- 1Department of Hepatobiliary Surgery, Renmin Hospital of Wuhan University, Wuhan, China
- 2Department of Oncology, Renmin Hospital of Wuhan University, Wuhan, China
Background: Hepatocellular carcinoma (HCC) has the highest cancer-related mortality rate. This study aims to create a nomogram to predict the cancer-specific survival (CSS) in patients with advanced hepatocellular carcinoma.
Methods: Patients diagnosed with advanced HCC (AJCC stage III and IV) during 1975 to 2018 were obtained from the Surveillance, Epidemiology, and End Results (SEER) database. Qualified patents were randomized into training cohort and validation cohort at a ratio of 7:3. The results of univariate and multivariate Cox regression analyses were used to construct the nomogram. Consistency index (C-index), area under the time-dependent receiver operating characteristic (ROC) curve [time-dependent area under the curve (AUC)], and calibration plots were used to identify and calibrate the nomogram. The net reclassification index (NRI), integrated discrimination improvement (IDI), and C-index, and decision curve analysis DCA were adopted to compare the nomogram’s clinical utility with the AJCC criteria.
Results: The 3,103 patients with advanced hepatocellular carcinoma were selected (the training cohort: 2,175 patients and the validation cohort: 928 patients). The C-index in both training cohort and validation cohort were greater than 0.7. The AUC for ROC in the training cohort was 0.781, 0.771, and 0.791 at 1, 2, and 3 years CSS, respectively. Calibration plots showed good consistency between actual observations and the 1-, 2-, and 3-year CSS predicted by the nomogram. The 1-, 2-, and 3-year NRI were 0.77, 0.46, and 0.48, respectively. The 1-, 2-, and 3-year IDI values were 0.16, 0.15, and 0.12 (P < 0.001), respectively. DCA curves in both the training and validation cohorts demonstrated that the nomogram showed better predicted 1-, 2-, and 3-year CSS probabilities than AJCC criteria.
Conclusions: This study established a practical nomogram for predicting CSS in patients with advanced HCC and a risk stratification system that provided an applicable tool for clinical management.
Introduction
Hepatocellular carcinoma (HCC) is the most common cause of cancer-related death and its incidence rate is increasing (1, 2). According to statistics, HCC accounts for 70–80% of the total burdens of liver disease (3, 4). Although diagnostic techniques for HCC have improved, only 20–35% of patients are diagnosed at an early stage (5), which meant approximately 80% of patients are detected at advanced stage (6). Extensive research results have reported that 5-year survival rate for patients with early-stage HCC can exceed 60% after treatment with tumor resection or liver transplantation (7, 8). Unfortunately, patients with advanced HCC (AJCC Stage III and IV) have been lost the opportunity of surgery, and the 5-year survival rate is only 10% after chemotherapy, radiotherapy, or other local treatment (9, 10). The low rate of early diagnosis and poor prognosis in advanced stage highlight the role of personalized treatment for patients with advanced HCC.
The prognostic models for early-stage HCC have been constructed and validated in several studies (11, 12). However, there is no predictive model for patients with advanced HCC. In the recent years, clinical models based on nomogram have been applied widely for survival prediction of oncology patients due to its advantages of intuitiveness and simplicity (13–15). Such new models can not only effectively promote personalized medicine, but also facilitate clinicians to utilize them for prognosis prediction. In this study, the purpose was to establish a nomogram with new risk stratification system for predicting the prognosis for patients with advanced HCC based on Surveillance, Epidemiology, and End Results (SEER) database.
Methods
Material
Patients enrolled in this study were extracted from the SEER18 registry database (1975–2018) by SEER*Stat 8.3.9.2 software for clinical-related data (including baseline demographics, tumor characteristics, therapeutic method, stage at diagnosis, survival status, and survival time) for patients diagnosed with HCC (AJCC Stage III and IV). The SEER database was publicly available and the private data of all patients have been eliminated from the SEER database. Therefore, informed consent and institutional review board approval were not required. The authorization account number for this study was 18419-Nov2020.
Variables
Fifteen variables were included in our study (age, gender, race, tumor size, tumor number, AJCC stage, bone metastasis, lymph node metastasis, lung metastasis, treatment, radiation therapy, chemotherapy, marital status, survival months, and survival status). In addition, we adopted the 7th edition AJCC TNM stage system. The inclusion criteria were as follows: (1) diagnosed as advanced HCC; (2) primary tumor location was in the liver; (3) known cause of death; (4) complete treatment information. And the exclusion criteria were as follows: (1) early stage HCC or metastatic liver cancer or other cancers; (2) incomplete information of treatment; (3) death caused by other cancers; and (4) unknown cause of death. The swipe selection process is shown in the flow chart (Figure 1).
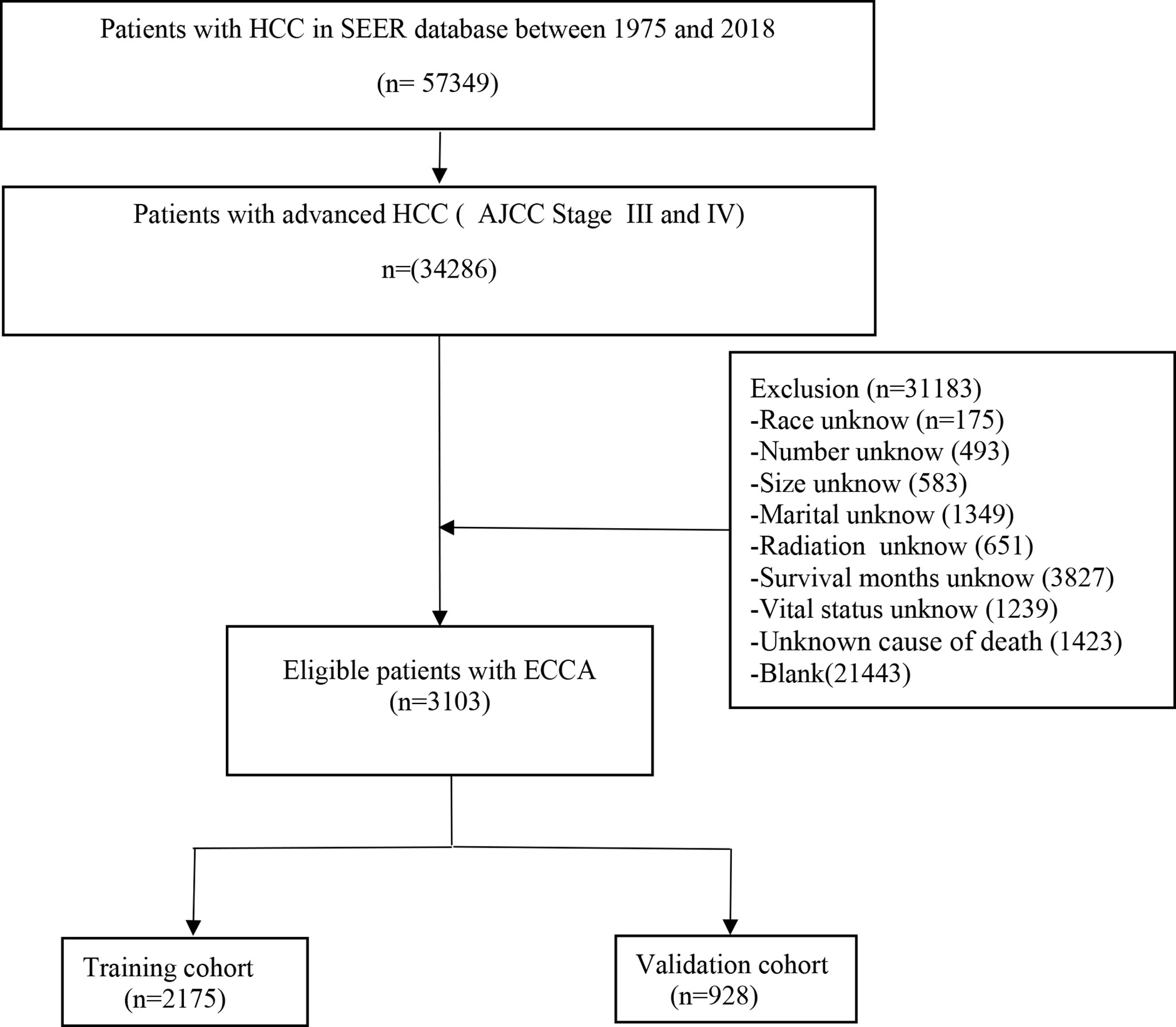
Figure 1 Flow diagram of the advanced hepatocellular carcinoma patients with training and validation cohorts.
Construction and Validation of the Nomogram Model
All patients were randomly divided into two groups at a ratio of 7:3. The training cohort was applied to create the nomogram and the validation cohort was performed for validation. Significant factors (P < 0.05), obtaining from univariate and multivariate Cox regression, were performed to construct the nomogram. The consistency index (C-index) and the time-dependent area under the curve (AUC) were calculated by bootstrapping to evaluate discriminative ability. The values of C-index and AUC ranged from 0.5 to 1.0 and were generally divided to low precision (0.5–0.7), moderate precision (0.71–0.90), and high precision (>0.9). The 1-, 2-, and 3-year calibration plots were plotted (1,000 self-help weight samples) to compare the predicted cancer-specific survival (CSS) with that observed in our study, and the 45-degree line was presented as the ideal prediction. DCAs were drawn to estimate the clinical practicality of the nomogram. New risk stratification, which divided patients into low-, middle-, and high-risk groups, was established by X-Tile software basing on the best cutoff value of risk score. Kaplan–Meier curves and log-rank tests were performed to compare the differences of CSS among patients in different risk stratification groups. The C-index, net reclassification index (NRI), integrated discrimination improvement (IDI), and decision curve analysis (DCA) were adopted to evaluate the improvement in predictive capability and effectiveness of the new model.
Statistical Analysis
SEER*Stat software (version 8.3.9.2) was applied to extract the data and the best cutoff value for the total score were select by X-Tile (version 3.6.1). All data analyses were performed using R software version 4.1.2 (http://www.r-project.org/). The R packages “regplot”, “mstate”, “survival”, “cmprsk”, “hmisc”, “timeROC”, “foreign”, “nricens”, “rmda”, and “DCA” were used to develop and validate the nomogram. All P values resulted from the use of two-sided statistical testing. It was statistically significant when P value was less than 0.05.
Results
Patient Demographic and Clinical Characteristics
The 3,103 patients were qualified with advanced HCC (AJCC Stage III and IV) and randomized into training cohort (2,175) and validation cohort (928). The median follow-up and the interquartile range (IQR) for the whole population, the training cohort and the validation cohort were 4 months and 1–12 months, respectively. The demographic and clinical characteristics of patients with advanced HCC were presented in Table 1. The 1,385 patients enrolled in the study received chemotherapy and 482 patients were treated with radiotherapy. In summary, there was no statistical difference between the training cohort and validation cohort in demographic and clinical characteristics (P > 0.05).
Univariate and Multivariate Cox Regression Analysis
The outcome of univariate Cox regression analysis of the training cohort revealed that age, gender, race, tumor size, tumor number, AJCC stage, bone metastasis, lymph node metastasis, lung metastasis, treatment, radiotherapy, chemotherapy, and marital status were prognostic factors for patients with advanced HCC (P < 0.05). Age, AJCC stage, lymph node status, tumor number, bone metastasis, lung metastasis, surgery, radiotherapy, chemotherapy, and marital status were independent prognostic factors for patients with advanced HCC (P < 0.05) identified in multivariate Cox regression analysis and were included in construction of the nomogram (Table 2).
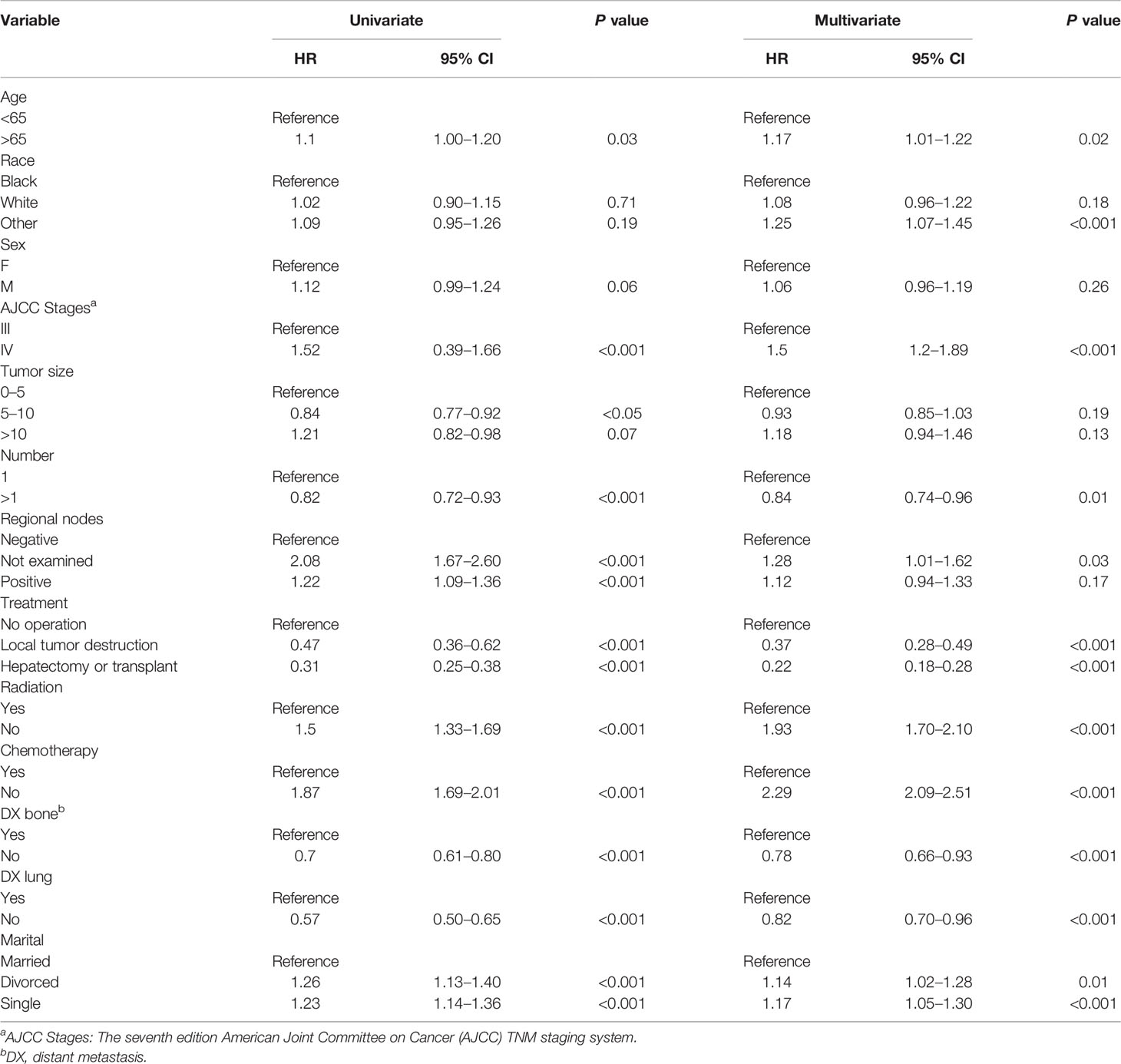
Table 2 The results of univariate and multivariate Cox regression analyses on variables for the prediction of CSS of advanced hepatocellular carcinoma patients.
Development and Validation of Nomogram
Finally, ten variables (age, AJCC stage, lymph node metastasis, number of tumors, bone metastases, lung metastases, treatment, radiotherapy, chemotherapy, and marriage) were selected to construct the nomogram for predicting the 1-, 2-, and 3-year CSS in patients with advanced HCC (Figure 2). To predict the CSS for patients with advanced HCC, the score in each row of variables was found and the total score of all variables were calculated. Then located the corresponding score in the total score of the row and the 1-, 2-, and 3-year probability of CSS could be inferred by drawing a straight line on the last three rows.
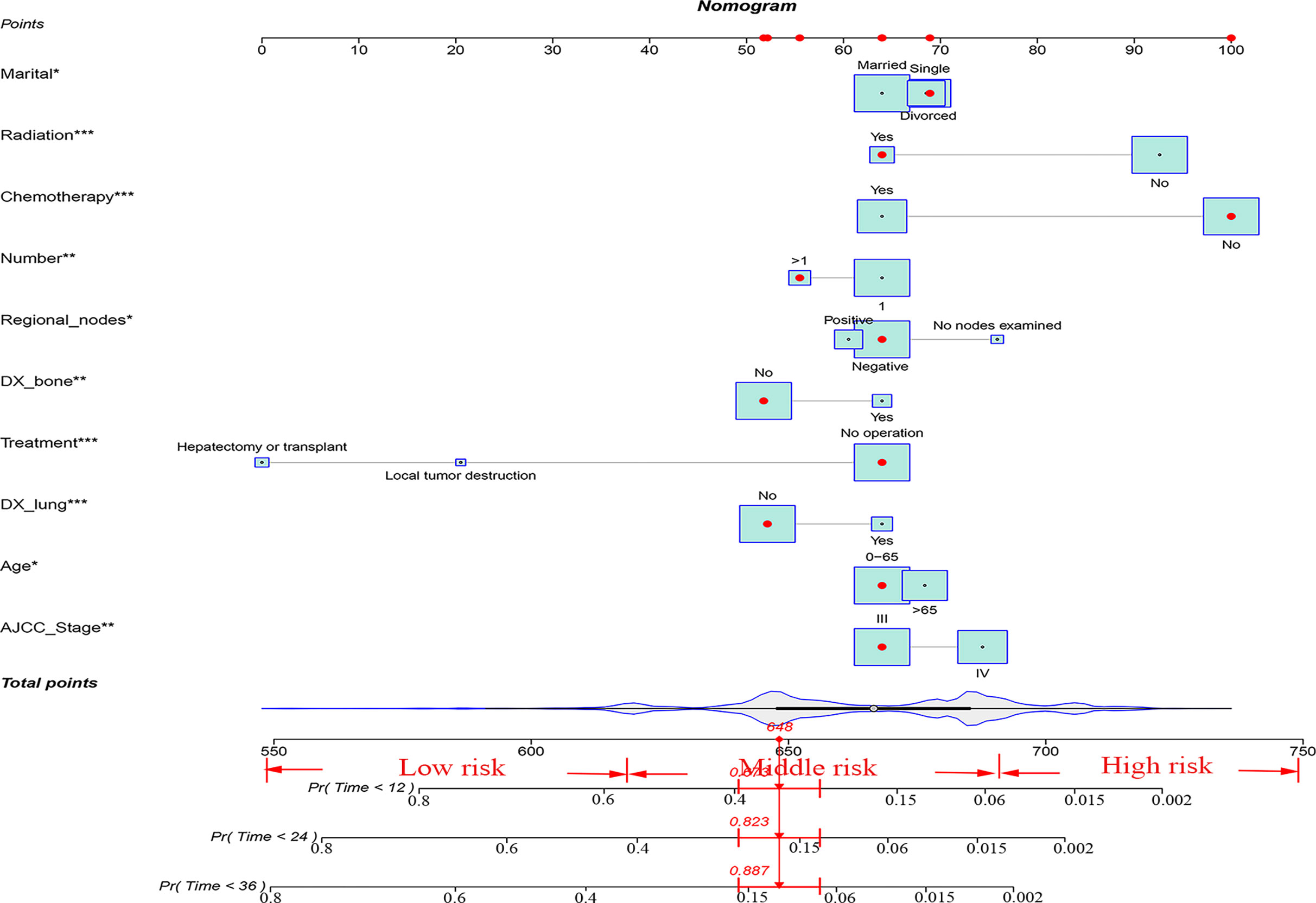
Figure 2 A nomogram for advanced hepatocellular carcinoma patients. *P < 0.05; **P < 0.01; ***P < 0.001.
The C-indexes for the training and validation cohorts were 0.734 (95% CI: 0.726–0.743) and 0.732 (95% CI: 0.726–0.744), respectively. The receiver operating characteristic (ROC) curves, calibration curves, and DCA curves were shown in Figures 3–5. The analysis of the ROC curve indicated the outstanding predictive performance of the nomogram (1-, 2-, and 3-year AUC for the training cohort were 0.781, 0.771, and 0.779; and 1-, 2-, and 3-year AUC for the validation cohort were 0.812, 0.816, and 0.818). In addition, the nomogram-related DCA curves at 1, 2, and 3 years in both the training and validation cohorts revealed outstanding promising clinical application and good positive net benefit. The calibration curves all displayed a high consistency between the predicted CSS rates at 1, 2, and 3 years and the observed results.
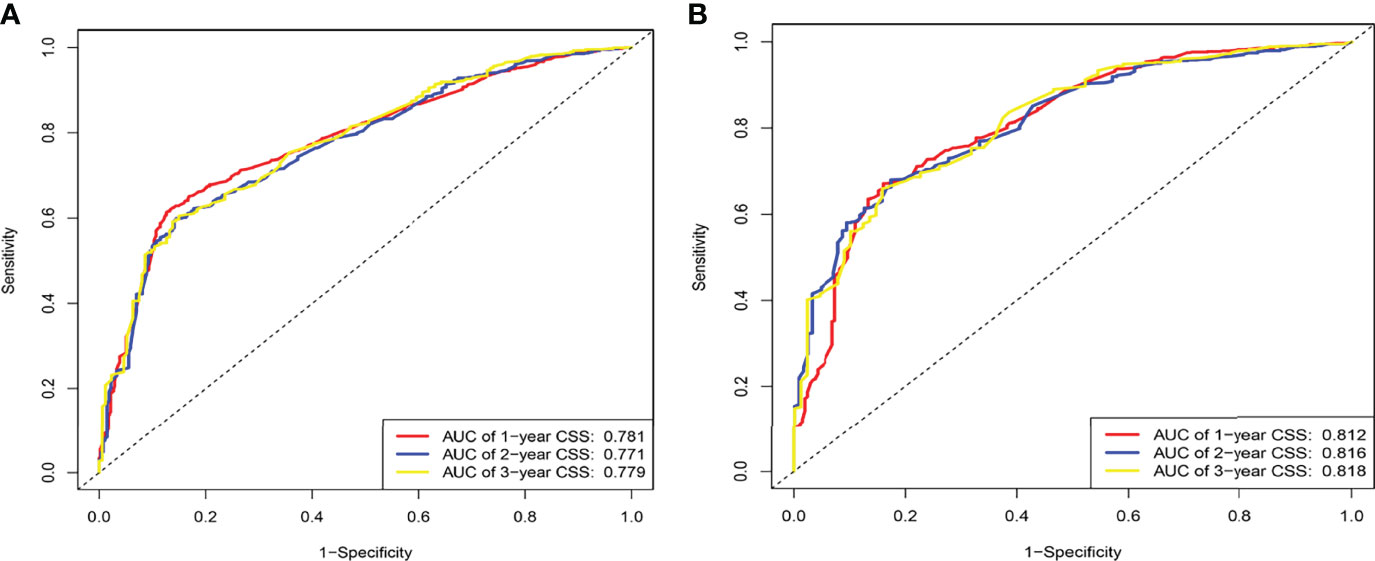
Figure 3 ROC of the nomogram for 1-, 2-, and 3-year prediction. (A) Training cohorts based on the nomogram. (B) Validation cohorts based on the nomogram.
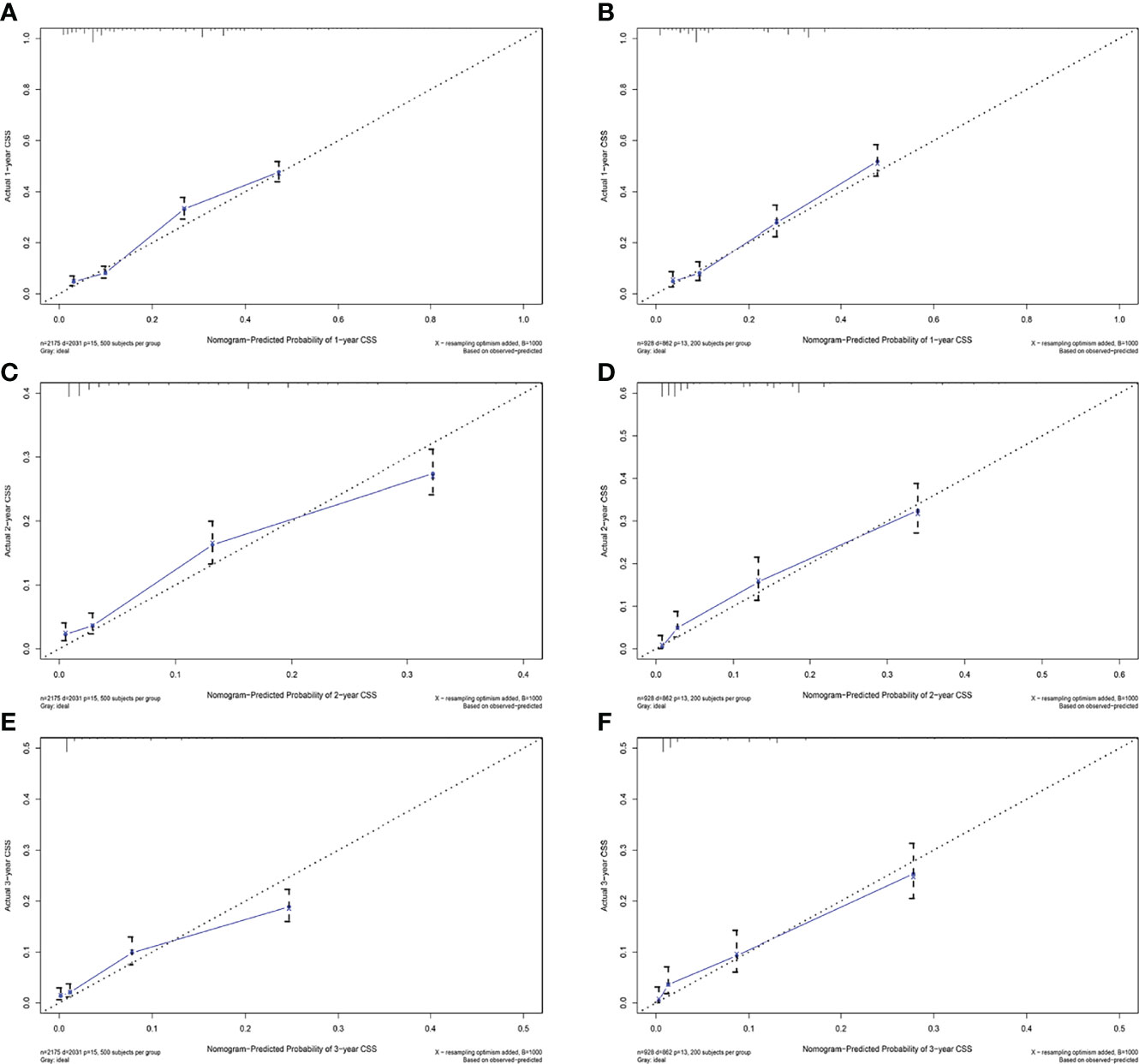
Figure 4 Calibration plots of 1-year, 2-year, and 3-year CSS for advanced hepatocellular carcinoma patients. (A, C, E) Calibration plots of 1-year, 2-year, and 3-year CSS in the training cohort. (B, D, F) Calibration plots of 1-year, 2-year, and 3-year CSS in the validation cohort.
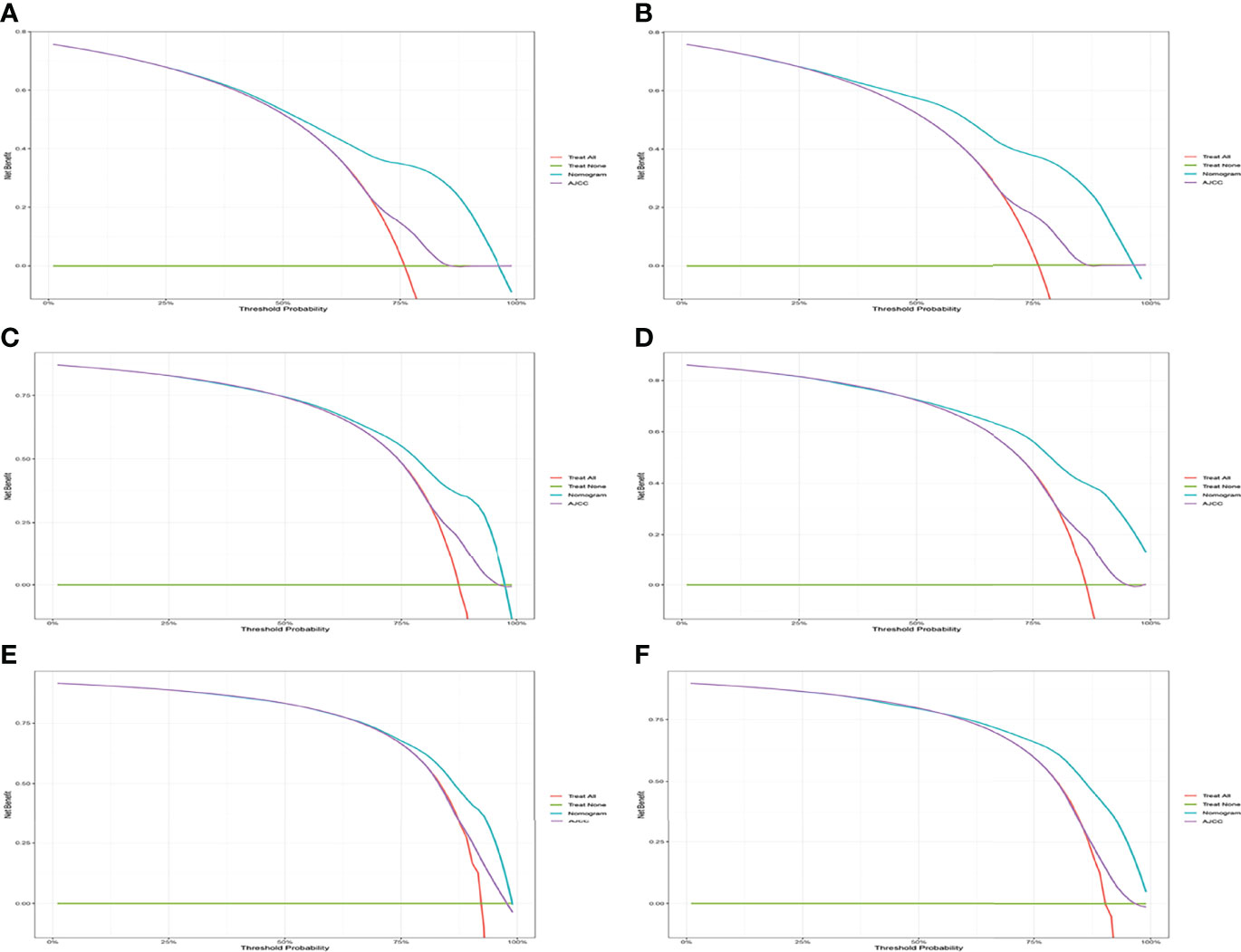
Figure 5 Decision curve analysis of CSS-associated nomogram and AJCC criteria. (A, C, E) DCA curves of 1-year, 2-year, and 3-year CSS in the training cohort. (B, D, F) DCA curves of 1-year, 2-year, and 3-year CSS in the validation cohort.
A comparison of the applied values of the nomogram and AJCC criteria with C-index, NRI, and IDI was performed. In the training cohort, the nomogram-related C-index was higher than that of the AJCC criteria (Figure 6). The 1-, 2-, and 3-year NRI were 0.77 (95% CI = 0.65–0.86), 0.46 (95% CI = 0.0.37–0.58), and 0.48 (95% CI = 0.35–0.61), respectively. The IDI values at 1, 2, and 3 years were 0.16 (95% CI 0.13–0.18, P < 0.001), 0.15 (95% CI 0.12–0.17, P < 0.001), and 0.12 (95% CI 0.09–0.16, P < 0.001; Table 3). The above results were strong enough to argue that the nomogram had a superior value of application and improved predictive capability than the AJCC stage system. In addition, the clinical benefits of columnar maps were evaluated, which was compared with those of the AJCC criteria. DCA curves in both the training and validation cohorts demonstrated that the nomogram showed better prediction for the 1-, 2-, and 3-year CSS probabilities because it produced a greater net benefit compared to the AJCC criteria and with both the treat-all-patients scheme and the treat-none scheme.
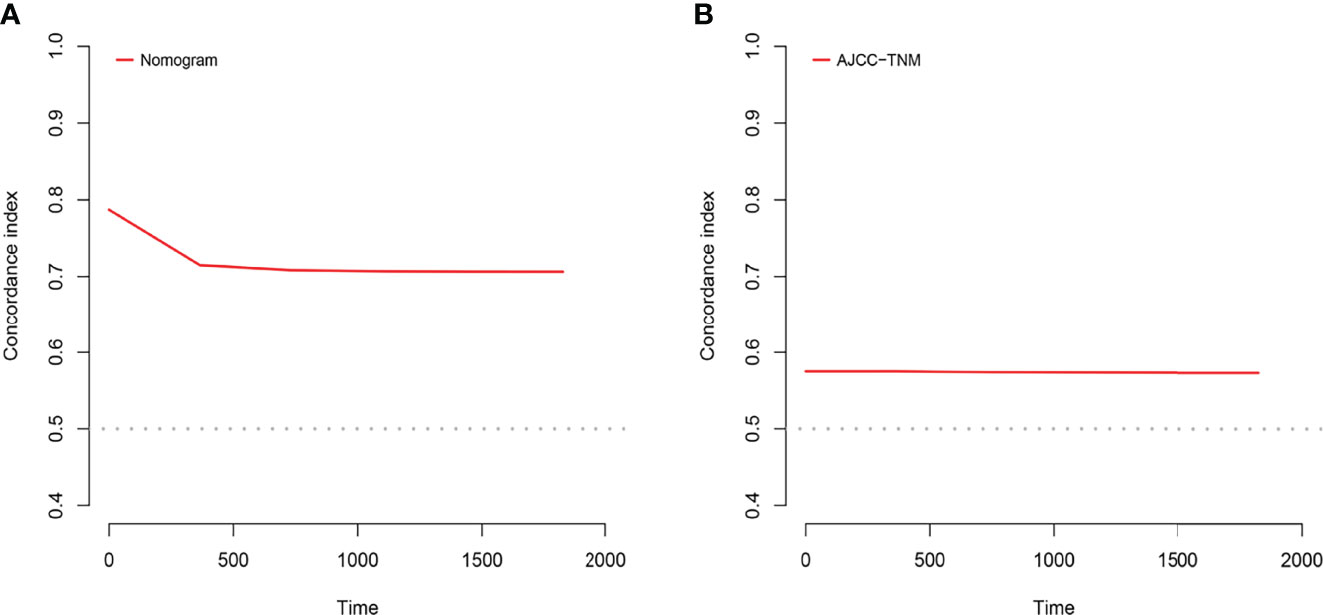
Figure 6 C-index analysis. (A) The nomogram related C-index. (B) AJCC staging criteria related C-index.
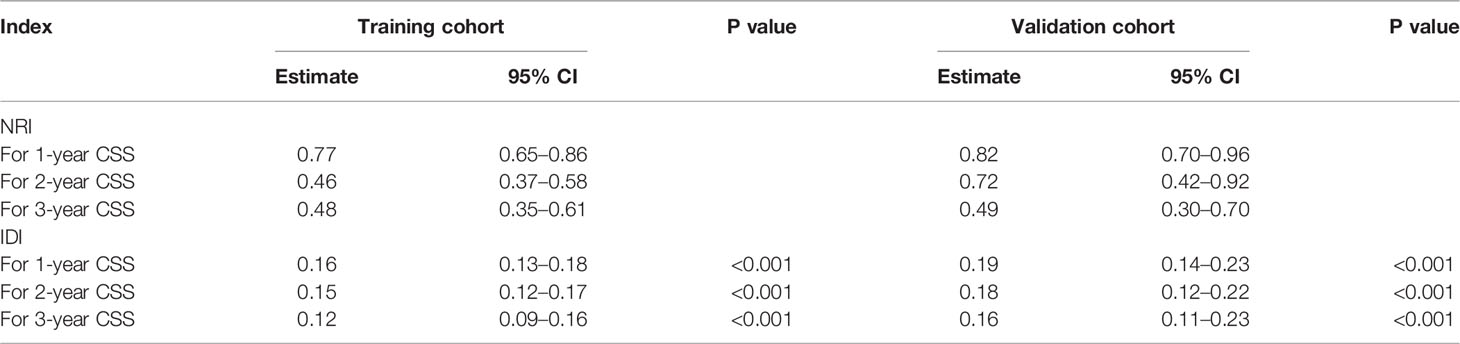
Table 3 NRI and IDI of the nomogram and AJCC staging criteria alone in CSS prediction for advanced hepatocellular carcinoma.
New Risk Stratification
Finally, risk stratification was performed by calculated with the nomogram. Patients with advanced HCC were divided into three risk groups low risk (total points < 638), middle risk (638 ≤ total points < 677) and high risk (total points ≥ 677; Figure 7). Kaplan–Meier curves exhibited a significant discriminatory in the three risk groups. In contrast, the AJCC criteria has shown limited ability to identify low-risk and high-risk patients in both the training cohort and validation cohort (Figure 8).
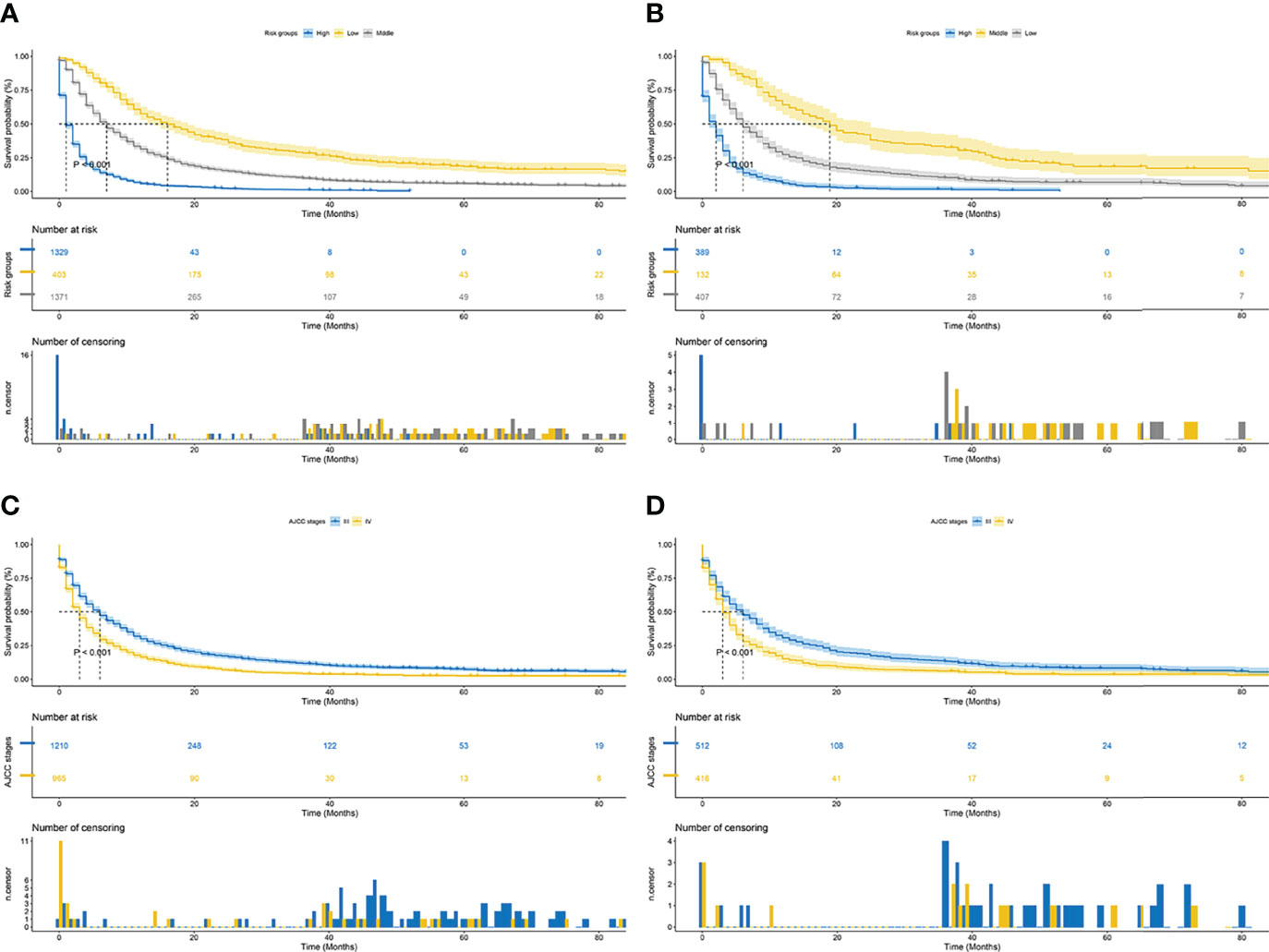
Figure 8 Kaplan–Meier CSS curves of patients with advanced hepatocellular carcinoma based on different criteria. (A, B) Kaplan–Meier CSS curves of training and validation cohorts based on the new risk stratification system. (C, D) Kaplan–Meier CSS curves of training and validation cohorts based on AJCC staging criteria.
Discussion
The prognosis of advanced HCC is extremely frustrating. Simultaneously, clinical prognostic models based on large cohorts are not available. Therefore, we established and validated a nomogram for predicting the prognosis of patients with advanced HCC by analyzing the data of patients obtained from the SEER database. Results of validations indicated that the nomogram had excellent predictive and discriminatory ability. Based on the nomogram, we developed a new risk stratification system for patients with advanced HCC by calculating the total score of patients (using X-tile software to select the cutoff value of the best grouping). This system divided all patients into low-, middle-, and high-risk groups. Compared with the AJCC criteria, this risk stratification has an outstanding ability to distinguish different risk groups. In addition, the system not only accurately predicts the prognosis of patients with advanced HCC, but also functions as a tool for individualized management and treatment. The significant characteristic of this study is that a new risk stratification system for patients with advanced hepatocellular carcinoma was built by applying multiple statistical methods. Based on this, the advantages and disadvantages of the new risk stratification system and AJCC staging system was explored, which were not mentioned in any other articles.
By univariate and multivariate Cox regression analysis, 10 variables (including age, AJCC stage, lymph node metastases, number of tumors, bone metastases, lung metastases, treatment, radiotherapy, chemotherapy, and marriage), which significantly affected CSS in patients with advanced HCC, and included in the nomogram. By measuring the range of scores of the incorporated variables on the nomogram score scale, treatments, lymph node metastases, chemotherapy, and radiotherapy were identified highly significant variables affecting the prognosis of patients with advanced HCC. Patients with early stage HCC did not have significant symptoms, and the majority of patients have developed advanced HCC when they were diagnosed (16). Systemic therapy was universally regarded as limited in its efficacy for patients with advanced HCC compared to other cancers (17). It was not until 2007 that sorafenib became the first drug proven to improve survival in advanced HCC. Results from several large randomized controlled trials have confirmed that compared to placebo, sorafenib prolonged the median survival time of patients with advanced HCC (18, 19), which was consistent with our findings. Recently, Llovet et al. (7) demonstrated that sorafenib combined with immunotherapy was superior to single agent efficacy, and the new findings were expected to improve the treatment paradigm for patients with advanced HCC (20–22). Local therapy was a bridge between liver transplantation and hepatectomy and was also the primary treatment modality for advanced HCC. Hanje et al. (23) reported 4-year survival rate was 92% for patients treated with liver transplantation after reaching Milan criteria at the descending stage. Salem et al. (24) reported an objective remission rate of 42% in patients treated locally. Although studies have confirmed the potential value of radiotherapy in specific patients with HCC (25, 26), especially in patients with early stage who were unable to be treated with transplantation or resection. However, the efficacy of radiotherapy in the treatment of advanced HCC remained controversial (27). Patients with advanced HCC were less likely to be tested for lymph node metastasis because of the inability to undergo surgery. Therefore, the prognostic effects of lymph node metastasis on patients with advanced HCC remained to be determined.
Tumor staging based on AJCC criteria was the predominant option for predicting prognosis in patients with advanced HCC. However, the effects of age, treatment, marital status, and other variables on patient prognosis were not considered in the traditional AJCC-based criteria (28, 29). We synthesized multiple variables affecting CSS in patients with advanced HCC (including demographic and clinicopathologic characteristics) into a nomogram. In addition, patients were divided into low-, middle-, and high-risk groups based on their total scores. On this basis, the power of the nomogram and the traditional AJCC-based criteria were compared, which other articles have not explored. The results of NRI, IDI, and C-index indicated that the nomogram had improved predictive power over tumor staging based on AJCC criteria alone. In addition, DCA demonstrated the clinical benefit and utility of our nomograms in predicting CSS over conventional staging systems. Remarkably, Kaplan–Meier analysis displayed significantly distinct CSS among the three risk groups, with considerably discriminatory power than the conventional staging system. In particular, the nomogram had a higher ability to distinguish between high-risk and low-risk groups than the traditional staging system, which can assist clinicians in individualizing the treatment and management.
Although the nomogram demonstrates outstanding utility, this study still has certain limitations. For example, the SEER database did not collected hematological indicators of patients, which therefore were not included in the screening. In addition, this study assessed these variables despite internal validation; our model lacks a multicenter clinical sample to perform external validation so as to provide more convincing evidence.
Conclusion
In conclusion, the nomogram exhibits powerful predictive performance, superior clinical benefit, and accurate predictive efficacy compared to the AJCC staging system. It can be applied to predict CSS in patients with advanced hepatocellular carcinoma.
Data Availability Statement
The datasets presented in this study can be found in online repositories. The names of the repository/repositories and accession number(s) can be found in the article/supplementary material.
Ethics Statement
The SEER database was publicly available and the private data of all patients have been eliminated from the SEER database. Therefore, informed consent and institutional review board approval were not required.
Author Contributions
Conceptualization: DY, YS, and FZ. Data curation: CC and KZ. Formal analysis: DY, XX, and YS. Writing—original draft: DY, YS, and FZ. Writing—review and editing: YD. All authors contributed to the article and approved the submitted version.
Conflict of Interest
The authors declare that the research was conducted in the absence of any commercial or financial relationships that could be construed as a potential conflict of interest.
Publisher’s Note
All claims expressed in this article are solely those of the authors and do not necessarily represent those of their affiliated organizations, or those of the publisher, the editors and the reviewers. Any product that may be evaluated in this article, or claim that may be made by its manufacturer, is not guaranteed or endorsed by the publisher.
References
1. Petrick JL, Florio AA, Znaor A, Ruggieri D, Laversanne M, Alvarez CS, et al. International Trends in Hepatocellular Carcinoma Incidence, 1978-2012. Int J Cancer (2020) 147(2):317–30. doi: 10.1002/ijc.32723
2. Cronin KA, Lake AJ, Scott S, Sherman RL, Noone AM, Howlader N, et al. Annual Report to the Nation on the Status of Cancer, Part I: National Cancer Statistics. Cancer (2018) 124(13):2785–800. doi: 10.1002/cncr.31551
3. Yang JD, Hainaut P, Gores GJ, Amadou A, Plymoth A, Roberts LR. A Global View of Hepatocellular Carcinoma: Trends, Risk, Prevention and Management. Nat Rev Gastroenterol Hepatol (2019) 16(10):589–604. doi: 10.1038/s41575-019-0186-y
4. Sung H, Ferlay J, Siegel RL, Laversanne M, Soerjomataram I, Jemal A, et al. Global Cancer Statistics 2020: GLOBOCAN Estimates of Incidence and Mortality Worldwide for 36 Cancers in 185 Countries. CA Cancer J Clin (2021) 71(3):209–49. doi: 10.3322/caac.21660
5. Marrero JA, Kulik LM, Sirlin CB, Zhu AX, Finn RS, Abecassis MM, et al. Diagnosis, Staging, and Management of Hepatocellular Carcinoma: 2018 Practice Guidance by the American Association for the Study of Liver Diseases. Hepatology (2018) 68(2):723–50. doi: 10.1002/hep.29913
6. Montella L, Palmieri G, Addeo R, Del Prete S. Hepatocellular Carcinoma: Will Novel Targeted Drugs Really Impact the Next Future? World J Gastroenterol (2016) 22(27):6114–26. doi: 10.3748/wjg.v22.i27.6114
7. Llovet JM, Kelley RK, Villanueva A, Singal AG, Pikarsky E, Roayaie S, et al. Hepatocellular Carcinoma. Nat Rev Dis Primers (2021) 7(1):6. doi: 10.1038/s41572-020-00240-3
8. Galle Peter R, Forner Alejandro, Llovet Josep M, et al. EASL Clinical Practice Guidelines: Management of Hepatocellular Carcinoma. J Hepatol (2018) 69(1):182–236. doi: 10.1016/j.jhep.2018.03.019
9. Siegel RL, Miller KD, Fuchs HE, Jemal A. Cancer Statistics, 2021. CA Cancer J Clin (2021) 71(1):7–33. doi: 10.3322/caac.21654
10. Connell LC, Harding JJ, Abou-Alfa GK. Advanced Hepatocellular Cancer: The Current State of Future Research. Curr Treat Options Oncol (2016) 17(8):43. doi: 10.1007/s11864-016-0415-3
11. Yu B, Liang H, Ye Q, Wang Y. Establishment of a Genomic-Clinicopathologic Nomogram for Predicting Early Recurrence of Hepatocellular Carcinoma After R0 Resection. J Gastrointest Surg (2021) 25(1):112–24. doi: 10.1007/s11605-020-04554-1
12. Yan B, Su BB, Bai DS, Qian JJ, Zhang C, Jin SJ, et al. A Practical Nomogram and Risk Stratification System Predicting the Cancer-Specific Survival for Patients With Early Hepatocellular Carcinoma. Cancer Med (2021) 10(2):496–506. doi: 10.1002/cam4.3613
13. Iasonos A, Schrag D, Raj GV, Panageas KS. How to Build and Interpret a Nomogram for Cancer Prognosis. J Clin Oncol (2008) 26(8):1364–70. doi: 10.1200/JCO.2007.12.9791
14. Huo TI, Ho SY, Ko CC. Nomogram for Surgical Hepatocellular Carcinoma: What Have We Missed? Liver Int (2021) 41(12):3034–5. doi: 10.1111/liv.15071
15. Balachandran VP, Gonen M, Smith JJ, DeMatteo RP. Nomograms in Oncology: More Than Meets the Eye. Lancet Oncol (2015) 16(4):e173–80. doi: 10.1016/S1470-2045(14)71116-7
16. Llovet Josep M, Ducreux Michel, Lencioni Riccardo, et al. EASL-EORTC Clinical Practice Guidelines: Management of Hepatocellular Carcinoma. J Hepatol (2012) 56(4):908–43. doi: 10.1016/j.jhep.2011.12.001
17. Anwanwan D, Singh SK, Singh S, Saikam V, Singh R. Challenges in Liver Cancer and Possible Treatment Approaches. Biochim Biophys Acta Rev Cancer (2020) 1873(1):188314. doi: 10.1016/j.bbcan.2019.188314
18. Kim TS, Kim JH, Kim BH, Lee YS, Yoo YJ, Kang SH, et al. Complete Response of Advanced Hepatocellular Carcinoma to Sorafenib: Another Case and a Comprehensive Review. Clin Mol Hepatol (2017) 23(4):340–6. doi: 10.3350/cmh.2016.0070
19. Chow PKH, Gandhi M, Tan SB, Khin MW, Khasbazar A, Ong J, et al. SIRveNIB: Selective Internal Radiation Therapy Versus Sorafenib in Asia-Pacific Patients With Hepatocellular Carcinoma. J Clin Oncol (2018) 36(19):1913–21. doi: 10.1200/JCO.2017.76.0892
20. Yau T, Park JW, Finn RS, Cheng AL, Mathurin P, Edeline J, et al. Nivolumab Versus Sorafenib in Advanced Hepatocellular Carcinoma (CheckMate 459): A Randomised, Multicentre, Open-Label, Phase 3 Trial. Lancet Oncol (2022) 23(1):77–90. doi: 10.1016/S1470-2045(21)00604-5
21. Vogel A, Qin S, Kudo M, Su Y, Hudgens S, Yamashita T, et al. Lenvatinib Versus Sorafenib for First-Line Treatment of Unresectable Hepatocellular Carcinoma: Patient-Reported Outcomes From a Randomised, Open-Label, non-Inferiority, Phase 3 Trial. Lancet Gastroenterol Hepatol (2021) 6(8):649–58. doi: 10.1016/S2468-1253(21)00110-2
22. Kudo M, Ueshima K, Yokosuka O, Ogasawara S, Obi S, Izumi N, et al. Sorafenib Plus Low-Dose Cisplatin and Fluorouracil Hepatic Arterial Infusion Chemotherapy Versus Sorafenib Alone in Patients With Advanced Hepatocellular Carcinoma (SILIUS): A Randomised, Open Label, Phase 3 Trial. Lancet Gastroenterol Hepatol (2018) 3(6):424–32. doi: 10.1016/S2468-1253(18)30078-5
23. Hanje AJ, Yao FY. Current Approach to Down-Staging of Hepatocellular Carcinoma Prior to Liver Transplantation. Curr Opin Organ Transplant (2008) 13(3):234–40. doi: 10.1097/MOT.0b013e3282fc2633
24. Salem R, Lewandowski RJ, Mulcahy MF, Riaz A, Ryu RK, Ibrahim S, et al. Radioembolization for Hepatocellular Carcinoma Using Yttrium-90 Microspheres: A Comprehensive Report of Long-Term Outcomes. Gastroenterology (2010) 138(1):52–64. doi: 10.1053/j.gastro.2009.09.006
25. Yang JF, Lo CH, Lee MS, Lin CS, Dai YH, Shen PC, et al. Stereotactic Ablative Radiotherapy Versus Conventionally Fractionated Radiotherapy in the Treatment of Hepatocellular Carcinoma With Portal Vein Invasion: A Retrospective Analysis. Radiat Oncol (2019) 14(1):180. doi: 10.1186/s13014-019-1382-1
26. Wahl DR, Stenmark MH, Tao Y, Pollom EL, Caoili EM, Lawrence TS, et al. Outcomes After Stereotactic Body Radiotherapy or Radiofrequency Ablation for Hepatocellular Carcinoma. J Clin Oncol (2016) 34(5):452–9. doi: 10.1200/JCO.2015.61.4925
27. Hong TS, Wo JY, Yeap BY, Ben-Josef E, McDonnell EI, Blaszkowsky LS, et al. Multi-Institutional Phase II Study of High-Dose Hypofractionated Proton Beam Therapy in Patients With Localized, Unresectable Hepatocellular Carcinoma and Intrahepatic Cholangiocarcinoma. J Clin Oncol (2016) 34(5):460–8. doi: 10.1200/JCO.2015.64.2710
28. Chun YS, Pawlik TM, Vauthey JN. 8th Edition of the AJCC Cancer Staging Manual: Pancreas and Hepatobiliary Cancers. Ann Surg Oncol (2018) 25(4):845–7. doi: 10.1245/s10434-017-6025-x
Keywords: advanced hepatocellular carcinoma, nomogram, cancer-specific survival, risk stratification, AJCC (TNM) staging system
Citation: Yang D, Su Y, Zhao F, Chen C, Zhao K, Xiong X and Ding Y (2022) A Practical Nomogram and Risk Stratification System Predicting the Cancer-Specific Survival for Patients With Advanced Hepatocellular Carcinoma. Front. Oncol. 12:914192. doi: 10.3389/fonc.2022.914192
Received: 06 April 2022; Accepted: 06 June 2022;
Published: 12 July 2022.
Edited by:
Khurum Hayat Khan, University College London, United KingdomReviewed by:
Zhendong Jin, Second Military Medical University, ChinaWeiqi Rong, Chinese Academy of Medical Sciences and Peking Union Medical College, China
Jingfeng Liu, First Affiliated Hospital of Fujian Medical University, China
Copyright © 2022 Yang, Su, Zhao, Chen, Zhao, Xiong and Ding. This is an open-access article distributed under the terms of the Creative Commons Attribution License (CC BY). The use, distribution or reproduction in other forums is permitted, provided the original author(s) and the copyright owner(s) are credited and that the original publication in this journal is cited, in accordance with accepted academic practice. No use, distribution or reproduction is permitted which does not comply with these terms.
*Correspondence: Youming Ding, ZGluZ3ltNjJAMTYzLmNvbQ==; Chen Chen, YXBwcmVjaWF0aW9uQHdodS5lZHUuY24=
†These authors have contributed equally to this work