helix, denoted as “α2-helixin” (13). In contrast, the inactive state adopts the “Tyr32down” and “α2-helixin” conformation. To be noted, specific mutations in the switches can also affect the conformational transition of RAS (14). For example, D33E in the switch I can stabilize the open conformation of RAS even with GTP binding (15).
Previously, Ras was considered as ‘‘undruggable” due to its picomolar affinities towards GDP/GTP in the orthosteric site and the lack of suitable binding sites along the surface (16–18). However, many of the newly proposed drug design strategies including reversed allosteric communication are gradually turning the ‘‘undruggable” RAS “drugged” (19–27). The most exciting advance is the advent of KRASG12C inhibitors (28–31). They bind covalently to the mutated cysteine 12 and lock the KRAS in the inactive state, thereby inhibiting the hyperactivation of downstream signaling (32–34). AMG510 (Sotorasib), one of these covalent inhibitors, binds irreversibly with the allosteric binding pocket of KRASG12C, which mainly consists of switch II and α3-helix, termed as the switch II pocket (35). AMG510 has shown particularly promising anticancer activity in clinical trials for KRASG12C mutant patients and is the first approval drug for KRAS targeted therapy by FDA (36–39). Drug resistance has always been a great challenge to the clinical use of drugs (40, 41) and the clinical Y96D acquired resistance mutation to AMG510 has been observed (42, 43). Cell viability assays showed that relative to the KRASG12C expressing controls, cells expressing the double KRASG12C/Y96D mutation showed marked resistance to AMG510, with an IC50 value shifting >100-fold. The crystal structure of AMG510−KRASG12C complex implies that the Y96D mutation disrupts the water-mediated hydrogen bond between Y96 and the carboxyl group of AMG510.
However, the water-mediated hydrogen bond between Y96 and the carboxyl group of AMG510 in the crystal structure seems relatively weak, hard to explain the significant divergence of IC50 induced by the mutation. Furthermore, crystal structures are a spatiotemporal average of the protein structure with crystal-packing contacts, which may not provide enough information on the conformational transition of proteins. Molecular dynamics (MD) simulations that explore protein conformational dynamics at the atomic level can be helpful to investigate the resistant mechanism of Y96D mutation. MD simulations have been applied to investigate the conformational dynamics of RAS proteins (44, 45). In recent work, we have used MD simulations to directly uncover biomolecular mechanisms and protein–ligand/protein recognitions (46–54). In addition, although previous studies have demonstrated that Y96 plays a critical role in AMG510 binding (55), no studies have shown how Y96D mutation affects the conformation of the KRAS protein.
Here, we performed an extensive large-scale MD simulations of the KRASG12C−AMG510 and KRASG12C/Y96D−AMG510 complexes to investigate the underlying mechanism of the additional Y96D mutation-based resistance to AMG510. The simulation revealed that the Y96D mutation impaired the van der Waals interaction between residue 96 and AMG510 and yielded higher flexibility and more coupled motion of the switch II and α3-helix, which led to the departing movement of the switch II and α3-helix. The departing motion induced the opening and loosening of the binding pocket, disrupting the interactions of KRAS−AMG510. Moreover, the opening of the binding pocket caused an increased solvent exposure of AMG510, which may yield the instability of AMG510. Collectively, this study revealed the underlying mechanism of the Y96D resistance mutation, which will help to offer guidance for the design of KRAS targeted drugs.
Materials and methods
System preparation
Two systems were performed, including KRASG12C−AMG510 (denoted as “G12C”) and KRASG12C/Y96D−AMG510 (denoted as “G12C/Y96D”). The crystal structure of KRASG12C−AMG510 complex (PDB ID: 6OIM) (43) was selected as the initial structure. The missing residues of the KRAS protein were added using the MODELLER program. The Y96D mutation was conducted using the Discovery Studio 2019.
MD simulations
The force field parameter of AMG510 was generated using the antechamber program of Amber. The systems were prepared with the LEaP program using ff14SB force field to describe the AMG510−protein complexes (56). The prepared complex was solvated to a truncated octahedron transferable intermolecular potential three point (TIP3P) water box (57) and Na+ and Cl- were added to neutralize and simulate normal saline environment. Two rounds of minimization were performed. The first round contained 5000 steps maximum minimization cycles with the complex fixed and the second round contained 10,000 steps maximum minimization cycles with no constraints. Subsequently, systems were heated from 0 to 300 K within 300 ps, followed by 700 ps equilibration running in a canonical ensemble (NVT). After all these preparations, 3 replicas of independent 1 μs simulations were performed with random velocities under isothermal isobaric (NPT) conditions for each system. For the long-range electrostatic interactions, the particle mesh Ewald method (58) was used. Covalent bonds involving hydrogens were restrained using the SHAKE method (59).
Principal component analysis (PCA)
Principal component analysis (PCA), widely used in describing the kinetic process during simulation, is a technique that can transform a series of potentially coordinated observations into orthogonal vectors. Among these vectors, the first principal component (PC1) provides the dominant motions throughout simulation (60). PCs were generated based on coordinate covariance matrix of Cα atoms in the KRAS protein using every frame in all two systems and these collected frames were all projected on the PC1 and PC2.
Dynamic cross-correlation matrix (DCCM) analysis
The DCCM of all protein Cα atoms was calculated to reflect the inter-residue correlations. The cross-correlation coefficient Ci,j was calculated by:
where i and j represent the ith and jth Cα atoms, respectively.
Generalized correlation analysis
Generalized correlation (GCi,j) analysis was applied to calculate the correlated motion. Comparing with the traditional DCCM analysis, GCi,j analysis has the advantage of capturing the non-liner correlations by calculating the correlations independently on the relative orientation of the atomic fluctuations. To reflect how much information of one atom’s position is provided by another, Mutual Information (MI) was introduced and calculated by:
The right side of equation can be related to the more widely known measure of entropy which calculated by:
To calculate based on the correlation between pairs of atoms:
[xi], was further related to a more intuitive Pearson-like correlation coefficient GCi,j which can be calculated by:
where d represents the dimensionality of xi and xj, which is 3 in our study. GCi,j calculation was done by g_correlation tool in Gromacs 3.3 (61) with the coordinates of Cα atoms in each residue as input.
Dynamic network analysis
Dynamic network analysis was performed to reflect the motion connection using the Network View plugin in VMD (62). In our analysis, the Cα atoms of KRAS were selected as nodes to represent their corresponding residues. Edges were drawn between nodes whose distances are within a cutoff of 4.5 Å for at least 75% of simulation time. The edge between nodes was calculated using:
where i and j represent the two nodes. Additionally, community, which means a combination of residues whose connections are stronger was calculated using the Girvan-Newman algorithm (63) through the communities program of VMD.
Markov state model (MSM)
Markov state model (MSM) is a mathematical framework to describe the dynamics of time-series data (64). In this analysis, MSM was used to differentiate conformational states throughout simulation and help to extract representative structures from each cluster. All MSM calculations were performed using the PyEMMA software. MSM transition matrix was first calculated based on the probability of transition between different states. Implied timescale (ITS) test was performed to check the Markovian property and choose the proper lag time(τ). ITS as a function of τ can be calculated by:
where λi means the eigenvalue gotten from MSM transition matrix of the ith process. In implied timescale plot, each curve represents an average transition time in one process. When the curve becomes approximately constant, the corresponding lag time is appropriate for following analysis and the system is with Markovian property. In this study, the lag time was set as 1 ns.
Every frame of each system was projected on the free energy plot according to the characteristic vectors (CV). Then, K-means-algorithm was applied to cluster the two-dimensional conformations into 100 microstates in each system. Based on these 100 microstates, PCCA+ algorithm was performed to divide the microstates into different clusters. With the divided clusters, the Chapman–Kolmogorov test was computed to testify the property of MSM. The frames near the K-centers (microstates) belonging to the certain state in energy landscape were extracted to constitute a new trajectory to represent the state. The structure that has the smallest root-mean-square deviation (RMSD) with frames in the representative trajectories was chosen as the representative structure. For only the states in the energy basin of free-energy landscape were analyzed, the first cluster in the G12C system was not analyzed and the two clusters differentiated by MSM in the G12C/Y96D system were combined.
Results
Y96D mutation affects conformational dynamics of KRAS
To investigate the effect of mutation on overall conformational dynamics of KRAS protein, we calculated the Cα atoms root-mean-square deviation (RMSD) of the KRAS protein relative to the initial structure. The results indicated that both systems reached equilibrium after 100 ns of simulation (Supplementary Figure 1A). The RMSD values were 1.40 ± 0.16Å for the G12C system and 1.39 ± 0.10Å for the G12C/Y96D system. The similarity of the RMSD values between the two systems indicated the minor effect of the Y96D mutation on overall protein conformational dynamics.
To further uncover the influence of mutation on local conformational dynamics, the root-mean square fluctuation (RMSF) of each residue (represented by the Cα atom) was calculated (Supplementary Figure 1B). To better show the differences, we subtracted the RMSF value of the G12C/Y96D system from its counterpart in the G12C system (Figure 1A) and projected the values on the protein structure. Notably, the RMSF of the switch II and α3-helix in the G12C/Y96D system was higher than that in the G12C system (Figure 1B), suggesting that the Y96D mutation induced higher plasticity of the switch II and α3-helix. Because AMG510 binds to the switch II pocket that is mainly composed of the switch II and α3-helix, the increased plasticity of the switch II and α3-helix can be ascribed to their impaired interaction with AMG510 induced by the mutation. Additionally, the RMSF value of the switch I in the G12C/Y96D system was slightly lower than that in the G12C system, indicating that the Y96D mutation may stabilize the switch I region of KRAS.
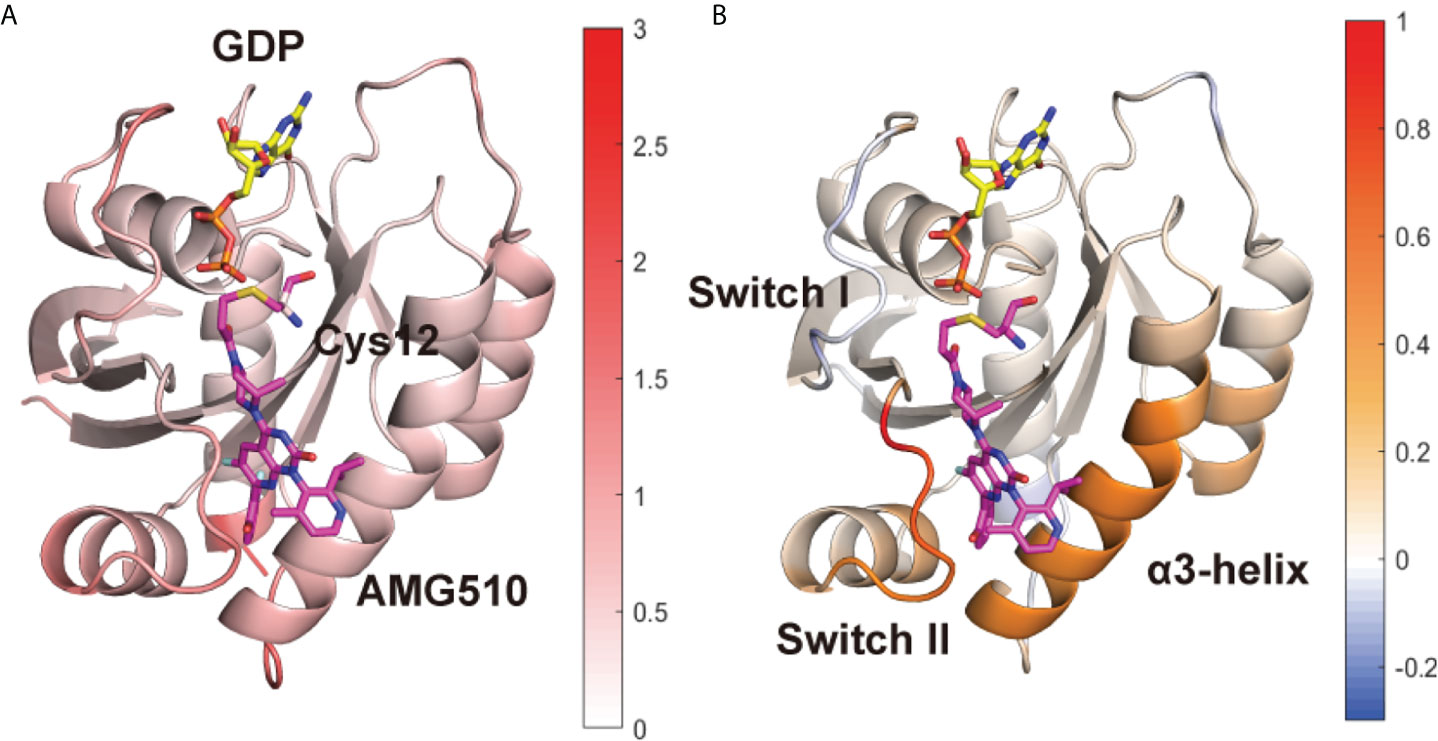
Figure 1 Conformational dynamics of KRAS protein. (A) The averaged RMSF values of each residue are plotted on the 3D structures of the KRAS protein in the G12C system. (B) The substation of RMSF values of the G12C/Y96D system from its counterpart in the G12C system. Positive regions (red) stand for higher RMSF values, whereas negative regions (blue) represent lower RMSF values.
Y96D mutation induces conformational transition of KRAS
To characterize the global conformational transition between the two systems, principal component analysis (PCA) was performed. We projected all MD snapshots from simulation of each system on the two-dimensional plot according to the first two principal components (PC1 and PC2) (Figure 2A) and the conformational motion along the PC1 was shown on the KRAS protein (Figure 2B). A significant increase of PC1 value was observed in the G12C/Y96D system compared with the G12C system. Along the PC1, the switch I moved towards the P-loop, and the switch II and α3-helix underwent the departing motion. Because the switch II and α3-helix constitute the most of the AMG510 binding pocket, this departing motion represented the opening of the binding pocket, reflecting the transition from the “closed” to “opening” motions.
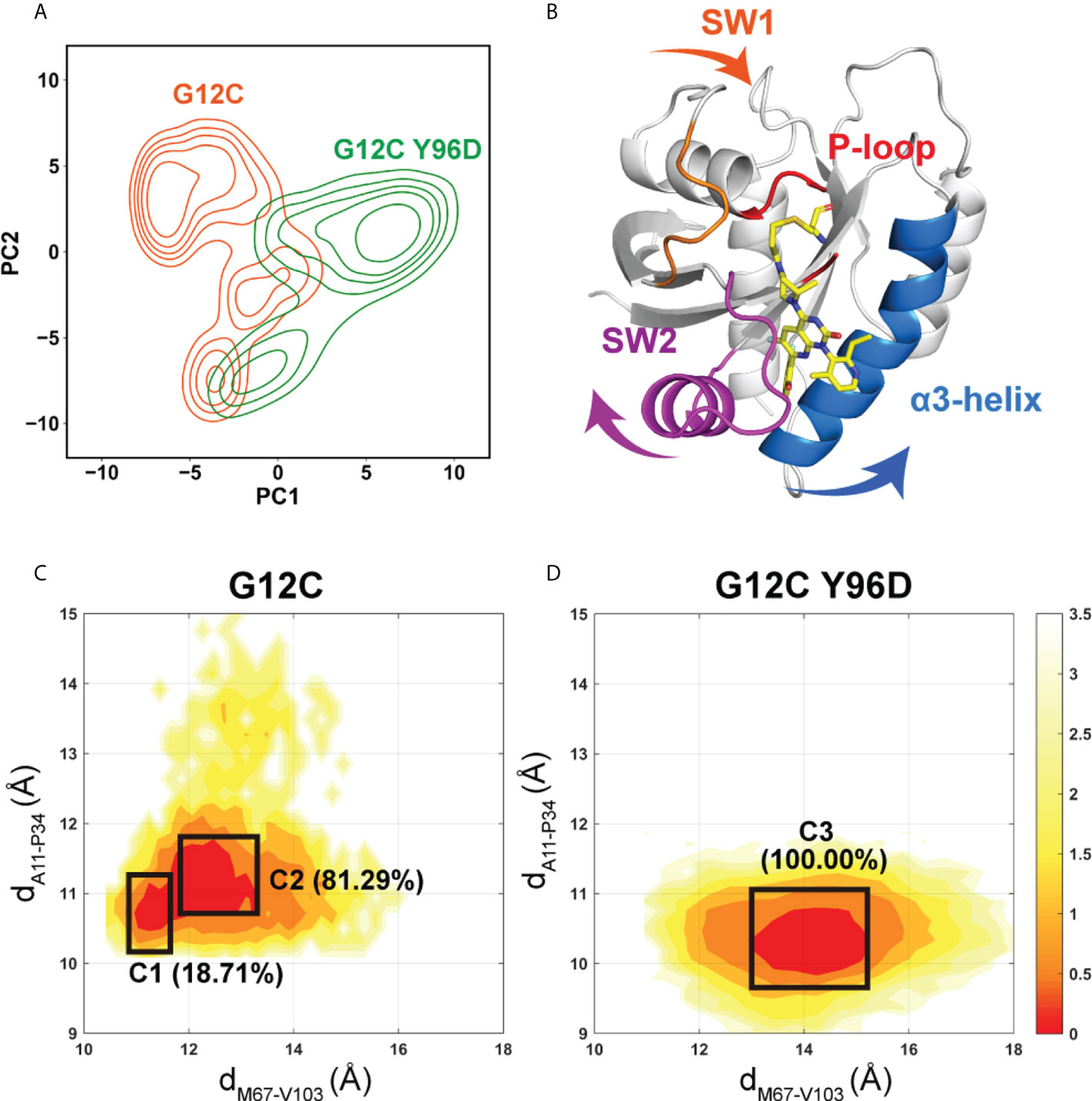
Figure 2 Global conformational transition of the KRAS protein. (A) Projections of the first and second principal components (PC1 vs PC2) from MD simulations of the G12C system (orange) and the G12C/Y96D system (green). (B) The motion along the PC1. Conformational landscapes generated using the dM67-V103 (distance from the M67 Cα atom to the V103 Cα atom) and dA11-P34 (distance from the A11 Cα atom to the P34 Cα atom) order parameters in the G12C system (C) and the G12C/Y96D system (D).
To further probe the conformational transition induced by the Y96D mutation, we projected all MD trajectories onto the two-dimensional surface according to the distances from the M67 Cα atom to the V103 Cα atom and from the A11 Cα atom to the P34 Cα atom (Figures 2C, D). DM67-V103 represents the distance between the switch II and α3-helix while dA11-P34 represents the distance between the switch I and P-loop (Supplementary Figure 2). In agreement with the motion along the PC1, the G12C/Y96D system had larger dM67-V103 and smaller dA11-P34 distances, indicating that the Y96D mutation induced the departure of the switch II and α3-helix and the approaching of the switch I to the P-loop. The range of dM67-V103 parameter was much broader in the G12C/Y96D system than that in the G12 system, which was in accordance with the increased plasticity of the switch II and α3-helix induced by the Y96D mutation shown in RMSF analysis. Similarly, the limited range of the dA11-P34 parameter in the G12C/Y96D system compared to the G12 system was consistent with the stabilization of the switch I induced by the Y96D mutation shown in RMSF analysis.
Markov state model (MSM) was used to differentiate the conformational states in the free-energy landscape, and only the states in the energy basin of free-energy landscape were analyzed. To testify the Markovian property, the implied timescale test and the Chapman−Kolmogorov test were applied (Supplementary Figure 3). The proportion, the representative trajectory, and the structure of each state were calculated based on the MSM. In the G12C system, with the increase of the dM67-V103 parameter, two states were observed: C1 and C2 (Figure 2C). The C2 state was the dominant state. In the G12C/Y96D system, only one state (C3) was observed (Figure 2D). In the G12C/Y96D system, the dM67-V103 parameter of the centroid of the C3 state in the free-energy landscape was larger than that of the C2 and C3 states in the G12C system. This indicated that the Y96D mutation could induce the departure of the switch II and α3-helix. To further illustrate the conformational transition, we superimposed the representative structures of the dominant state in the G12C system (C2) and the dominant state in the G12C/Y96D system (C3) (Figure 3). The departure of the switch II and α3-helix was observed and the motion was particularly obvious in the loop region of the switch II, the key region that interacts with AMG510. The surface representations of the representative structures showed that the direct interaction between the switch II and α3-helix was disrupted, which led to the opening of the binding pocket (Figures 4A, B).
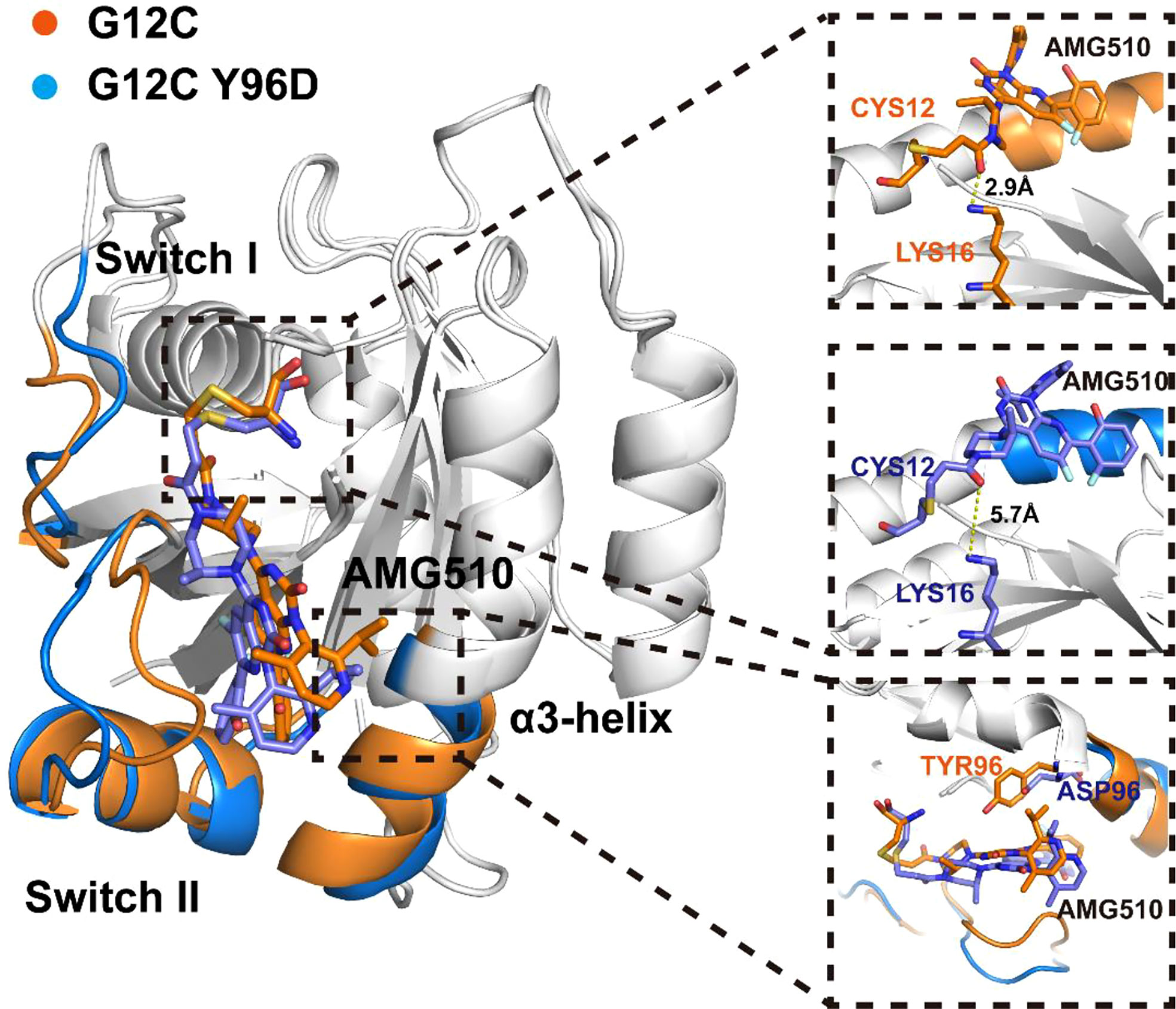
Figure 3 Superposition of the representative structures of the G12C system (orange) and the G12C/Y96D system (blue).
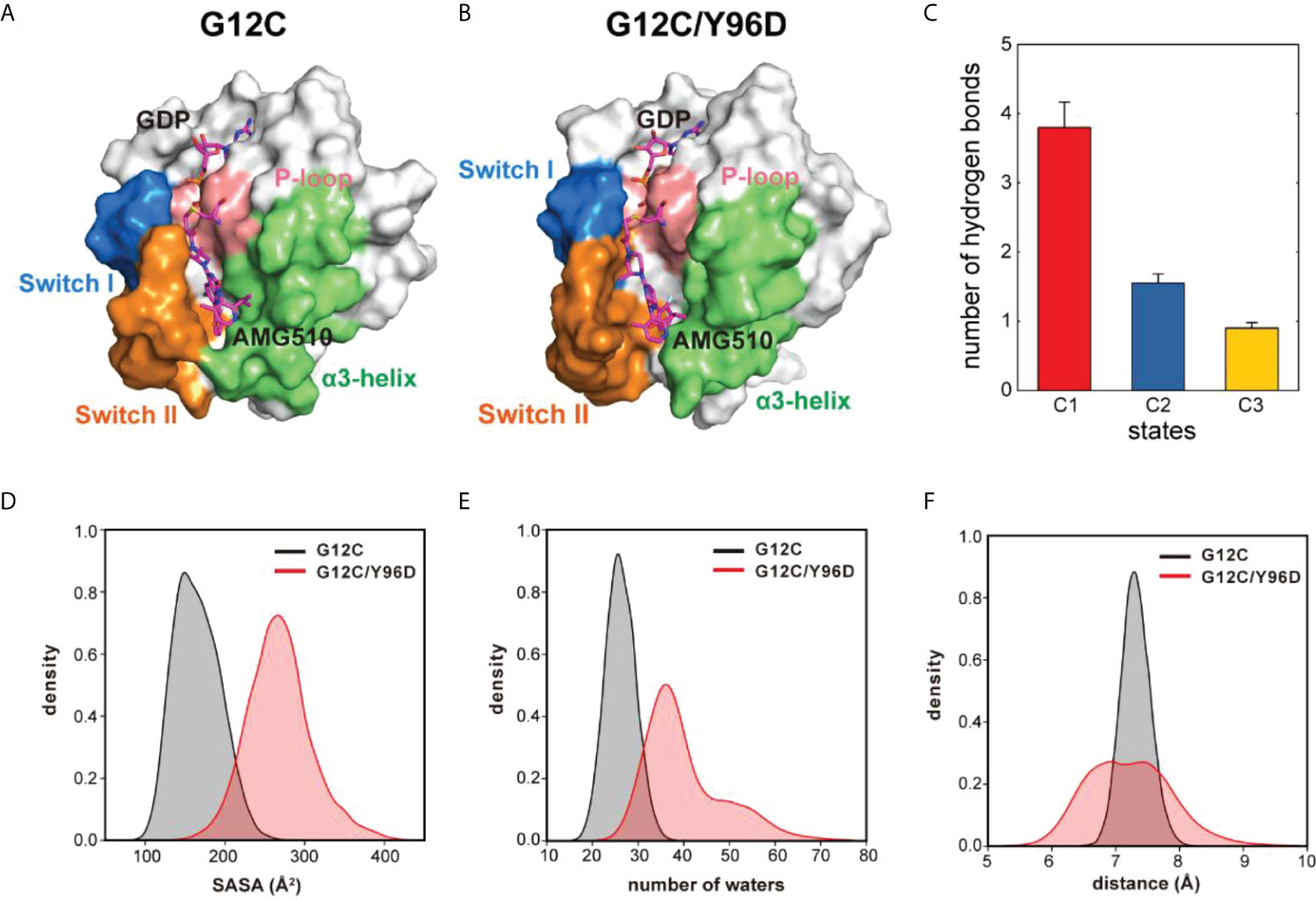
Figure 4 The Y96D mutation induced the opening and loosening of the binding pocket. (A) The surface representation of the representative structure of the G12C system. (B) The surface representation of the representative structure of the G12C/Y96D system. (C) The number of hydrogen bonds formed between the switch II and α3-helix in each state. (D) SASA of AMG510 in the G12C system (black) and the G12C/Y96D system (red). (E) Number of waters in the 5.4 Å watershell of AMG510 in the G12C system (black) and the G12C/Y96D system (red). (F) The distance between the CB atom of residue 96 and the centroid of azaquinozoline of AMG510 in the G12C system (black) and the G12C/Y96D system (red).
To further investigate the transition between the switch II and α3-helix, we calculated the hydrogen bonds formed between these two regions in the C1, C2 and C3 states (Figure 4C). The number of hydrogen bonds between the switch II and α3-helix decreased from the C1 to the C3, indicating that the departing motion of the switch II and α3-helix would impair the switch II and α3-helix interaction from the C1 to C3. The decreased hydrogen bonds occupancy between the switch II and α3-helix in the G12C/Y96D mutation further proved that the G12C/Y96D mutation could induce the departing motion of the switch II and α3-helix, which impaired the interaction between these two regions and led to the opening of the binding pocket (Supplementary Table 1). Taken together, the departure of the switch II and α3-helix induced by the Y96D mutation disrupted the interaction between these two regions and led to the loosening and opening of the binding pocket.
Y96D mutation induces the increased solvent exposure of AMG510
The solvent accessible surface area (SASA) of AMG510 was calculated to quantify the solvent exposure of AMG510. The SASA value in the G12C/Y96D system was significantly higher than that in the G12C system (Figure 4D), indicating a more loosened wrapping of binding pocket. Because SASA was limited to reflect the real solvent exposure, we calculated the number of waters in the solvent shell that wrapped AMG510 and the shell radius was set as 5.0 Å. With the Y96D mutation, the number of waters in the solvent shell increased (Figure 4E), suggesting an increased solvent exposure of AMG510 induced by the Y96D mutation. The extended range of the number of waters in the solvent shell in the G12C/Y96D system implied the instability of AMG510 binding pocket. The increased solvent exposure can be ascribed to the observation that the Y96D mutation promoted the departure of the switch II and α3-helix and induced the loosening and opening of binding pocket. The closed binding pocket could protect AMG510 from water solvent attack in the G12C system, while such protection was disturbed in the G12C/Y96D system, which led to the decreased binding affinity.
Y96D mutation impairs the interaction between KRAS and AMG510
Although AMG510 covalently binds to Cys12 of KRAS, other residues also play a significant role in the stable binding (65). One stable hydrogen bond was observed between Lys16 and the carbonyl oxygen of AMG510 whose occupancy was 83.76% in the G12C system while it was 7.10% in the G12C/Y96D system, indicating that the Y96D mutation disrupted the hydrogen bond between Lys16 and AMG510. The superimposition of representative structures of the two systems validated the disruption of the hydrogen bond in the G12C/Y96D system (Figure 3). Crystal structure shows water-bridged hydrogen bonds between Tyr96 and AMG510. The water-bridged hydrogen bonds were calculated to investigate the effect of the Y96D mutation on water bridges (Supplementary Table 2). The water-bridged hydrogen bond between AMG510 and Gln99 was the most stable water-bridged interaction between AMG510 and protein whose occupancy was 28.43% in the G12C system and 23.60% in the G12C/Y96D system. Interestingly, two additional water-bridged hydrogen bonds were strengthened in the Y96D mutation. One was formed with the mutated Asp96 and the other was formed with Lys16 which formed stable hydrogen bonds with AMG510 in the G12C system. Moreover, no conserved water bridges were observed for the highest occupancy of waters, implying that these water-bridged hydrogen bonds were delicate and may not the key interaction in keeping AMG510 binding.
To further investigate the interaction between AMG510 and KRAS, we calculated the linear interaction energy between key residues in the binding pocket and AMG510. The residues whose linear interaction energy having obvious variation between two systems were shown in Table 1 and the electrostatic energy contribution and van der Waals energy contribution were shown, respectively. The van der Waals energy contribution from the residue 96 significantly decreased in the G12C/Y96D mutant, indicating that the Y96D mutation disrupted the van der Waals interaction between residue 96 and AMG510. By superimposing the representative structures of the G12C and the G12C/Y96D systems, we observed that the phenylol group of Tyr96 pointed directly to AMG510 while the mutated Asp96 was distant from AMG510 to form direct interaction (Figure 3). The distance between the CB atom of residue 96 and the centroid of azaquinozoline of AMG510 was calculated to reflect the interaction between residue 96 and AMG510. The distance range was more limited in the G12C system than in the G12C/Y96D system, indicating a more stable interaction between residue 96 and AMG510 in the G12C system (Figure 4F). The linear interaction energy of other key residues also decreased to varied degrees in the G12C/Y96D system and these key residues are concentrated at the loop of the switch II and α3-helix, consistent with the conformational transition induced by the Y96D mutation that the departure of the switch II and α3-helix from AMG510.
Y96D mutation disturbs the correlated motions and community networks
The above analysis indicated the coordinated motions of the switch I, switch II, and α3-helix. To further investigate the correlated motion, the generalized correlation analysis and dynamic cross-correlation matrix (DCCM) analysis were applied. The absolute value of coefficients is proportional to the correlation. The generalized correlation indicated an increased correlated motion between the switch II and α3-helix in the G12C/Y96D system (Figures 5A, B). In the dynamic cross-correlation matrix, the negative coefficients represent the motions towards the opposite direction and the positive coefficients represent the motions towards the same direction. Consistent with the generalized correlation, the opposite motion between the switch II and α3-helix (A region) was strengthened due to the Y96D mutation (Figures 5C, D), suggesting that the mutation yielded more coupled opposite motion of the switch II and α3-helix which led to their departing movement. Moreover, the Y96D mutation weakened the opposite motion between the switch I and switch II (B region) (Figures 5C, D), which was in line with the switch I motion observed in PCA analysis.
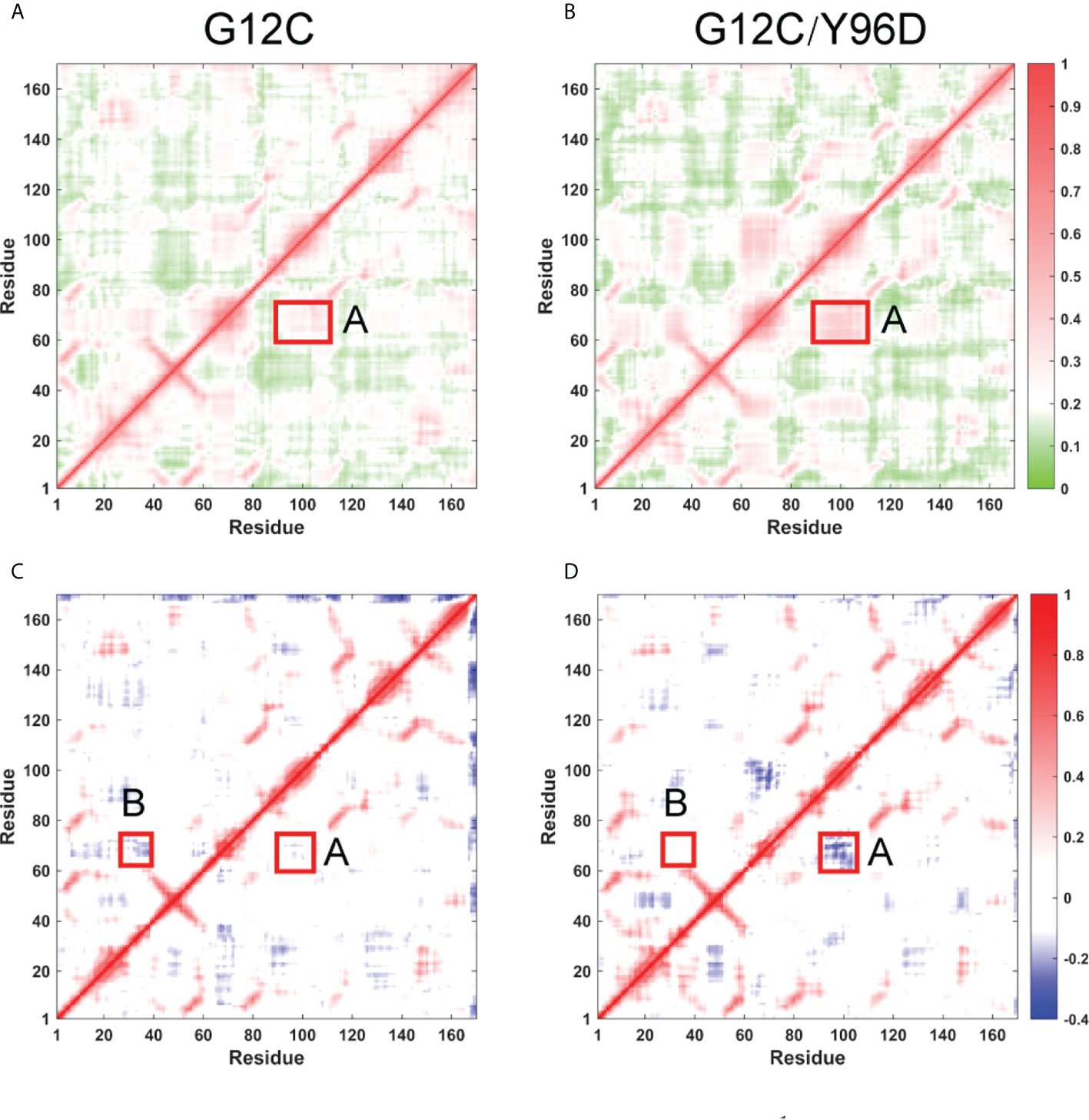
Figure 5 Inter-residue correlation of KRAS. Generallized residue correlation of the G12C system (A) and the G12C/Y96D system (B). DCCM plot of the G12C system (C) and the G12C/Y96D system (D). The significant correlation differences are highlighted with a rectangle in each panel. Regoin A represents the correlation between switch II and α3-helix while regoin B represents the correlation between switch I and switch II.
Community network analysis was performed to uncover the correlation network. Each community was showed as colored circles whose area is proportional to the number of residues it contains (Figures 6A, B). The intensity of inter-community connection was represented by the width of sticks connecting circles. In general, the Y96D mutation induced an obvious variation in community composition and connection. Switch II was mainly composed of Community F whose connection with Community D vanished in the G12C/Y96D system. For the cysteine 12 that covalently binds to AMG510 belonging to Community D, the vanished connection between Community D and Community F may suggest the damaged correlation between AMG510 and switch II. The optimal pathways that link Cys12 and Met67 (represents switch II) were calculated to prove the hypothesis. The shortest distance in the node map between Cys12 and Met67 in the G12C system is 244 Å, much shorter than that in the G12C/Y96D system (457 Å). Collectively, the community connection and the path length both reflected the impaired interaction between AMG510 and switch II. The connection between Community F (composed switch II) and Community G (composed α3-helix) also vanished in the G12C/Y96D system compared with the G12C system. This seemed to be in contradiction with the previous observation that the Y96D mutation yielded more coupled motion of the switch II and α3-helix. However, it should be noted that the Y96D mutation altered the composition of Community C that newly contained part of switch II (Q70, Y71 and T74) and α3-helix (V103) compared with the G12C system (Figures 6C, D). The coexistence of part of the switch II and α3-helix in the same community revealed a more correlated motion of the switch II and α3-helix in the G12C/Y96D system, consistent with previous observation. Overall, the Y96D mutation impaired the connection between the switch II and Cys12 that covalently binds to AMG510, and yielded more coupled motion of the switch II and α3-helix, which led to the departing movement of the switch II and α3-helix.
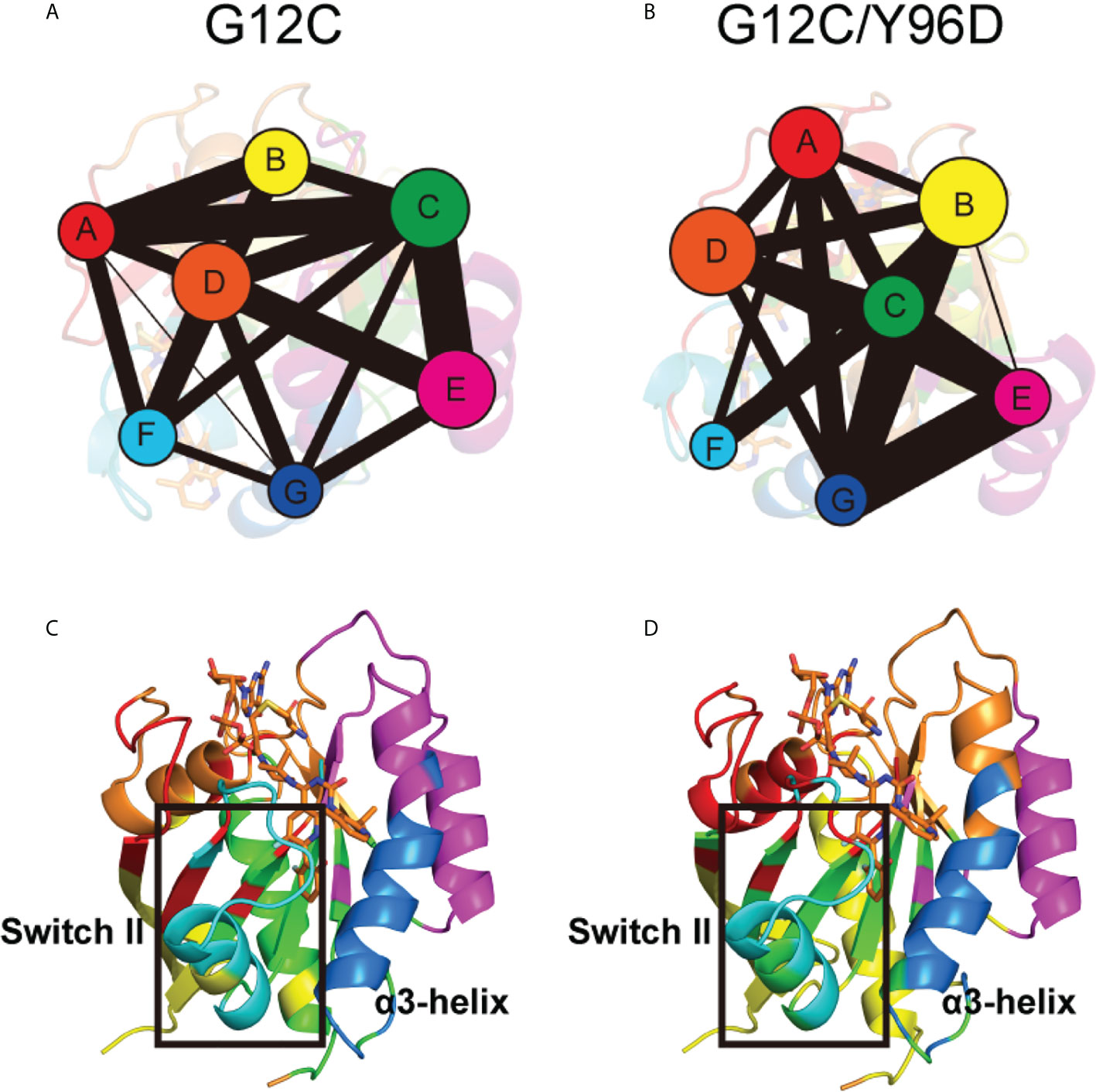
Figure 6 Community network of the KRAS protein. The community network in the G12C system (A) and the G12C/Y96D system (B). Areas of the circles represent the numbers of residues in corresponding communities, and the widths of sticks connecting communities represent the intercommunity connections. Community composition in the G12C system (C) and the G12C/Y96D system (D). The color of each community corresponds to (A, B).
Discussion
RAS proteins, as binary switches, control multiple intracellular signaling pathways. Specific oncogenic mutations disrupt the inactivation of RAS proteins and lead to the hyperactivation of downstream signaling pathways. Among them, mutations of KRAS account for 85% of observed RAS mutations in cancer and the mutation of residue 12 is one of the dominant mutations in KRAS (8). AMG510, the first drug for KRAS approved by FDA, covalently binds to Cys12 in the KRASG12C protein and has shown promising anti-cancer activity in clinical trials. The response that may induce drug resistance to these KRASG12C inhibitors can be divided into following categories, (i) mutations in KRAS, e.g., at G13, R68, H95, Y96, or multiple copies of KRASG12C; (ii) mutations in genes other than KRAS; (iii) Transitioning to other cancers, e.g., transitioning from adenocarcinomas to squamous cell carcinomas (66). Among them, the resistance caused by the acquired Y96D mutation severely affects the effectiveness of AMG510. Here, using MD simulations, we investigated the resistance mechanism to AMG510, thereby providing guidance for optimization and design of targeted KRAS drugs.
The Y96D mutation disrupted the direct interaction between residue 96 and AMG510. By calculating the linear interaction energy, we found that the Y96D mutation disrupted the van der Waals interaction between residue 96 and AMG510. In contrast, the electrostatic interaction between residue 96 and AMG510 even slightly strengthened with the Y96D mutation. The paradoxical change can be ascribed to the substitution from the phenolic hydroxyl (Tyr) to the carboxyl group with negative charge (Asp). Thus, rebuilding the interaction between Asp96 and AMG510 by forming new electrostatic interaction might be an effective strategy to overcome the resistance. By superimposing the representative structures in the G12C system and the G12C/Y96D system, we found that the isopropyl group of AMG510 in the G12C system and the G12C/Y96D system were close to the carboxyl group of the mutated Asp96 (Supplementary Figure 4), just right for hydrogen bonding, implying that the substitution from the isopropyl group to a polar group such as amino might be viable to form additional electrostatic interaction with Asp96. The distance calculation between residue 96 and AMG510 also indicated a more stable interaction between them in the G12C system compared with the G12C/Y96D system. By forming the new electrostatic interaction mentioned above, the instability of AMG510 induced by the Y96D mutation could also be recovered.
The previously reported crystal structure of the KRASG12C−AMG510 complex showed a bridged hydrogen bond mediated by two waters between Y96 and the carboxyl group of AMG 510. Tanaka et al. speculated that the Y96D mutation could impair the water-bridged hydrogen bond (43). However, the water-mediated hydrogen bond between residue 96 and AMG510 even strengthened in the simulation with the Y96D mutation. This can be ascribed to the disturbed interaction and the increased capacity to form hydrogen bond with the mutation from the phenolic hydroxyl (Tyr) to the carboxyl group with negative charge (Asp). Moreover, no conserved water bridges were observed for the waters participated in hydrogen bond bridge varied throughout simulation, indicating that these water-bridged hydrogen bonds were delicate and not the key interaction in keeping AMG510 binding. Such instability of water-bridged hydrogen bonds may be correlated with the mobility of solvation waters compared with crystal waters.
The Y96D mutation also affected the conformational dynamics and correlated motion of the KRAS protein. It is not surprising that the Y96D mutation changes the α3-helix’s dynamics because the Y96D mutation is located at the α3-helix. In addition, we found that the mutation affected the global dynamics of KRAS protein rather than only the α3-helix. The Y96D mutation yielded increased plasticity and more coupled motion of the switch II and α3-helix, which led to the departing movement of the switch II and α3-helix. The departing motion disrupted the interaction between the switch II and α3-helix and induced the opening and loosening of binding pocket. The “closed” to “opening” motion induced by the Y96D mutation further disrupted the interaction between the key residues in binding pocket and AMG510. Moreover, the opening of binding pocket caused an increased solvent exposure of AMG510, which may intensify the instability of AMG510. The communication between the switch II and α3-helix was also observed in other studies. These studies focused on the conformational changes in the switch II and α3-helix induced by mutations, which could impair the “activation” to “inactivation” conformational transition in GTPase circle of KRAS (67–69). Tanaka et al. found that KRASG12C/Y96D appeared to have higher basal activation than KRASG12C for a higher proportion of the active GTP-bound form of KRAS. From our research and other studies on the communication between the switch II and α3-helix, we can speculate that the increased activation of KRASG12C/Y96D was correlated with the coupled motion of the switch II and α3-helix. This is a possible direction in future research.
Research on the resistance to G12C covalent inhibitors identified many other acquired mutations in KRAS, which greatly affect the efficacy of KRASG12C targeted drugs, including Y96C, R68S and H95D/Q/R (70). Among them, H95D/Q/R mediated resistance to adagrasib (MRTX849) rather than sotorasib (AMG510) although His95 is part of the binding pocket of AMG510 (42). The linear interaction energy calculation in our study revealed that the contribution of His95 in AMG510 binding was very limited (Table 1), much less than key residues such as Tyr96.
Previously, Ras was considered as ‘‘undruggable” due to its picomolar affinities towards GDP/GTP in the orthosteric site and the lack of suitable binding pockets along the surface. Here, using fpocket software (71), we detected two new potential druggable pockets in the representative structures, which did not exist in the crystal structure. The two pockets can be detected in both the G12C system and the G12C/Y96D system with high druggability scores. The composition and location of these two pockets were shown in Supplementary Figure 5. This inspires future research aimed at the design of drugs targeting KRAS bound to these two new pockets.
Collectively, these results provided novel atomic-level insights to the resistance mechanism of the Y96D mutation to KRASG12C targeted drugs. First, they revealed the direct impairment of the Y96D mutation on the interaction between residue96 and AMG510. Second, they indicated that the Y96D mutation could promote the flexibility and the coupled motion of the switch II and α3-helix, which led to the loosening and opening of binding pocket. Finally, the coordinated motion of the switch II and α3-helix induced by the Y96D mutation further disrupted the interaction between key residues in the binding pocket and caused the increased solvent exposure of AMG510. These results elucidated the resistance mechanism on atomic level, which will help to offer guidance for the design of the next-generation of KRAS targeted drugs.
Data availability statement
The original contributions presented in the study are included in the article/Supplementary Material. Further inquiries can be directed to the corresponding authors.
Author contributions
Conceptualization, SL, YL and QW; methodology, HZhu and JF; validation, HZhu, JF, ML, HZha, XY and LL; formal analysis, HZhu and JF; investigation, HZhu and JF; writing—original draft preparation, HZhu and JF; writing—review and editing, SL and YL; visualization, HZhu, JF, ML, HZha, XY and LL; supervision, SL, YL and QW; project administration, SL, YL and QW; funding acquisition, XY All authors have read and agreed to the published version of the manuscript.
Funding
This study was partly supported by grants from the Zhiyuan Scholar Program (Grant No. ZIRC2021-11) and UM Distinguished Visiting Scholar to XY.
Conflict of interest
The authors declare that the research was conducted in the absence of any commercial or financial relationships that could be construed as a potential conflict of interest.
Publisher’s note
All claims expressed in this article are solely those of the authors and do not necessarily represent those of their affiliated organizations, or those of the publisher, the editors and the reviewers. Any product that may be evaluated in this article, or claim that may be made by its manufacturer, is not guaranteed or endorsed by the publisher.
Supplementary material
The Supplementary Material for this article can be found online at: https://www.frontiersin.org/articles/10.3389/fonc.2022.915512/full#supplementary-material
References
1. Sexton RE, Mpilla G, Kim S, Philip PA, Azmi AS. Ras and exosome signaling. Semin Cancer Biol (2019) 54:131–7. doi: 10.1016/j.semcancer.2019.02.004
2. Khan AQ, Kuttikrishnan S, Siveen KS, Prabhu KS, Shanmugakonar M, Al-Naemi HA, et al. Ras-mediated oncogenic signaling pathways in human malignancies. Semin Cancer Biol (2019) 54:1–13. doi: 10.1016/j.semcancer.2018.03.001
3. Scheffzek K, Shivalingaiah G. Ras-specific gtpase-activating proteins-structures, mechanisms, and interactions. Cold Spring Harb Perspect Med (2019) 9(3):a031500. doi: 10.1101/cshperspect.a031500
4. Wang CX, Wang TT, Zhang KD, Li MY, Shen QC, Lu SY, et al. Pan-kras inhibitors suppress proliferation through feedback regulation in pancreatic ductal adenocarcinoma. Acta Pharmacol Sin (2022). doi: 10.1038/s41401-022-00897-4
5. Nussinov R, Tsai CJ. 'Latent drivers' expand the cancer mutational landscape. Curr Opin Struct Biol (2015) 32:25–32. doi: 10.1016/j.sbi.2015.01.004
6. Prior IA, Lewis PD, Mattos C. A comprehensive survey of ras mutations in cancer. Cancer Res (2012) 72(10):2457–67. doi: 10.1158/0008-5472.CAN-11-2612
7. Simanshu DK, Nissley DV, McCormick F. Ras proteins and their regulators in human disease. Cell (2017) 170(1):17–33. doi: 10.1016/j.cell.2017.06.009
8. Prior IA, Hood FE, Hartley JL. The frequency of ras mutations in cancer. Cancer Res (2020) 80(14):2969–74. doi: 10.1158/0008-5472.CAN-19-3682
9. Lu S, Jang H, Nussinov R, Zhang J. The structural basis of oncogenic mutations G12, G13 and Q61 in small gtpase K-Ras4b. Sci Rep (2016) 6:21949. doi: 10.1038/srep21949
10. Mo SP, Coulson JM, Prior IA. Ras variant signalling. Biochem Soc Trans (2018) 46(5):1325–32. doi: 10.1042/BST20180173
11. Pantsar T. The current understanding of kras protein structure and dynamics. Comput Struct Biotechnol J (2020) 18:189–98. doi: 10.1016/j.csbj.2019.12.004
12. Lu S, Jang H, Muratcioglu S, Gursoy A, Keskin O, Nussinov R, et al. Ras conformational ensembles, allostery, and signaling. Chem Rev (2016) 116(11):6607–65. doi: 10.1021/acs.chemrev.5b00542
13. Lu S, Ni D, Wang C, He X, Lin H, Wang Z, et al. Deactivation pathway of ras gtpase underlies conformational substates as targets for drug design. ACS Catalys (2019) 9(8):7188–96. doi: 10.1021/acscatal.9b02556
14. Spoerner M, Hozsa C, Poetzl JA, Reiss K, Ganser P, Geyer M, et al. Conformational states of human rat sarcoma (Ras) protein complexed with its natural ligand gtp and their role for effector interaction and gtp hydrolysis. J Biol Chem (2010) 285(51):39768–78. doi: 10.1074/jbc.M110.145235
15. Lu J, Bera AK, Gondi S, Westover KD. Kras switch mutants D33e and A59g crystallize in the state 1 conformation. Biochemistry (2018) 57(3):324–33. doi: 10.1021/acs.biochem.7b00974
16. Cox AD, Fesik SW, Kimmelman AC, Luo J, Der CJ. Drugging the undruggable ras: Mission possible? Nat Rev Drug Discovery (2014) 13(11):828–51. doi: 10.1038/nrd4389
17. Qiu Y, Wang Y, Chai Z, Ni D, Li X, Pu J, et al. Targeting ras phosphorylation in cancer therapy: Mechanisms and modulators. Acta Pharm Sin B (2021) 11(11):3433–46. doi: 10.1016/j.apsb.2021.02.014
18. Nussinov R, Zhang M, Maloney R, Tsai CJ, Yavuz BR, Tuncbag N, et al. Mechanism of activation and the rewired network: New drug design concepts. Med Res Rev (2022) 42(2):770–99. doi: 10.1002/med.21863
19. Huang L, Guo Z, Wang F, Fu L. Kras mutation: From undruggable to druggable in cancer. Signal Transd Targ Ther (2021) 6(1):386. doi: 10.1038/s41392-021-00780-4
20. Fan J, Liu Y, Kong R, Ni D, Yu Z, Lu S, et al. Harnessing reversed allosteric communication: A novel strategy for allosteric drug discovery. J Med Chem (2021) 64(24):17728–43. doi: 10.1021/acs.jmedchem.1c01695
21. Lu S, Shen Q, Zhang J. Allosteric methods and their applications: Facilitating the discovery of allosteric drugs and the investigation of allosteric mechanisms. Acc Chem Res (2019) 52(2):492–500. doi: 10.1021/acs.accounts.8b00570
22. Zeng M, Lu J, Li L, Feru F, Quan C, Gero TW, et al. Potent and selective covalent quinazoline inhibitors of kras G12c. Cell Chem Biol (2017) 24(8):1005–16.e3. doi: 10.1016/j.chembiol.2017.06.017
23. Cagir A, Azmi AS. Kras inhibitors on the horizon. Future Med Chem (2019) 11(9):923–5. doi: 10.4155/fmc-2018-0304
24. Patricelli MP, Janes MR, Li LS, Hansen R, Peters U, Kessler LV, et al. Selective inhibition of oncogenic kras output with small molecules targeting the inactive state. Cancer Discovery (2016) 6(3):316–29. doi: 10.1158/2159-8290.CD-15-1105
25. Lu S, Zhang J. Designed covalent allosteric modulators: An emerging paradigm in drug discovery. Drug Discovery Today (2017) 22(2):447–53. doi: 10.1016/j.drudis.2016.11.013
26. Nussinov R, Tsai CJ. The design of covalent allosteric drugs. Annu Rev Pharmacol Toxicol (2015) 55:249–67. doi: 10.1146/annurev-pharmtox-010814-124401
27. Nussinov R, Tsai CJ. Allostery in disease and in drug discovery. Cell (2013) 153(2):293–305. doi: 10.1016/j.cell.2013.03.034
28. Moore AR, Rosenberg SC, McCormick F, Malek S. Ras-targeted therapies: Is the undruggable drugged? Nat Rev Drug Discovery (2020) 19(8):533–52. doi: 10.1038/s41573-020-0068-6
29. Kargbo RB. Inhibitors of G12c mutant ras proteins for the treatment of cancers. ACS Med Chem Lett (2019) 10(1):10–1. doi: 10.1021/acsmedchemlett.8b00576
30. Janes MR, Zhang J, Li LS, Hansen R, Peters U, Guo X, et al. Targeting kras mutant cancers with a covalent G12c-specific inhibitor. Cell (2018) 172(3):578–89.e17. doi: 10.1016/j.cell.2018.01.006
31. Fell JB, Fischer JP, Baer BR, Ballard J, Blake JF, Bouhana K, et al. Discovery of tetrahydropyridopyrimidines as irreversible covalent inhibitors of kras-G12c with in vivo activity. ACS Med Chem Lett (2018) 9(12):1230–4. doi: 10.1021/acsmedchemlett.8b00382
32. Westover KD, Janne PA, Gray NS. Progress on covalent inhibition of Kras(G12c). Cancer Discovery (2016) 6(3):233–4. doi: 10.1158/2159-8290.CD-16-0092
33. Shipman L. Anticancer drugs: Putting the brakes on kras-G12c nucleotide cycling. Nat Rev Drug Discovery (2016) 15(3):159. doi: 10.1038/nrd.2016.40
34. Lito P, Solomon M, Li L-S, Hansen R, Rosen N. Allele-specific inhibitors inactivate mutant kras G12c by a trapping mechanism. Science (2016) 351(6273):604–8. doi: 10.1126/science.aad6204
35. Ni D, Li X, He X, Zhang H, Zhang J, Lu S. Drugging K-Ras(G12c) through covalent inhibitors: Mission possible? Pharmacol Ther (2019) 202:1–17. doi: 10.1016/j.pharmthera.2019.06.007
36. Fakih MG, Kopetz S, Kuboki Y, Kim TW, Munster PN, Krauss JC, et al. Sotorasib for previously treated colorectal cancers with kras mutation (Codebreak100): A prespecified analysis of a single-arm, phase 2 trial. Lancet Oncol (2022) 23(1):115–24. doi: 10.1016/S1470-2045(21)00605-7
37. Skoulidis F, Li BT, Dy GK, Price TJ, Falchook GS, Wolf J, et al. Sotorasib for lung cancers with P.G12c mutation. N Engl J Med (2021) 384(25):2371–81. doi: 10.1056/NEJMoa2103695
38. Fakih M, O'Neil B, Price TJ, Falchook GS, Desai J, Kuo J, et al. Phase 1 study evaluating the safety, tolerability, pharmacokinetics (Pk), and efficacy of amg 510, a novel small molecule Krasg12c inhibitor, in advanced solid tumors. J Clin Oncol (2019) 37(15_suppl):3003. doi: 10.1200/JCO.2019.37.15_suppl.3003
39. Canon J, Rex K, Saiki AY, Mohr C, Cooke K, Bagal D, et al. The clinical Kras(G12c) inhibitor amg 510 drives anti-tumour immunity. Nature (2019) 575(7781):217–23. doi: 10.1038/s41586-019-1694-1
40. Ni D, Li Y, Qiu Y, Pu J, Lu S, Zhang J. Combining allosteric and orthosteric drugs to overcome drug resistance. Trends Pharmacol Sci (2020) 41(5):336–48. doi: 10.1016/j.tips.2020.02.001
41. Nussinov R, Tsai CJ, Jang H. A new view of pathway-driven drug resistance in tumor proliferation. Trends Pharmacol Sci (2017) 38(5):427–37. doi: 10.1016/j.tips.2017.02.001
42. Koga T, Suda K, Fujino T, Ohara S, Hamada A, Nishino M, et al. Kras secondary mutations that confer acquired resistance to kras G12c inhibitors, sotorasib and adagrasib, and overcoming strategies: Insights from in vitro experiments. J Thorac Oncol (2021) 16(8):1321–32. doi: 10.1016/j.jtho.2021.04.015
43. Tanaka N, Lin JJ, Li C, Ryan MB, Zhang J, Kiedrowski LA, et al. Clinical acquired resistance to Kras(G12c) inhibition through a novel kras switch-ii pocket mutation and polyclonal alterations converging on ras-mapk reactivation. Cancer Discovery (2021) 11(8):1913–22. doi: 10.1158/2159-8290.CD-21-0365
44. Lu S, Banerjee A, Jang H, Zhang J, Gaponenko V, Nussinov R. Gtp binding and oncogenic mutations may attenuate hypervariable region (Hvr)-catalytic domain interactions in small gtpase K-Ras4b, exposing the effector binding site. J Biol Chem (2015) 290(48):28887–900. doi: 10.1074/jbc.M115.664755
45. Jang H, Zhang M, Nussinov R. The quaternary assembly of Kras4b with raf-1 at the membrane. Comput Struct Biotechnol J (2020) 18:737–48. doi: 10.1016/j.csbj.2020.03.018
46. Wang Y, Ji D, Lei C, Chen Y, Qiu Y, Li X, et al. Mechanistic insights into the effect of phosphorylation on ras conformational dynamics and its interactions with cell signaling proteins. Comput Struct Biotechnol J (2021) 19:1184–99. doi: 10.1016/j.csbj.2021.01.044
47. Zhang Q, Chen Y, Ni D, Huang Z, Wei J, Feng L, et al. Targeting a cryptic allosteric site of Sirt6 with small-molecule inhibitors that inhibit the migration of pancreatic cancer cells. Acta Pharm Sin B (2021) 12(2):876–89. doi: 10.1016/j.apsb.2021.06.015
48. Lu S, He X, Yang Z, Chai Z, Zhou S, Wang J, et al. Activation pathway of a G protein-coupled receptor uncovers conformational intermediates as targets for allosteric drug design. Nat Commun (2021) 12(1):4721. doi: 10.1038/s41467-021-25020-9
49. Lu S, Chen Y, Wei J, Zhao M, Ni D, He X, et al. Mechanism of allosteric activation of Sirt6 revealed by the action of rationally designed activators. Acta Pharm Sin B (2021) 11(5):1355–61. doi: 10.1016/j.apsb.2020.09.010
50. Li X, Wang C, Peng T, Chai Z, Ni D, Liu Y, et al. Atomic-scale insights into allosteric inhibition and evolutional rescue mechanism of streptococcus thermophilus Cas9 by the anti-crispr protein Acriia6. Comput Struct Biotechnol J (2021) 19:6108–24. doi: 10.1016/j.csbj.2021.11.010
51. Zhang H, Zhu M, Li M, Ni D, Wang Y, Deng L, et al. Mechanistic insights into Co-administration of allosteric and orthosteric drugs to overcome drug-resistance in T315i bcr-Abl1. Front Pharmacol (2022) 13:862504. doi: 10.3389/fphar.2022.862504
52. Ni D, Wei J, He X, Rehman AU, Li X, Qiu Y, et al. Discovery of cryptic allosteric sites using reversed allosteric communication by a combined computational and experimental strategy. Chem Sci (2020) 12(1):464–76. doi: 10.1039/d0sc05131d
53. Li X, Dai J, Ni D, He X, Zhang H, Zhang J, et al. Insight into the mechanism of allosteric activation of Pi3kalpha by oncoprotein K-Ras4b. Int J Biol Macromol (2020) 144:643–55. doi: 10.1016/j.ijbiomac.2019.12.020
54. Ni D, Liu D, Zhang J, Lu S. Computational insights into the interactions between calmodulin and the C/Nsh2 domains of P85alpha regulatory subunit of Pi3kalpha: Implication for Pi3kalpha activation by calmodulin. Int J Mol Sci (2018) 19(1):151. doi: 10.3390/ijms19010151
55. Pantsar T. Kras(G12c)-amg 510 interaction dynamics revealed by all-atom molecular dynamics simulations. Sci Rep (2020) 10(1):11992. doi: 10.1038/s41598-020-68950-y
56. Maier JA, Martinez C, Kasavajhala K, Wickstrom L, Hauser KE, Simmerling C. Ff14sb: Improving the accuracy of protein side chain and backbone parameters from Ff99sb. J Chem Theory Comput (2015) 11(8):3696–713. doi: 10.1021/acs.jctc.5b00255
57. Jorgensen WL, Chandrasekhar J, Madura JD, Impey RW, Klein ML. Comparison of simple potential functions for simulating liquid water. J Chem Phys (1983) 79(2):926–35. doi: 10.1063/1.445869
58. York DM, Wlodawer A, Pedersen LG, Darden TA. Atomic-level accuracy in simulations of Large protein crystals. Proc Natl Acad Sci Unit States America (1994) 91(18):8715–8. doi: 10.1073/pnas.91.18.8715
59. Ali Hassan NZ, Mokhtar NM, Kok Sin T, Mohamed Rose I, Sagap I, Harun R, et al. Integrated analysis of copy number variation and genome-wide expression profiling in colorectal cancer tissues. PLoS One (2014) 9(4):e92553. doi: 10.1371/journal.pone.0092553
60. Daidone I, Amadei A. Essential dynamics: Foundation and applications. WIRE Comput Mol Sci (2012) 2(5):762–70. doi: 10.1002/wcms.1099
61. Lindahl E, Hess B, van der Spoel D. Gromacs 3.0: A package for molecular simulation and trajectory analysis. Mol Model Annu (2001) 7(8):306–17. doi: 10.1007/s008940100045
62. Eargle J, Luthey-Schulten Z. Networkview: 3d display and analysis of Protein·Rna interaction networks. Bioinformatics (2012) 28(22):3000–1. doi: 10.1093/bioinformatics/bts546
63. Girvan M, Newman MEJ. Community structure in social and biological networks. Proc Natl Acad Sci Unit States America (2002) 99(12):7821–6. doi: 10.1073/pnas.122653799
64. Husic BE, Pande VS. Markov State models: From an art to a science. J Am Chem Soc (2018) 140(7):2386–96. doi: 10.1021/jacs.7b12191
65. Statsyuk AV. Let K-ras activate its own inhibitor. Nat Struct Mol Biol (2018) 25(6):435–7. doi: 10.1038/s41594-018-0066-0
66. Nussinov R, Tsai CJ, Jang H. Anticancer drug resistance: An update and perspective. Drug Resist Update (2021) 59:100796. doi: 10.1016/j.drup.2021.100796
67. Sayyed-Ahmad A, Prakash P, Gorfe AA. Distinct dynamics and interaction patterns in h- and K-ras oncogenic p-loop mutants. Proteins (2017) 85(9):1618–32. doi: 10.1002/prot.25317
68. Vatansever S, Erman B, Gümüş ZH. Oncogenic G12d mutation alters local conformations and dynamics of K-ras. Sci Rep (2019) 9(1):11730. doi: 10.1038/s41598-019-48029-z
69. Pantsar T, Rissanen S, Dauch D, Laitinen T, Vattulainen I, Poso A. Assessment of mutation probabilities of kras G12 missense mutants and their long-timescale dynamics by atomistic molecular simulations and Markov state modeling. PLoS Comput Biol (2018) 14(9):e1006458. doi: 10.1371/journal.pcbi.1006458
70. Zhang J, Zhang J, Liu Q, Fan X-X, Leung EL-H, Yao X-J, et al. Resistance looms for kras G12c inhibitors and rational tackling strategies. Pharmacol Ther (2022) 229:108050. doi: 10.1016/j.pharmthera.2021.108050
Keywords: KRAS, G12C, molecular dynamics simulations, AMG510, drug resistance
Citation: Zhuang H, Fan J, Li M, Zhang H, Yang X, Lin L, Lu S, Wang Q and Liu Y (2022) Mechanistic insights into the clinical Y96D mutation with acquired resistance to AMG510 in the KRASG12C. Front. Oncol. 12:915512. doi: 10.3389/fonc.2022.915512
Received: 08 April 2022; Accepted: 18 July 2022;
Published: 10 August 2022.
Edited by:
Ran Su, Tianjin University, ChinaReviewed by:
Ruth Nussinov, National Cancer Institute (NIH), United StatesBrian Diskin, New York University, United States
Copyright © 2022 Zhuang, Fan, Li, Zhang, Yang, Lin, Lu, Wang and Liu. This is an open-access article distributed under the terms of the Creative Commons Attribution License (CC BY). The use, distribution or reproduction in other forums is permitted, provided the original author(s) and the copyright owner(s) are credited and that the original publication in this journal is cited, in accordance with accepted academic practice. No use, distribution or reproduction is permitted which does not comply with these terms.
*Correspondence: Shaoyong Lu, lushaoyong@sjtu.edu.cn; Qing Wang, jushi1984@163.com; Yaqin Liu, liuyaqing7811@126.com
†These authors have contributed equally to this work